Research Discussion Paper – RDP 2016-06 Jobs or Hours? Cyclical Labour Market Adjustment in Australia
September 2016
1. Introduction
A firm's demand for labour is derived from the demand for its output. During a downturn in demand, firms can reduce their use of labour by reducing either the number of workers they employ (the ‘extensive margin’ of labour adjustment) or by reducing the hours worked by their employees (the ‘intensive margin’ of labour adjustment).[2] From an economy-wide perspective, whether adjustment occurs through the number of employees or average hours worked by each employee has implications for the costs of a downturn. If workers have their hours reduced but remain employed, many of the costs associated with unemployment, such as skill atrophy and reliance on government assistance, are mitigated. The nature of adjustment also has implications for the measurement of spare capacity in the economy. If significant adjustment can occur through average hours, then policymakers should monitor alternative measures of spare capacity – such as the underemployment rate, which takes into account whether employees would like to work more hours – in addition to the unemployment rate, which is based on headcount.
A firm's choice of whether to adjust the number of employees or the hours worked by current employees depends on a range of factors. If the costs of hiring and firing workers are non-trivial, then firms may choose to adjust hours rather than make employees redundant. Expectations of future demand are also important, as firms may be more inclined to adjust working hours if the downturn in demand is expected to be relatively short and shallow. Labour market institutions are also relevant. For example, laws and regulations or wage determination processes may provide incentives for firms to adjust via employee numbers or hours worked. Figure 1 provides tentative evidence that Australian firms have made use of both types of adjustment, with downturns in total hours worked reflecting declines in both employment growth and average hours worked.[3]
In this paper we explore the nature of cyclical labour market adjustment in Australia. That is, we focus on deviations of labour inputs from their longer-run trends, such as during downturns in the economy. The first part of our paper uses aggregate time series data to estimate the relative importance of employment and average hours adjustment. We find that, while both employment and average hours worked tend to adjust over the cycle, the share of labour market adjustment due to changes in average hours worked has increased since the late 1990s. Indeed, the contribution of average hours to the cyclical variability in total hours worked has tripled, from 20 per cent over 1978–98 to 58 per cent over 1999–2016. Such a large increase in the importance of average hours adjustment was not observed in other developed economies, such as the United States, Germany and Japan (e.g. Merkl and Wesselbaum 2011; Kakinaka and Miyamoto 2012).
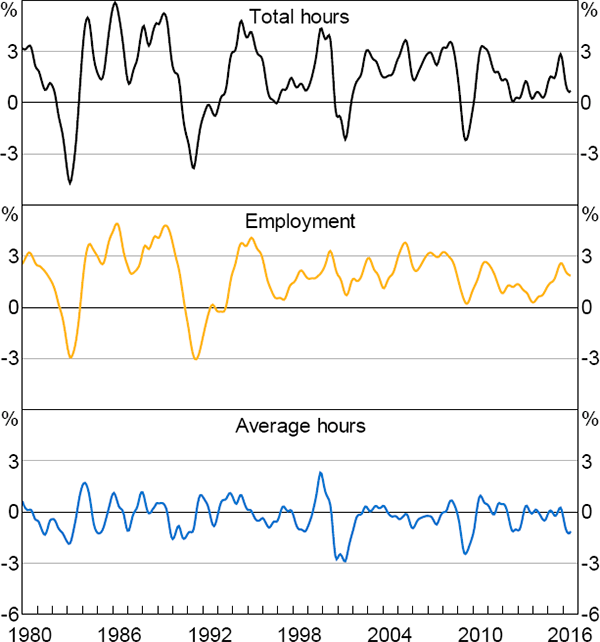
Note: Year-ended growth rates are smoothed using a 13-period Henderson moving average
Sources: ABS; Authors' calculations
We investigate several plausible explanations for this change. We argue that the less severe nature of economic downturns in the 2000s, labour market reforms and increases in hiring costs may all help explain the relatively larger contribution of average hours adjustment in Australia since the late 1990s. However, it is difficult to assess the relative importance of these explanations using statistical models that relate average hours to GDP (or the output gap), as each explanation implies that changes in average hours worked have been more sensitive to the business cycle since the late 1990s.
Changes in the composition of employment between jobs involving longer hours of work and jobs involving fewer hours have also contributed to declining average hours during downturns.[4] However, we find that the primary driver of declines in average hours during downturns has been declines in average hours worked within the different categories of employment. This is confirmed through our analysis of individual-level panel data from the ABS Labour Force Survey (LFS), which suggests that the main driver of the decline in average hours worked during the 2008–09 downturn was a reduction in the average number of hours worked by employees who remained in the same job (i.e. labour hoarding), rather than changes in the average number of hours worked by individuals who changed jobs or entered or exited employment.
The remainder of the paper is structured as follows. Section 2 provides estimates of the contribution of average hours worked and employment to the overall cyclical variability in total hours worked, and how this has changed over time. Section 3 outlines the main explanations for the increased role of average hours adjustment and the evidence for each. Section 4 sheds further light on cyclical labour market adjustment in Australia by decomposing the fall in average hours worked during the 2008–09 downturn using data on individual workers. Section 5 concludes.
2. Changes in Labour Market Adjustment over Time
To analyse cyclical adjustments in the Australian labour market, the total hours worked, employment and average hours worked variables are detrended (see Appendix A for further details). This helps to isolate changes related to the business cycle, by abstracting from the trend decline in average hours worked since the late 1970s and the trend increase in employment. For example, while total employment was little changed during the 2008–09 downturn, it went from being 1.1 per cent above its trend level in September quarter 2008 to 0.6 per cent below its trend in September quarter 2009 – a cyclical change of −1¾ percentage points.
To assess whether labour market adjustment has changed over time, the cyclical decline in total hours worked is calculated for each of the past four economic ‘downturns’, as well as the contributions of employment and average hours worked to each decline (Figure 2).[5]
The first point evident from this analysis is that the cyclical declines in total hours worked were larger in the 1980s and 1990s recessions, reflecting the greater severity of these recessions compared with the economic downturns in the 2000s. Second, in the early stages of each downturn both employment and average hours worked contributed to the cyclical decline in total hours worked. Third, the absolute cyclical decline in average hours worked was similar in all four downturns, with the peak-to-trough declines in the 2000s downturns (2¼ per cent) only slightly larger than the declines in the 1980s and 1990s recessions (1¾ per cent). Finally, a larger proportion of the declines in total hours worked during the 1980s and 1990s recessions came about through reductions in employment, rather than via average hours; employment accounted for around 75 per cent of the peak-to-trough decline in total hours worked during the 1980s and 1990s recessions, compared with a contribution of only around 45 per cent in the 2000s downturns.
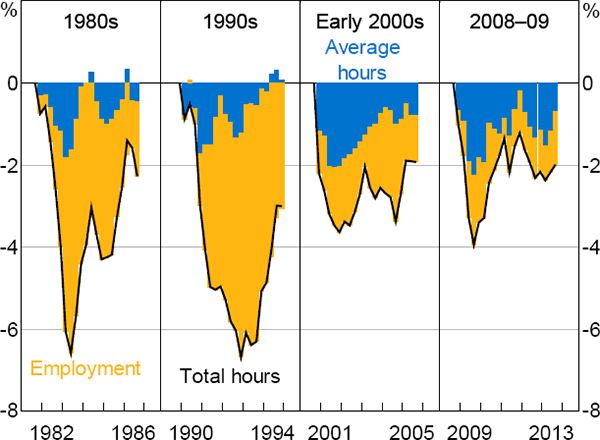
Notes: Log-difference relative to previous peak, multiplied by 100; peaks in total hours are dated as 1981:Q3, 1989:Q4, 2000:Q3 and 2008:Q3; all series are detrended using an HP filter with smoothing parameter λ = 1,600
Sources: ABS; Authors' calculations
Decomposing the overall cyclical variation in total hours worked since 1978 – using the entire sample of quarterly data and taking into account the correlation between employment and average hours – provides further evidence that relatively more adjustment has occurred through average hours worked since the late 1990s (Table 1). This decomposition follows Merkl and Wesselbaum (2011) (see Appendix A for details). While estimates suggest that average hours worked have accounted for around one-quarter of the variability in total hours worked since the late 1970s, statistical tests point to a structural break in the cyclical relationship between total hours and average hours in the late 1990s (see Appendix A). The estimated contribution of average hours tripled after the late 1990s, to 58 per cent. This reflects both an increase in the variability of average hours worked and a decrease in the variability of employment over the past two decades (Figure 1).
