RDP 2019-06: The Effect of Mortgage Debt on Consumer Spending: Evidence from Household-level Data 4. Does Mortgage Debt Affect Spending?
July 2019
- Download the Paper 1,513KB
4.1 Identification
As discussed above, there are several challenges associated with identifying the effect of mortgage debt on spending. To see this, consider a simple regression of spending on mortgage debt at the household level:
where the dependent variable (Eh,t) is the level of non-housing spending of household h in year t, and the key variable of interest is the level of owner-occupier mortgage debt (Dh,t).
First, reverse causality is a problem. A household may choose to spend more than it earns, implying more borrowing and hence a higher stock of debt.[10] To partly mitigate this, we estimate the relationship between households' current spending and the previous year's mortgage debt. It is also worth noting that reverse causality would drive a positive bias in the coefficient estimate, implying that it would be harder to pin down a potential negative debt overhang effect.
Second, omitted variables can influence both spending and mortgage debt. For example, an increase in households' income expectations may lift both their intention to spend and their desire to take on debt. Alternatively, an increase in risk aversion may discourage both spending and borrowing. Again, this is most likely to induce a positive correlation between spending and debt and attenuate any negative debt overhang effect.
Some of the challenges in identifying the causal effect of mortgage debt on spending are highlighted in an ‘event study’ around the time of home purchase. Most home purchases in Australia are financed at least in part through mortgage debt. When a household buys a home for the first time (or when they trade up to a larger/higher-quality home) they typically take on a large amount of debt. And when they buy a new home they also tend to spend more on either furnishing the new home or renovating their existing home for sale.
Given the HILDA Survey is longitudinal in nature we can observe the spending, income and debt of a household both before and after home purchase (Figure 4, left panel). In the year of home purchase, there is a notable jump in spending on durable goods. In the year after home purchase, durable goods spending returns to the pre-purchase level. This pattern of home purchase-related debt accumulation and spending in one year followed by lower spending the next year would lead us to empirically find a negative correlation between current spending and lagged mortgage debt. But the relationship would not be causal – it would be driven by an omitted variable – the decision to buy a new home. This would be similar to the ‘spending normalisation’ hypothesis (Andersen et al 2016). In contrast to the findings of Gross (2017) for the United States, we find little evidence of a fall in non-durable spending around the time of home purchase.
Another notable feature of this event study is the rise in household income in the years leading up to the purchase. We find that this is partly due to households working longer hours, presumably to save for a home deposit. But, in line with Gross (2017), it also seems to reflect a ‘selection effect’; the households that choose to buy a new home are those that received an increase in income (through, say, a promotion or bonus). Either way, this event study highlights the need to control for factors that influence both debt and spending behaviour, such as the age, income, wealth and labour force characteristics of the household.
A similar event study can be undertaken around the year in which households fully pay off their mortgage. Under the PIH, household spending should not respond to anticipated changes in scheduled debt. We would expect spending to remain constant. At odds with this prediction, and suggesting that debt may constrain spending, we find that durables spending increases in the year that households fully repay their mortgage debt and non-durables spending increases in the years following (Figure 4, right panel). The increase in spending is larger than both the observed increase in disposable income and the average mortgage payment prior to paying off the debt, which suggests that the spending response cannot be fully explained by cash flow effects.
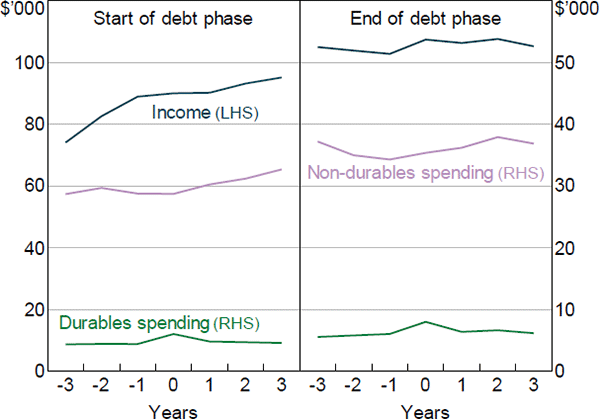
Notes: 2017/18 dollars; households with owner-occupier housing debt
Sources: ABS; Authors' calculations; HILDA Survey Release 17.0
4.2 Household Fixed Effects Model
To deal with the issues highlighted above, we exploit the rich longitudinal information available in the HILDA Survey. To test whether debt levels directly influence spending, we first estimate the following regression model, which we refer to as the fixed effects (FE) model:
This model includes the lagged level of owner-occupier mortgage debt (Dh,t − 1) as the key variable of interest, household disposable income (Yh,t) and the lagged reported home value (Ah,t − 1). The model also includes a set of control variables (Xh,t), to summarise the other observed determinants of spending, including factors associated with a household's permanent income, such as age, education and labour force status of the household reference person.[11] The model includes a household fixed effect which captures household characteristics that determine spending but are plausibly invariant over time (e.g. degree of impatience and risk aversion). Estimates are presented with and without the household fixed effect to gauge the importance of these characteristics. Our results are robust to including year fixed effects.
