RDP 2023-09: Does Monetary Policy Affect Non-mining Business Investment in Australia? Evidence from BLADE 4. Results with Tax Data on Actual Investment
December 2023
- Download the Paper 1.74MB
Figure 1 shows the results for the full sample. A 100 basis point contractionary monetary policy shock leads to a decline in investment both at the intensive and extensive margin. The decline in both margins is statistically significant (at the 95 per cent level), and peaks after around two years. In terms of magnitude, the share of firms investing falls by a little less than 5 percentage points two to three years after the shock, and the average investment for previously investing firms falls by around 10 per cent.
Interpreting the relative importance of the two channels is difficult, given their different nature. To try to provide some insight we take a simple, back-of-the-envelope approach to quantification. In 2016, the average investment by a firm investing both in the current and previous period (those in the intensive margin estimation) was around $273,000. Taking the peak 10 per cent decline in investment, this equates to $27,300 less per continuing firm. And these firms account for around 17 per cent of the population, so average firm-level investment falls by $4,700. For the extensive margin, average investment for firms investing this period, but not in the previous period, is $52,000. Multiplying this by the peak 5 per cent decline in the probability of investing lowers average firm-level investment by $2,600. So using this simple framework, the intensive margin is about twice as important as the extensive margin.[5]
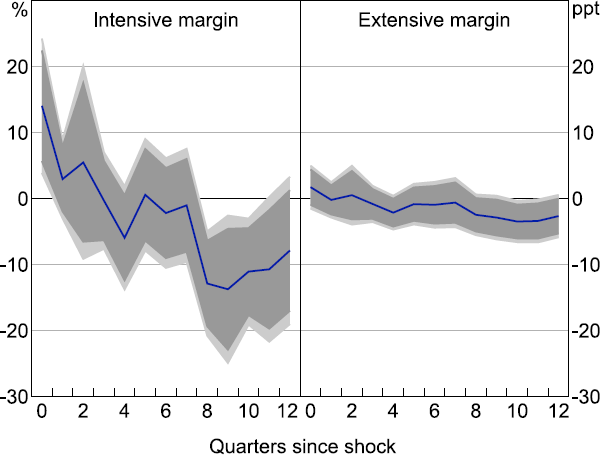
Note: Lighter shaded areas show 95 per cent confidence interval; darker show 90 per cent confidence interval.
Sources: ABS; Authors' calculations.
The timing of the lags with which monetary policy affects investment is consistent with macro models of the Australian economy such as the RBA's MARTIN (Ballantyne et al 2019) and DSGE (Gibbs, Hambur and Nodari 2018) models. However, the response of investment in our results is somewhat larger to that suggested by MARTIN and the DSGE.
One explanation for the larger effects in our results could be the fact that we use nominal measures of investment if tighter monetary policy lowers the price of investment goods. However, when using the aggregate investment deflator we find little evidence that contractionary monetary policy shocks lower the price of investment goods (Figure B3). That said, there is a slight decline in the point estimates, suggesting our estimates of the effect of monetary policy on real investment may be slightly overstated.
Another explanation could be the use of microdata. To consider this, we can estimate the equivalent local projection model using (log) aggregate real non-mining investment (gross fixed capital formation) from the national accounts. We estimate this over a longer sample given the longer sample available (from 1994 to match the shock availability). We again include lagged controls for GDP and CPI growth but have no firm-level controls.
Using the aggregate data, the magnitude and timing of the effects of monetary policy on investment are consistent with aggregate results from the microdata, and are much larger than those from the macroeconomic models (Figure 2). This finding is consistent with Durante et al (2022) and Holm, Paul and Tischbirek (2021) for the euro area and Norway, respectively. It indicates the results are not simply driven by our use of microdata. The finding is robust to changing the number of lags in the controls, adding current controls (to loosen the inherent assumption of no contemporaneous effect from the shock) and accounting for breaks or trends in the level of investment. The results are also similar if we incorporate the Beckers (2020) shocks into a structural VAR with the components of GDP, ordering the shock first, as suggested by Plagborg-Møller and Wolf (2021) (Figure B1).
