RDP 2021-05: Central Bank Communication: One Size Does Not Fit All 4. Survey Results
May 2021
- Download the Paper 2,051KB
4.1 Summary
4.1.1 Survey respondents
The survey was sent to approximately 300 RBA staff and complete responses were received from 199. These respondents work in a range of areas including: IT, facilities management, the library, and various economic policy areas of the RBA. In terms of formal education in economics, about 45 per cent of our survey respondents had a bachelor's degree or above, 26 per cent had taken a high school course and about 30 per cent had not received any formal economic education. More details are in Table 2.
Count | Share | |
---|---|---|
By economic literacy (Q1) | ||
1 | 23 | 12 |
2 | 32 | 16 |
3 | 62 | 31 |
4 | 37 | 19 |
5 | 45 | 23 |
By education background (Q2) | ||
Bachelor's degree in economics or a related discipline | 47 | 24 |
Masters or PhD in economics or a related discipline | 42 | 21 |
High school economics course | 52 | 26 |
None | 58 | 29 |
By economic-related job (Q3) | ||
No | 111 | 56 |
Yes | 88 | 44 |
Source: Authors' calculations using survey results |
Based on the answers to questions 2 and 3 we divide the 199 respondents into 2 broad groups: economists and non-economists. We define economists as those who have a university-level education in economics and whose work involves economics. Non-economists are those who are either working in a role that is not economics related or have no university-level education in economics. Using this division, 71 respondents are defined as economists, accounting for 36 per cent of the respondents, and 128 are non-economists (64 per cent).
We also asked each person to self-assess their economic literacy using a scale ranging from 1 to 5. Almost every economist assessed their economic literacy as somewhat above average (4) or above average (5). This is in line with their reported education background in economics as having a bachelor's degree or above. By contrast, most non-economists assessed their economic literacy as average or lower. More details on the distribution of economic knowledge in our sample are shown in Figure 1. The proportion of economists, as defined above, in each category of economic literacy and education background are shown in Figure 1 through the colour of the bubbles.[12]
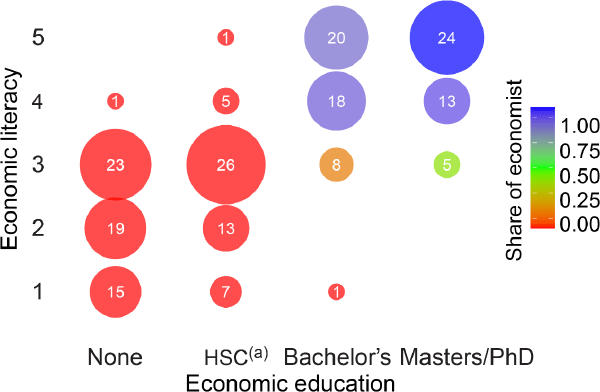
Notes:
Bubble size represents total respondents
(a) Higher School Certificate or equivalent
Source: Authors' calculations using survey results
4.1.2 Survey responses
We received 1,695 valid responses covering 833 unique paragraphs. The left panel of Figure 2 shows the distribution of those scores for reasoning and readability. As can be seen, the modal score for both is 4 although the mean rating for readability is higher than for reasoning. As discussed above, however, different respondents appear to have different default scores – for example, some default to a score of 4 while others default to 3 – so we decided to standardise the scores. The distribution of standardised scores is shown in the right panel of Figure 2.
