RBA Annual Conference – 2007 The Rise of Household Indebtedness Christopher Kent, Crystal Ossolinski and Luke Willard[1]
1. Introduction
A large rise in household indebtedness has been common to many, though not all, advanced economies over the past few decades and is a key feature of the broader trend of financial deepening. This reflects a number of factors, including an easing in credit constraints following financial deregulation – which, among other things, has allowed for greater competition among lending institutions – and a decline in inflation, and nominal and real interest rates. Where these factors have been especially strong, or operated simultaneously, debt has risen rapidly and has typically been associated with a sharp rise in real house prices. For instance, in Australia, the Netherlands and the United Kingdom, the household debt-to-income ratio has increased substantially since the 1980s (Figure 1[2] and Table 1). In contrast, in countries such as France, Germany and Switzerland, indebtedness has increased only moderately over a similar period.
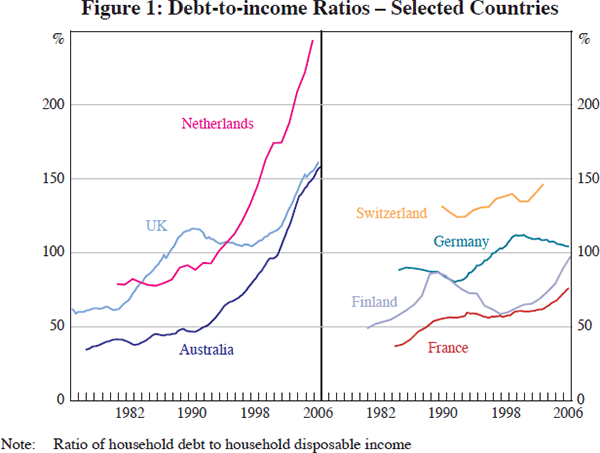
Debt-to-income ratio | Real mortgage rate | ||||||
---|---|---|---|---|---|---|---|
Increase over available sample (% pts) |
Date of inflection(a) | Annual average change since inflection(b) (% pts) |
Level at peak(c) (%) | Date of peak | Change between peak and average 2001–05 (% pts) |
||
Belgium | 6.1 | 0.6 | 6.2 | 1991 | −2.8 | ||
Finland | 48.8 | 1986 | 0.8 | 8.2 | 1992 | −5.0 | |
Germany | 17.0 | 0.9 | 6.3 | 1986 | −1.9 | ||
Switzerland | 14.9 | 1.1 | 3.6 | 1986 | −0.9 | ||
Sweden | 28.9 | 1985 | 1.1 | 6.6 | 1992 | −2.5 | |
France | 13.3 | 1986 | 1.4 | 6.2 | 1986 | −3.6 | |
Japan | 37.5 | 1986 | 1.8 | 6.4 | 1987 | −3.5 | |
Italy | 49.8 | 1988 | 2.6 | 9.6 | 1992 | −6.6 | |
US | 75.5 | 1984 | 2.7 | 8.8 | 1982 | −5.2 | |
Canada | 67.8 | 1986 | 2.9 | 7.6 | 1994 | −3.7 | |
Norway | 91.5 | 1983 | 2.9 | 10.0 | 1992 | −5.4 | |
UK | 95.3 | 1981 | 3.7 | 7.8 | 1986 | −5.1 | |
South Korea | 87.6 | 1987 | 4.6 | 7.3 | 1997 | −4.3 | |
NZ | 91.2 | 1991 | 5.8 | 8.8 | 1988 | −3.9 | |
Spain | 65.4 | 1997 | 7.3 | 6.2 | 1993 | −6.4 | |
Australia | 123.8 | 1992 | 7.3 | 9.3 | 1991 | −5.5 | |
Netherlands | 156.4 | 1987 | 8.4 | 7.1 | 1979 | −4.7 | |
Denmark | 110.5 | 8.8 | 9.3 | 1990 | −6.0 | ||
(a) The year when the increase in the debt-to-income ratio exceeds 5 percentage
points for the first time (after 1975) Sources: BIS; national sources; authors' calculations |
As both sides of household balance sheets have expanded, the debt-to-income ratio may give only a partial impression of the change in the household financial position. Two other key measures of the health of household balance sheets are the gearing ratio – the stock of debt relative to the stock of assets (both housing and non-housing) – and the interest-payments ratio – the flow of interest payments relative to pre-interest disposable income. Figure 2 shows these ratios for those countries for which these data are readily available. Relative to the debt-to-income ratios, these measures have tended to rise considerably less. For example, in Australia the debt-to-income ratio has trebled since the beginning of the 1990s, while the gearing ratio has doubled. The fall in interest rates in the early 1990s means that the interest-payments ratio in Australia has not risen nearly as dramatically. Indeed, the fall in interest rates is one of the reasons for the rise in debt. Similarly, in the Netherlands both the gearing and interest-payments ratios increased more slowly than the debt-to-income ratio, while in the United Kingdom, the gearing ratio and the interest-payments ratio remain below previous peaks despite further increases in the debt-to-income ratio. The relatively slow increase in gearing and fast growth in asset prices also implies that in most countries net worth has risen, both in real terms and as a proportion of income; in Australia, net worth as a proportion of income was 50 per cent higher in 2006 than in 1990. Keeping in mind these other measures of balance sheet developments, in this paper we choose to focus on the debt-to-income ratio, which is readily available across a much wider range of countries.
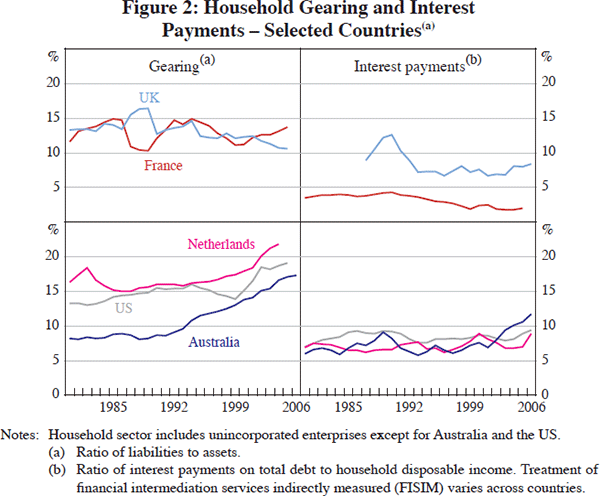
While the trend increase in indebtedness may continue for some time, it is not clear what constitutes a sustainable level of indebtedness over the long run, how rapidly such a level will be reached, or the implications of rising indebtedness for the ability of households to smooth their consumption and continue to meet their repayment obligations in the face of adverse shocks. We attempt to address these issues in three ways. First, we examine factors that have underpinned the rise in household debt over the past two decades or so across a range of developed economies. Second, we present some relatively simple simulations to gauge the extent to which the rise in debt may be linked to changes in the extent of credit constraints.
Third, using a partial equilibrium model we consider the endogenous response of household demand for debt to changes in the level of overall risk in the economy. It is often said that greater debt implies greater vulnerability because it leaves households less able to smooth consumption in the face of adverse shocks and more likely to default for a large enough shock.[3] However, the level of debt is not a sufficient description of vulnerability because it says nothing about the likelihood of adverse shocks. This is important because the rise in indebtedness may have been due in part to a greater ability of households to service debt and an assessment by borrowers and lenders alike that the probability distribution over adverse shocks has shifted favourably. So, for example, while rising debt implies that households will be more vulnerable to a large rise in interest rates, such a rise may be less likely, particularly in an environment of low and stable inflation. It follows that unconstrained borrowers may have decided to increase borrowing, and at the same time credit constraints have eased, including via a relaxation of lending standards by financial institutions. Clearly then any assessment of the implications of debt for vulnerability will need to carefully consider the factors driving up debt.[4]
The remainder of the paper is structured as follows. Section 2 summarises the literature regarding the forces contributing to higher indebtedness. Section 3 builds on this by examining data from 18 advanced economies over the past three decades and establishing a few stylised facts regarding changes in indebtedness and a number of plausible explanatory factors. Section 4 presents simulations regarding changes in supply constraints in order to gauge the relative contributions of various factors to the rise in aggregate household indebtedness. Section 5 uses a simple model in which household debt is determined endogenously to consider the implications of higher debt for the vulnerability of the household sector to adverse shocks. It also introduces an explicit measure of financial system vulnerability relevant to this question. Conclusions are drawn in Section 6.
2. Forces Driving Indebtedness
This section sets out the key theoretical considerations and examines the factors that have been identified in the literature as having contributed to a rise in debt.
Households demand credit for a number of reasons. Perhaps chief among these is the desire to purchase residential property. This reflects the value of obtaining the services provided by owning a home, and is also an important strategy for accumulating wealth, in part because of the beneficial tax treatment afforded to the leveraged purchase of property in many countries.[5] The purchase of such an asset with debt may also be a device that households use to commit to a savings plan. Another reason for going into debt is to smooth consumption over the life-cycle. Subject to individual rates of time preference, and expectations about income and interest rates, individuals will typically borrow early in life when income is relatively low and gradually repay this, building up net assets ahead of retirement. Consumption smoothing in the face of temporary adverse shocks to income may also lead some households to want to incur debt.
In equilibrium, the quantity of debt will also depend on the ability and willingness of financial institutions (and financial markets) to extend credit. This will be affected by a range of factors, including the nature of regulatory controls, the competitiveness of credit markets and the risks associated with lending, which will have a bearing on the extent of endogenous credit constraints. Credit constraints exist because of difficulties associated with asymmetric information. Lenders are unable to determine precisely the ability and willingness of borrowers to repay their debts and, therefore, may be unwilling to lend more (even at higher interest rates) for fear of attracting higher-risk borrowers. In making their decisions, lenders may use various models, rules of thumb or lending standards to guide their decisions about who to lend to, how much and on what terms. Credit constraints will not necessarily be binding for all households all of the time, but to the extent that they are binding for a large part of the population, developments that alter these constraints could have a significant impact on the supply of credit. The extent and nature of credit constraints have evolved (and continue to evolve) across a wide range of countries. One aspect of this is that various structural changes (such as to inflation) can lead to an easing in credit constraints for given lending standards. In addition, financial institutions may have modified their lending standards so as to ease credit constraints (over the course of the past decade or so) – perhaps in response to structural changes and/or their ability to better identify or control the risks associated with lending to particular households.
The literature points to a number of developments which have worked to increase both demand and supply of household credit over the past few decades. Debelle (2004) and RBA (2003a) attribute much of the rise to a reduction in credit constraints due to financial deregulation and the decline in real and nominal interest rates associated with the moderation in inflation. In addition to these factors, the Committee on the Global Financial System (CGFS 2006) emphasises the role of other macroeconomic developments and a range of technological innovations. The key contributing factors can be summarised as follows:
- Financial market flexibility (or completeness). This has a number of aspects. First, there is the extent of deregulation. This took the form of easing entry restrictions for new and/or foreign banks; allowing non-bank financial institutions to compete in the mortgage market; and removing interest rate controls and lending guidelines (Table B1 in Appendix B provides country-specific details). A second aspect, for which deregulation is necessary though not sufficient, is an increase in competitive pressures leading to an easing in credit constraints and a reduction in lending margins. A third aspect, which is likely to flow from deregulated and competitive markets, is the extent of product innovation – that is, the range of different loans available, such as loans requiring limited documentation or products facilitating housing equity withdrawal.
- A reduction in the level of inflation. Financial institutions use a range of criteria to determine the amount they are willing to lend to eligible borrowers. One common rule of thumb is to set the maximum loan such that initial repayments are no more than some amount of the borrowers' income.[6] A decline in inflation that reduces nominal interest rates will ease credit constraints by reducing this initial repayment ratio.
- A reduction in the real cost of funds for financial institutions. Real interest rates declined in many countries after central banks successfully fought high levels of inflation. Greater financial market integration (both domestically and internationally) may also have helped to reduce funding costs. Furthermore, technological innovation has reduced the costs of information and administration associated with lending, driving down margins in competitive markets (CGFS 2006).