Full sample | 1978–98 | 1998–2016 | |
---|---|---|---|
Employment | 73 | 80 | 42 |
Average hours | 27 | 20 | 58 |
Notes: All variables are in logs and detrended with an HP filter (λ = 1,600); see Appendix A for details about the decomposition Sources: ABS; Authors’ calculations |
Comparable estimates for other countries suggest that average hours worked in Australia now play a much larger role in labour market adjustment compared to the United States and Germany, but a smaller role than in Japan (Figure 3). However, Australia is the only one of these countries to have experienced a substantial pick-up in the contribution of average hours since the 1990s. Some potential explanations for this are discussed below.
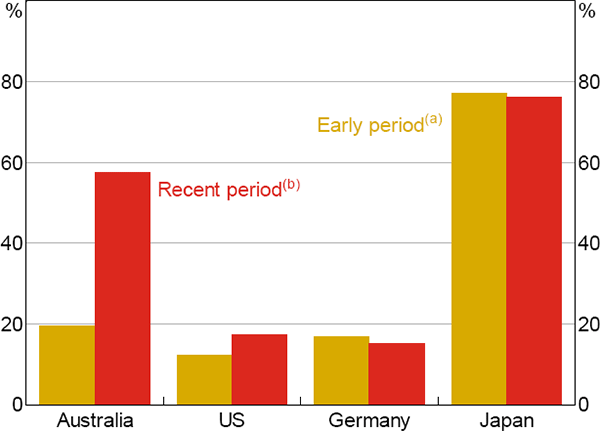
Notes:
See Appendix A for further details about the decomposition
(a) 1978–98 for Australia, 1970–90 for the United States and Germany and 1970–99 for Japan
(b) 1998–2016 for Australia, 1991–2009 for the United States and Germany and 2000–2012 for Japan
Sources: ABS; Authors' calculations; Kakinaka and Miyamoto (2012); Merkl and Wesselbaum (2011)
3. Why Has More Adjustment Occurred through Average Hours Worked?
The estimated date of the break in the late 1990s provides clues as to the source of this change in the nature of labour market adjustment. This date broadly coincides with a decline in the volatility of GDP growth in Australia. Also, this followed a period of substantial labour market reforms in the 1980s and 1990s. However, because these changes overlapped, it is difficult to disentangle their separate effects (see Section 3.3). The following sections discuss these influences in more detail.
3.1 Explanation 1: Downturns in the 2000s were Less Severe
A plausible explanation for the higher share of average hours adjustment since the late 1990s is that the economic downturns in the 2000s were relatively short and shallow compared with the recessions in the 1980s and 1990s (Figure 4).[6] Firms are likely to prefer to adjust to weaker demand, at least in the first instance, by reducing employees’ hours rather than employee numbers because of the costs and other difficulties associated with firing employees and future rehiring. Had the downturns in the 2000s been more severe, firms may have needed to shed more labour eventually. This is consistent with analysis from the Organisation for Economic Co-operation and Development (OECD) (2010) – based on 68 recessions in 18 countries – which concluded that adjustments to average hours tend to make the largest contribution to the decline in labour input at the early stages of a downturn. As the downturn progresses, employers increasingly resort to reducing the number of employees. In each of the downturns shown in Figure 2, the cyclical decline in average hours worked accounted for around half of the adjustment during the first year of the downturn. But for the deeper recessions of the 1980s and 1990s, employment became the dominant source of adjustment as weak demand conditions persisted.[7]
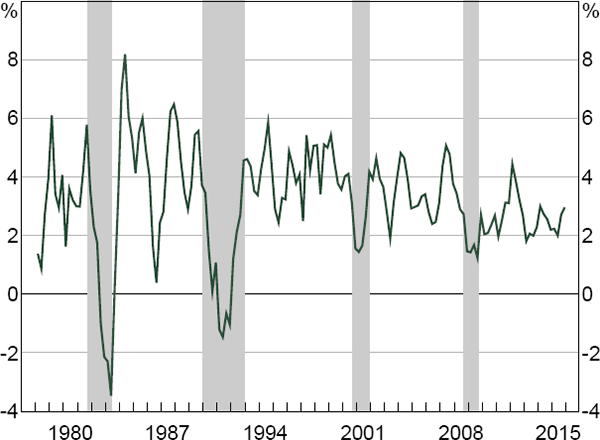
Note: Downturns in total hours worked are shaded
Source: ABS
The lower severity of downturns in Australia in the 2000s may help explain why it was the only country shown in Figure 3 to experience a large increase in the contribution of average hours worked to the change in total hours. The volatility of Australian output – measured by the standard deviation of year-ended growth in real GDP – more than halved between the periods 1978–98 and 1999–2016. In contrast, the volatility of output in the other countries was broadly unchanged, because these countries experienced much deeper downturns in the 2000s.
One qualification to this argument is that economic theory suggests that the decision about whether to adjust via employment or hours is influenced by both current and expected demand conditions. Thus, if firms expect a substantial downturn in demand, they may be more likely to shed labour pre-emptively rather than adjust working hours of their staff. While the 2008–09 downturn in Australia turned out to be less severe than those in the 1980s and 1990s, at the time there were widespread expectations that demand conditions would deteriorate to a similar extent to those earlier recessions (e.g. RBA 2009). Notwithstanding these expectations, there are a number of possible reasons why firms did not engage in more adjustment through redundancies in this episode:
- While future demand was expected to be very weak, current demand conditions at the time were not especially weak.
- The labour market was very tight in the lead-up to the 2008–09 downturn, with the unemployment rate falling to around 4 per cent and a historically high proportion of firms reporting that it was difficult to find suitable labour (Figure 5; Plumb, Baker and Spence (2010)). Given this, when growth in demand slowed, firms may have been reluctant to part with their staff to avoid the costly process of rehiring once demand recovered.
- There was heightened uncertainty surrounding economic conditions during the 2008–09 downturn compared with previous downturns (Figure 5; Moore (2016)). In these circumstances, theory suggests that firms will be less inclined to make investment decisions that are difficult or costly to reverse, including either hiring or firing workers.
- Workers were very pessimistic about their future employment prospects during the 2008–09 downturn, with self-reported perceptions about future unemployment prospects rising to unprecedented levels (Figure 5). Therefore, employees may have been more reluctant to leave their jobs amid concerns about being able to find new ones, and more willing than usual to negotiate over working hours and other conditions in return for job security.
While these factors should, in theory, be related to average hours worked, the volatile and noisy nature of measures of average hours makes it difficult to find empirical evidence of such relationships. We were not able to find evidence of a relationship between average hours and the above factors in a regression model.