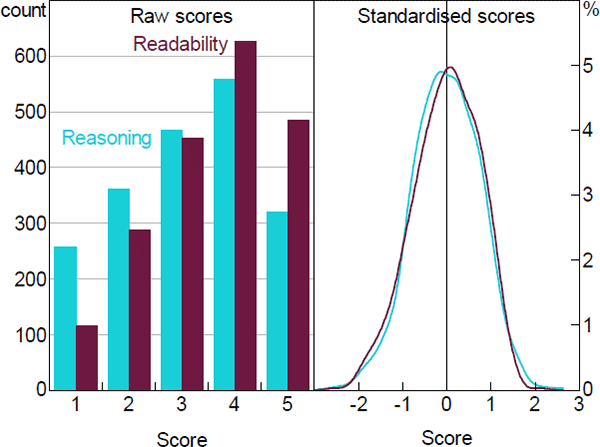
Note: The distribution panel is generated using the density function in R
Source: Authors' calculations using survey results
The fact that we have more valid responses than paragraphs partly reflects the design objective of getting both economist and non-economist ratings for the same paragraph. Of the 833 unique paragraphs, 465 were rated by both an economist and a non-economist, 53 by an economist only and 315 by a non-economist only. A second reason for the higher number of responses is that, due to the randomisation settings in the online survey, some paragraphs were rated by more than one person in each group. As shown in Figure 3, there were over 400 such paragraphs.
The overlapping ratings for a given paragraph, on the one hand, give us an opportunity to investigate the way people's ratings for a given paragraph vary. On the other hand, as mentioned above, they present a challenge in deciding the appropriate score for a given paragraph.
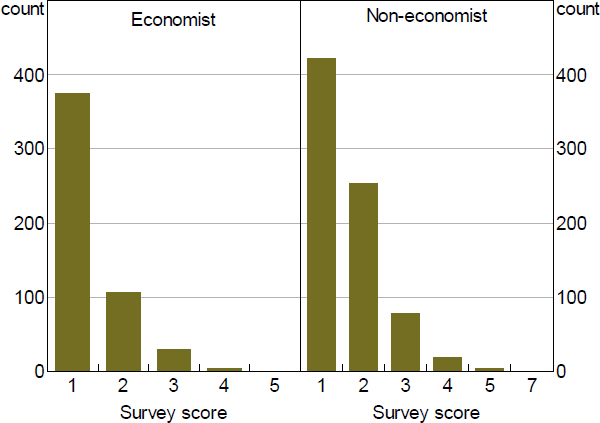
Source: Authors' calculations using survey results
Figure 4 shows the distribution of scores around the average score for a given paragraph that is rated by 2 or more people . We can see that the scores generally cluster around the average – indicating that there is a degree of agreement across respondents about the quality of a given paragraph.[13] The results suggest somewhat more disagreement among economists than non-economists and more dispersion in the ratings for reasoning than readability. The wider dispersion of reasoning scores is unsurprising given that reasoning is a harder concept to define and possibly more subjective in its evaluation. We leave the reader to make their own judgement about the reason for the greater disagreement among economists than non-economists.
As discussed above, one interpretation of the divergence is that each paragraph has one ‘true’ rating and each observation we have is a noisy signal about that true quality. Under this interpretation, taking the average rating would give the best signal about the true paragraph quality. An alternative interpretation is that different people – perhaps reflecting different backgrounds, knowledge or attitudes – have different interpretations of any given paragraph. Under this interpretation, divergence of ratings about a given paragraph is a signal that the paragraph is inherently ambiguous. That would suggest that each observation should be included in our dataset but, absent information about the reader that might explain the divergence in ratings across multiple readers, it would not be possible to correctly classify all of these paragraphs.
While exploration of the dispersion of ratings for a given paragraph could possibly reveal some subtle insights about effective writing for different audiences, it would also make our machine learning task considerably harder and require significantly more data than we have. Additionally, the single-peaked distribution of ratings for a given paragraph (Figure 4) suggest that assuming there is a true rating for any given paragraph is a reasonable assumption. Thus, we use the simple average of survey scores from multiple respondents as the final score for those repeatedly rated paragraphs.