- A reduction in macroeconomic volatility. For markets dominated by variable interest rate loans, a fall in interest rate volatility should reduce the risk of default for a given loan amount. It should also reduce funding costs for institutions providing fixed-rate loans. For financial institutions with a diversified pool of loans, a reduction in the volatility of aggregate economic activity could reduce the extent of non-performing loans, leading to lower lending margins in competitive markets. However, some studies suggest that greater macroeconomic stability has been associated with greater flexibility in product and labour markets which could contribute to greater volatility of individual incomes.[7]
- A fall in the unemployment rate and rise in employment growth. A fall in the frequency or expected duration of unemployment spells would be likely to increase both credit demand and supply. Lower unemployment reduces the probability of default by those who have jobs, easing credit constraints as well as lessening the motive for precautionary saving and increasing the value of illiquid assets such as housing (Carroll and Dunn 1997). Also, higher employment adds to the pool of eligible borrowers if financial institutions are reluctant to extend credit to those without savings and/or jobs and reduces the risk of lending to households that gain an extra source of income.
- A rise in the expected rate of income growth. This would make it more attractive to purchase assets (such as housing) using debt. It could also increase the demand for debt relative to current income as households seek to smooth consumption, and will ease credit constraints if financial institutions also expect better economic prospects (Barnes and Young 2003). However, if and when this higher expected growth is realised, it is reasonable to anticipate a decline in indebtedness (as incomes rise but debts do not); otherwise indebtedness might decline through a retrenchment of debt as people revise down their expectations regarding growth prospects.
- Demographic changes. Ageing of the population driven by declines in the rate of fertility may ultimately lead to a reduction in aggregate indebtedness, since older households typically hold less debt. However, increasing longevity (which also contributes to ageing) may lead households to want to hold debt over a longer period. Similarly, with longer working lives, financial institutions may be willing to lend to households later on in their lives.
- Changes in taxes and subsidies may alter the demand for mortgages by owners and investors (Debelle 2004; CGFS 2006; Ellis 2006).
Many of these developments will play out over a considerable period, leading to a gradual rise in indebtedness. Even when these driving forces have stabilised, it may still take time before a new higher steady state level of aggregate indebtedness is reached. This reflects the fact that the response of older generations – particularly those who have passed their prime borrowing years – may be relatively modest in the face of changing circumstances compared with the response of generations that have yet to come of age and will take advantage of the easier credit conditions over their full lifetime.
3. The Rise in Indebtedness – Some Stylised Facts
In this section of the paper we examine the behaviour of the household debt-to-income ratio across 18 countries for which we have data; our sample starts in 1975 but for some countries the data begin as late as the early 1990s. Despite considerable variation, all countries in the sample have experienced some increase in indebtedness (Figure 1 and Table 1). The time at which debt begins to rise more rapidly varies somewhat, with many countries experiencing an upward inflection in the early 1980s (for example, Norway and the UK) while for others this occurred some years later. The behaviour of indebtedness around the longer-term trend also varies, with some countries (such as Australia and New Zealand) experiencing a near-monotonic increase, while others (such as Finland, Norway, Sweden and the UK) have had periods of sharp or protracted declines – associated with periods of widespread financial distress – before resuming an upward trend. Table 1 also shows the average annual change in indebtedness, starting either when the series becomes available (when the sample is limited), or from the point of upward inflection. While the correlation between the total change in debt over the available sample and the average annual change is high (at about 0.8), the latter provides a useful robustness check for the cross-country comparisons given that sample periods differ across countries.
Accompanying the rise in debt, there has been a fall in the real mortgage rate across all countries.[8] There is a fairly consistent trend across countries, with real mortgage rates tending to peak between the mid 1980s and early 1990s and trending down thereafter. Figure 3 shows that those countries that experienced larger declines in mortgage rates since their peaks also tended to experience larger increases in indebtedness. Hence, the rise in indebtedness will overstate the rise (if any) in the ratio of interest payments to income. Shading of the countries indicates two groups according to a measure of financial market flexibility (see below). Those with more flexible markets tend to have had larger rises in indebtedness relative to the trend shown, and vice versa for those with less flexible markets. The decline in real mortgage rates suggests that, at least over the past two decades or so, the rise in debt has been driven by supply expanding more than demand.[9]
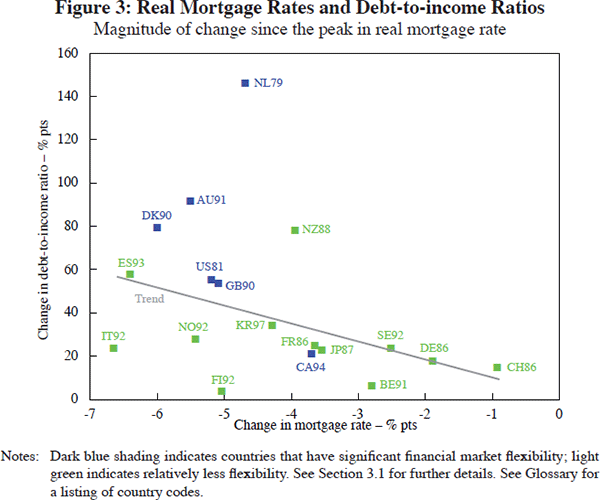
With much of the additional debt channelled into housing markets, it is not surprising that in countries where the rise in debt-to-income ratios has been sizeable, real house prices have also risen substantially (Australia, Denmark and the Netherlands for instance – Figure 4). Likewise, countries that have had smaller rises in debt-to-income ratios have generally experienced smaller increases (and even declines) in real house prices, although there is quite a wide dispersion in outcomes across countries. As housing is not easily traded across borders, domestic factors can create considerable differences in the incentives for owner-occupiers and investors to hold housing. Relevant factors to consider include the extent of public and corporate ownership of the housing stock, the role of non-residents in housing markets, geographic features such as the concentration of the population in key cities, the ability to access housing debt for non-housing investment or consumption (that is, housing equity withdrawal) and government regulations, particularly those relating to taxation arrangements and the balance of rights between landlords and tenants, which influence incentives for investors (Ellis 2005).
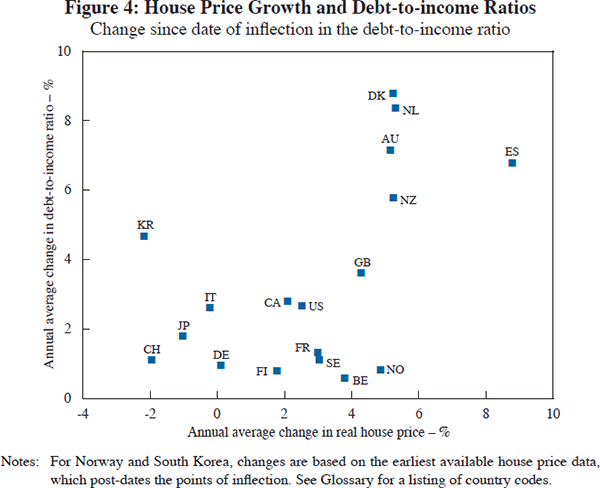
3.1 Explaining the increase in debt-to-income ratios
We can exploit the variation in the behaviour of the debt-to-income ratio across countries to explore whether the factors set out in Section 2 appear to explain rising indebtedness. We use a broad-brush approach suitable for cross-country comparisons in the absence of a long time-series of indebtedness for all countries. For each of the explanatory factors identified in Section 2 for which we can obtain reasonable data, we give countries a score of 2 if that factor changed in a way so as to be likely to cause a (sizeable) rise in debt and 1 otherwise (Table 2). Thresholds for each of the first four variables shown in Table 2 are chosen so as to split the sample of countries in half. Qualitative results are robust to alternative thresholds that place a smaller share of the countries into the low-score category. For a discussion of the timing used in this table and the robustness of results to alternative timing assumptions, see footnote 12.
CPI inflation(a) |
Volatility in output growth(b) | Volatility in nominal mortgage rate(c) | Unemployment rate(d) | Financial market flexibility(e) | Average score | |
---|---|---|---|---|---|---|
Australia | 1 | 2 | 2 | 2 | 2.0 | 1.8 |
Belgium | 1 | 1 | 1 | 1 | 0.5 | 0.9 |
Canada | 1 | 2 | 1 | 2 | 2.0 | 1.6 |
Denmark | 1 | 1 | 2 | 2 | 2.0 | 1.6 |
Finland | 2 | 2 | 2 | 2 | 1.5 | 1.9 |
France | 2 | 1 | 1 | 1 | 0.5 | 1.1 |
Germany | 1 | 1 | 2 | 1 | 1.5 | 1.3 |
Italy | 2 | 2 | 2 | 1 | 0.0 | 1.4 |
Japan | 2 | 1 | 1 | 1 | 0.0 | 1.0 |
Netherlands | 1 | 2 | 1 | 2 | 2.0 | 1.6 |
NZ | 2 | 2 | 2 | 2 | 1.5 | 1.9 |
Norway | 1 | 1 | 1 | 1 | 1.0 | 1.0 |
South Korea | 2 | 2 | 2 | 1 | 1.5 | 1.7 |
Spain | 2 | 2 | 2 | 2 | 0.5 | 1.7 |
Sweden | 2 | 1 | 2 | 1 | 1.5 | 1.5 |
Switzerland | 1 | 1 | 1 | 1 | 0.5 | 0.9 |
UK | 2 | 1 | 1 | 2 | 2.0 | 1.6 |
US | 1 | 2 | 1 | 2 | 2.0 | 1.6 |
(a) Score of 2 if inflation fell by more than 9.5 percentage points, 1
otherwise. Sources: See Appendix A |
We use a more granular and somewhat more subjective approach to scoring financial market flexibility – assigning countries scores of between 0 and 2. The move to greater financial market flexibility – less regulation, greater competition and more product innovation – has followed broadly similar patterns across countries but to differing degrees. With all countries starting from fairly stringent regulations that restricted competition and product innovation in financial markets in the 1970s, the current level of financial market flexibility is likely to be a reasonable proxy for the extent of change over this period (which in turn should influence the increase in debt). Currently, those countries at the less-regulated end of the spectrum – such as Australia, the Netherlands, the UK and the US – have experienced a wide range of reforms: both banks and non-bank financial intermediaries are able to compete in the mortgage market; interest rate controls have been completely removed; there are no longer quantitative restrictions on lending to households; and securitisation of residential mortgages is possible. Competitive pressures have also been relatively strong and product innovation extensive in these economies (Ellis 2005). We assume that an active residential mortgage-backed securities (RMBS) market indicates a more deregulated, more competitive market, so we raise the financial market flexibility score by 1 for those countries that have made extensive use of RMBS; for countries that use RMBS, but only in a limited way, we raise their score by 0.5. The availability of products that facilitate mortgage equity withdrawal (MEW) would also appear to be a reasonable proxy for a flexible market that provides a wide range of loan products, and so we add 1 to the flexibility score of these countries.[10] The total flexibility score across countries (Table 3) accords with qualitative information and our approach is not too dissimilar to that of the Mercer Oliver Wyman (2003) mortgage market completeness index used by Catte et al (2004).[11] Predictably, Australia, Canada, Denmark, the Netherlands, the UK and the US all receive high scores, while countries that have only recently eased the relatively tight regulations governing the lending sector, such as Belgium, France, Italy and Japan, all score quite low.
