RDP 2020-08: Start Spreading the News: News Sentiment and Economic Activity in Australia 2. How Do We Measure News Sentiment?
December 2020
- Download the Paper 1.48MB
Sentiment is hard to measure as it is not directly observed. Common survey-based measures of sentiment typically ask respondents about their beliefs about current economic conditions as well as expectations for future economic conditions. We take a different approach and construct a proxy for sentiment based on the language used by journalists in news reports on the economy.
There are two general approaches for quantifying sentiment in text. The dictionary-based approach relies on pre-defined lists of words with each word either classified as positive, negative, neutral, or indicating uncertainty. The machine learning approach predicts the sentiment of any given set of text after training models with a large set of text that has been assigned sentiment ratings by human readers. For example, models have been developed using social media data, such as Twitter, that provide text that is combined with user feedback to identify the sentiment of the posts. This approach is better able to capture the nuances in human language but it is more complex and less transparent.
We follow the simpler dictionary-based approach to construct our NSI. The NSI measures the net balance of words used by journalists that are considered to be ‘positive’ and ‘negative’. When journalists use more positive words and/or fewer negative words, this is an indicator that sentiment is rising in the economy. This type of index has been used before for other regions, such as the United States, Japan and Europe (see, for instance, Fraiberger (2016); Scotti (2016); Larsen and Thorsrud (2018) and Buckman et al (2020)).
The raw data used in constructing the NSI consist of daily news extracted from Dow Jones Factiva. Each article listed in the database includes metadata such as publication time, language, region and category. After removing duplicates and selecting only articles that are written in English by Australian media outlets to cover the Australian economy, the resulting dataset includes around 300,000 articles. The data span the period from September 1987 to June 2020 and the sample covers more than 600 newspapers, though The Australian, The Sydney Morning Herald and The Australian Financial Review are the main sources.
Common steps in the natural language processing literature are taken to clean the raw dataset before analysis: numbers, punctuation marks, white spaces and common stop words are removed from each article. All words are then reduced to their respective ‘stem’, which is the part of a word that is common to all of its inflections (for example, ‘performs’, ‘performing’, and ‘performed’ are reduced to ‘perform’).
To measure the sentiment of a set of text, that is, whether or not the news is positive or negative, the Loughran–McDonald dictionary is used. This is a word list specific to the domain of economics and finance (see Loughran and McDonald (2011) for more details). The NSI is constructed by counting the number of times that negative and positive words appear in the cleaned text of articles.[2] A news uncertainty index (NUI) is also constructed by counting the number of articles that contain uncertain words.[3] The most common positive, negative and uncertain words in March 2020 are shown in Figure 1.
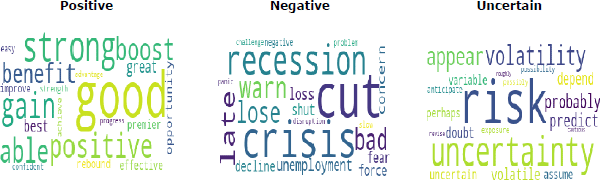
Sources: Authors' calculations; Dow Jones Factiva
To construct the time series of the NSI, the articles are sorted by date of publication and the data are divided into blocks of time, which could be a day. For each time period (t), we compute the sentiment index by subtracting the count of negative words from the count of positive words and then dividing by total word count:
Between September 1987 and March 2020 there are, on average, around two more negatives than positives for every 100 words in the articles, with a standard deviation of less than 1 word. We standardise the indicator to have a mean of zero and a standard deviation of one.
Footnotes
The individual words are not weighted by the degree of positivity or negativity.[2]
The uncertainty index is therefore measured on a different basis to the sentiment index. This is mainly due to practical reasons – the terms in the uncertainty dictionary do not appear very frequently within articles. This approach to measuring uncertainty using text analysis is equivalent to that used by others in the literature (e.g. Moore 2017).[3]