4.3 Instrumental Variables Model
To further alleviate any endogeneity concerns about unobserved time-varying confounding factors (such as changes in income expectations or local labour demand shocks), we also adopt an instrumental variables approach. For this, we exploit the home purchase history of each owner-occupier household in the survey. We use information on the timing of their most recent home purchase relative to other home owners in the same postcode as an instrument for the level of owner-occupier housing debt held by the household. The logic behind this instrument is that households living in the same area are exposed to identical time-varying local demand shocks, but differ in their debt holdings based on when they happened to purchase their home. The instrument should therefore be correlated with outstanding mortgage debt but uncorrelated with differences in spending for borrowers in the same postcode other than their level of debt.[12]
To take a hypothetical example, suppose there are two households that own identical homes in the same street. The only difference between them is that household A bought before a local housing boom happened while household B bought after. It is plausible that household A borrowed less (in dollars) than household B because housing prices in the area were lower when household A made their purchase decision. The timing of the purchase decision should not affect the spending of household A relative to household B over and above its impact on their respective levels of indebtedness.
Australia experienced a large housing price boom in the early 2000s. The timing of this boom varied by state, generally starting in 2001 in the larger capital cities of Sydney, Melbourne and Brisbane, and in 2002 in other capital cities. We can think of the households that bought just before the boom in housing prices as the ‘lucky’ households while the comparable households that bought just after the boom are ‘unlucky’. To the extent there are other differences between households that bought before and after the boom that affect their consumption behaviour (such as the level of housing wealth), we can only control for observable differences in the model.
To gauge the relevance of the instrument, we compare the average initial debt holdings of households purchasing homes just before and just after the boom (Figure 5). There is a clear jump in average mortgage debt for those households that were ‘unlucky’ to buy just after the housing boom compared to the ‘lucky’ households that bought just before the boom.[13] Based on the weak identification test in Stock and Yogo (2005), the instrument is found to be significantly correlated with the household's current holdings of mortgage debt (even after the age of loan is taken into account).
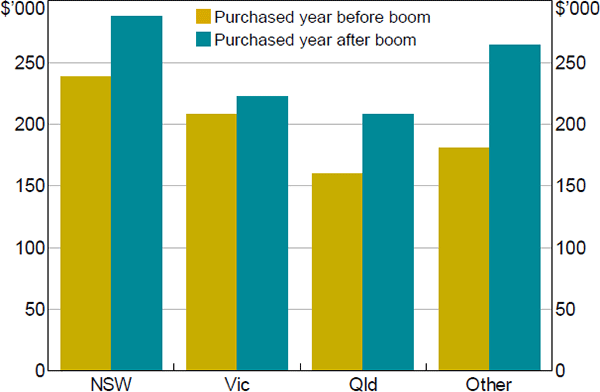
Notes: Average debt levels in 2017/18 dollars; debt levels are observed one year after purchase, housing price boom is assumed to have occurred in 2001 for NSW, Vic and Qld and 2002 for other states and territories
Sources: ABS; Authors' calculations; HILDA Survey Release 17.0
This second model is based on a two-stage least squares regression, which we refer to as the instrumental variables (IV) model:
where most of the variables are as denoted before and p denotes the postcode in which household h lives. The main difference is that owner-occupier housing debt is estimated in the first-stage regression using a dummy variable (BOOMhp) as an instrument. The dummy variable takes the value of one if a household purchased their home after the early 2000s housing price boom in the state of purchase and zero otherwise.[14] To control for the location choice of the household in their home purchase decision, we drop the household fixed effects and instead include postcode fixed effects in the model. Otherwise the timing of home purchase (the ‘birth cohort’ of the mortgage) will be absorbed by the household fixed effect. Thus, whilst this approach may help to alleviate endogeneity concerns by controlling for unobserved time-varying factors such as local demand shocks, the resulting estimator will include the effects of household debt on spending both within households (over time) and between households (at a point in time).