Use of securitisation | Availability of MEW products | Market flexibility score (0–2)(b) | Memoranda | ||
---|---|---|---|---|---|
Loan term (years) | Loan-to-valuation ratio (%) | ||||
Australia | Yes | Yes | 2.0 | 25–30 | 90 |
Belgium | Limited | No | 0.5 | .. | 90 |
Canada | Yes | Yes | 2.0 | 25 | 70–80 |
Denmark | Yes | Yes | 2.0 | 30 | 80 |
Finland | Limited | Yes | 1.5 | 15–18 | 75–80 |
France | Limited | No | 0.5 | 15 | 80 |
Germany | Limited | Yes | 1.5 | 25–30 | 70–80 |
Italy | No | No | 0.0 | 15 | 80 |
Japan | No | No | 0.0 | 25–30 | 80 |
Netherlands | Yes | Yes | 2.0 | 30 | 87 |
NZ | Limited | Yes | 1.5 | 25–30 | .. |
Norway | No | Yes | 1.0 | 15–20 | 70 |
South Korea | Limited | Yes | 1.5 | 3 | 56 |
Spain | Limited | No | 0.5 | 15 | 70–80 |
Sweden | Limited | Yes | 1.5 | 30–45 | 80–90 |
Switzerland | Limited | No | 0.5 | 15–20 | <80 |
UK | Yes | Yes | 2.0 | 25 | 75 |
US | Yes | Yes | 2.0 | 30 | 80 |
(a) Where sources were inconsistent, the most recently published estimate
was preferred. (b) The sum of the scores for securitisation (1 if ‘Yes’, 0.5 if ‘Limited’ and 0 if ‘No’) and MEW (1 if ‘Yes’, 0 if ‘No’). Sources: ABS, Household Expenditure Survey, Cat No 6530.0; CGFS (2006); Ellis (2005); Girouard and Blondal (2001); Hoeller and Rae (2007) |
We calculate the average score across the different explanatory factors for each country and see how these correlate with changes in the debt-to-income ratios. The scores for each country are presented in Table 2, and are compared to the average annual increase in that country's debt-to-income ratio in Figure 5. There is a clear positive correlation between the average score on the explanatory variables and increases in debt-to-income ratios across countries, although even among countries with similar scores there are a wide range of outcomes. No doubt this reflects the fact that the increase in financial market flexibility and the movement toward greater macroeconomic stability has occurred at different speeds and to a different extent in each country. These results are relatively robust to dropping any one of the explanatory variables from the calculations, and to using the total increase in indebtedness (as per Table 2) in place of the average annual increase in indebtedness.
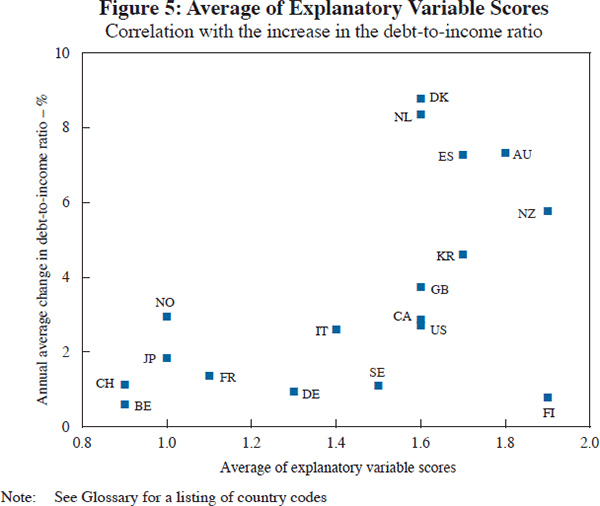
Looking separately at the correlation between each explanatory factor and indebtedness may shed further light on their explanatory power. Figures 6–8 illustrate these bivariate correlations for up to 18 countries (subject to data availability).[12] In each case the trend is shown excluding the Netherlands, which appears to be a consistent outlier (see Section 3.2). Each figure also illustrates financial market flexibility (see below).
Financial market deregulation by itself does not appear to have been sufficient to initiate a sustained increase in the debt-to-income ratio, although there are strong links for certain countries (see, for example, Casolaro, Gambacorta and Guiso 2006 for a discussion of Italy's experience). If we compare the timing of deregulation across countries with the timing of the acceleration in debt we find no strong correlation – for many countries, such as Sweden and the UK, the debt-to-income ratio began to increase before the major elements of deregulation were completed. However, less-regulated credit markets might mean that other structural changes are more likely to lead to an adjustment in borrowing and lending practices and increases in debt. Consistent with this, we find that countries with flexible financial markets (a score of 2, as indicated by dark blue boxes) tend to lie above the trend lines in Figures 6 and 7, while countries with relatively less flexibility (scores of between 0 and 1.5 – light green boxes) tend to lie close to or below the trends.
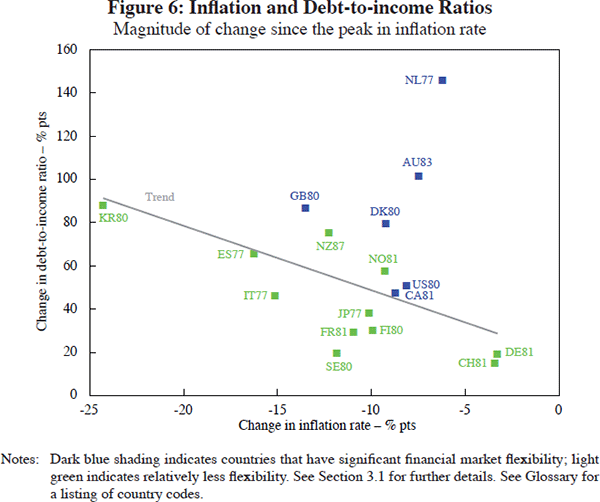
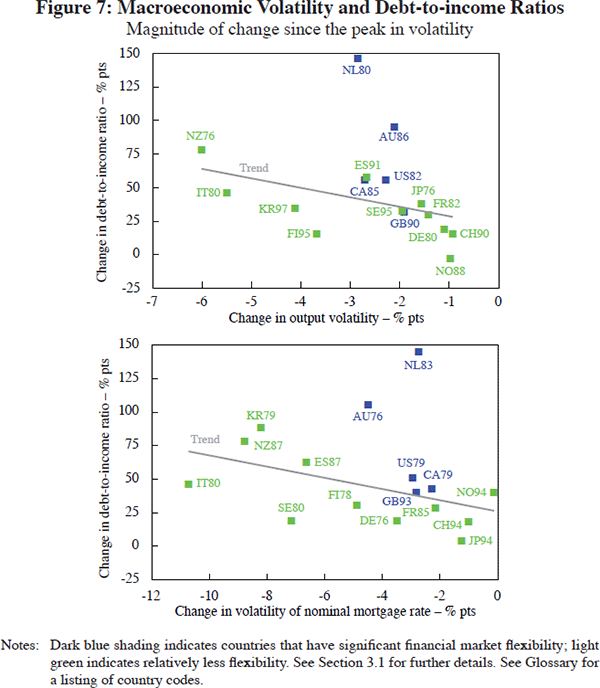
Figure 6 shows that there is a clear positive, though weak, correlation between the fall in inflation and the average annual increase in the debt-to-income ratio. In Australia, the fall in inflation has been given considerable prominence as an explanation for the rise in debt, given the widespread use of initial repayment rules by lending institutions (at least over much of the sample period). But in countries where the more binding constraint is a low maximum LTV ratio, such as in Italy until the late 1990s, there is likely to be a much weaker direct link between the fall in inflation and the rise in debt.[13]
To examine the potential role of the ‘Great Moderation’ we compare the reduction in the volatility of output growth and nominal mortgage rates with the rise in the debt-to-income ratio across countries (Figure 7). There is a positive correlation between the fall in volatility (of output and mortgage rates) and indebtedness, although there is some variation around these trends. This may suggest that macroeconomic volatility has had a relatively modest effect on debt, or that the fall in volatility has coincided with a fall in the rate of nominal income growth (due to declining inflation), which may have contributed to a slower repayment of debt (for example, if households repay according to a fixed share of their income – see Debelle 2004). It may also be that the volatility of individual households' income has not fallen in line with the volatility of aggregate economic activity.
Figure 8 demonstrates what is perhaps a surprisingly close correlation between the fall in the unemployment rate and the increase in the debt-to-income ratio across countries. This may indicate a key role for this explanatory variable due to its potential to boost the supply of debt. It may also reflect the fact that the decline in unemployment has an especially large correlation with the extent of financial market flexibility (of about –0.4 compared with close to zero correlation between each of the other explanatory variables and financial market flexibility). This may reflect a tendency for countries to deregulate and encourage greater competition across a number of different markets at the same time (indeed the financial flexibility score has a correlation of 0.8 with an OECD measure of product market regulation for 2003). This correlation explains why countries (other than the Netherlands) tend to be clustered more closely around the trend shown in Figure 8 (irrespective of their financial market flexibility scores) when compared with Figures 6 and 7. Finally, it could be that a decline in unemployment is also capturing important demand-side factors that have acted to boost debt.
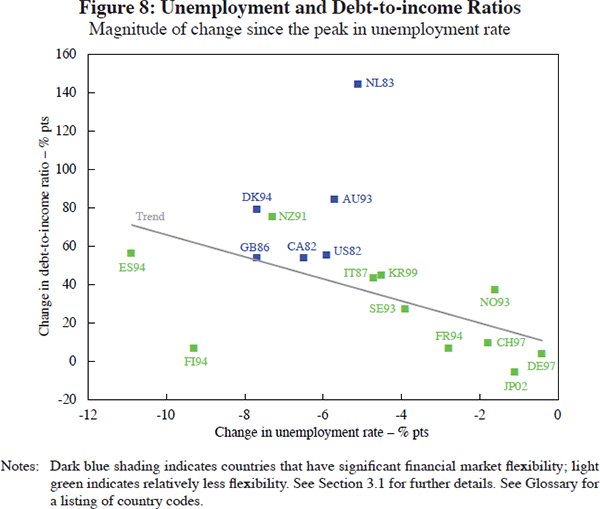
3.2 Differences across countries
While developments affecting indebtedness have a number of aspects that are common across most countries, important differences remain. These often reflect variation in tax laws as well as geographical and cultural factors. The Netherlands, for example, is a consistent outlier, having experienced the largest increase in the debt-to-income ratio, but only relatively average changes in many of the explanatory factors considered above. This is likely to be due to the extensive credit market deregulation combined with a tax system that encourages households to expand both sides of their balance sheet. With no regulations governing LTV ratios it is common practice to borrow enough to cover all the expenses related to moving house, including the transaction costs; in 2001–02 over 70 per cent of mortgages had an initial LTV ratio of over 100 per cent (Debelle 2004). In addition, households in the Netherlands make extensive use of products designed to exploit the tax deductibility of interest payments, which promote a slow rate of repayment. Over 90 per cent of mortgagees do not repay any principal over the life of the loan. Instead, they make compulsory payments into savings or investment accounts, and use the earnings on the account to repay the loan upon completion (indeed, Debelle 2004 suggests that debt should be measured net of funds in these accounts). Finally, during the 1990s, lenders expanded the types of income they would consider for calculating the initial debt-servicing ratio, contributing to a further easing of credit constraints (Debelle 2004).
Australia also lies above the trend lines shown in Figures 6 to 8. Again, some features of the Australian housing and credit markets may help to explain this. In particular, as discussed in RBA (2003b), demand by investors played an increasingly significant role in the growth of household debt from the late 1990s, driven by a combination of an expectation of significant capital gains for property, increasingly easier access to finance for investors, and the tax treatment of investments in residential property. Ellis (2006) also points to regulations that favour the rights of landlords over tenants in Australia compared with other countries.
Finland, Norway and Sweden stand out as having experienced sharp increases in debt earlier than most countries, followed by a sharp correction in the early 1990s. Deregulation appears to have played a key role in these events, with rapid deregulation of credit markets in the mid 1980s leading to credit and asset-price booms. Taking Norway as an example, house price controls and quantitative lending guidelines were removed between 1983 and 1986, yet interest rates were held low by government guidelines and international capital movements remained regulated until 1990. As a result, there were rapid rises in both the price of domestic assets and credit until the recession and financial crisis in 1991 (Steigum 2004). In all three countries, it took over a decade for household debt-to-income ratios to return to their peak levels of the late 1980s.
