RDP 2020-02: The Distributional Effects of Monetary Policy: Evidence from Local Housing Markets 4. Heterogeneity in the Sensitivity of Local Housing Prices to Monetary Policy
February 2020
- Download the Paper 1,879KB
4.1 Identification
We estimate Equation (1) using a combination of panel regression techniques and local projections (Jordà 2005). We refer to this as the ‘unrestricted model’:
where:
- is the log difference in the real dwelling price index for region i from quarter t to quarter t + h, where h [1,16] quarters.
- is a region-specific dummy. This controls for time-invariant factors within each local housing market, such as the level of local amenities or distance to the coast. The estimate of this dummy is allowed to change for each horizon h.
- represents the quarterly percentage point change in the cash rate target.
- is a vector of controls for both local and aggregate market conditions.
Equation (2) is estimated separately for each local housing market and at each time horizon. The resulting estimates of the coefficients provide direct estimates of the sensitivity of local housing prices to monetary policy.
The controls include variables that capture macroeconomic conditions, including GDP growth, the terms of trade and the unemployment rate, as well as lagged changes in the cash rate. This addresses concerns that the local housing market sensitivities could be driven by differential sensitivities to aggregate shocks, rather than interest rates per se.
We experimented with including controls for both local housing and labour market conditions, such as rental growth and the local unemployment rate. However, the sample period (and frequency) for which the local level controls are available is somewhat restricted. Over the smaller sample period, the local controls do not meaningfully change the key results.[12]
4.2 Results
The estimates of the coefficients (at a two-year horizon) for all local areas are plotted as a density in Figure 2. As the density shows, the bulk of the coefficient estimates are negative, implying that housing prices fall in response to tighter monetary policy in most local housing markets. However, as is also clear, there is substantial heterogeneity in the response of local housing prices to changes in monetary policy.
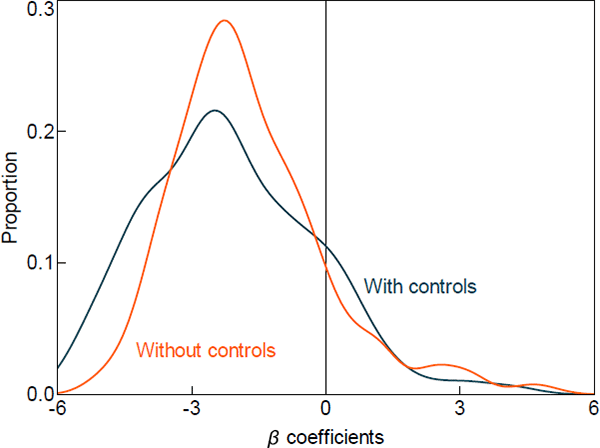
Sources: ABS; Authors' calculations; CoreLogic data; RBA
But how much of this heterogeneity is due to monetary policy per se, and how much is due to the fact that some regions may be more sensitive to aggregate shocks, such as the national business cycle, and monetary policy is responding endogenously to these aggregate shocks? We can examine this by comparing the distributional effects of monetary policy when estimated both with and without controls for macroeconomic conditions. We find that the densities are reasonably similar, though the inclusion of controls for macroeconomic conditions produces slightly more negative responses and ‘flattens’ the distribution (Figure 2). This suggests that some of the heterogeneity across local housing markets is due to varying sensitivity to the business cycle and that the effects of monetary policy on local housing prices are stronger (and more dispersed) when we account for this.
We can also look at how this distribution evolves over time and hence examine the heterogeneity in the dynamics of local housing prices in response to monetary policy (Figure 3). The responses follow a similar pattern and are reasonably close immediately after the change in monetary policy. However, the distribution widens over time. For example, in the median region, housing price falls peak at 2.3 per cent after two years in response to a 100 basis point increase in the cash rate. But at the 25th and 75th percentiles, housing prices fall by 0.9 per cent and 3.5 per cent in response to the same increase in the cash rate.[13] This suggests that the dynamics of housing prices vary substantially across time and local housing markets in response to interest rate changes.