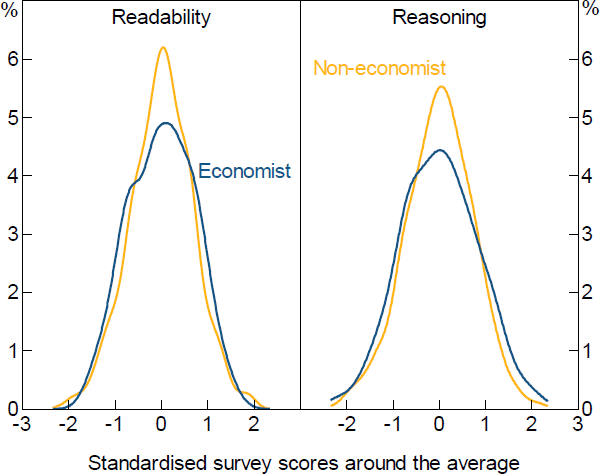
Source: Authors' calculations using survey results
4.2 Correlation between readability and reasoning
Figure 5 plots the distribution of reasoning and readability scores across sample paragraphs by text source. In general, all sources contain both high and low readability paragraphs as well as high and low reasoning ones and all combinations thereof. This wide distribution will be useful for training the machine-learning algorithm as it means we have examples of all possible types of paragraphs to help predict the quality of out-of-sample text.
Overall, what is most striking is the lack of correlation between readability and reasoning. There is only a slight positive correlation between reasoning and readability. The lack of close correlation between the scores emphasises how multidimensional writing is. This leads to one of our key observations: Trying to summarise the quality of a paragraph or document with any one metric must inevitably miss many important features of writing.
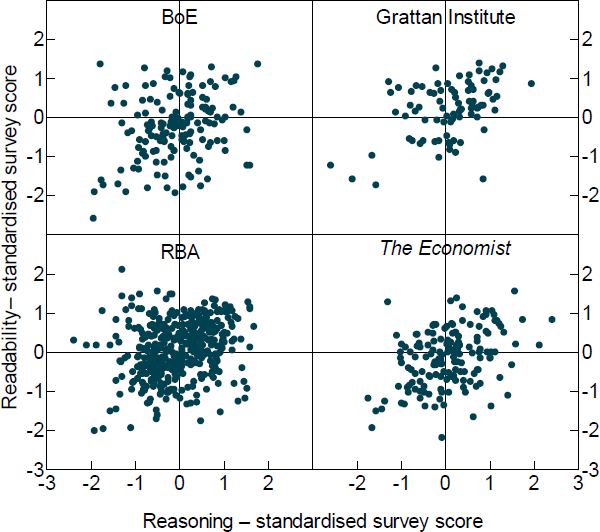
Source: Authors' calculations using survey results
4.3 Correlation with readability formula
Simple readability scores, such as the FK grade level, have been widely used in the literature as a measurement of text quality. However, as noted in Section 2, there are a number of criticisms of their accuracy. Given that we have a direct evaluation of readability from our survey, it is interesting to look at the correlation between one of these measures, the FK grade level, and our survey responses. Figure 6 shows the correlation between our 2 measures of text quality and the FK grade level.
We can see a significant, but weak, correlation between the FK grade level and readability scores from the survey. The coefficient is of the expected sign and the value of –0.021 indicates that an increase of 10 in the FK grade level is associated with a readability rating that is 0.21 standard deviations lower. However, the value of R2 is only 0.008. This is a very low value, indicating that the FK score may be a poor indicator of the readability of any given sample paragraph. There is no significant correlation between the FK grade level and the reasoning scores.
These findings lend some support to the criticisms of, at least, the FK grade level but are likely much more widely applicable. In addition to the fact seen above that single metrics can miss important aspects of communication, widely used readability metrics may not even measure readability well.