4. Some ‘Back of the Envelope’ Calculations Regarding Indebtedness
The previous section provides some evidence regarding plausible factors contributing to rising indebtedness, but does not address the relative importance of each factor, the likely long-run level of indebtedness or the time it might take to reach this level. A few studies have examined these issues using calibrated models with households choosing debt optimally, though they have difficulty capturing key aspects of the data. Barnes and Young (2003) use an overlapping generations (OLG) model for the US, but suggest that an easing in credit constraints – which they do not model – may have been an important driver of the upward trend in debt since the 1980s.[14] Campbell and Hercowitz (2006) present a model that focuses on one aspect of regulatory change that led to an easing in credit constraints in the US in the early 1980s; although Hurst's (2006) discussion of that paper suggests a number of other developments may have been important for rising indebtedness from the 1990s onwards. The approach we adopt in this section is instead to assume that credit constraints bind for all households and that financing and repayment behaviour follow a few simple rules of thumb. We consider likely paths of individual indebtedness over the life-cycle under different scenarios and different rules of thumb (of borrowers and lenders), and then calculate economy-wide measures of the debt-to-income ratio. This is similar to the approach used by RBA (2003a), Debelle (2004) and Ellis (2005), though the exact assumptions used vary between papers.
Key features of our OLG simulations are loosely based on the Australian market. In our benchmark scenarios all individuals work from age 20 to 64 and borrow at age 30 (we allow for re-borrowing later on). They are credit-constrained and borrow an amount such that repayments on the loan are initially 30 per cent of their income. This rule of thumb has been used by many lending institutions in Australia (RBA 2003a), although we also discuss alternatives in line with evidence that this has more recently been replaced by less-constrained rules/models (Laker 2007). In our benchmark scenarios we assume no downpayment (or LTV ratio) constraint, though we discuss the implication of such a constraint in the context of a scenario that also allows for unemployment and default.[15] Individuals finance their debt using a credit-foncier loan over 30 years and their incomes grow by 2 per cent in real terms per year. To obtain an economy-wide measure of indebtedness, we assume population growth such that each year the cohort entering the labour force is 2 per cent larger than the previous cohort (Appendix C outlines some of the relevant calculations for this section of the paper).
Figure 9 shows the evolution of the debt-to-income ratio over an individual's life for two key baseline scenarios. The first is a high inflation, high interest rate scenario (roughly matching the Australian experience of the late 1980s when inflation and the real mortgage rate were about 8 and 6½ per cent, respectively). The second is a scenario with low inflation (2½ per cent) and a low interest rate (3½ per cent), representative of recent Australian experience. Figure 9 also provides a sense of the contribution of each of these factors in isolation.
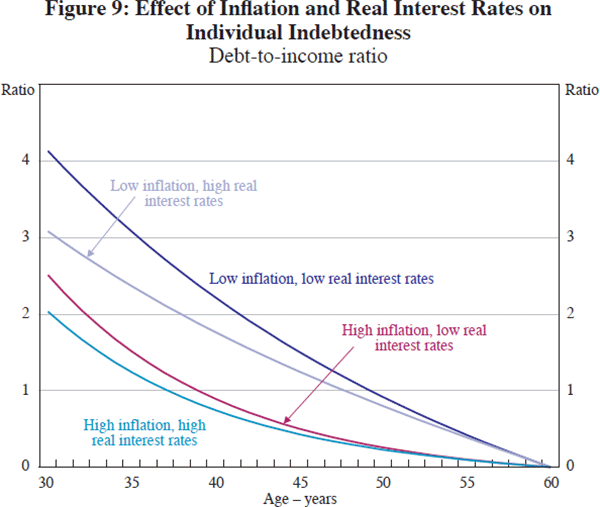
4.1 Aggregate indebtedness under different scenarios
Table 4 shows the economy-wide steady state debt-to-income ratios obtained by aggregating these results across individuals (assuming all individuals are credit-constrained). Baseline results, shown in row I, suggest that the decline in inflation has been a major determinant of the rise in indebtedness, though the decline in real interest rates also seems to have played an important role.
I Baseline assumptions | High inflation, high interest rates | High inflation, low interest rates | Low inflation, high interest rates | Low inflation, low interest rates |
---|---|---|---|---|
46 | 56 | 96 | 122 | |
II Additional assumptions | 10% unemployment | 5% unemployment | ||
44 | 119 | |||
III Additional assumptions | 1989 age distribution | 2040 age distribution | ||
49 | 121 | |||
IV Additional assumptions | 1989 age distribution and non-uniform wages | 2040 age distribution and non-uniform wages | ||
45 | 110 | |||
V Additional assumptions | 30% income share on repayments | 30% income share on repayments | ||
25 | 87 | |||
VI Additional assumptions | As per baseline above | One-off refinancing/trading up after 5 years | ||
46 | 166 | |||
Notes: Relevant baseline assumptions apply for all columns. The additional assumptions are relevant only to the relevant rows (that is, they do not cumulate). Population distributions are those of the Productivity Commission (2005). |
One dimension in which the above simulations could be considered unrealistic is that they assume that all individuals are employed. There are a number of simple ways to incorporate the likely effect of unemployment on indebtedness in this framework. Unemployment could be viewed as affecting everyone equally with relatively short unemployment spells, which do not affect the ability to service loans or save for a deposit, but which prevent banks from granting loans. Under this scenario, unemployment has modest effects on indebtedness. Row II of Table 4 reports the case where the share of the population unable to borrow (at age 30) is equal to the unemployment rate (with unemployed persons having an income that is half the working wage).[16]
We might expect unemployment to have a larger effect if unemployment spells persist for some time, and in the presence of a downpayment constraint. In this case, unemployment is likely to decrease indebtedness by reducing the size of the initial loan (if unemployment occurs while saving for a deposit) or by increasing the extent of defaults (if unemployment occurs while the loan is being repaid). Defaults will act to reduce indebtedness by eliminating the debt associated with the current loan and by reducing the amount of equity available as a deposit and, in our model, the size of any new loan. However, even under these conditions, and assuming that the probability of being unemployed in any year is equal to the unemployment rate, changes in the unemployment rate have only a modest effect on aggregate indebtedness. For example, we consider a scenario that assumes that those that become unemployed after age 45 default on their debt, while those unemployed at age 30 never take out a loan. In this case, the level of indebtedness rises from 42 per cent when unemployment is 10 per cent (and inflation and interest rates are high) to 115 per cent when unemployment is 5 per cent (and inflation and interest rates are low).[17] One limitation of these simulations is that they cannot account for endogenous changes in the supply and demand for credit associated with a reduction in the risk of shocks to income, including via unemployment.
The baseline scenarios can be extended by accounting for the effects of changes in the population age structure and moving away from the assumption that individuals across the age distribution earn the same income in a given year. First, we use the 1989 age distribution (for the high inflation, high interest rate scenario) and compare this to the results based on the projected 2040 age distribution (for the low inflation and low interest rate scenario) based on estimates for Australia (Productivity Commission 2005). Even though the median age of the population is projected to rise by about 5 years across these two scenarios, this has only a minor impact on the aggregate debt-to-income ratios (row III). If we also assume that there is an upward-sloping wage profile over the life-cycle reflecting the benefit of experience (and matching the wage profile in Kulish, Smith and Kent 2006), the rise in the debt-to-income ratio is not as great as in the baseline scenarios, reflecting the lower debt ratios of older workers (row IV).[18]
Another dimension in which the baseline scenarios can be extended is to alter the speed with which debt is repaid since in practice debt tends to be paid off more quickly than the maximum specified in loan contracts (assumed to be 30 years for our purposes). If it is assumed that individuals spend a constant 30 per cent share of their income on servicing the debt over the life of the loan, then debts are repaid in roughly 15 years (the exact outcome depends on factors like inflation). As debt is paid off much more quickly in this scenario, the initial and new levels of indebtedness are both much lower (row V). Of potentially greater interest is the fact that the debt-to-income ratio associated with the move to low inflation and low interest rates under this alternative scenario is much larger in proportion to the starting value of the ratio, reflecting in part the slower rate of debt repayment when nominal income growth falls.
There are a number of other factors that could have some ongoing influence on levels of indebtedness. One is the effect of increased longevity. Exactly what the effects of increased longevity may be is unclear, but it seems likely that longer life spans might increase the retirement age and lead to a roughly proportional increase in the number of years people are willing to remain in debt. Certainly there is evidence of this in Australia, with substantial increases in the share of older households with owner-occupier debt (RBA 2006). One way of capturing this is to assume that loan periods and working lives expand so that they remain roughly constant as a share of total life spans. Under the scenario that life expectancy has risen from about 75 to 82 years, the expected retirement age would increase from 64 to about 69 years and the loan life from 30 to 33 years. This scenario would lead to only a modest rise in indebtedness to about 125 per cent (compared to 122 per cent in the baseline scenario). If instead the loan life increased without a commensurate increase in longevity, the level of indebtedness would increase to about 133 per cent.[19]
A factor that may have an important effect on indebtedness is the extent to which existing borrowers increase their debt either by refinancing their loan on an existing property or obtaining a new loan as they trade up to a more valuable property. While it is hard to know the extent to which this may have increased over time, some increase is consistent with evidence cited above of the rising share of older households with owner-occupier debt, as well as a rise in refinancing as a share of total credit in Australia over recent years and a trend increase in MEW. Among other things, this behaviour might reflect the effect of rising longevity. Again this is speculative but, given available information, it is plausible that households refinance their initial loan after five years such that the new loan is 1.4 times the size of the outstanding balance of the loan (during the year prior to refinancing).[20] If the contract is for another 30 years and inflation and interest rates are low, the steady state level of indebtedness would be 166 per cent.
What about the effect of an easing in credit constraints due to financial institutions altering the rules of thumb or lending standards they use to determine eligibility for, and the magnitude of loans? In Australia, as elsewhere, there is certainly evidence that financial institutions have been providing credit more readily than in the past (at least up until the period of financial market turmoil in the second half of 2007; on the earlier relaxation of credit constraints, see Laker 2007 for the case of Australia; Fernandez-Corugedo and Muellbauer 2006 discuss the UK; and Bernanke 2007 discusses the US). This has involved lenders altering the basis of their lending standards in a number of ways that do not translate readily into rules based on initial repayment ratios. Nevertheless, the effect of these adjustments will be proportional to the effect that they have on the initial repayment ratio (for credit-foncier fixed repayments). So, for example, if the repayment on the loan were to rise from 30 to 40 per cent of initial income (and all households borrow according to this relaxed standard), the steady state level of indebtedness would rise from 122 to 163 per cent (under baseline assumptions with low inflation and low interest rates).
4.2 Transition between steady states
It is worth considering the likely transition path between different steady states since OLG models imply that it will take time for indebtedness to adjust to changing conditions. This is because an unexpected relaxation of credit constraints is less relevant for older households that have typically accumulated assets and paid down their debts, while it will provide new opportunities for younger borrowers to increase and possibly maintain higher levels of debt than would otherwise have been the case. Also, to the extent that contracts are fixed (either in terms of the interest rate or the ability to change the length of the loan or the amount borrowed), the capacity to adjust will be limited. This implies a gradual transition to higher levels of indebtedness as new generations take advantage of easier credit as they come of age. A simple scenario to consider is a one-off shift where existing borrowers do not adjust their level of borrowing but maintain the same life of the loan and loan rates are fixed (obviously this is not realistic for Australia, where loans are typically based on flexible interest rates, but it simplifies the calculations significantly and is likely to have only a small effect on the transition between steady states). Under such an assumption and assuming a 30-year contract, it takes 30 years for complete adjustment under the baseline scenarios (as it takes this long for debts incurred during the old regime to be repaid). However, about 80 per cent of the adjustment occurs within 12 years. If instead individuals spend a constant 30 per cent share of their income on servicing the debt over the life of the loan (as per the scenario outlined in row V of Table 4), complete adjustment to the new steady state occurs within about 15 years and about 80 per cent of the adjustment occurs within 10 years (Figure 10).