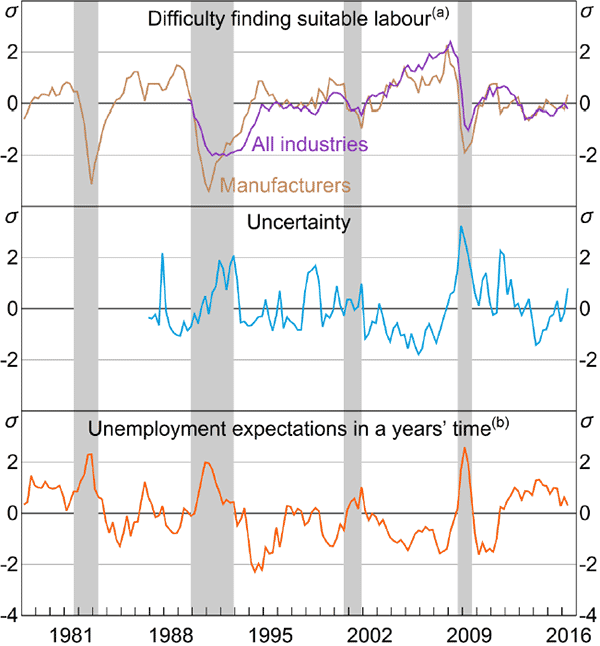
Notes:
Downturns in total hours worked are shaded
(a) ‘All industries’ is the share of firms indicating that the availability of suitable labour is a constraint on output; ‘Manufacturers’ is the net balance of firms finding it harder to get labour than three months ago
(b) Net balance of consumers expecting unemployment to be higher in the coming 12 months
Sources: ACCI-Westpac; Moore (2016); NAB; Westpac and Melbourne Institute
3.2 Explanation 2: Labour Market Reforms and Rising Hiring Costs
Another possible explanation for the larger contribution of average hours to labour market adjustment since the late 1990s is that the reforms to industrial relations arrangements in the late 1980s and early 1990s made it easier for firms to bargain directly with their employees over matters like wages and working hours. This may have provided firms with more scope to reduce working hours in an effort to lower labour costs while retaining employees. As noted by Borland (2011), the 2000s were the first time in which these reforms were ‘tested’ by an economic downturn.
In practice, measuring flexibility of the labour market and how it has changed over time is not straightforward. The cyclical adjustment in average hours was larger in the 2000s, which provides tentative evidence that the labour market reforms played a role; the peak-to-trough decline in average hours was 2¼ per cent in both downturns, compared with 1¾ per cent in the early 1980s and early 1990s recessions (Figure 2). However, these differences are fairly modest.
The cost of terminating employment and the cost of screening and training new employees can also affect the nature of labour market adjustment. If firing or hiring costs are high, firms may be more inclined to respond to weaker demand conditions by decreasing the hours worked by existing staff. The average employment termination payment in Australia was nearly $14,000 in 2012/13, or 25 per cent of an average annual salary.[8] Broader measures, such as the OECD's employment protection legislation (EPL) indices, suggest that firing costs in Australia are lower than in most European countries, although higher than in the United States where employment protection is relatively limited.[9] However, these indicators showed little change between the mid 1980s and late 2000s for Australia, so it is unlikely that changes in firing costs have been a significant driver of changes in the nature of labour market adjustment.
In terms of hiring costs, the cost of screening and training labour is likely to have risen over time, given the increase in the number of jobs requiring specialist skills and training (Faccini and Hackworth 2010). Hiring costs are particularly high during periods of labour market tightness, since firms face higher search costs to fill vacant jobs. This might suggest that higher hiring costs can explain part of the increase in the contribution of average hours adjustment over time. However, it cannot explain why average hours adjustments have become more important in Australia but not in other advanced economies, since these countries are also likely to have seen an increase in the cost of screening and training labour.
3.3 Empirical Evidence
The analysis in the previous sections suggests that the less severe nature of downturns in the 2000s, labour market reforms and increases in hiring costs may all help to explain the increased role of changes in average hours in Australian labour market adjustment since the 1990s. However, it is difficult to distinguish between these explanations using statistical techniques given the available data, as all imply that changes in average hours worked have been more sensitive to changes in the business cycle (relative to employment) since the late 1990s. Specifically:
- If average hours adjust more during the initial stages of a downturn and only up to a point, then econometric models would tend to find that average hours worked are relatively more responsive to changes in GDP during less severe downturns (and relatively less responsive during severe downturns, in which more adjustment occurs through employment). Since the downturns in Australia were less severe after the late 1990s, this suggests that the relative sensitivity of average hours worked to a given change in GDP should have risen in recent decades.
- Labour market reforms and rising hiring costs suggest that the sensitivity of average hours to a given change in GDP should have increased (or that the sensitivity of employment has decreased, or both). Given that many important labour market reforms had been completed by the late 1990s, the relative sensitivity of average hours worked should have increased after the late 1990s.
Nevertheless, it is useful to test whether at least one of these explanations contributed to the changes in labour market adjustment in Australia in recent decades. One way of exploring whether labour market variables have become more or less sensitive to the cycle is to estimate a vector autoregression (VAR) model, which captures the dynamic relationships between GDP, average hours worked and employment.[10] The response of labour market variables to a negative 1 per cent ‘shock’ to GDP is shown in Figure 6. The left- and right-hand panels show the impulse response functions (IRFs) estimated over the 1978–98 and 1998–2016 sub-samples, respectively, along with the +/− 2 standard error bands. Comparing the left- and right-hand panels reveals whether the relationship between GDP and each variable changed over time.
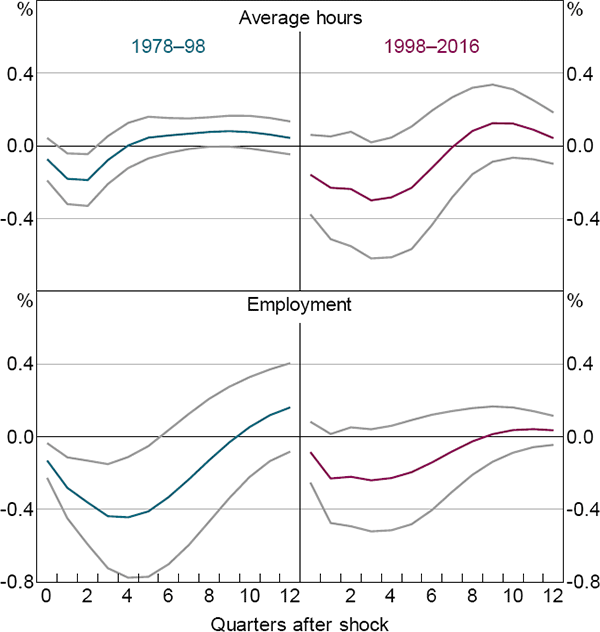
Note: Grey lines show +/− 2 standard error bands
Sources: ABS; Authors' calculations
In general, the volatile and noisy nature of measures of average hours worked makes it difficult to find empirical evidence of relationships between average hours worked and the other variables that should, in theory, be related to it. That said, the VAR model provides some tentative evidence that average hours became more sensitive to the cycle after 1998, although the difference between the IRFs in the two sub-periods is not statistically significant. The model also suggests that the responsiveness of employment declined after 1998, although again the difference between the sub-periods is not statistically significant. Thus, while it is difficult to draw any firm conclusions from this model, it seems likely that either the reduction in the severity of downturns or labour market reforms (or both) explains at least part of the greater contribution of average hours worked to labour market adjustment in recent decades.
One distinction is that the ‘severity of downturns’ explanation (at least as defined above) only relates to downturns, while the ‘labour market reforms’ explanation is relevant for both downturns and non-downturn periods. So, if the contribution of average hours adjustment has increased since the late 1990s even if we focus only on non-downturn periods, then one interpretation is that this supports the ‘labour market reforms’ explanation.
To examine this, we re-estimate the contributions of average hours and employment to the variation in total hours worked after excluding the downturn periods, and find that the results are almost identical to those shown in Table 1. While at first glance this suggests that labour market reforms have been an important factor, this type of analysis does not adequately control for the moderation in the economic cycle since the late 1990s. Figures 4 and A1 show that the economic cycle was more pronounced in the earlier sub-sample, even if we focus only on non-downturn periods. In particular, economic downturns were often preceded by a large cyclical upswing in the labour market. If firms respond to booms in a similar way to downturns – by adjusting hours in the initial stages, and then adjusting employment as the boom/recession persists – then simply excluding the downturn periods does not help us to isolate the separate contribution of ‘labour market reforms’. In other words, the ‘severity of downturns’ explanation could be framed more broadly in terms of the ‘severity of the economic cycle’ – both booms and busts.