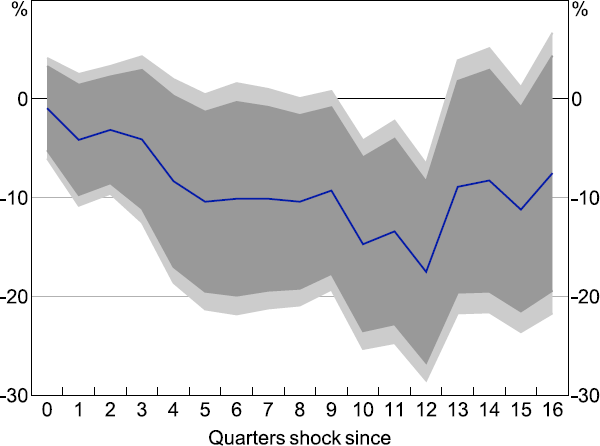
Note: Lighter shaded areas show 95 per cent confidence interval; darker show 90 per cent confidence interval.
Sources: ABS; Authors' calculations.
As a final robustness check, we also consider a shock measure derived from high-frequency changes in yields around the time of the RBA's monetary policy announcements (30 minutes before and 90 minutes after) in our microdata regressions. In particular, we use the level shock from Hambur and Haque (2023), which focuses on high-frequency changes in the policy rate.[6] Doing so yields a similar profile for the response of investment to monetary policy, but the size of the response is much smaller and there is less evidence of a significant effect (Figure C7). In part this likely reflects differences in the implicit persistence of these shocks, which can make it hard to compare across shock measures.
More generally, part of the difference between our results and those from existing macroeconomic models likely reflects differences in the implied persistence of the shock. For example, the decline in the cash rate following the shock in Beckers (2020) is much more persistent than that implied by the Taylor rules in MARTIN or the RBA's DSGE model. As such, we may not be comparing like for like. Following an initial shock of 100 basis points, the cumulative change in the cash rate is almost 900 basis points in Beckers (2020) and only around half that in the DSGE, while in MARTIN interest rates very quickly fall and overshoot such that the cumulative change in interest rates over three years is near zero.
Still, taken together the results provide strong evidence that contractionary monetary policy leads to lower investment, though the exact magnitude of the effect is uncertain and can vary depending on the modelling approach and the shock measured used.
4.1 Results by firm size and age
We next explore whether our microdata results differ by firm size or age. This is important for two reasons. First, any difference in results along these dimensions can help us to think about the transmission channels of monetary policy. Small and young firms may tend to be more financially constrained, with lower levels of free cash flow. As such, if these firms' investment decisions are more responsive to monetary policy, this may suggest that monetary policy affects investment by influencing financing constraints.[7] Second, over the past decade there has been a sharp decline in firm entry and exit, meaning that there are fewer young firms in the economy. If young firms' investment is more responsive to monetary policy, this change in firm demographics could weaken the effects of monetary policy and potentially help to explain the subdued levels of non-mining investment evident in the 2010s.
Focusing first on size, Figures 3 and 4 show the results from splitting the sample into small (< 20 staff), medium (20–199 staff) and large firms (200+ staff). Overall, there is some statistical evidence that the extensive margin plays a slightly more important role for small firms, consistent with these firms investing less regularly than larger firms, but aside from this the differences appear small (see Figure C1).
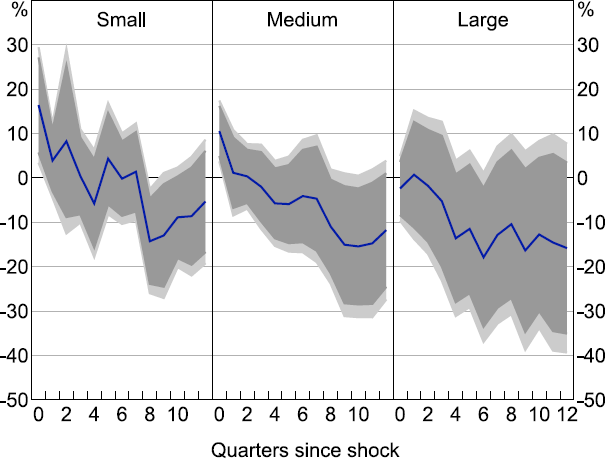
Note: Lighter shaded areas show 95 per cent confidence interval; darker show 90 per cent confidence interval.