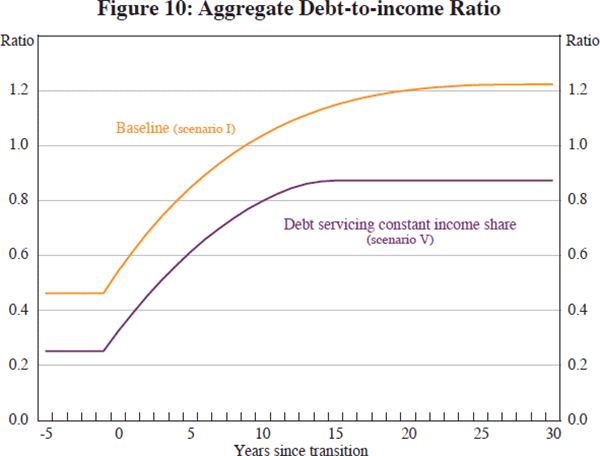
In summary, these simulation exercises suggest that declines in inflation and, to a lesser extent, real mortgage interest rates (which have fallen by less in percentage point terms) have made a sizeable contribution to the rise in indebtedness in Australia. It seems likely that the effects of the decrease in inflation played out over a number of years but that they have probably largely flowed through to aggregate indebtedness. It is plausible that other factors like lower unemployment and increased longevity have also played some role, although the effect of these types of changes cannot be fully captured without considering the endogenous response of demand and supply to these factors. The trend of general reductions in credit constraints across a number of countries over recent years (and notwithstanding events of recent months) may continue to increase indebtedness for some time.
5. Implications for Consumption Behaviour and Financial Stress
In this section of the paper we attempt to assess the implications of rising household indebtedness for the vulnerability of the household sector to adverse shocks. We do this in a framework that explicitly defines financial vulnerability and models the household's decision regarding their optimal level of debt, incorporating their preferences for, and understanding of, the degree of risk and how it might be changing over time. This part of the modelling exercise is not dissimilar to that performed by Barnes and Young (2003) for the United States and Tudela and Young (2005) for the United Kingdom. While these papers present more complex models, which are calibrated so as to match both aggregate and individual level data, they do not consider changes in credit constraints or the degree of uncertainty, which we attempt in a simple way.
5.1 A definition of financial system vulnerability
Before presenting the model of the household's decision, we define a measure of financial system vulnerability (or risk) and highlight its relevance to developments affecting the household sector. For a policy-maker charged with maintaining financial system stability, perhaps the chief concern with respect to the household sector is to avoid situations of widespread financial distress. At the very least, such distress can lead to a curtailment of household consumption with adverse consequences for the macroeconomy.[21] In cases of more acute and widespread distress, a large share of households may find themselves unable (or unwilling) to service their debts, leading to the possibility of a forced sale of assets and significant losses for lending institutions, both of which could act to trigger and/or exacerbate a macroeconomic downturn.[22] The framework we adopt loosely matches that described in Kent and Debelle (1999). In particular, the policy-maker cares about macroeconomic losses (to be defined more carefully below) associated with financial stress that could occur in various adverse states of the world. Though our focus is on households, the concept is broadly consistent with Schinasi (2004) who emphasises the ability of the financial system to facilitate economic transactions, manage risks and absorb shocks.[23] As well as allowing for the possibility that financial institutions may be weakened to the extent that they can no longer provide sufficient funding to meet the demands of households, our definition also allows for the possibility that household expenditure may be constrained or even substantially curtailed if they are unable to borrow sufficient funds in the face of a temporary adverse shock.
We assume that the macroeconomic losses that occur in adverse states of the world (indexed by i) can be quantified as L(i|Ωt), where losses are conditioned on Ωt, which summarises the state of the world at time t. This feature allows the losses to be path-dependent, such as on the current level of debt, for example. If necessary, losses measured in units of output could be transformed in order to capture the preferences of the policy-maker, who may be averse to the risk of especially large losses. In any case, losses could be based on the cumulative deviations of output below some threshold level, with output above this being assigned a loss value of zero, representing relatively benign states of the world. Such a threshold could be specified in terms of potential output with strictly positive losses arising when output was sufficiently far below potential.[24] This captures the notion that macroeconomic cycles are normal events that policy-makers cannot help to avoid altogether, but that they would like, where feasible, to avoid periods of especially weak economic activity associated with financial instability. Of course, in practice it may be quite difficult to distinguish between financial stability concerns and more general macroeconomic stability concerns.
To complete the definition of financial system vulnerability requires us to account for the likelihood of different states of the world. We assume that these can be described by a probability density function, f(i|Ωt), where again the distribution is conditioned on the current state of the economy. Combining these two elements we can in principle construct an index of financial system vulnerability as follows:
In short, this is the expected macroeconomic loss resulting from adverse shocks, which is over and above the loss associated with a ‘standard’ economic cycle reflecting the effect of financial distress. Such an index accounts for the notion that conditional on a shock of a particular type/size, higher current levels of debt might imply a larger macroeconomic loss. However, it also allows for the possibility that the probability of such a shock may have diminished, and that this may have helped to spur the rise in debt in the first place.
5.2 A model of household debt
This section of the paper presents a very simple two-period partial equilibrium model in which the motive for debt is to smooth consumption over the life-cycle. We briefly discuss other motivations for debt later in the paper – in particular, consumption smoothing in the face of temporary shocks – but do not model these explicitly. Households are assumed to live for two periods, derive utility from consumption in each period (c1 and c2, respectively), and earn (non-interest) income in both periods, w1 and w2. For simplicity only the latter is subject to uncertainty as follows:
where is strictly greater than
. To ensure that aggregate consumption varies over
states of the world, we assume that the forces leading to low income operate
equally across all households – that is, the shocks are not independently
distributed. (We also incorporate an idiosyncratic shock to income in the second
period conditional on the bad state of the world, l, in order to allow
for default.)
Preferences and parameter values are such that households borrow in period 1, up
to a limit . The household's problem is to choose debt
in the first period (d1 ≡ c1
— w1) to maximise expected utility:
where: β is the discount rate; r is the risk-free interest rate; ρ is the premium that compensates lenders for expected losses; u(○) is the within-period, strictly increasing utility function, which displays some degree of risk aversion; and E(○) is the expectations operator.
We allow for the possibility of default in an elementary way by assuming that in
the low-income state of the world an individual household experiences default
with probability q. Specifically, in the low-income state of the world, households
are susceptible to an idiosyncratic adverse shock that would reduce their income
below , by an amount equal to a share α
of their debt. We assume that by defaulting, the same value of their debt is
forgiven – that is, their disposable income after default is the same
as it is for
non-defaulters[25].
Lenders charge a premium,
, so as to satisfy a zero expected profit condition.
A certainty-equivalent level of consumption in period 1, Ĉ1, can be derived by assuming that households receive income in period 2 equal to E(w2) and that credit constraints do not bind. With log utility, households would consume a share, 1/(1 + β), of the expected net present value (NPV) of lifetime income:
where for β = 1, Ĉ1 = Ĉ2. However, with uncertain income and risk aversion, consumption in period 1 will be less than the certainty equivalent. This difference, I ≡ Ĉ1 — c1, can be thought of as a measure of self-insurance against the possibility of low income in period 2, and is inversely related to the degree of consumption smoothing. If the probability of the low-income state of the world declines, households will have an incentive to respond by increasing debt. In this way, the fall in the risk of low income is ‘offset’ by households taking out less insurance against that outcome (borrowing more), leaving them more vulnerable if that low-income state of the world actually occurs.[26] Households will also increase debt if binding credit constraints are eased, again leaving them more vulnerable to adverse shocks. These are two key features of this model.
The response to an easing in credit constraints may appear to run counter to the idea that such an easing may better enable borrowers to smooth consumption in the face of temporary shocks to income. To consider such shocks formally would require the model to be extended to at least three periods. However, the key results of the two-period model will still be relevant in the following way. In a three-period extension of the model, borrowing in period 1 would help to smooth lifetime consumption while borrowing in period 2 could occur in the event of a temporary adverse shock. Because both of these possibilities will be valued by households, we would expect that an easing in (binding) credit constraints would be used on both fronts – that is, borrowing more in period 1 to allow for more life-cycle consumption smoothing, but holding some borrowing capacity in reserve to help insure against a temporary adverse shock in period 2. It would be worth exploring how this extra borrowing capacity would be apportioned to these competing demands and the implications of this for consumption smoothing in further research. One important practical consideration here though is that financial institutions may be reluctant to extend credit in the face of an especially adverse temporary shock that affects a large share of the household sector, reducing the scope for consumption smoothing and increasing the value of liquid assets and products that allow households unconditional access to extra debt.
Returning to the two-period model, we suppose that the losses relevant to the instability
index, L(i|Ωt), are zero in the high-income
state of the world (that is, L(i = h|Ωt)
= 0) and are proportional to the fall in consumption from period 1 to period
2 in the low-income state of the world. In what follows, we assume that . We abstract from the costs associated with default
since the lending premium is such that lenders are fully compensated for expected
losses. At the expense of increased complexity, we could assume that default
is a costly option for households, thereby providing them with an incentive
to cut back on consumption in the second period
of their lives if they were otherwise at risk of default. This would imply
lower consumption for households that are close to, or in default, leading
to a larger loss L(i|Ωt).
In the following sections we consider the implications of this model for the degree
of consumption smoothing (that is, the inverse of I),
financial vulnerability (V) and welfare (EU) in the face
of developments that would lead to a rise in debt. We illustrate key features
of the model by assuming log utility and using the following baseline parameterisation:
w1 = 1; = 1.5; r = 0.2; β = 0.83;
q = 0.2; and α = 0.5. We consider a range of values
for the probability of the low-income state of the world, p, and for
the level of
(from 0.3 to up to 1.4).
5.2.1 An easing of credit constraints
Consider the effect of an easing of the credit constraint (a rise in , from 0.05 to 0.2); we discuss why this might occur
below. To the extent that the constraint was initially binding, households
will borrow more and increase the degree of their consumption smoothing; that
is, the level of insurance, I, against low consumption in period 2
will fall. Obviously, this easing in the constraint increases welfare (EU
rises). However, because debt rises, the loss in period 2 associated with the
low-income state, L(i = l | d1),
increases.
The impact of this on the index of financial vulnerability, V, will depend crucially on factors that have driven the change in
the credit constraint. At one extreme is the case where the probability of
the low-income state is unchanged. This could occur via competitive pressures
leading to reductions in , independent of other factors affecting risk in
the economy. In this case, the index of financial vulnerability, V,
will rise. This is not to say that this is necessarily a bad thing, since social
welfare and the measure of vulnerability are not one in the same. By focusing
on adverse outcomes, the measure of vulnerability fails to account for the
value to the household of being able to raise consumption in period 1 at the
expense of risking lower consumption in period
2.[27]
Another case to consider is one in which credit constraints are relaxed by either a regulator or by lending
institutions responding to factors that imply a reduction in the risk of adverse
shocks. If such a change is consistent with what a well-informed and prudent
borrower would choose to do in the absence of binding constraints, then it
need not imply an increase in financial vulnerability and will enhance social
welfare. However, an easing of constraints that goes beyond this may be of
concern, particularly if households are not well-informed about the risks they
face.
5.2.2 A decline in ‘risk’
The effect of a decline in the probability, p, of the low-income state of the world can be considered more explicitly as follows (with d unrestricted and other parameters as above). This change may reflect a number of structural changes that increase the likelihood of higher income in period 2. One possibility is a decline in macroeconomic volatility, although this would not necessarily impinge favourably upon q (as discussed in Section 2). Nevertheless, the decline in p has the effect of lowering the premium, ρ, required to compensate lenders for expected losses (Table 5).