3.4 Changes in the Composition of Employment
Average hours worked are affected not just by changes in the hours of workers in given jobs, but also by changes in the composition of employment between jobs involving long hours of work and jobs involving fewer hours. Falls in average hours worked could reflect changes in the industry composition of the economy during a recession. For example, if industries with longer working hours incur relatively more job losses during an economic downturn, this will contribute to a decline in overall average hours worked. Thus, another possible explanation for larger adjustments in average hours worked since the late 1990s is that compositional effects changed over time.
To estimate whether compositional changes have played a role, deviations in average hours worked can be decomposed into two effects (Figure 7; see Appendix B for details):
- A ‘composition effect’, which is the change in average hours worked owing to shifts between different categories of employment, while holding hours unchanged within each category. Within-category hours are kept at their August 2008 levels, and the categories include age group, gender, industry and occupation of employment, marital status and part-time and full-time employment status.
- A ‘within effect’, which captures changes in average hours worked within each category of employment; for example, a fall in average hours worked by employees within the manufacturing sector or a fall in average hours worked within part-time employment.
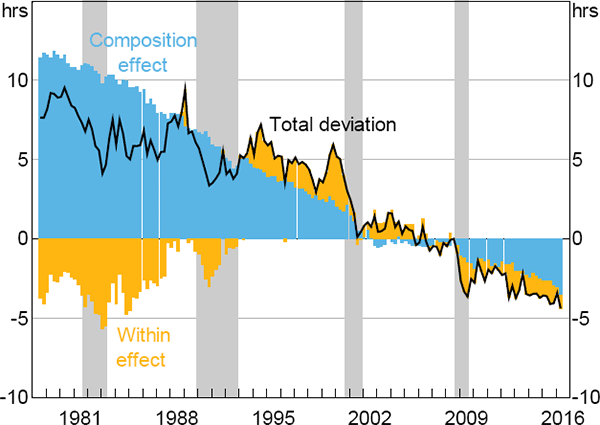
Notes: Downturns in total hours worked are shaded
Source: ABS; Authors' calculations
The decline in average hours worked since the late 1970s is entirely due to longer-run changes in the composition of employment. In particular, part-time employment has grown rapidly relative to full-time employment. However, during downturns, the primary driver of shifts in average hours is changes in average hours worked within categories, rather than changes in the composition of employment.[11] The ‘within effect’ contributed more than two-thirds of the peak-to-trough decline in average hours worked in the 1980s and 1990s recessions and more than half in the 2000s downturns.
Closer examination of the decomposition in Figure 7 reveals that the estimated ‘composition effect’ during the 2008–09 downturn is driven almost entirely by the increase in the part-time share of employment, from 28.3 per cent in August 2008 to 30.1 per cent in August 2009. Less than 0.1 hours of the 3.3 hours decline in average monthly hours worked between 2008 and 2009 can be accounted for by other compositional changes. The other compositional forces were small and offsetting; shifts in the industrial and occupational structures, and the rise in the female share, all exerted downward pressure on overall average hours worked. These effects were partly offset by a sharp decline in the employment share of 15–19 year olds, who tend to work shorter hours than other age groups.[12]
The problem with attributing changes in the part-time share of employment during 2008–09 to the ‘composition effect’ is that it could also reflect a within-job shift to shorter hours, rather than more part-time jobs being created. That is, during a downturn, firms can temporarily downgrade full-time workers to part-time hours (i.e. labour hoarding). Indeed, during the 2008–09 downturn there was an increase in the share of part-time employees who reported that they ‘usually’ work full time, but were working part-time hours because they were ‘stood down’, placed on ‘short time’, or because of ‘insufficient work’. Such workers accounted for 10 per cent of the overall rise in the part-time share during the downturn. The next section addresses this concern, by analysing changes at the job level rather than at the category level.
With this in mind, although compositional effects were relatively more important in the 2000s – and therefore may account for some of the increased contribution of average hours adjustments – they do not appear to have been the main driver.
4. A Closer Look at the 2008–09 Downturn
Greater insights into the within-job shift to shorter working hours during downturns can be gleaned by looking at the individual-level data underlying the LFS.[13] The main benefit of these data is that they are longitudinal in nature, meaning that individual workers can be tracked over the period of time during which they remain in the sample to see if they had their hours reduced. The main disadvantage of these data is that they are only available for the period 2008 to 2010, meaning that analysis is restricted to the most recent economic downturn.
The individual-level data let us distinguish changes in aggregate average hours worked due to:
- Labour hoarding: a reduction in hours for workers remaining in the same job. This could reflect, for instance, firms temporarily reducing the hours of their staff, such as by asking them to work shorter hours or to take leave.
- Labour market churn: this includes the churn of workers into new jobs within the same industry (and occupation) or into new jobs in different industries. It also includes the impact on aggregate average hours due to changes in the average hours of people entering or exiting employment. For example, it may be that manufacturing jobs lost during a downturn are replaced with new manufacturing jobs that offer shorter hours. This is broader in definition than the ‘composition effect’, because in addition to capturing net movements of workers into different categories, it also captures changes due to the churn of workers within a given category.
We are not the first to attempt to distinguish between these factors using Australian data. Using data on a panel of workers in the Household, Income and Labour Dynamics in Australia (HILDA) Survey, Wooden (2012) found an increase in the share of employees who remained in the same job and had their hours reduced during the 2008–09 downturn. However, he found that most of the fall in average hours worked was due to the destruction of jobs with relatively long hours and the creation of jobs with relatively short hours. van Wanrooy et al (2009) used longitudinal data on workers from the Australia at Work survey, finding that both labour hoarding and labour market churn were important contributors to the overall decline. In terms of labour hoarding, the main drivers were changes in patterns of leave taking and an increase in the share of full-time workers being asked by their employer to work shorter hours, while in terms of churn, the most important contributors were increases in both the job destruction rate and the share of labour market entrants undertaking part-time work.
However, there are two limitations to these previous studies relating to the data. First, both the HILDA and Australia at Work surveys provide data on usual hours of work, rather than actual hours. It is not clear how survey respondents interpret the word ‘usual’, and whether it picks up short-term changes in work hours. Second, these surveys are administered relatively infrequently – every 12 months – and are thus more likely to miss the high-frequency dynamics in hours worked through a downturn.
Unlike previous studies for Australia, we use the individual-level data underlying the LFS. These data were made available to researchers in 2012 for the first time in the survey's 50-year history. In addition to providing a larger sample size (around 27,000 dwellings per month), these data also allow us to follow cohorts of workers every month. These data are uniquely suited to our research question, since they are the only nationally-representative, high-frequency, longitudinal data on hours worked. The survey also provides information on both actual and usual hours, which aggregate up to the official figures published in the monthly labour force release.[14]
4.1 Matched Sample
We use the LFS data to construct short panels of individual workers. Individuals are surveyed every month for eight months, before being retired from the sample. Thus, there are up to eight monthly surveys for each individual. While the LFS collects information on hours worked every month, some additional information (e.g. industry, occupation and job tenure) is only collected in the February, May, August and November surveys, which we refer to as the ‘mid-quarter’ surveys. We focus on mid-quarter surveys, since the additional data items are important for our analysis.