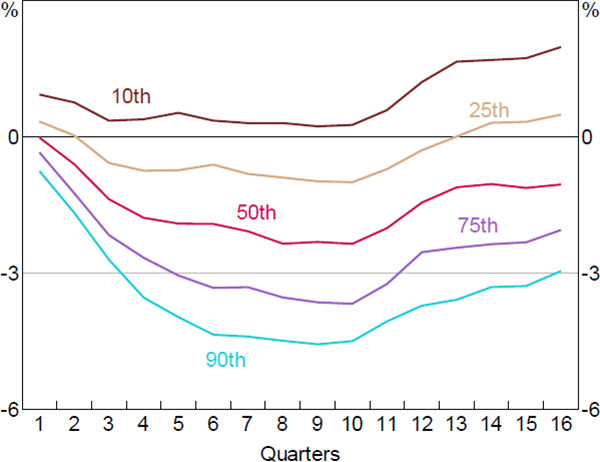
Sources: ABS; Authors' calculations; CoreLogic data; RBA
4.3 Inspecting the Mechanism
Given the wide cross-sectional distribution of housing price responses to monetary policy, we examine factors that might be driving these differences. Guided by the theoretical framework, we consider a range of possible drivers of the link between monetary policy and growth in housing prices, including both housing demand and supply-side factors.
- Housing price expectations: existing research suggests that expectations could play an important role in driving the heterogeneous effects of monetary policy (e.g. Kaplan, Moll and Violante 2018; Ben-David, Towbin and Weber 2019). As such, we consider a range of variables that are likely to be associated with household expectations about future capital gains. This includes the extent to which housing supply is constrained by geography or regulation (Fischer et al 2018; Kendall and Tulip 2018). For instance, expected housing price appreciation may vary by region due to differences in available land supply (Himmelberg et al 2005; Glaeser, Gyourko and Saiz 2008). But we also consider demand-side factors. For example, research indicates that expectations of housing prices are linked to local economic conditions and household demographics, including income (Niu and van Soest 2014). Also, housing investors may have different expectations of capital gains on housing relative to the rest of the population, so we also include estimates of the share of investors in the population.
- Discount rates: there is empirical evidence that discount rates vary across (and even within) individuals (Frederick, Loewenstein and O'Donoghue 2002). So it is plausible that discount rates vary across regions. We consider a range of variables that may be associated with household impatience, and therefore discount rates. Some studies suggest that households that are less patient also have relatively low levels of net wealth (or high levels of debt) (e.g. Carroll et al 2017). Therefore, their discount rates may be relatively sensitive to short-term interest rates, such as the cash rate.[14] It follows that regions that are more indebted may be more sensitive to changes in monetary policy. We proxy for indebtedness across regions based on the share of households with mortgage debt and the share of households that report spending more than 30 per cent of their income on housing costs. More generally, there can be a disconnect between private discount rates and market interest rates because of personal preferences and experiences, including exposure to market instruments (Glaeser, Gottlieb and Gyourko 2012). For example, richer individuals could be more sensitive to changes in market interest rates as changes in market interest rates directly affect their optimal asset allocation.
To assess the relative importance of each factor we take the housing price responses to a 100 basis point increase in the cash rate at an eight-quarter horizon in our unrestricted model ( from Equation (2)) and explore the cross-sectional correlation across regions between the housing price responses and various factors.
where
- is a vector of housing price responses, estimated from Equation (2), for each of 284 local housing markets at an eight-quarter horizon;[15]
- Q represents an n by p matrix of p explanatory variables (including factors associated with housing price expectations, discount rates etc), and represents a p by 1 vector of coefficients;
- is a constant and is a white noise error.
We take two complementary approaches to study these cross-sectional correlations. First, we take a ‘data-driven approach’ and implement some variable selection methods, including elastic net and least angle regression (LAR).[16] We do this given it is difficult to know which variables to include in the determinants of the local housing price responses and we want to avoid overfitting and selection bias. We evaluate over 80 different local area characteristics. Most of the variables are derived from regional data produced by the Australian Bureau of Statistics (ABS) or longitudinal household survey data obtained from the Household, Income and Labour Dynamics in Australia (HILDA) Survey.[17] The optimal model is chosen based on 10-fold cross validation and selecting the maximum penalty (elastic net)/minimum step (LAR) that produces a mean squared error within one standard deviation of the minimum mean squared error.
Second, we take a ‘judgement-based approach’ and examine multivariate OLS regressions for a select number of variables across a range of time horizons.[18] The variables chosen are informed by both theory and the data-driven methods. A ‘general-to-specific’ approach is applied to narrow down the variables to those that were more statistically significant.