![]() |
||||||||
---|---|---|---|---|---|---|---|---|
p | ρ | 0.3 | 0.4 | 0.5 | 0.6 | 0.7 | 0.8 | 0.9 |
0.05 | 0.005 | 0.119 | 0.084 | 0.058 | 0.040 | 0.027 | 0.018 | 0.011 |
0.10 | 0.010 | 0.150 | 0.114 | 0.085 | 0.061 | 0.043 | 0.030 | 0.020 |
0.15 | 0.015 | 0.165 | 0.130 | 0.100 | 0.075 | 0.055 | 0.039 | 0.026 |
0.20 | 0.020 | 0.172 | 0.138 | 0.109 | 0.083 | 0.062 | 0.045 | 0.031 |
0.25 | 0.026 | 0.174 | 0.142 | 0.113 | 0.088 | 0.066 | 0.049 | 0.034 |
0.30 | 0.031 | 0.172 | 0.142 | 0.114 | 0.090 | 0.069 | 0.051 | 0.036 |
0.35 | 0.036 | 0.168 | 0.139 | 0.113 | 0.090 | 0.069 | 0.052 | 0.037 |
0.40 | 0.042 | 0.161 | 0.134 | 0.110 | 0.088 | 0.069 | 0.052 | 0.037 |
0.45 | 0.047 | 0.153 | 0.128 | 0.106 | 0.085 | 0.067 | 0.051 | 0.037 |
0.50 | 0.053 | 0.143 | 0.121 | 0.100 | 0.081 | 0.064 | 0.049 | 0.036 |
Notes: The upper-right region is one of indebtedness; the lower-left region is one of saving. Shading indicates maximum self-insurance. |
Debt rises monotonically as the probability of low income in period 2 declines. In
contrast, the relationship between the extent of consumption smoothing and
p follows a U-shaped pattern (for constant , with the least amount of consumption smoothing
(maximum insurance, I) occurring at intermediate levels of p (in the case
where
. These points are shaded in Table 5 for selected
values of
; the upper-right portion of the table indicates
combinations of p and
consistent with debt. If we consider only those
parameter values for which the household is willing to take on debt, the extent
of consumption smoothing increases as p declines (from sufficiently
low levels). Because debt rises unambiguously with the decline in p,
so too does the loss, L(i = l | d1).
However, for low enough p this is more than offset by the decline
in the probability of the low-income state so that the index of vulnerability,
V, declines with p (for values of p less than 0.35
for the parameters underlying Table 6). Expected utility is always increasing
with the decline in p.
![]() |
||||||||
---|---|---|---|---|---|---|---|---|
p | ρ | 0.3 | 0.4 | 0.5 | 0.6 | 0.7 | 0.8 | 0.9 |
0.05 | 0.005 | −0.044 | −0.043 | −0.041 | −0.038 | −0.035 | −0.031 | −0.027 |
0.10 | 0.010 | −0.075 | −0.073 | −0.071 | −0.067 | −0.062 | −0.056 | −0.049 |
0.15 | 0.015 | −0.097 | −0.096 | −0.093 | −0.088 | −0.082 | −0.075 | −0.066 |
0.20 | 0.020 | −0.114 | −0.112 | −0.109 | −0.104 | −0.097 | −0.089 | −0.079 |
0.25 | 0.026 | −0.125 | −0.124 | −0.120 | −0.115 | −0.108 | −0.099 | −0.088 |
0.30 | 0.031 | −0.132 | −0.131 | −0.127 | −0.122 | −0.115 | −0.105 | −0.094 |
0.35 | 0.036 | −0.135 | −0.134 | −0.130 | −0.125 | −0.118 | −0.108 | −0.096 |
0.40 | 0.042 | −0.135 | −0.133 | −0.130 | −0.125 | −0.117 | −0.107 | −0.096 |
0.45 | 0.047 | −0.131 | −0.130 | −0.126 | −0.121 | −0.113 | −0.104 | −0.092 |
0.50 | 0.053 | −0.124 | −0.123 | −0.119 | −0.114 | −0.107 | −0.098 | −0.086 |
Notes: The upper-right region is one of indebtedness; the lower-left region is one of saving. Shading indicates maximum self-insurance. |
Now consider a fall in the lending premium associated with a decline in the share, q, of borrowers in default in the low-income state (from 0.2 to 0.1) and in the loss-given default α (from 0.5 to 0.25). This may reflect a more benign macroeconomic environment, such as a decline in the structural unemployment rate. The impact of this on the lending premium increases roughly in proportion with p, hence the increase in debt in response to this change is much larger for higher values of p. However, the rise in debt is not so large as to offset the positive impact on income (after interest payments) for debtors in period 2. For this reason the loss associated with low income in period 2, L(i = l | d1), declines. Accordingly, the measure of vulnerability, V, also declines.[28]
5.2.3 Accounting for structural differences across countries
We briefly discuss three differences in the structure of credit and housing markets across countries that could have important implications for these types of models (a formal treatment is beyond the scope of this paper). The first is the role of assets, both housing and financial. Much of the rise in debt across a range of countries appears to have been used to purchase housing. In some countries, tax incentives (and/or direct subsidies) have encouraged leveraged purchases of property (including for the purpose of pure investment). These incentives may also encourage households to maintain debt for longer than might otherwise be the case. However, this does not necessarily imply higher net debt, since households may choose to accumulate wealth in the form of financial assets. But whatever the motivation behind greater gross (mortgage) debt, the liquidity of housing and financial assets, and the risk of sharp reductions in their prices, will have increasingly important implications for financial vulnerability in countries with higher indebtedness.[29]
A second and related issue is that structural features of debt and asset markets may have important implications for financial vulnerability via their effect on credit constraints, liquidity, the likelihood of default and loss given default. Ellis (2006) provides a cross-country comparison of tax, financial and legal systems with regards to their impact on housing and debt markets. One key difference is whether households with debt secured against property pay off debt and accumulate housing equity relatively rapidly, or instead maintain debt for longer and accumulate financial assets. The former tends to occur where flexible interest rate mortgages are the norm, and interest costs cannot be offset against income tax. In these countries, households with debt are susceptible to interest rate shocks. However, working in the other direction, they tend to accumulate prepayment buffers, which provide the option of ‘payment holidays’ or housing equity withdrawal at times of stress. And unlike financial assets, consumption can be funded in this way without liquidating assets, which would otherwise put downward pressure on asset prices when they may already be under pressure from an economic downturn.
A third important issue for financial vulnerability is the distribution of debt and assets across different households. In a number of countries that have experienced rapid rises in debt over the past decade or more, much of this is held by higher-income households who spend a small share of their disposable income servicing that debt (Debelle 2004; Girouard, Kennedy and André 2007; RBA 2007).
5.3 Factors to consider beyond the confines of this model
In the model presented above, households that take on more debt are assumed to account for the additional risk that this implies in an optimal way. Indeed, aside from a purely exogenous reduction in credit constraints, many other developments would have reduced risk at unchanged levels of debt. Not surprisingly then, households have taken on more debt and financial institutions have eased credit constraints. For reductions in credit constraints that are unrelated to changes in structural factors affecting risk, the degree of vulnerability rises but from a level that may have been sub-optimal.
Given this endogenous response of debt so as to maintain an optimal degree of risk, what might cause a policy-maker to be wary of the effect of rising indebtedness on financial stability? Perhaps the foremost concern is that in a world of imperfect information, households and financial institutions may misjudge the true risks they face. They may give too much weight to recent experience, leading them to underestimate risks during benign periods and overstate risks following adverse shocks. At the same time, individual household and financial institutions can adversely affect others in ways that they do not account for when making their decisions. These two features can lead financial system developments to amplify business cycles.
Amplification arises in large part because of the existence of financial market frictions, the extent of which can vary in response to different shocks. The extent of credit constraints, which can be ameliorated by the use of collateral, is one such example.[30] In this case, the extent of constraints can be affected by changes in the prices of collateral, which are determined in forward-looking asset markets and depend in turn on the availability of credit. Amplification can affect our measure of vulnerability in two ways:
- accelerator affects will increase L(i|Ωt), since an adverse shock can lead to a larger fall in asset prices, tightening credit constraints as well as increasing the likelihood of default (q) and the loss given default (α). A tightening of lending standards in response to adverse shocks can compound these effects; and
- while the likelihood of adverse shocks may have declined over a long period of time, households and financial institutions may perceive a larger reduction in risk than has actually occurred. Hence, the true probability distribution of states of the world, f(i|Ωt), may be less benign than that embodied in the perceptions of private agents. Following adverse shocks, these perceptions can overshoot in the other direction, leading to excessive caution by private agents, which can exacerbate the initial adverse shock.
For these reasons, the index of vulnerability could increase by more during expansionary phases than would be the case in a world of perfect information. In practise it will be difficult to measure an increase in vulnerability in real time given the range of structural changes that are likely to have been responsible for triggering the rise in indebtedness in the first place. Even so, empirical evidence suggests that expansions accompanied by the following developments may be more risky than others:
- financial deregulation – where financial institutions, households and the regulator(s) are learning rapidly about a new regime with more readily available credit (CGFS 2006);
- rapid growth of asset prices and credit (Borio and Lowe 2002, 2004); and
- especially vigorous competition between financial institutions attempting to maintain market shares.
In short, it may take time (and experience) to accurately assess the true nature and extent of sustainable structural change. Therefore, very rapid rises in debt may indicate excessive risk-taking by private agents throughout the economy, leaving them more vulnerable to adverse shocks than they expect.
6. Conclusions
Most, though not all, advanced economies have experienced a substantial rise in indebtedness over the past two decades or so. This has been accompanied by a decline in real mortgage rates, suggesting that much of the rise in debt can be attributed to supply-side factors. This paper makes use of the considerable variation in the extent of the trend rise in debt-to-income ratios across countries over a long period to examine the role of a number of potential explanatory factors identified in the literature. In particular, we show that countries that experienced larger trend increases in indebtedness can be roughly characterised as having had larger declines in inflation, macroeconomic volatility and unemployment. They also tend to have less regulated, and more competitive and innovative mortgage markets.
We examine simulations based on the assumption that households are credit-constrained to explore the relative importance of various factors for the rise in indebtedness. This analysis suggests that the decline in inflation may have been relatively more important than the decline in real interest rates, at least for countries in which maximum repayment ratios are an important feature of credit constraints. And while aggregate indebtedness is likely to have adjusted gradually over the course of a decade or so, the bulk of these effects are likely to have run their course by now. Falling unemployment and a willingness of households to refinance and hold debt for longer has also played some role. This latter effect may in part reflect the effect of rising longevity, which is likely to have an ongoing effect. In addition, there is evidence of a further relaxation of lending standards in a number of countries over recent years, which if sustained would imply some further expansion in indebtedness.
Using a model in which household decisions regarding debt are made optimally with regard to various factors affecting risk, we explore the implications of rising debt for the vulnerability of the household sector. If the risks affecting the ability of households to service their debts have shifted over the longer term so as to become more benign, then it makes sense for debt to rise. For this reason, higher debt does not necessarily imply an increase in vulnerability. Yet even if vulnerability does rise, this may well be welfare-improving (particularly if credit had previously been unduly restricted). In either case, higher debt means that, ex post, an adverse shock of a given size will imply greater costs for households and, potentially, financial institutions. In this respect, higher indebtedness can have important implications for the transmission of monetary policy. From the perspective of policy-makers charged with maintaining financial system stability, a key concern is that financial institutions and households may tend to underestimate the true degree of risk. If they are ill-informed and base their decisions on recent experience then vulnerability may be rising after a period of relatively favourable economic conditions, particularly if competition has intensified and debt has been rising especially rapidly.
Appendix A: Data Definitions and Sources
Debt-to-income ratios
Total household debt as a percentage of household disposable income. For most countries the data for debt and income are sourced separately and the measure available uses SNA93 definitions, so that debt includes all liabilities of households and unincorporated enterprises and disposable income is measured after interest payments and includes the income of unincorporated enterprises. Exceptions are detailed in Table A1.