We define the period t matched sample as all individuals at time t (e.g. May 2008) who also responded to the mid-quarter survey at t − 1 (e.g. February 2008). Since workers are surveyed for eight consecutive months in the LFS, this suggests that five-eighths of the overall LFS sample at t would be in the period t matched sample, and the remaining three-eighths would be in the unmatched sample. In practice, however, the period t matched sample makes up less than five-eighths of the full sample at t due to attrition from the survey (it is closer to 55 per cent).[15]
A potential concern with any analysis based on a matched sample is that estimates could be biased if attrition from the sample does not occur at random. For example, young people are systematically less likely to remain in the matched sample than older people, as are individuals that are less stably employed (ABS 2013). The population weights used to produce the monthly labour force statistics (provided in the unit-record data) do not adjust for non-random attrition. Thus, for our analysis, we construct and use a set of ‘longitudinal weights’ that account for differential rates of attrition amongst population sub-groups (at least along the observable dimensions that we can adjust for using the information contained in the dataset).[16]
4.2 Which Groups Contributed to the Decline in Average Hours?
We classify each worker at time t into one of four categories:
- Job stayers are workers employed in the same job at t − 1 and t
- Job movers are workers who move to a different job between t − 1 and t
- Job finders are workers who move from unemployment or not in the labour force (NILF) at t − 1 into employment at t
- Job leavers are workers who move from employment at t − 1 to unemployment or NILF in t.
Job finders and job leavers can be directly identified using the LFS individual-level data by simply comparing the labour force status of each individual from survey to survey. We can also directly identify whether individuals were employed in consecutive surveys. But in order to distinguish job stayers from job movers, we need to draw on other information in the dataset (see Appendix C for details).
We estimate that job stayers account for 90 per cent of total employment, on average. This large share means that they are likely to drive most of the movements in overall average hours worked. However, during periods of labour market upheaval, such as during downturns and recessions, those exiting from (or entering into) employment could also influence movements in overall average hours worked, even though they comprise only a small share of employment.[17]
Figure 8 shows average hours worked for each of the four categories of employment over the period 2008 to 2010 (the period for which individual-level data are available). For job leavers, we plot their average hours before their exit from employment. An increase in the average hours of job leavers will contribute to a fall in overall average hours, all else equal. In contrast, an increase in the average hours of job finders will contribute to a rise in overall average hours. The figure shows that the average hours worked by job leavers rose during the 2008–09 downturn, contributing to the fall in average hours. This is consistent with full-time workers being laid off more extensively than part-time workers, relative to more ‘normal’ times. Average hours worked by job finders also rose during the downturn, but at a more gradual pace, partly offsetting the negative contribution due to job leavers.
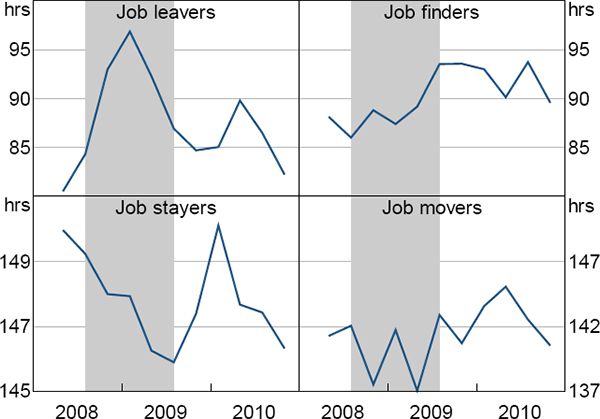
Note: 2008–09 downturn in total hours worked is shaded
Sources: ABS; Authors' calculations
Average hours worked by job stayers fell during the 2008–09 downturn – which also contributed to the overall decline in average hours worked – before recovering to their pre-crisis levels (Figure 8). Average hours worked by job movers were volatile, but were little changed overall during the 2008–09 downturn.
The contribution of job stayers, job movers and job finders to the overall decline in average hours worked is estimated in Figure 9. These contributions depend on the change in average hours worked for each category in Figure 8, along with the change in the relative employment share of each category. The combined effect of changes in employment shares is included as a separate term – labelled as the ‘between effect’. An important drawback of the decomposition is that it does not incorporate the contribution of job leavers to the overall decline in average hours. We are unable to include job leavers due to sample rotation in the LFS, which complicates the calculation of the exact contribution for each employment category (see Appendix D for a discussion of this issue and for further details about the decomposition).
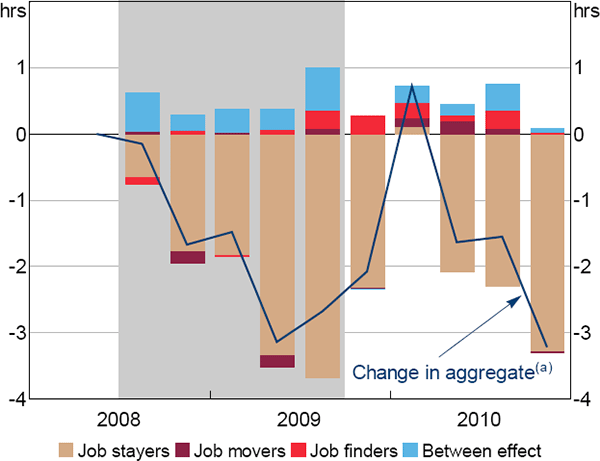
Notes:
2008–09 downturn in total hours worked is shaded
(a) Change in average hours worked in the matched sample, rather than all workers surveyed in the LFS
Sources: ABS; Authors' calculations
We estimate that the decline in average hours worked by job stayers accounted for the majority of the decline in average hours worked during the 2008–09 downturn (Figure 9). This is unsurprising, given that job stayers account for 90 per cent of employment. In contrast, within-category changes in the average number of hours worked by job finders and movers made a negligible contribution to the overall decline.
Changes in the employment shares of each category – the ‘between effect’ – had a positive, but small, impact on overall average hours worked during the downturn. This reflected an increase in the employment share of job stayers – who tend to work longer hours on average than workers in the other categories – and a decrease in the employment share of job movers and job finders, who tend to work shorter hours. These patterns are consistent with a fall in the job-finding rate and a decline in the rate of job-to-job mobility typically observed during economic downturns.
After considering the evidence presented in both Sections 3.4 and 4.2, we conclude that reductions to the hours of job stayers accounted for at least one-half of the overall fall in average hours worked during the 2008–09 downturn, and probably more. The fact that the ‘labour hoarding’ effect in Figure 9 is estimated to be larger than the ‘within effect’ in Figure 7 may indicate that the latter is capturing changes in average hours worked due to churn of workers within given categories (and in particular, within the part-time/full-time employment category).
4.3 Skills Shortages
We can also use the individual-level data to gain a clearer picture about the role that industry skills shortages played in the labour market adjustment. For each industry, Figure 10 shows the adjustment to average hours worked by job stayers during the 2008–09 downturn (y-axis) against the proportion of firms who reported skills shortages during the economic boom leading up to the downturn (x-axis).[18]
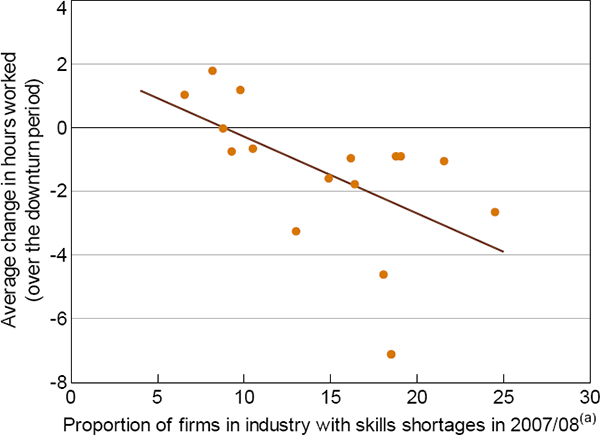
Notes: (a) Proportion of firms reporting that a lack of skilled persons was a barrier to core business or performance in 2007/08, as reported in ‘Selected Characteristics of Australian Business, 2007–08’ (ABS Cat No 8167.0); excludes agriculture, forestry & fishing, public administration & safety and education & training industries
Sources: ABS; Authors' calculations
Reductions in hours worked for those staying in the same job were largest for workers in industries that had experienced skills shortages prior to the downturn (Figure 10). Firms in these industries may have been ‘overutilising’ staff prior to the downturn, and started to reduce hours to more normal levels as demand eased. As discussed earlier, firms may also have been reluctant to let go of skilled workers because labour had been so difficult to source just prior to the downturn. We cannot draw strong conclusions from this analysis, however, as we have not controlled for other factors, such as the size of the industry demand shock.