The variables selected by the data-driven approach are presented in Table 1.[19] The variables and estimates chosen by the judgement-based approach are presented in Table 2. Based on these two approaches, the factors determining the sensitivity of local housing prices to monetary policy can be loosely tied to the theoretical framework outlined earlier and grouped under the following broad headings:
-
Housing price expectations:
- housing supply conditions (e.g. average price level; share of the value of the home due to land)
- investor density (e.g. share of investors in the population)
-
Discount rates:
- income per capita
- mortgage debt (e.g. share of households with mortgage debt; share of households paying more than 30 per cent of their income on housing costs).
To be clear, this framework provides a useful way of summarising the determinants of the sensitivity of local housing prices to monetary policy. But some variables could fit under either heading. For example, average income may affect both people's expectations about housing prices and their degree of impatience. Similarly, housing investors may have different expectations and discount rates to the broader population.
Table 1: Determinants of Housing Price Responses Selected by all methods – elastic net and LARTheme Elastic net Least angle regression Sensitivity Income Median total income More Median employee income Median employee income More Hand-to-mouth households as a share of working-age population Less Share of individuals claiming low income tax offset(a) Share of individuals claiming low income tax offset(a) Less Share of working-age population earning negative income Less Low income tax offset per capita Less Share of individuals earning
allowances, earnings, tips or
director fees(a)Less Mortgage debt Share of households with high housing servicing costs More Mortgage servicing ratio Mortgage servicing ratio More Housing servicing ratio Housing servicing ratio More Housing supply conditions Average sales price Average sales price More Average price growth Less Investor concentration Rent interest deductions per capita Rent interest deductions per capita More Share of individuals claiming rent
interest deductions(a)Share of individuals claiming rent
interest deductions(a)More Investor density as a share of the working-age population More Investor density as a share of the population More State/territory Victoria Victoria Less Queensland Queensland More Western Australia More Notes: Details about the construction of the various measures are outlined in Appendix A
(a) ‘Share of individuals’ is based on individuals recorded by the ATO based on tax statisticsSources: ABS; Australian Taxation Office; Authors' calculations; CoreLogic data; DSS and Melbourne Institute (2018); RBA
Table 2: Determinants of Housing Price Responses OLS regression estimates at various horizonsVariable Horizon 1-year 2-year 3-year Median sale price (log level, dollars) −0.287
(−0.688)0.077
(0.138)0.524
(0.737)Share of value determined by land (%) −0.012**
(−2.000)−0.011
(−4.880)−0.022**
(−4.642)Rent servicing share (%) 0.029
(0.724)0.043
(0.768)0.153**
(2.155)Mortgage servicing share (%) −0.133***
(−3.339)−0.236***
(−4.880)−0.300***
(−4.642)Mortgagor rate (%) 0.008
(0.806)0.004
(0.286)−0.025
(−1.585)Outright ownership rate (%) 0.033*
(1.824)0.054***
(2.710)0.114***
(4.596)Investor density (%) −0.036**
(−2.432)−0.052***
(−3.300)−0.095***
(−4.944)Employee income (log level, dollars) −2.746**
(−2.138)−3.181*
(−1.723)−0.697
(−0.300)Price growth (percentage change) 0.008***
(3.528)0.012***
(4.215)0.015***
(4.539)R-squared 0.332 0.427 0.414 No of observations 274 274 274 Notes: State dummies for Victoria and Western Australia and constant are omitted from the table; standard errors are robust; *, ** and *** denote statistical significance at the 10, 5 and 1 per cent levels, respectively; t-statistics are in parentheses
Sources: ABS; Australian Taxation Office; Authors' calculations; CoreLogic data; RBA
The results to these two complementary approaches yield a few key findings.
First, both the model selection and OLS results suggest that factors associated with housing supply conditions are important. Specifically, the effect of monetary policy on housing prices is stronger in local housing markets in which supply conditions are tight. For instance, the average sales price is chosen by both selection methods and is likely associated with the availability of housing supply. However, the coefficients are insignificant in the OLS regressions. But when the proportion of housing value determined by land is included in the regressions this points to tighter supply conditions producing larger housing price responses to monetary policy changes.
Second, investors appear to play an important role in housing market dynamics. Specifically, investor-dense regions appear to be more sensitive to changes in monetary policy. The OLS regression estimates indicate that an area with 10 percentage points more investors would experience around a half a percentage point larger fall in housing prices two years after a 100 basis point increase in the cash rate. This may indicate that, relative to the broader population, housing investors have different expectations of capital gains on housing. Alternatively, due to personal preferences, housing investors may have discount rates that are more closely tied to short-term interest rates.