Country | Sources | Definition of debt and disposable income | Time period |
---|---|---|---|
Australia | RBA Bulletin | Debt: excluding UE HDI: before interest payments; excluding UE | 1976–2006 |
Canada | Bank of Canada; Statistics Canada through Datastream | SNA93 | 1975–2006 |
Denmark | Statistics Denmark; Statistics Denmark through Datastream | SNA93 | 1995–2005 |
Finland | Statistics Finland | SNA93 | 1990–2004 |
Germany | Deutsche Bundesbank | SNA93 | 1984–2005 |
Netherlands | CPB Netherlands Bureau for Economic Policy Analysis; Statistics Netherlands; unpublished data from De Nederlandsche Bank | SNA93 | 1970–2006 |
NZ | RBNZ | HDI: before interest payments | 1990–2005 |
Norway | Norges Bank Financial Stability | Loan debt as a percentage of liquid disposable income less estimate of reinvested dividend payments | 1987–2006 |
UK | Office for National Statistics | SNA93 | 1975–2005 |
US | Board of Governors of the Federal Reserve System Flow of Funds Accounts of the United States | HDI: excluding UE; before deduction of mortgage interest payments | 1975–2006 |
France | OECD Economic Outlook, Vol Nos 78–81, Statistical Annex Table 58 | SNA93; published as a ratio of debt to income | 1993–2005 |
Italy | 1980–2005 | ||
Japan | 1984–2004 | ||
Belgium | CGFS (2006) | Exact treatment of components not stated; provided as a ratio of debt to income | 1993–2003 |
South Korea | 1984–2004 | ||
Spain | 1984–2004 | ||
Switzerland | 1990–2003 | ||
Sweden | 1984–2005 | ||
Notes: UE is unincorporated enterprises; HDI is household disposable income. |
Real mortgage interest rates and their volatility
Data are monthly and sourced from the Bank of International Settlements (BIS) through DBSonline for: Belgium, France, Germany, Italy, Japan, Sweden, Switzerland, the UK and the US. A discontinued series (also sourced through DBSonline) with the most similar definition and coverage was additively spliced to the current series for each of: Belgium (November 2003), Germany (December 2002), Italy (December 1994), Japan (March 1986) and the UK (December 1983). The sources of data for other countries are (splice dates are shown in brackets): Australia – RBA; Denmark – Danmarks Nationalbanken and (prior to 2003) Bloomberg; Finland – Finlands Bank and (prior to 2003) IMF International Financial Statistics (IFS) (through Datastream); South Korea – IFS (through Datastream); Netherlands – CGFS (2006) and (prior to December 1984) IFS (through Datastream); NZ – RBNZ and (prior to June 1998) IFS (through Datastream); and Norway – IFS (through Datastream). For Denmark and NZ, the splice series is the 3-year swap rate, not the historical mortgage rate. The real mortgage rate is the nominal rate deflated using the rate of realised consumer price inflation.
Volatility is calculated as the rolling five-year end-of-period standard deviation of the nominal mortgage rate.
Consumer price inflation
Based on the consumer price index from national statistical agencies through Datastream. Exceptions are: Australia – CPI less interest charges prior to the September quarter 1998 and adjusted for the tax change of 1999–2000 (RBA); South Korea – OECD.Stat; Japan – Management and Coordination Agency (through Datastream); US – Bureau of Labor Statistics (through Datastream).
Unemployment rates
Data are sourced from national statistical agencies through Datastream. Exceptions are: Australia – ABS; Finland, Italy, South Korea, Netherlands, Norway and Spain – OECD (through Datastream); Japan – Ministry of Health, Labour and Welfare; US – Bureau of Labor Statistics (through Datastream).
Real house prices
Data on nominal house prices are sourced from the BIS through DBSonline. Exceptions are: Australia – ABS; Finland, France, Norway, Switzerland – national statistical agencies through Datastream; Germany – BulwienGesa AG through Datastream; Japan – Japan Real Estate Institute through CEIC; South Korea – Kookmin Bank through CEIC; NZ – Real Estate Institute of New Zealand through Datastream and backcast (prior to March quarter 1992) using data from the BIS; UK – Nationwide through Datastream; US – Office of Federal Housing Enterprise Oversight through Datastream. The nominal house price is deflated by the CPI for each country.
Volatility of real output growth
Rolling five-year end-of-period standard deviation of annual real GDP growth. Calculation uses real GDP data sourced from national statistical agencies through Datastream. Exceptions are: Belgium, Netherlands – OECD sourced through Datastream; Denmark, Finland, Norway, Switzerland – BIS through DBSonline; Australia – ABS Cat No 5206.0, South Korea – CEIC. If historical data were unavailable from this source, data from the World Bank World Development Indicators were used to backcast the series. The splice dates are: 1989 – Denmark; 1974 – Finland; 1977 – France, Norway; 1979 – Spain, Sweden; 1980 – Switzerland; 1986 – NZ; 2000 – Italy. Exception: Japan – spliced backward between 1980 and 1970 using SNA68 data and prior to 1970 using OECD data.
Gearing
Measured as the ratio of total household debt to total household assets (financial and non-financial assets). Data for debt are sourced as for the debt-to-income ratio. Data on assets are sourced from: Australia – RBA Bulletin; Netherlands – CPB Netherlands Bureau for Economic Policy Analysis and Statistics Netherlands; UK – Office for National Statistics; US – Board of Governors of the Federal Reserve System ‘Flow of Funds Accounts of the United States’. Debt and asset data for France are published in OECD Economic Outlook Statistical Annex, Table 58 as a percentage of disposable income – the gearing ratio is calculated as the debt-to-income ratio divided by the assets-to-income ratio.
Interest-payments ratio
The ratio of interest payments on total household debt (including unincorporated enterprise debt) to disposable income before the deduction of interest payments. Exceptions: Australia, US – interest payments on housing and consumer debt only; disposable income excludes the income of unincorporated enterprises. Sources: Australia – RBA Bulletin; France – National Institute for Statistics and Economic Studies (INSEE); Netherlands – Statistics Netherlands; UK – Office for National Statistics; US – Bureau of Economic Analysis.
Product market regulation
Countries are classified on a 0–6 scale from least to most restrictive for each regulatory and market feature of the seven non-manufacturing industries: airlines, railways, road, gas, electricity, post and telecommunications. Data are from Conway and Nicoletti (2006).
German data
German data refer to West Germany prior to 1991.
Appendix B
Country | Year | Reform |
---|---|---|
Australia | 1980 | Bank specialisation requirements abolished for large domestic banks |
1982 | Quantitative lending guidance eliminated | |
1986 | Removal of ceiling on mortgage interest rate | |
1988 | Securitisation first adopted | |
Canada | 1967 | Ceiling on interest rate on bank loans eliminated |
1967 | Restrictions on banks' participation in mortgage financing abolished | |
1980 | Banks allowed to have mortgage loan subsidiaries | |
1987 | Securitisation introduced | |
Denmark | 1982 | Liberalisation of mortgage contract terms |
1982 | Interest rate deregulation | |
1989 | Elimination of restriction on mortgage bond issuance | |
1991 | Enhanced freedom of entry | |
Finland | 1984 | Funding quotas from the Central Bank to commercial banks eliminated |
1986 | Interest rate deregulation | |
1987 | Guidelines on mortgage lending removed | |
1989 | Securitisation introduced | |
France | 1984 | Bank specialisation requirements reduced |
1984 | Ending of priority lending/sectoral guidelines | |
1987–89 | Elimination of credit and exchange controls | |
1999 | Removal of monopoly right to issue mortgage bonds | |
Germany | 1967 | Interest rate deregulation |
1992 | Enhanced freedom of entry | |
Italy | 1983 | Interest rate deregulation |
1983 | Credit ceilings eliminated | |
1993 | Enhanced freedom of entry | |
1994 | Separation of long-term and short-term credit institutions abolished | |
Japan | 1993 | Bank specialisation requirements reduced |
1994 | Interest rate deregulation completed (begun in early 1980s) | |
South Korea | 1982 | Direct government control of banks removed |
1984 | Entry and operations restrictions eased | |
1991 | Interest rate controls completely removed | |
Netherlands | 1980 | Interest rate deregulation |
1992 | Enhanced freedom of entry | |
NZ | 1984 | Credit allocation guidelines removed |
1984 | Interest rate deregulation | |
Norway | 1984 | Lending controls abolished |
1985 | Interest rate deregulation | |
Spain | 1986 | Entry of foreign banks |
1987 | Interest rates deregulated | |
1990 | Credit ceilings eliminated | |
Sweden | 1985 | Interest rate deregulation |
1985 | Lending controls for banks abolished | |
UK | 1979–80 | Abolition of exchange and credit controls |
1981 | Banks allowed to compete with building societies for housing finance | |
1981 | Minimum lending rates abolished | |
1986 | Guidelines on mortgage lending removed | |
1987 | Securitisation introduced | |
US | 1971 | Securitisation introduced |
1980 | Beginning of four-year interest rate deregulation | |
1980 | Elimination of portfolio restrictions for thrifts | |
Sources: Girouard and Blondal (2001); G10 (2003); Hao, Hunter and Yang (1999) |
Appendix C: Calculations from Section 4
The credit constraint requires that the constant repayment is a set percentage of income where the repayment is given by:
where: i is the annual nominal interest rate; V is the value of the loan; and N is the duration of the loan. Assuming that the loan is a credit-foncier and individuals only take out a loan when they are 30, then the aggregate debt to income ratio can be derived from:
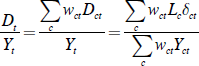
where: D is nominal debt; L is the loan taken out at 30; Y is income; c is a cohort identifier; w is the population size of the cohort; t identifies a particular year; and δ indicates the fraction of the original loan still outstanding, which can be calculated as:
where: N is the length of the loan; and s is how long the loan has already existed at time t (that is, cohort c's age at time t minus 30).