5. Conclusion
Since the late 1990s, a larger share of cyclical labour market adjustment in Australia has come about via changes in average hours worked, as opposed to changes in employment. While empirical evidence is inconclusive (partly due to the difficulty in modelling average hours worked), our view is that the relatively short and shallow economic downturns in the 2000s have played a role in this. Had these downturns been more severe, like the recessions in the 1980s and 1990s, firms eventually may have needed to shed more workers than they did. In other words, it is likely that both employment and average hours tend to adjust in the early stages of a downturn, but relatively more adjustment occurs through employment as the downturn persists and becomes more severe. It is also possible that labour market reforms over recent decades have provided firms with more scope to reduce their use of labour by reducing working hours rather than by redundancies.
We also find that the main driver of the adjustment in average hours during the 2008–09 economic downturn was a reduction in hours worked for employees who remained in the same job (i.e. labour hoarding). Consistent with this, a longer-run historical analysis suggests that changes in the composition of employment have not been the main driver of the decline in average hours during downturns and recessions.
Appendix A: Decomposing the Cyclical Adjustment of Total Hours
Data for total hours worked, employment, average hours worked and real GDP in Sections 2 and 3.3 are in logs and individually detrended using a HP filter with smoothing parameter λ = 1,600. The top panel of Figure A1 shows the raw data (in logs) and the HP trend of each series, while the bottom panel shows the detrended version of each series.
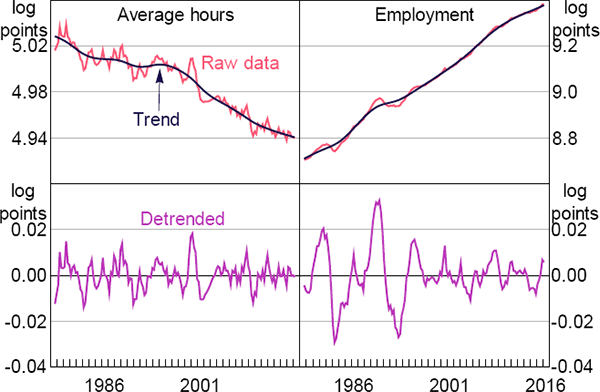
Notes: Trend is HP filter with smoothing parameter λ = 1,600; detrended series are the difference between the raw data series and trend
Sources: ABS; Authors' calculations
The estimates in Table 1 are based on the decomposition outlined in Merkl and Wesselbaum (2011). The contribution of average hours to cyclical adjustment in total hours is given by
where tothrst and avhrst are total hours worked and average hours worked, respectively. The estimate gives the proportion of variation in total hours that derives from average hours, both directly and indirectly through its correlation with employment. Similarly, the contribution of employment to cyclical adjustment in total hours is given by
where empt is employment. These estimates are equivalent to the slope coefficients in simple ordinary least squares regressions of average hours on total hours and employment on total hours, respectively.
Using alternative methods to detrend the data does not affect the main conclusions (Table A1). Using four-quarter-ended log differences rather than the HP filter does not have a material effect on the results. Using one-quarter-ended log differences suggests a larger role for average hours adjustments prior to 1998 relative to the other detrending methods, although this is likely to reflect the high quarter-to-quarter volatility in the average hours data.
The regression framework also allows us to perform the Quandt-Andrews structural break test for an unknown break point. This test suggests that the break in the coefficient on total hours worked (in either the average hours or employment specification) in 1998:Q2 is significant at the 1 per cent level. The test is for a break in the coefficient in the regression equation, although also allowing for a break in the constant does not change the result. We also tested the robustness of this break date using alternative detrending methods based on a Chow test with the break date set to 1998:Q2. There was evidence of a statistically significant break in 1998:Q2 using one-quarter-ended or four-quarter-ended log differences, rather than the HP filter.
Full sample | 1978–98 | 1998–2016 | |
---|---|---|---|
HP filter (λ = 1,600) | |||
Employment, θE | 73 | 80 | 42 |
Average hours, θA | 27 | 20 | 58 |
Log differences (four-quarter-ended) | |||
Employment | 70 | 79 | 45 |
Average hours | 30 | 21 | 55 |
Log differences (one-quarter-ended) | |||
Employment | 51 | 57 | 40 |
Average hours | 49 | 43 | 60 |
Notes: In both the HP filter and the two-stage least square specifications, all variables are in logs and detrended with an HP filter (λ = 1,600); for a definition of ‘downturn periods’, see footnote 5 Sources: ABS; Authors' calculations |
Appendix B: Composition Effects
Rather than considering all potentially relevant compositional factors individually (e.g. age, gender, industry, part-time/full-time employment), a multiple regression-based approach can be used to consider the combined effect of these variables simultaneously. This approach can be used to estimate the effect of compositional shifts in employment on the change in average hours worked between August 2008 and any given quarter since the late 1970s.
The first step is to estimate a regression using data on individual workers from the August 2008 cross-section of the LFS:
where Hi,2008 is actual hours worked per month by individual i, is a vector of explanatory variables and β is the vector of coefficients to be estimated. The regression is weighted using the ABS's population weights. The explanatory variables include: part-time or full-time employment status (1 dummy), age group (11 dummies), gender (1 dummy), industry (18 dummies), occupation (7 dummies), marital status (1 dummy), whether the employee was born in Australia or another main English or non-main English-speaking country (2 dummies), and whether the worker is an employee, employer or self-employed (2 dummies).
The contribution of compositional change to the overall change in average hours between August 2008 and quarter t is given by
where is a vector of estimated coefficients from the August 2008 cross-section of the LFS and
and
are the means across individuals of the observed values in August 2008 and quarter t, respectively. This is referred to as the ‘composition effect’. While LFS micro data are not currently available on a consistent basis prior to 2008 or after 2010,
is available from the published LFS employment time series data. The ‘composition effect’ measures the change in average hours that would have occurred between August 2008 and quarter t if all within-category (e.g. within-industry and within-age group) hours had remained constant at their August 2008 levels. The difference between the actual change in average hours and the ‘composition effect’ measures the effect on average hours of changes in hours within categories of employment, holding composition constant.[19] In this paper, this is referred to as the ‘within effect’.
Appendix C: Classifying Job Stayers and Movers
While the LLFS can identify whether an individual was employed in consecutive mid-quarter surveys, it does not contain a variable that indicates whether the individual was in the same job. To infer whether an individual changed jobs, we use information on the individual's tenure in their current job or business. This tenure variable (TENUREC) records whether an individual has been employed in their current job or business for less than one year, or at least one year. By comparing this variable to its value in the previous mid-quarter survey – for the matched sample of people employed in both surveys – we can infer whether the individual was in the same job (job stayer) or not in the same job (job mover).
We assume that an individual is a job stayer (i.e. working in the same job as in the previous mid-quarter survey) if they reported to have been in their current job for at least one year. Conversely, an individual is classified as a job mover if his or her tenure changed from at least one year in the previous mid-quarter survey to less than one year in the current survey. This classification scheme is summarised in Table C1.