Sources: ABS; Authors' calculations.
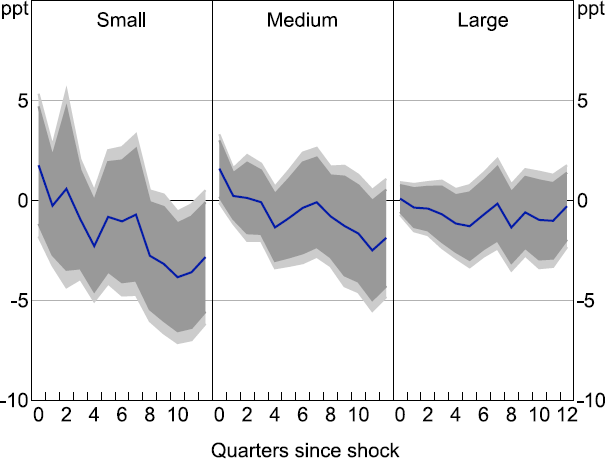
Note: Lighter shaded areas show 95 per cent confidence interval; darker show 90 per cent confidence interval.
Sources: ABS; Authors' calculations.
Considering age, the results are again similar across young (0–5 years) and old (5+ years) firms with no evidence of a significant difference in the effects (see Figures 5 and C2). This suggests that the decrease in the share of young firms in the economy witnessed over the past decade is unlikely to have caused aggregate investment to become less responsive to monetary policy.
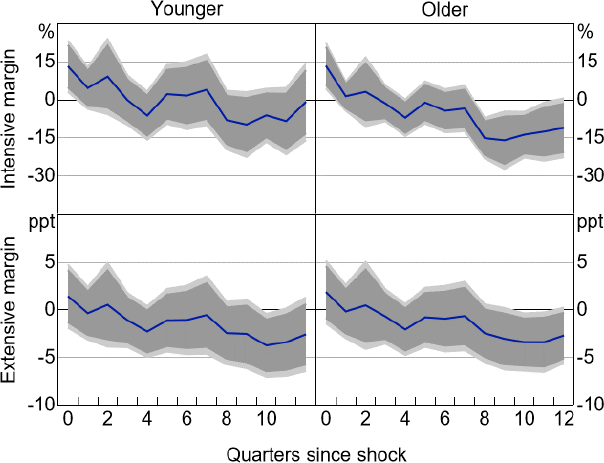
Note: Lighter shaded areas show 95 per cent confidence interval; darker show 90 per cent confidence interval.
Sources: ABS; Authors' calculations.
This finding is somewhat different to Cloyne et al (forthcoming), who find that younger firms in the United States, particularly non-dividend-paying ones, respond more strongly to shocks, reflecting greater financing constraints. One difference is that they focus on listed firms, whereas we focus on a much larger sample of firms; as such, more of the older firms could be financially constrained in our sample. They also focus on a higher age cut-off of 15 years for young firms.[8]
4.2 Results by leader versus non-leader
Another way to think about firm size is to define industry ‘leaders’, the largest 5 per cent of firms in a 4-digit ANZSIC industry, and ‘non-leaders’. This allows us to abstract from inherent differences in firm sizes across industries and to focus on the largest firms in each industry.
