RDP 2023-06: Firms' Price-setting Behaviour: Insights from Earnings Calls 5. Measuring Tense
September 2023
- Download the Paper 1.6MB
As highlighted above, our newly constructed indices contain valuable information for assessing current economic conditions. This notwithstanding, when studying some aspects of firms' price-setting behaviour – as we do in the next section – it is useful to focus exclusively on discussions that are forward looking. To do this, we apply temporal tagging to paragraphs. This is a challenging task with no dominant methodology established in the field of NLP. Because of this, we trial two approaches: a rule-based tense tagging method and a zero-shot text classifier approach.[11]
Inspired by Byrne et al (2023), the first approach employs a rule-based tool called the SUTime tense tagger (Chang and Manning 2012). The tagger can recognise a wide range of temporal representations through dictionary look-up and date–time detection rules. It maps phrases such as ‘the future’ and ‘currently’ to the relevant tense tag. It also detects a variety of date formats, either absolute formats such as ‘date/month/year’ or relative dates such as ‘next Wednesday’. The outputs that SUTime generates are either categorical flags indicating past, present or future, or numerical dates. As we are interested in only the forward-looking tendency of a paragraph, we determine the tense by observing only the ‘future’ tag or whether the numerical date detected is before or after the reference date.
An alternative approach is additionally explored. It involves using the zero-shot text classifier to organise paragraphs into two categories: future or forward looking and now present tense or past. The classifier is configured to perform binary classification to ensure the output labels are mutually exclusive. The category keywords are selected through trial and error such that the best classification results are achieved.
To evaluate tense-tagging accuracy, we manually assigned tense labels to 100 randomly sampled paragraphs to create a set of ‘true labels’. We then construct a confusion matrix and calculate the tense prediction accuracy for SUTime and the zero-shot classifier, respectively (Figure 8). The confusion matrix shows the performance of each algorithm (SUTime on the left and the zero-shot classifier on the right), with the rows representing the actual values and the columns showing predicted classifications. The confusion matrix shows that the two models perform similarly, with both producing high true negative rates. SUTime correctly classifies 58 out of 71 paragraphs as in the past/present tense, while the zero-shot classifier correctly classifies 63 out of 71 paragraphs. That is, both models perform well in filtering out paragraphs that are in the past or present tense. The models do not perform as well when classifying paragraphs into the future tense. SUTime performs marginally better with 17 out of 29 correct classifications, so we proceed with SUTime as our tense tagger. A comparison of the aggregate forward-looking indices is shown in Figure C1.
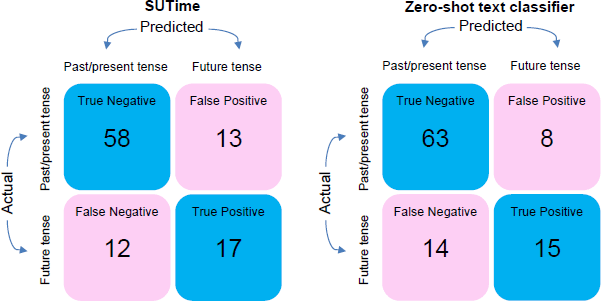
Sources: Authors' calculations; Reuters.
Footnote
We also experimented with a third (and ultimately inferior) approach, which was to restrict our analysis to only the Q&A sections of the transcripts. Previous work has shown that analysts' questions have more forward-looking information content than managements' remarks, possibly because they are more interested in the outlook and less likely to put a positive spin on things (Wigglesworth 2022). [11]