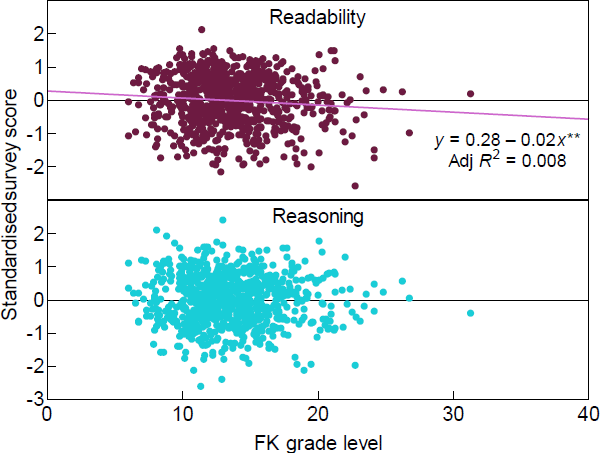
Note: ** denotes statistical significance at the 1 per cent level
Source: Authors' calculations using survey results
4.4 Economists versus non-economists
A key focus of this project is to look at how different audiences understand the same piece of text. This question of audience is another dimension that is missing from simple readability metrics – people with similar education levels but different backgrounds will understand communication differently. Given our focus, we look at the difference between economists and non-economists.
Figure 7 shows the correlation between economist and non-economist ratings for a given paragraph – each dot represents a paragraph that was rated by both an economist and a non-economist. As can be seen, there is very little correlation.
One possible explanation is that non-economists find the language used unfamiliar. As noted by Andy Haldane (2017): ‘“Inflation and employment” leaves the majority of [non-economists] cold. “Prices and jobs” warms them up. “Annuity” deep freezes [non-economists], whereas “investment” thaws’. Nonetheless, while jargon and word choice may explain some of the difference, the variation is more likely to arise due to the different ways people comprehend a paragraph based on their background knowledge. As noted by Goldman and Rakestraw:
Generally, in situations of high content knowledge, readers will be less reliant on structural aspects of the text than in low content knowledge situations because they can draw on preexisting information to create accurate and coherent mental representations. In low content knowledge situations, processing may be more text driven, with readers relying on cues in the text to organize and relate the information and achieve the intended meanings (Goldman and Rakestraw 2000, p 313).
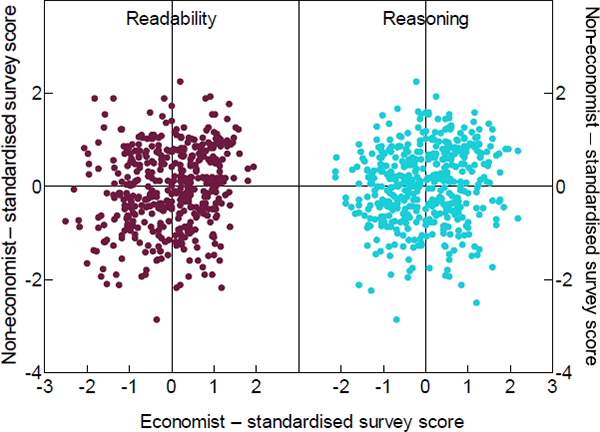
Source: Authors' calculations using survey results
In other words, economists have sufficient background to understand the significance of pieces of information in a text without needing explicit pointers to their relationships. Conversely, non-economists may need the relationships between pieces of information spelt out explicitly through the structure of the text. A surprising implication is that non-economists might prefer longer sentences (with correspondingly higher FK grade levels) that provide the necessary structure for their understanding. They might find it harder to understand shorter sentences if these just stick to the facts and assume the reader can fill in the linkages. Alternatively, short sentences with sufficient explicit contextual information and a lot of attention to coherence between sentences might also achieve the same goal. More generally, this leads to a second key insight: one size does not fit all.
Footnotes
Some responses appear anomalous (which is one of the reasons we adopted the definition we use). For example, one respondent reported holding a bachelor's degree in economics but having a very low economic literacy. We think this response (and a couple of others who rated their economic literacy as average despite also reporting holding a postgraduate degree in economics) points to the possibility that some respondents may have overlooked the term ‘in economics’ when answering the question on education background. That is, they hold a bachelor's or masters degree, but not in economics. [12]
We tested normality using the Shapiro-Wilk test, and the results suggested that the distributions, except for the economist–readability data, are not significantly different from a normal distribution. For the full results, please refer to the online supplementary information. [13]