4.4 Results
4.4.1 Baseline models
Table 1 presents the key results from estimating the OLS, FE and IV models for durables, non-durables and total spending (see Appendix F for full table of results).
Model | |||||||||||
---|---|---|---|---|---|---|---|---|---|---|---|
Non-durables spending 2006–17 |
Durables spending 2006–10 |
Total spending 2006–10 |
|||||||||
OLS | FE | IV | OLS | FE | IV | OLS | FE | IV | |||
Lagged mortgage debt | >−0.00 (0.77) |
−0.01** (0.03) |
−0.15*** (<0.00) |
−0.07* (0.05) |
−0.10* (0.10) |
−0.80*** (<0.00) |
−0.01 (0.11) |
−0.03*** (0.01) |
−0.20*** (<0.00) |
||
Income | 0.26*** (<0.00) |
0.10*** (<0.00) |
0.28*** (<0.00) |
1.02*** (<0.00) |
0.36** (0.02) |
1.09*** (<0.00) |
0.30*** (<0.00) |
0.09*** (<0.00) |
0.33*** (<0.00) |
||
Lagged home value | 0.21*** (<0.00) |
0.17*** (<0.00) |
0.32*** (<0.00) |
0.55*** (<0.00) |
0.28 (0.28) |
1.01*** (<0.00) |
0.26*** (<0.00) |
0.11** (0.04) |
0.38*** (<0.00) |
||
First-stage: Boom dummy | 0.46*** (<0.00) |
0.45*** (<0.00) |
0.45*** (<0.00) |
||||||||
Household FE | No | Yes | No | No | Yes | No | No | Yes | No | ||
Postcode FE | No | No | Yes | No | No | Yes | No | No | Yes | ||
Observations | 21,460 | 21,460 | 21,460 | 6,622 | 6,622 | 6,622 | 6,622 | 6,622 | 6,622 | ||
Notes: The sample excludes non-indebted households in the previous year and the top and bottom 1 per cent of income growth, spending growth and housing price growth; controls include household income, lagged home value, age dummies, education dummies, number of children, number of adults, marital status, unemployment and not in the labour force status; standard errors are clustered by household; *, **, *** represent statistical significance at the 10, 5 and 1 per cent levels, respectively; p-values are in parentheses Sources: Authors' calculations; HILDA Survey Release 17.0 |
We find that higher mortgage debt reduces household spending across all specifications. The overall similarity between our FE estimates and the OLS estimates suggests that unobservable time-invariant variables do not play a major role after controlling for a range of household socio-economic characteristics. The FE model specification suggests that the effect of debt on spending is relatively small with a 10 per cent increase in debt reducing households' non-durables, durables and total spending by 0.1, 1.0 and 0.3 per cent, respectively. The stronger response of durables relative to non-durables spending is consistent with the common finding of larger wealth effects for durables spending.
The IV model estimates provide further evidence that the negative debt overhang effect is not driven by unobservable, time-varying factors such as local demand shocks. The first-stage regression estimates indicate that our instrument is relevant and that households that bought after the boom held mortgage debt levels that were about 45 per cent higher than comparable households living in the same area that bought before the boom. The second-stage IV estimates are more economically significant than those in the FE model and suggest that a 10 per cent increase in debt lowers non-durables, durables, and total spending by 1.5, 8.0 and 2.0 per cent, respectively. While the IV model may help to further remove time-varying confounding factors, it does not account for unobserved household fixed effects. We therefore take the FE model estimates as our benchmark estimates for the remainder of the paper.