Third, regions with relatively high average incomes display stronger responses to monetary policy. The OLS estimates indicate that a 1 percentage point increase in income is associated with a 3.2 basis points larger negative response of housing prices two years after a percentage point increase in the cash rate. This suggests that high income areas are more sensitive to monetary policy because their discount rates are more closely tied to market interest rates (Glaeser et al 2012).[20] Alternatively, because average incomes are likely to be correlated with the value of local amenities, it may be that areas with relatively high average incomes are those in which housing supply is tight (and these supply conditions are not fully captured by the average housing price level).
Fourth, there is some evidence that, in more indebted regions, housing prices are more sensitive to changes in interest rates. The model selection methods find that areas with relatively high housing costs (either mortgage payments or rents) are more responsive to monetary policy. In addition, the OLS model suggests that these results are driven by high mortgage servicing ratios, as opposed to rental payments.
Lastly, we find evidence that the housing markets in Western Australia (more sensitive) and Victoria (less sensitive) have systematically different responses to monetary policy compared to other states and territories. This may reflect how monetary policy responds to activity in different states. In particular, the Reserve Bank focuses on aggregate measures of economic activity and inflation in setting monetary policy. For example, monetary policy may be less sensitive to activity in Western Australia as its business cycle could be tied to factors that are unrepresentative for the rest of Australia, such as global demand for commodities. Conversely, monetary policy could be more sensitive to activity in Victoria where the business cycle is more representative of the aggregate business cycle. This response could drive systematically different responses of housing prices to monetary policy across states. Specifically, when economic activity is high in Victoria this likely corresponds to increasing housing prices in Victoria. And if monetary policy were to tighten in response to this economic activity it would serve to curb the housing price movements in Victoria; resulting in more muted housing price responses. At the same time, if Western Australia's economy and housing market were weaker, the change in monetary policy could have a larger effect on the Western Australian housing cycle. That is, monetary policy could offset housing cycles in one state or territory and amplify them in another.
These variables are not an exhaustive list of possible determinants. Indeed, the R-squared on these regressions range from 33 to 43 per cent, suggesting that the OLS models do not fully capture the variation in housing price responses. It is possible that the local housing price sensitivities depend on factors in a nonlinear fashion (e.g. interactions between debt and income). Such nonlinear effects have not been considered. Moreover, the results are not necessarily causal (e.g. because the level of mortgage debt depends on past changes in monetary policy). And the results are quite sensitive to the choice of variables, the time horizon and the controls that are included in the baseline regression. But the results do indicate that housing supply conditions are not the only factor that matters to the sensitivity of local housing prices.[21]
Footnotes
This provides some tentative evidence that the effects of monetary policy on housing price growth are not coming through current rents but instead through its effect on housing price expectations and discount rates. But the lack of data, and hence degrees of freedom, reduces the precision of the estimates. [12]
These estimates are smaller than estimates for other recent Australian studies. For example, Saunders and Tulip (2019) and the RBA's MARTIN model estimate a peak effect of around 9 per cent and 4 per cent respectively, following a temporary 100 basis point change in the cash rate. However, the Saunders and Tulip estimates are based off a temporary three-year increase in the cash rate. [13]
In the model, the coefficient would be positive and relatively large, which implies a larger negative effect of monetary policy on housing prices. [14]
The sample is smaller than the population of SA3 regions due to missing data. [15]
More information on these methods can be found in Hastie, Tibshirani and Friedman (2009). [16]
The ABS regional data are typically drawn from the Census or Australian Taxation Office (ATO) data. The full list of variables can be found in Appendix A. [17]
To fully account for the dynamics of housing price responses to monetary policy it is important to consider different time horizons. It is possible that a variable may be important at one horizon but not at others. Though model selection methods could be used we do not think that would produce interpretable results across multiple horizons. For more consistent and interpretable results across time horizons we take signal from our model selection results while applying judgement and focusing on OLS estimates. [18]
Elastic net is less discriminate than LAR. This is because elastic net is designed to allow for ‘groupings’, which allows for multiple highly correlated variables to be selected by averaging their effects, as opposed to choosing one over the rest. [19]
It is also consistent with US research that suggests that financing constraints have a limited effect on housing prices because constrained individuals comprise a small share of the housing market. Instead, it is the unconstrained individuals that drive housing prices (Kiyotaki, Michaelides and Nikolov 2011). [20]
It is worth noting that there are many variables that were tested and proved to not be correlated with the local housing price sensitivity. These variables include those that have been identified in past research as being potentially important, such as the age structure of the population (Wong 2019) and the industry composition of each region (Vespignani 2013). [21]