Footnotes
The authors would like to thank Andrea Brischetto, Laura Berger-Thomson, Chris Carroll, Guy Debelle, Malcolm Edey, Jeremy Lawson, Philip Lowe, Kristoffer Nimark, Tony Richards and Chris Stewart for helpful comments and suggestions. The views expressed in this paper are those of the authors and do not necessarily reflect those of the Reserve Bank of Australia. [1]
Details of the calculations and the sources for data can be found in Appendix A. [2]
Girouard, Kennedy and André (2007) provide a recent example of this line of argument. [3]
Macfarlane (2003) makes this general point. He also suggests that in the context of the period of rapidly rising house prices in Australia, greater indebtedness had made households more sensitive to economic conditions. [4]
In fact all countries afford some tax benefits to owner occupiers, who do not pay tax on imputed rents for their homes. [5]
In Australia, this was typically around 30 per cent of gross income (RBA 2003a), although it has been relaxed more recently (Laker 2007). Debelle (2004) suggests that since the fall in inflation will erode the real value of debt less rapidly, debt-to-income ratios might be higher than otherwise. However, this implicitly assumes that repayments are a constant share of income. [6]
For example, see Comin and Mulani (2004) and Dynan, Elmendorf and Sichel (2006). Peek and Wilcox (2006) note that financial deepening may help to explain the moderation in output volatility due to its potential role in enhancing consumption smoothing. [7]
The real mortgage rate is the nominal rate as at the end of the year less actual year-ended inflation. This may have some shortcomings as a measure of the cost of debt for some countries, particularly earlier in the sample period when actual inflation may be a poor indicator of expected inflation and regulations on mortgage lending imply that this measure may be too narrow; even so, mortgage debt has always been a significant component of total household debt (except for South Korea). [8]
At least for small open economies, the drop in real mortgage rates is consistent with an increase in the global supply of funds as well as a significant outward shift of demand. [9]
As an alternative, we also examined the extent of MEW (that is, withdrawal versus injection of equity). This has a high correlation (of around 0.9) with the availability score shown in Table 3 for 15 of the 18 countries for which MEW data are readily available. [10]
The mortgage market completeness index uses data on a range of market features, including loan-to-valuation (LTV) ratios, product availability, repayment structures and loan types; however, it is only available for eight European countries. The IMF (2006) constructs a broader measure of financial market structure using a similar methodology. We examined the level of the margin on mortgage lending interest rates as an alternative indicator of competitive pressures and, therefore, of the extent of market flexibility. However, because the measure of mortgage lending rates is published in different forms across countries (prime rate, all mortgages versus only new mortgages, a weighted-average actual rate rather than an indicator rate), a comparable measure in levels is not readily available. Nevertheless, changes in this margin over time roughly accord with the market flexibility scores described in the main text (with a correlation of about −0.2). [11]
In the figures, the dates in the country labels indicate the peak in the explanatory variable. The change in that variable is measured between the date of the peak and the end of 2004, the latest date for which we have debt data for all countries in the sample. Where possible, the same time period has been used to calculate the change in the debt-to-income ratio. However, when these data are not available, a shorter time period for the change in indebtedness has been used, provided the peak was not more than 10 years before the start of the debt series. (This is consistent with the fact that the change in the explanatory variables can operate with a long lag – see Section 4.) If the peak is too far in advance of the available debt data, then we either use a local peak closer but prior to the start of the debt data (which is true for Switzerland in the case of inflation and output volatility) or omit that country from the graph (in the case of Belgium and Denmark for some graphs). In the case of inflation, we identify the local peak from 1977 onwards, as earlier peaks reflect the extreme volatility of inflation during the 1970s and occurred well before the rise in debt in most countries. Finally, the trends identified in the figures were relatively robust to alternative dating options, such as matching the period for the change in the explanatory variable to the available data for indebtedness (where this was sufficiently long). [12]
Until the early 1990s, Italy still had a highly regulated debt market. From 1997 to 2003, household debt in Italy has grown at a faster pace than all other euro area countries except Spain, consistent with deregulation in the 1990s (Casolaro et al 2006). [13]
Tudela and Young (2005), who examine UK indebtedness using an OLG model, make an adjustment to the model of Barnes and Young, which they believe could reflect omitted factors like liquidity constraints. [14]
Ellis (2005) discusses the effects of downpayment constraints, including how rising demand induced by changes in inflation or real rates could lead downpayment constraints to become binding thereby moderating, at least temporarily, the tendency for indebtedness to rise. [15]
The numbers in row II may be unrealistically high, particularly in the high unemployment case. This is because we have assumed that those with debt who become unemployed suffer from lower incomes but continue to service their debts. This effect by itself tends to imply that high unemployment leads to high debt-to-income ratios. Also, the ability to service debt is likely to be impaired by extended periods of unemployment, which are more likely during periods of high unemployment. [16]
We also find there to be relatively small effects of unemployment in variants of the model where: (a) those who are unemployed for much of their 20s do not get loans or get smaller loans; (b) individuals can potentially get a new loan even after defaulting; and(c) the probability of unemployment in any 5- or 10-year spell is equal to the unemployment rate. Simulations like these are likely to be only guides as to the effect of unemployment on indebtedness because they impose strong assumptions on the probability of transition in and out of unemployment and the relationship between unemployment spells, default and ability to get a loan. [17]
For this model we assume that the growth rate of real income of the cohort entering the labour force is 2 per cent. [18]
In reality, observed increases in loan duration could also be due to lower risk aversion of more recent generations of borrowers. [19]
This is broadly consistent with revenue data from some Australian State governments. It is also roughly comparable to the increase in borrowing that would occur if households refinanced after five years according to the 30 per cent repayment ratio rule of thumb, but applied to current nominal income, which increases considerably over five years. [20]
Maki (2000) summarises the literature exploring the link between consumption and credit and concludes that there is little empirical evidence that household debt service burdens or other credit quality variables are negatively related to future consumption in the short run. However, this is consistent with households raising debt in response to a reduction in the risk of adverse shocks. Also, it says nothing about the implication of large rises in debt for the behaviour of consumption over the longer term. [21]
Most financial/banking crises have been related to problems in the commercial property market, corporate or international lending, rather than to the household sector. Since household default rates tend to be low, the magnitude of any reductions in consumption provides a measure of the size of adverse shocks and the desire to avoid default (Barrell, Davis and Pomerantz 2006; CGFS 2006). [22]
Schinasi (2004) discusses some key issues relevant to financial stability/vulnerability and provides a useful summary of recent definitions by a range of academics and central bankers. While many of these definitions are impressionistic, they broadly accord with our more explicit formulation. See also Haldane et al (2004) and Allen and Wood (2006) for a recent summary of this literature. [23]
This accords with the characterisation by Cecchetti (2006) of the preferences of central bankers charged with maintaining economic and financial system stability and the notion of ‘GDP at risk’. [24]
These assumptions simplify the solution at the expense of some realism – namely, that default is costly. [25]
Murphy (1999) presents evidence on the precautionary saving motive that suggests that agents borrow more under lower uncertainty. [26]
This highlights the fact that minimising financial vulnerability is not equivalent to maximising welfare, since policy-makers also need to consider the benefits associated with debt. One way to do this is for policymakers to maximise some index of financial efficiency subject to maintaining vulnerability below some level. In practise, this sort of approach would make sense if it was easier to derive suitable measures of efficiency and vulnerability than to characterise expected utility. Kent and Debelle (1999) discuss the trade-off between efficiency and vulnerability in a model of banking consolidation. Schinasi (2004) touches on this point, but notes that much work remains to be done in this area; the nature of the benefits associated with financial deregulation and other financial system developments affecting the household sector are highlighted by CGFS (2006). [27]
Our measure of consumption smoothing declines in response to the lower lending premium
for low values of , reflecting the influence of the falling cost
of borrowing on the NPV calculation. If instead we base the NPV solely on
the risk-free interest rate, the measure of self-insurance declines (implying
increased consumption smoothing) in response to the reduced risk of default.
[28]
It is worth noting that new financial products that make it easier for mortgagors to gain access to housing equity may have increased the liquidity of housing assets. This itself may have encouraged households to take on more debt. [29]
See Haldane et al (2004) and references therein for a discussion of models of financial frictions and their role in financial stability. [30]
References
Allen WA and G Wood (2006), ‘Defining and Achieving Financial Stability’, Journal of Financial Stability, 2(2), pp 152–172.
Barnes S and G Young (2003), ‘The Rise in US Household Debt: Assessing its Causes and Sustainability’, Bank of England Working Paper No 206.
Barrell R, EP Davis and O Pomerantz (2006), ‘Costs of Financial Instability, Household-sector Balance Sheets and Consumption’, Journal of Financial Stability, 2(2), pp 194–216.
Bernanke BA (2007), ‘The Subprime Mortgage Market’, remarks at the Federal Reserve Bank of Chicago's 43rd Annual Conference on ‘Bank Structure and Competition’, Chicago, 17 May.
Borio C and P Lowe (2002), ‘Asset Prices, Financial and Monetary Stability: Exploring the Nexus’, BIS Working Paper No 114.
Borio C and P Lowe (2004), ‘Securing Sustainable Price Stability: Should Credit Come Back from the Wilderness?’, BIS Working Paper No 157.
Campbell JR and Z Hercowitz (2006), ‘The Macroeconomic Transition to High Household Debt’, paper presented at the ‘Financial Innovations and the Real Economy’ conference sponsored by the Center for the Study of Innovation and Productivity, Federal Reserve Bank of San Francisco, San Francisco, 16–17 November.
Carroll CD and WE Dunn (1997), ‘Unemployment Expectations, Jumping (S,s) Triggers, and Household Balance Sheets’, NBER Working Paper No 6081.
Casolaro L, L Gambacorta and L Guiso (2006), ‘Regulation, Formal and Informal Enforcement and the Development of the Household Loan Market: Lessons from Italy’, in G Bertola, R Disney and C Grant (eds), The Economics of Consumer Credit, MIT Press, Boston, pp 93–134.
Catte P, N Girouard, R Price and C André (2004), ‘Housing Markets, Wealth and the Business Cycle’, OECD Economics Department Working Paper No 394.
Cecchetti SG (2006), ‘Measuring the Macroeconomic Risks Posed by Asset Price Booms’, NBER Working Paper No 12542.
Comin D and S Mulani (2004), ‘Diverging Trends in Macro and Micro Volatility: Facts’, New York University, mimeo.
CGFS (Committee on the Global Financial System) (2006), ‘Housing Finance in the Global Financial Market’, CGFS Publications No 26.
Conway P and G Nicoletti (2006), ‘Product Market Regulation in the Non-Manufacturing Sectors of OECD Countries: Measurement and Highlights’, OECD Economics Department Working Paper No 530.
Debelle G (2004), ‘Macroeconomic Implications of Rising Household Debt’, BIS Working Paper No 153.
Dynan KE, DW Elmendorf and DE Sichel (2006), ‘Financial Innovation and the Great Moderation: What Do Household Data Say?’, paper presented at the ‘Financial Innovations and the Real Economy’ conference sponsored by the Center for the Study of Innovation and Productivity, Federal Reserve Bank of San Francisco, San Francisco, 16–17 November.
Ellis L (2005), ‘Disinflation and the Dynamics of Mortgage Debt’, in Investigating the Relationship between the Financial and Real Economy, BIS Papers No 22, BIS, Basel, pp 5–20.
Ellis L (2006), ‘Housing and Housing Finance: The View from Australia and Beyond’, RBA Research Discussion Paper No 2006-12.
Fernandez-Corugedo E and J Muellbauer (2006), ‘Consumer Credit Conditions in the United Kingdom’, Bank of England Working Paper No 314.
Girouard N and S Blondal (2001), ‘House Prices and Economic Activity’, OECD Economics Department Working Paper No 279.
Girouard N, M Kennedy and C André (2007), ‘Has the Rise in Debt Made Households More Vulnerable?’, OECD Economics Department Working Paper No 535.
G10 (Group of Ten) (2003), ‘Turbulence in Asset Markets: The Role of Micro Policies’, Report of the Contact Group on Asset Prices, January.
Haldane A, V Saporta, S Hall and M Tanaka (2004), ‘Financial Stability and Macroeconomic Models’, Bank of England Financial Stability Review, Issue 16, pp 80–88.
Hao J, WC Hunter and WK Yang (1999), ‘Deregulation and Efficiency: The Case of Private Korean Banks’, Federal Reserve Bank of Chicago Research Department Working Paper No WP/99/27.
Hoeller P and D Rae (2007), ‘Housing Markets and Adjustments in Monetary Union’, OECD Economics Department Working Paper No 550.
Hurst E (2006), ‘Discussion of Campbell and Hercowitz's “The Macroeconomic Transition to High Household Debt”’, presented at the ‘Financial Innovations and the Real Economy’ conference sponsored by the Center for the Study of Innovation and Productivity, Federal Reserve Bank of San Francisco, San Francisco, 16–17 November.
IMF (International Monetary Fund) (2006), ‘How Do Financial Systems Affect Economic Cycles?’, World Economic Outlook – Financial Systems and Economic Cycles, World Economic and Financial Surveys, IMF, Washington DC, September, pp 105–138.
Kent C and G Debelle (1999), ‘Trends in the Australian Banking System: Implications for Financial System Stability and Monetary Policy’, RBA Research Discussion Paper No 1999-05.
Kulish M, K Smith and C Kent (2006), ‘Ageing, Retirement and Savings: A General Equilibrium Analysis’, RBA Research Discussion Paper No 2006-06.
Laker JF (2007), ‘Credit Standards in Housing Lending – Some Further Insights’, speech to the Institute of Chartered Accountants in Australia, Melbourne, 20 June.
Macfarlane I (2003), ‘Do Australian Households Borrow Too Much?’, RBA Bulletin, April, pp 7–16.
Maki DM (2000), ‘The Growth of Consumer Credit and the Household Debt Service Burden’, Board of Governors of the Federal Reserve System Finance and Economics Discussion Series No 2000-12.
Mercer Oliver Wyman (2003), Study on the Financial Integration of European Mortgage Markets, European Mortgage Federation, Brussels.
Murphy RG (1999), Household Debt and Aggregate Consumption Expenditures, Boston College, mimeo, March.
Peek J and JA Wilcox (2006), ‘Housing, Credit Constraints, and Macro Stability: The Secondary Mortgage Market and Reduced Cyclicality of Residential Investment’, Fisher Center for Real Estate & Urban Economics, University of California Berkeley, Working Paper No 298.
Productivity Commission (2005), Economic Implications of an Ageing Australia, Research Report, Canberra.
RBA (Reserve Bank of Australia) (2003a), ‘Household Debt: What the Data Show’, RBA Bulletin, March, pp 1–11.
RBA (2003b), ‘Submission to the Productivity Commission Inquiry on First Home Ownership’, RBA Occasional Paper No 16.
RBA (2006), Financial Stability Review, September.
RBA (2007), Financial Stability Review, March.
Schinasi GJ (2004), ‘Defining Financial Stability’, IMF Working Paper No WP/04/187.
Steigum E (2004), ‘Financial Deregulation with a Fixed Exchange Rate: Lessons from Norway's Boom-Bust Cycle and Banking Crisis’, in TG Moe, JA Solheim and B Vale (eds), The Norwegian Banking Crisis, Norges Bank Occasional Paper No 33, Norges Bank, Oslo, pp 23–75.
Tudela M and G Young (2005), ‘The Determinants of Household Debt and Balance Sheets in the United Kindgom’, Bank of England Working Paper No 266.