We use this split to think about two key aspects of monetary policy pass-through. The first is whether evidence that firms have sticky hurdle rates should be taken as evidence that they do not respond to monetary policy. For example, survey evidence suggests that firms' hurdle rates do not respond to interest rate changes (Lane and Rosewall 2015; Edwards and Lane 2021). Some of these surveys are focused on industry leaders. If leader firms' investment does respond to monetary policy shocks, this would indicate that either monetary policy affects their investment through channels other than by lowering the marginal cost of investment, or that the survey responses and actual behaviour differ somewhat.
The second aspect is whether monetary policy could influence the degree of competition in the economy. In particular, recent work in the United States has found that expansionary monetary policy disproportionately supports investment for leader firms, thus undermining competitive pressures in the economy (Kroen et al 2022; Liu et al 2022). We can test whether this is also the case in Australia.
We define leaders based on sales over the previous year. Overall, leaders' investment is slightly less responsive to monetary policy than that of non-leaders, particularly on the extensive margin. But the differences are not statistically significant and are fairly small (Figure 6). And while the responses of leader firms are not significant, this appears to reflect the much smaller sample size, which leads to larger standard errors, rather than a smaller mean response.[9]
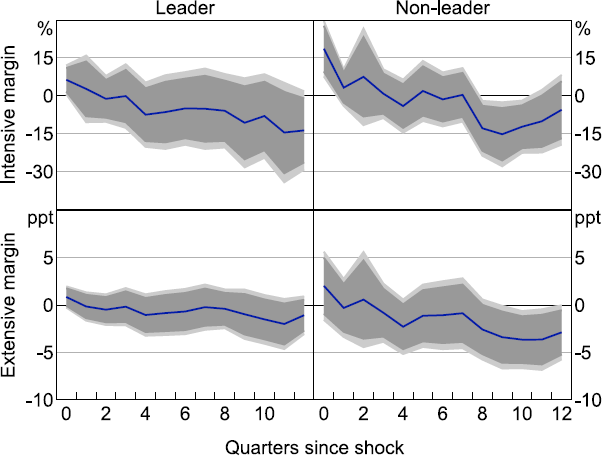
Note: Lighter shaded areas show 95 per cent confidence interval; darker show 90 per cent confidence interval.
Sources: ABS; Authors' calculations.
This finding has two implications. First it suggests that survey evidence that firms', particularly large firms, hurdle rates are sticky should not be taken as evidence that monetary policy does not affect their investment. Monetary policy still affects their investment, though this could be through channels other than lowering their marginal cost of capital. It could also be the case that the surveyed and actual responses differ or that hurdle rates do respond to changing interest rates even if they are sticky. This would be consistent with findings in La Cava (2005) and Hambur and La Cava (2018) that listed companies do change their investment as their cost of finance changes. Our results do not differentiate between these explanations, but they do indicate that evidence of sticky hurdle rates should not be taken as evidence that monetary policy does not affect investment.
The finding also provides some evidence that expansionary monetary policy does not weaken competitive pressures in Australia, as leader firms do not expand their investment by more than other firms following an expansionary shock, in contrast to the US findings. There are several explanations for why our findings differ to the US findings. One is that we use a larger, more representative dataset, which includes more small firms. In contrast, the US work includes only listed firms. This could be important as non-listed firms could be more credit constrained, and so be more responsive to monetary policy.[10]
To consider this, we perform the same exercise, but focus on listed firm data from Morningstar, using the annual dataset outlined in Nguyen (2022). We apply the cleaning method implemented in Kroen et al (2021), removing outliers from the sample. For this analysis we consider both a flow measure of gross investment (as above), and changes in the capital stock (as in Kroen et al (2021)). Unfortunately, the short sample available (from 2001) makes it difficult to draw conclusions around significance.[11] However, focusing on the point estimates there is some tentative evidence that leaders' investment is more responsive to shocks when focusing on larger, listed companies (Figure B2).[12] Taken at face value this could suggest the Kroen et al (2021) result may not hold when considered in a larger, more representative sample, though this would be better tested using comparable US data.
Other potential explanations for the different findings could include the different structure of corporate borrowing in Australia, or the fact that Australia is a small open economy, and so larger firms with access to international capital markets may be relatively more exposed to overseas credit conditions.