Survey t | |||
---|---|---|---|
<1 year in job | ≥1 year in job | ||
Survey t − 1 | <1 year in job | Indeterminate | Job stayer |
≥1 year in job | Job mover | Job stayer | |
Note: Indeterminate means that job mover/stayer status cannot be determined using job tenure alone |
The mover/stayer status of some workers cannot be determined using the tenure variable alone; specifically, those whose tenure was less than one year in both the current and previous mid-quarter survey. These workers either started a new job prior to the previous survey (but less than one year ago) and were still in that job, or held a different job at both survey dates, both of which were for a period of less than one year. On average, such workers represent around 13 per cent of the total matched sample of people employed in consecutive mid-quarter surveys. In order to categorise these workers as either job stayers or job movers we use information on a number of other factors that may be predictive of changing jobs, namely:
- the individual changed their industry or occupation of employment
- the individual moved from part-time to full-time work (or vice versa), or changed from being an employer to an employee or own account worker (or vice versa)
- the individual experienced a spell of unemployment or NILF in any of the months in between the two mid-quarter surveys
- in the previous mid-quarter survey, the individual reported that he or she did not anticipate to be working in their current job or business in one year's time
- the individual reported a ‘change in job’ during the reference week of any of the previous three monthly LFS surveys (including the current survey).
To estimate the extent to which the above factors are associated with job changing, we estimate a probit model for the probability of being a job mover using the above factors as the explanatory variables. The model is estimated on the sample of individuals whose job status is able to be identified using job tenure alone. We then use this model to obtain the predicted probability of being a job stayer for all people employed in consecutive mid-quarter surveys, including those for whom job status was not able to be determined using job tenure. We then assign all people in the latter group to the ‘job mover’ category if their predicted probability of being a job mover exceeds some threshold level, which we set at 0.15.[20] We assign the remaining individuals (those whose predicted probability of being a job mover is less than 0.15) to the ‘job stayer’ category. Our choice of threshold was based on an analysis of what proportion of job movers the probit model correctly classified (the sensitivity), and what proportion of job stayers were correctly classified (the specificity) at every possible threshold. A 0.15 cut-off resulted in job stayers being correctly classified 97.5 per cent of the time, and job movers 33.5 per cent of the time. The ‘default’ threshold of 0.5 would have biased correct predictions towards the more common outcome at the expense of the less common one.[21]
Appendix D: Decomposing the Change in Average Hours Worked
Average hours worked in period t can be expressed as the weighted average of the average hours worked by job movers (M), job stayers (S) and job finders (F):
where is overall average hours worked,
is the combined weight of the individuals in category i ∈ (M,S,F) and
is average hours worked by the individuals in category i.
The change in average hours worked between two quarters can then be written as:
which can be rearranged to obtain the contributions from the change in the weight of each category and the change in average hours worked within each category:
The first term on the right-hand side represents the contributions from changes in average hours worked within each category of employment and the second term represents the contributions from the change in the weight of each category (the between effect).
The main drawback of this decomposition is that is does not explicitly incorporate the effect due to changes in average hours worked by those leaving employment (job leavers). All else equal, if the average hours worked by job leavers increases, aggregate average hours worked should decrease.
In particular, the omission of job leavers could affect the within contribution for job stayers. To get an estimate of the potential impact of the increase in average hours worked by those leaving employment on average hours worked by job stayers, we constructed a counterfactual where a number of stayers were assumed to work additional hours equal to the change in average hours worked by leavers:
where is the counterfactual estimate of average hours by stayers,
is the number of leavers at time t and
is the number of stayers. This represents a case where those leaving employment came from a lower part of the distribution of actual hours worked. This counterfactual only had a marginal impact on the estimated within contribution from job stayers.
While indicative, this represents only one of several possible ways that the increase in average hours worked by leavers during the 2008–09 downturn could have affected the estimated contribution of job stayers to the decline in aggregate average hours worked. For example, the counterfactual does not incorporate the cumulative effect of the increase in average hours worked by leavers. Accounting for this could potentially further reduce the estimated within contribution for stayers.
There are two other caveats to the decomposition in Figure 9. First, we have treated our panel as if it was balanced (that is, that the workers in the matched sample do not change over time), when in fact it is unbalanced (the workers in the matched sample do change over time). As such, the decomposition assumes that changes in average hours worked within a category reflect the actual underlying behaviour of the individuals in that category. For example, changes in average hours worked by job stayers are assumed to reflect changes in the hours worked by individuals that stayed in the same job, rather than differences between successive cohorts of job stayers in the sample over time.[22] However, although flows of workers into and out of the matched sample may have contributed to the estimated decline in average hours worked by job stayers, we do not believe this to be the case. Figure D1 shows the average hours worked for successive cohorts of job stayers over time. For each cohort, we have either two or three observations on each worker (with each representing a separate balanced panel). We plot cohorts with two observations in the left panel, and those with three observations in the right panel. By looking at individual cohorts (i.e. balanced panels) we can abstract from the effects of rotation into and out of the matched sample. We find that average hours worked fell for every cohort of job stayers during the 2008–09 downturn.[23]
A second caveat is that the decomposition in Figure 9 is only for workers in the matched sample, rather than all workers surveyed in the LFS. The unmatched workers that we excluded from our analysis make up around 45 per cent of the overall LFS sample. This group experienced an even larger decline in average hours worked during the 2008–09 downturn than the matched sample of workers, which may reflect the fact that these two groups typically have different characteristics; for example, unmatched workers tend to be younger and less stably employed than those who are matched. If the decline in average hours worked within the unmatched sample was mainly due to labour market churn, then we may have overstated the role of labour hoarding by focusing on the matched sample.
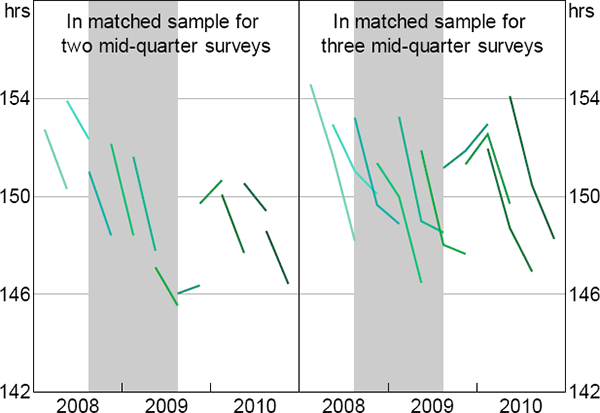
Note: 2008–09 downturn in total hours worked is shaded
Sources: ABS; Authors calculations
References
ABS (Australian Bureau of Statistics) (2013), ‘Labour Statistics: Concepts, Sources and Methods, 2013’, Cat No 6102.0.55.001, online, accessed 5 April 2016.
ATO (Australian Taxation Office) (2015), ‘Taxation Statistics 2012–13, Individuals, Table 1: Selected items for Income Years 1978–79 to 2012–13’, online, accessed 1 February 2016.
Bishop J and M Plumb (2016), ‘Cyclical Labour Market Adjustment in Australia’, RBA Bulletin, March, pp 11–20.
Borland J (2011), ‘The Australian Labour Market in the 2000s: The Quiet Decade’, in H Gerard and J Kearns (eds), The Australian Economy in the 2000s, Proceedings of a Conference, Reserve Bank of Australia, Sydney, pp 165–218.
Faccini R and C Hackworth (2010), ‘Changes in Output, Employment and Wages during Recessions in the United Kingdom’, Bank of England Quarterly Bulletin, 50(1) Q1, pp 43–50.
Fluss R, D Faraggi and B Reiser (2005), ‘Estimation of the Youden Index and its Associated Cutoff Point’, Biometrical Journal, 47(4), pp 458–472.
Kakinaka M and H Miyamoto (2012), ‘Extensive vs. Intensive Margin in Japan’, International University of Japan Economics & Management Series EMS-2012-14.
Merkl C and D Wesselbaum (2011), ‘Extensive versus Intensive Margin in Germany and the United States: Any Differences?’, Applied Economics Letters, 18(9), pp 805–808.
Moore A (2016), ‘Measuring Economic Uncertainty and Its Effects’, RBA Research Discussion Paper No 2016-01.
