RDP 2022-07: The Term Funding Facility: Has It Encouraged Business Lending? 5. Identification Strategies, Challenges and Assumptions
December 2022
- Download the Paper 2.1MB
5.1 Identification strategies
This paper attempts to estimate the causal effect of the TFF on the amount of banks' lending to businesses. We do so by focusing on three key policy questions. First, we examine whether the incentive in the TFF's additional allowance was effective in stimulating growth in business credit. Second, we investigate whether the availability of the TFF affected a bank's business lending. Finally, we assess whether a lender's take-up of the TFF affected their business lending.
Our analysis is performed in a differences-in-differences set-up, which seeks to estimate the causal effect of an intervention by comparing changes in outcomes between a ‘treatment’ and a ‘control’ group. In our first analysis, where we examine whether the additional allowance feature was effective in stimulating growth in business credit, we exploit the fact that banks received five times the additional allowance for lending to SMEs relative to large businesses, with large businesses acting as the control group in this exercise. Since these regressions focus on the difference between lending to SMEs and large businesses, they are designed to capture only the effect of the incentive provided by the additional allowance. The subsequent analysis captures the broader range of channels, including the additional allowance as well as the effect of the TFF on banks' funding costs and their certainty of funding.[9] We assess the effect of the availability of the TFF on aggregate business lending by examining the difference in business lending growth for banks relative to non-banks. Non-banks are the control group in this exercise as they were not eligible for the TFF. Finally, we aim to estimate the effect of banks accessing the TFF, as distinct from the effect of its availability. To address this question, we use instrumental variables to avoid endogeneity issues.
In our analysis, we face a number of identification challenges, which we address in a variety of ways, including additional control variables, instrumental variables and a triple-difference framework. As discussed above, one identification challenge common with other international literature is disentangling the effects of credit supply and credit demand. Liaison with banks and other data sources indicated that demand for business credit was generally weak from the beginning of the pandemic through to early 2021, reflecting the reluctance of firms to take on credit in an environment of economic uncertainty. In addition, the provision of government support such as JobKeeper provided businesses with substantial liquidity meaning that they had less need to access credit.[10] For our baseline model comparing growth in credit outstanding to SMEs and large businesses, an identification issue would arise if credit outstanding to SMEs would have evolved differently to that of large businesses in the absence of the TFF. For example, SMEs were particularly targeted by various government policy support measures, which may have reduced the need for finance. Larger businesses may have been more willing to borrow, including by drawing on lines of credit for precautionary reasons. While international papers have commonly dealt with this issue by adding fixed effects for the borrowing firms, we are not able to conduct similar analysis as we do not have access to data on individual borrowers.
We use a regression in the spirit of a triple-difference framework to address the potential difference in the effect of COVID-19 on lending to SMEs and larger businesses. This regression assesses the difference between lending to SMEs and large businesses by banks relative to non-banks. Under this model, we assume that if COVID-19 restrictions did have different effects on SME and large business lending, including their demand for debt, then these differences would be common between the business customers of banks and non-banks.
In addition, COVID-19 restrictions had the largest effects on industries such as accommodation, hospitality and tourism, which tend to have a larger share of small firms. Small businesses have generally always found it more difficult to access finance than large businesses and (all else equal), these difficulties might have been expected to worsen during the pandemic (Kent 2021a). We address this issue by using data at the bank and industry level. This allows us to control for the uneven effects of COVID-19 across industries by examining whether the TFF had a differential effect on SME lending compared to large business lending within industries. The triple-difference regression may also address this issue, so long as this difference in industries between small and large firms was common across banks and non-banks.
Closely related to these challenges, data and bank liaison suggest that, in the early months of the COVID-19 pandemic, large businesses drew down on existing lines of credit for precautionary reasons. This resulted in a sharp increase in business credit, which subsequently reversed as these businesses generally repaid these facilities within a couple of months. As these moves in business credit were driven by large businesses, and generally did not have an effect on banks' final additional allowance allocation, these developments may suggest higher large business credit growth by banks than what would otherwise be expected in an ‘ideal’ counterfactual scenario. We attempt to address this issue by using only fixed-term loans, which abstracts from businesses drawing on their existing revolving credit facilities.