4.3 Results by financial dependency
One of the key channels through which monetary policy is thought to affect investment is by influencing financial constraints. This can be through influencing the value of collateral that firms have available to pledge for loans – the so-called financial accelerator channel (Bernanke and Gertler 1995) – or more generally by influencing firms' cash flow and liquidity constraints, or credit supply.
To better understand the financial constraint channel, we explore whether firms that may be more subject to financial constraints, frictions, or are generally more reliant on borrowing, respond differently to other firms following a monetary policy shock. There is a large literature examining the effects of financing frictions and credit availability on firm investment and growth. This literature measures financial frictions and credit supply in numerous ways, and there is no one agreed upon ‘best’ measure of frictions or financial dependence.
For example, Ottonello and Winberry (2020) focuses on leverage and finds that less leveraged firms respond more to monetary policy shocks, which they argue reflects the fact that they have a flatter marginal cost curve. Jeenas (2023) instead focuses on liquidity, and finds that less liquid firms respond more strongly, consistent with monetary policy easing constraints. Finally, Cloyne et al (forthcoming) assume non-dividend-paying young firms are constrained, and find that such firms are more responsive to monetary policy. More generally, thresholds for constraints could differ across firm age, size and industries. And there are difficulties in differentiating between distressed firms, who may be unresponsive, and constrained firms, who may be responsive. As such, we take a different approach.
As our first approach we use industry-level measures of external finance dependence. Specifically we use the measures of US industry-level finance dependence from Demmou, Stefanescu and Arquie (2019), who update Rajan and Zingales’ (1998) approach, and assume these funding structures apply to Australian firms. We split firms based on whether they were in one of the most dependent sectors (top quartile) or least dependent (bottom quartile). Demmou et al (2019) produce these measures on an ISIC Revision 4 basis, and we map them to ANZSIC 4-digit industries.
This measure is constructed using information on the average of the funding requirements of US firms. Using US-based metrics has some advantages and some disadvantages. The obvious disadvantage is that industries may finance themselves differently in Australia and the United States. Still, there are several advantages: using an external dataset limits potential endogeneity concerns; the US data are based on a much longer sample than we have available; the US-based metrics are well-documented and have been used in numerous different studies, making them a robust and comparable set of indicators.
As expected, in sectors that are more dependent on external finance firms' investment appears slightly more responsive to monetary policy shocks (Figure 7). These differences are statistically significant at the 10 per cent level, indicating that more financially dependent sectors are somewhat more responsive, though the magnitude of the difference is not large.[13]
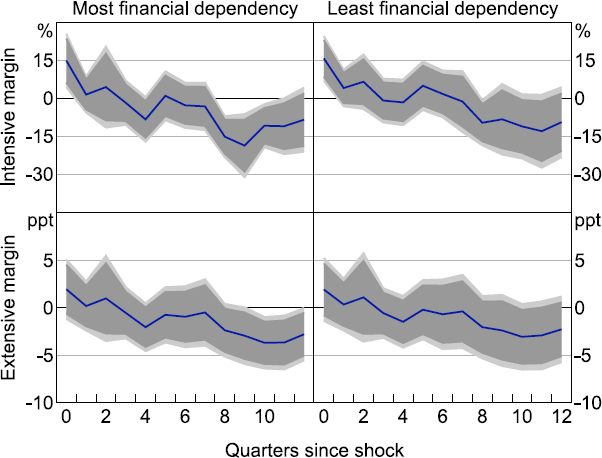
Note: Lighter shaded areas show 95 per cent confidence interval; darker show 90 per cent confidence interval.
Sources: ABS; Authors' calculations.