OECD (Organisation for Economic Co-operation and Development) (2010), ‘Moving Beyond the Jobs Crisis’, OECD Employment Outlook: Moving Beyond the Jobs Crisis, OECD Publishing, Paris, pp 15–102.
Plumb M, M Baker and G Spence (2010), ‘The Labour Market during the 2008–2009 Downturn’, RBA Bulletin, March, pp 1–6.
RBA (Reserve Bank of Australia) (2009), ‘Economic Outlook’, Statement on Monetary Policy, May, pp 67–71.
RBA (2014), ‘Box B: Lags from Activity to the Labour Market’, Statement on Monetary Policy, May, pp 39–41.
van Wanrooy B, S Wright, J Buchanan, S Baldwin and S Wilson (2009), Australia at Work: In a Changing World, Workplace Research Centre, University of Sydney.
Wooden M (2012), ‘Hours of Work and Job Mobility’, in R Wilkins and D Warren, Families, Incomes and Jobs, Volume 7: A Statistical Report on Waves 1 to 9 of the Household, Income and Labour Dynamics in Australia Survey, HILDA Survey Annual Statistical Report, Melbourne Institute of Applied Economic and Social Research, University of Melbourne, Melbourne, pp 155–156.
Acknowledgements
We would like to thank Rochelle Guttmann, David Jacobs, Christopher Kent, Marion Kohler, Gianni La Cava, John Simon, Peter Tulip and seminar participants at the Reserve Bank of Australia and the University of Melbourne for their helpful comments. The views expressed in this paper are those of the authors and do not necessarily reflect the views of the Reserve Bank of Australia. The authors are solely responsible for any errors.
Footnotes
Bishop and Plumb (2016) summarise many of the key results of this paper. The Australian Bureau of Statistics (ABS) has since made revisions to its modelled monthly hours worked series and these revisions have been incorporated into the analysis in this paper. [1]
In addition to reducing hours worked and/or employment, a firm can also reduce growth in its labour costs by reducing growth in the wages it pays its employees. In this paper, we only consider adjustment in the quantity of labour (employment and average hours worked) and not the price (wages). [2]
In this paper, total hours worked is based on the ABS measure of ‘monthly hours worked in all jobs’ and average hours worked is implied from total hours and employment. [3]
Compositional changes in employment are important for explaining longer-run trends (as opposed to cyclical adjustment) in average hours. In this paper we focus on cyclical adjustment. [4]
The term ‘downturn’ is used in this paper to refer to the recessions in the early 1980s and the early 1990s, and the growth slowdowns in the early 2000s and late 2000s. This captures every period since 1978 (our period of data availability) in which there was an increase in the unemployment rate of at least 1 percentage point over a period of less than twelve months. [5]
The downturn periods shaded in Figures 4–5, 7–9 and D1 are dated based on the peak-to-trough decline in (detrended) total hours worked. [6]
It is also possible that the downturns in the 2000s were less severe because more adjustment occurred through average hours. However, examining this hypothesis is beyond the scope of this paper. [7]
These calculations are based on the total value of employment termination payments (ETP) and income from salary or wages from the Australian Taxation Office (ATO 2015). The value of ETP measures the taxable component of payments related to resignation, dismissal, redundancy, retirement or death. [8]
The EPL index is available from the mid 1980s and covers a range of termination cost indicators, including dismissal costs, procedural inconveniences (i.e. ‘red tape’), notification requirements and the potential compensation if a dismissal is found to be unfair. [9]
The results in Figure 6 are from a VAR(3) model with three variables, ordered as follows: real GDP, average hours worked and employment. Following RBA (2014), this ordering assumes that GDP is the most exogenous variable in the system, with the ordering of the other variables reflecting the relative speed at which they are assumed to respond to a shock to GDP. In turn, GDP is assumed not to respond contemporaneously to any of the labour market variables, but may respond with a lag. All variables in the VAR are in logs and detrended with an HP filter (λ = 1,600). The results from VAR models can be sensitive to the variables included and the structure of the VAR, so the results shown here should be considered as illustrative only. [10]
The ‘within effect’ is not the same as the ‘cyclical’ average hours worked series derived earlier using the HP filter. The ‘within effect’ captures all changes in average hours that are not explained by compositional changes in employment. In contrast, the ‘cyclical’ change in average hours captures all deviations of average hours from trend, due to both cyclical ‘within effects’ and cyclical ‘composition effects’. Nonetheless, the two series are highly correlated, reflecting the limited contribution of compositional effects to cyclical movements in average hours. [11]
These estimates are based on an Oaxaca-Blinder decomposition of the change in average hours worked between August 2008 and August 2009 (using the individual LFS cross-sections at both points in time). Including the parttime dummy attenuates the effects of industry, occupation, gender and age composition, which suggests that the effect of these factors largely operates through their effect on the part-time share of employment. [12]
The dataset is called the Longitudinal LFS (LLFS). It currently includes the full set of individual unit records for each monthly LFS conducted between January 2008 and December 2010. [13]
Actual hours worked include paid or unpaid overtime hours, but exclude hours paid for but not worked, such as paid annual leave, public holidays or paid sick leave. The LFS questionnaire does not give a definition of ‘usual hours’, so it is not immediately clear how respondents interpret the term. [14]
We use the term ‘attrition’ loosely to refer to the situation where an individual is not matched from one mid-quarter survey to the next. This will include workers that drop out of the sample (e.g. those that could not be contacted for a subsequent interview), but also those that are not matched for other reasons, such as being rotated out of the survey panel, being surveyed in a non-private dwelling or becoming ‘out of scope’ of the LFS (e.g. by joining the defence force). [15]
Details on the construction of the longitudinal weights are available from the authors on request. The intuition behind this procedure is that it gives more weight to individuals that have similar initial observed characteristics to those that subsequently drop out of the sample than to individuals with characteristics that make them more likely to remain in the sample. For example, if young people are relatively more likely to drop out of the sample, then we adjust for this fact by giving the young people that do remain in the sample a larger weight, relative to the older people in the sample. Nevertheless, we cannot rule out the possibility that some individuals ‘select out’ of the matched sample based on unobservable factors that we are unable to account for. [16]
Job movers account for 4½ per cent of employment, on average over our sample period, while job finders and job leavers account for 5 per cent of employment in periods t and t − 1, respectively. [17]
The hours adjustment plotted on the y-axis of Figure 10 is calculated as the difference between the average change in hours worked during the downturn (2008:M11 to 2009:M8) and the average change in hours worked during the post-downturn period (2009:M11 to 2010:M8). Normalising relative to the post-downturn period helps to control for trend changes in hours in each industry. Taking averages across four quarterly average changes accounts for seasonality. [18]
This approach is equivalent to estimating a Oaxaca-Blinder decomposition between August 2008 and every other quarter t. However, rather than estimating a separate regression for every quarter in time (along with August 2008), it simply treats any difference between the actual change and the composition effect as the ‘unexplained (or within) effect’. [19]
The implicit assumption is that the unclassifiable population is a random subset of the general population, meaning that the results for the classifiable population can be extrapolated. [20]
With a threshold set at 0.5, job stayers and job movers are correctly classified 99.8 per cent and 8.0 per cent of the time, respectively. Fluss, Faraggi and Reiser (2005) recommend that cut-off probabilities be chosen to maximise the sum of sensitivity and specificity. Our threshold of 0.15 is close to this value. [21]
We also formulated a decomposition that takes the unbalanced nature of the matched sample into account. However, the decomposition terms were hard, if not impossible, to give a meaningful interpretation to. [22]
The change in the average hours worked for a given category of employment (i.e. job stayers) will not necessarily be equal to the average change in the hours worked by employees in that category. These two measures should only be expected to coincide if we were dealing with a balanced panel. Measures of the average change in hours worked by job stayers suggests an even larger decline in the hours worked by job stayers during the downturn than suggested by Figure 9. [23]