In these regressions, we control for household income and the lagged value of gross housing assets. As expected, higher household income and housing prices raise spending. In contrast to the estimated effect on debt, the OLS coefficient estimates on income and home value are considerably larger than the FE estimates, suggesting that unobserved time-invariant factors are significant drivers behind the positive relationship between spending and both income and housing prices. For example, it may be the case that impatient households spend more and buy more expensive homes than patient households, and this partly explains the positive link between spending and home values.
We also estimate all three models using three additional debt measures (the debt-to-income, debt-to-assets and debt service-to-income ratios) that are commonly used in the literature. The results are presented in Appendix E. Using the IV model, we find some evidence that higher debt ratios negatively affect household spending, consistent with a number of papers that have found evidence of a significant negative relationship between these debt ratios and spending. In particular, we find that a 10 percentage point increase in the debt-to-assets ratio or debt-servicing ratio significantly reduces total household spending by 0.1 per cent.
4.4.2 Gross versus net housing wealth
In the previous regressions, we control for gross housing wealth. Another possibility is to control for net housing wealth (housing equity), equal to the difference between the reported value of the home and any outstanding mortgage debt. This allows us to directly test whether the composition of household balance sheets matters for spending. The results can be found in Table 2.
Overall, our estimates of the effect of debt on household spending are broadly unchanged when we control for households' housing equity instead of the value of their home. Importantly, this implies that households lower their spending when the gross value of both their debt and their assets increases. In other words, we find that a deepening of household balance sheets is associated with less household spending, even if it is not associated with rising net indebtedness. This directly violates conventional consumption theories such as the PIH that assume the composition of a household's balance sheet does not affect consumption (Garriga and Hedlund 2017). Our results suggest a small, and occasionally negative, effect of lagged housing equity on spending. When using contemporaneous housing equity, we recover the expected positive effect.
Model | |||||||||||
---|---|---|---|---|---|---|---|---|---|---|---|
Non-durables spending 2006–17 |
Durables spending 2006–10 |
Total spending 2006–10 |
|||||||||
OLS | FE | IV | OLS | FE | IV | OLS | FE | IV | |||
Lagged mortgage debt | 0.02*** (<0.00) | –0.01 (0.19) | –0.12*** (<0.00) | –0.01 (0.74) | –0.08 (0.17) | –0.75*** (<0.00) | 0.02* (0.06) | –0.03** (0.04) | –0.17*** (<0.00) | ||
Income | 0.33*** (<0.00) | 0.12*** (<0.00) | 0.34*** (<0.00) | 1.19*** (<0.00) | 0.38** (0.01) | 1.22*** (<0.00) | 0.38*** (<0.00) | 0.10*** (<0.00) | 0.38*** (<0.00) | ||
Lagged housing equity | <0.00*** (0.01) | >–0.00 (0.85) | –0.01*** (<0.00) | 0.01 (0.47) | 0.01 (0.59) | –0.05*** (<0.00) | 0.01** (0.01) | <0.00 (0.31) | –0.01*** (<0.00) | ||
First-stage: Boom dummy | 0.47*** (<0.00) | 0.44*** (<0.00) | 0.44*** (<0.00) | ||||||||
Household FE | No | Yes | No | No | Yes | No | No | Yes | No | ||
Postcode FE | No | No | Yes | No | No | Yes | No | No | Yes | ||
Observations | 21,460 | 21,460 | 21,460 | 6,622 | 6,622 | 6,622 | 6,622 | 6,622 | 6,622 | ||
Note: See notes to Table 1 Sources: Authors' calculations; HILDA Survey Release 17.0 |
Footnotes
Households in Australia can use their mortgage debt to buy a consumption item (e.g. car, holiday) through their offset or redraw facilities; see Appendix B for more information. [10]
See Table D1 for definitions of the variables used in the regression models. We identify the household reference person as the individual with the longest household membership, the highest personal income, or the highest age, in that order. [11]
We assume that households make mortgage prepayments at the same speed across the age of the loan. [12]
The jump in average household debt levels is unique to the reference years chosen around state housing price booms. We find little difference in the average debt levels when using alternative reference years. [13]
Purchased after 2001 in New South Wales, Victoria and Queensland, and after 2002 in all other states and territories. [14]