As a second approach, we take a more direct firm-level financial constraint measure. Rather than base this on balance sheet metrics, we exploit the fact that our data are integrated with survey data from the ABS Business Characteristics Survey, which includes a question asking whether a lack of funds has hampered the firm's operations.[14] While this is only available for a subset of generally slightly larger and older firms, it still provides a large sample for analysis. We also increase the effective size of the sample by classifying firms as constrained if they ever report being constrained in the survey, and unconstrained if they never report being so.[15]
Figure 8 shows the results. The effects for the constrained and unconstrained groups are not significantly different from zero. That said, the effects do appear slightly larger for constrained firms on the extensive margin, and this is borne out in tests of statistical significance, with constrained firms having larger falls in investment at the 10 per cent level (Figure C4). That said, again the differences are not necessarily large economically.
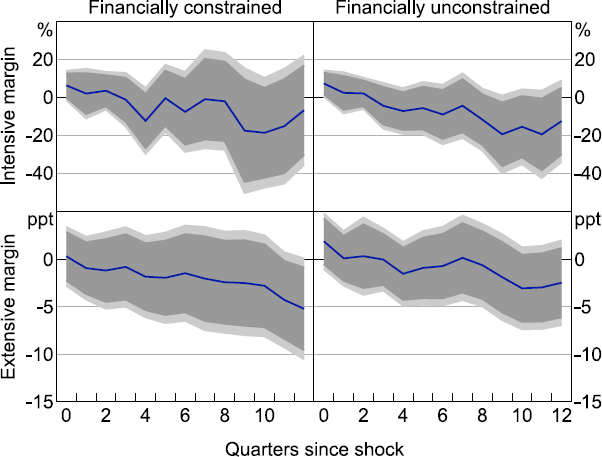
Note: Lighter shaded areas show 95 per cent confidence interval; darker show 90 per cent confidence interval.
Sources: ABS; Authors' calculations.
Overall, we do find some evidence that firms in sectors that are more reliant on external finance have larger investment responses following a monetary policy shock, and that firms that appear financially constrained are more responsive. This is consistent with monetary policy influencing firms' investment in part by influencing financial constraints.
Footnotes
This is not surprising given continuing investors account for around 90 per cent of investment. Note that this approach does not take any account of the fact that firms with lower levels of investment may tend to have higher percentage increases, or differences between the marginal firm incentivised to invest by policy and the average firm that moves from non-investing. As such, it should be seen as a very simple indication, not a precise quantification. [5]
This measure is only available for a slightly shorter sample, starting in 2004. [6]
That said, many young firms may not have established banking relationships or may be heavily constrained. This could make them less responsive to monetary policy if policy does not loosen their credit constraints (Casiraghi, McGregor and Palazzo 2021). [7]
While we could explore this higher age cut-off, it is quite high compared to standard definitions of young firms used in administrative data. Moreover, our data has some issues with truncation of ages around the year 2000, with a disproportionate share of firms being born that year. So while we can be confident that for most of the sample (i.e. from around 2005 onwards) firms are at least five years old, the same cannot be said for assessing whether or not they are fifteen years old. [8]
The results are robust to using the current or previous period, and quarterly or annual sales. [9]
Morlacco and Zeke (2021) argue that these dynamics could come through intangible investment, such as investing in branding and customer base. Unfortunately our data are not well suited to test this hypothesis. [10]
We have a very small number of clusters, which can lead to incorrect inference with standard errors. Various approaches have been examined to adjust for small numbers of clusters, but given this regression is not our main focus we do not adopt these. [11]
Results are shown including industry by year fixed effects and removing firm-growth controls to be consistent with Kroen et al (2021). However, the results are very similar if the specification is changed to be similar to our BLADE regressions. [12]
See Figure C3 for test of significance of differences. [13]
The survey covers around 8,000 firms per year. It is a census of firms with more than 300 employees and a stratified random sample for firms with less than 300 employees. Stratification by industry and business size is implemented to produce data that are representative of Australian businesses. The ABS does not provide the sample weights so our analysis is based upon the unweighted data. Firms with fewer than 300 employees are included on a rotating five-year basis. [14]
Splitting firms based on whether they reported being constrained in the previous year provides a similar result. We do not use constrained in the current year as the policy shock itself may influence the firm's constraint status. [15]