In addition to complications related to lending to SMEs and large businesses, other identification challenges are present in the regressions that estimate the effect of the availability of the TFF to banks. In particular, non-banks may not be an ideal control group for banks when comparing business credit growth. A range of factors affect competition between banks and non-banks. While non-banks were not able to access the TFF, they did benefit from the Australian Office of Financial Management's (AOFM's) Structured Finance Support Fund, which improved the certainty of funding and reduced funding costs. In addition, non-banks benefited from less bond issuance by banks, which helped to narrow spreads on ABS. Working in the other direction, non-banks were unable to benefit from the strong pace of growth in deposits during the pandemic. Non-banks' business customers may have also been affected by the pandemic differently to firms that borrow from banks, including because non-banks may lend to a different population of borrowers than banks. It is likely that, in net terms, the effect of the Structured Finance Support Fund and low cost of securitisation issuance would support non-banks' business lending relative to banks' lending. This may suggest higher business credit growth by non-banks than what would otherwise be expected in an ‘ideal’ counterfactual scenario, causing the effects of the TFF on bank lending to be understated.
Another challenge that is common across the international literature is that participation in the TFF was voluntary. It is possible that banks who accessed TFF funding may have increased lending anyway (or the reverse). This identification issue is particularly relevant for our third analysis, which seeks to identify a differential effect between banks that did or did not access the TFF. Similar to international literature, we address this issue through an instrumental variables strategy.
Our first choice of instrument is the availability of self-securitised assets prior to the TFF. A valid instrument should be strongly correlated with a bank's decision to participate in the TFF but have no direct effect on business credit growth during the pandemic (except through its effect on influencing TFF participation). As noted above, banks with self-securitisations could access the TFF at lower cost, since they did not need to fund the acquisition of collateral (or establish processes to create self-securitisations). As such, banks with self-securitised assets were much more likely to access the TFF, suggesting the first condition holds (we test this formally below). The second condition is generally untestable, and this identification strategy will be problematic if the availability of self-securitisations is correlated with banks' business lending through channels other than access to the TFF. As an example, smaller banks and foreign banks are less likely to have self-securitised assets than larger Australian banks. If small or foreign banks' business lending did respond to the pandemic in a different way to that of large Australian banks, this would pose a challenge to this identification strategy.
In our second instrumental variable regression, we use the status of lenders as banks or non-banks as an instrument for whether an institution participated in the TFF. Since only banks were eligible for the scheme, we use this to estimate the effect of accessing the TFF on lending. The effects of accessing the scheme should be larger than the effect of it being available, since not all banks drew on the facility.
Finally, there are some very large growth rates in our sample as some lenders had very low levels of credit in the base period. This is an issue in the analysis comparing SMEs and large businesses, as some SME lending in particular can be quite volatile, suggesting that some smaller banks may drive the results. For our analysis comparing SMEs and large businesses, we address this by dropping three outliers (two banks and one non-bank). We also check our results by weighting the regressions based on the level of each lender's credit outstanding in the base period.
5.2 Parallel trends assumption
A difference-in-differences framework requires a ‘parallel trends assumption’ between the treatment and control groups, such that outcomes in the control group are an appropriate counterfactual for how outcomes in the treatment group would have evolved without the intervention. While we endeavour to create suitable control groups, identification is challenging because these assumptions may be invalidated by factors that are not observable. This is especially pertinent as the introduction of the TFF coincided with significant shocks resulting from the pandemic and other policy interventions.
Although imperfect, one way to examine whether outcomes in our control groups are an appropriate counterfactual is to visually inspect the trends in the lead-up to the TFF. SME and large business loan growth appears to be broadly correlated in the few months immediately prior to the TFF introduction, as measured by the simple average of bank-level growth rates (Figure 6). The pre-TFF trends are also similar for aggregate business credit extended by banks and non-banks (Figure 7). A statistical test of our pre-trends period for SME and large business lending growth, and banks relative to non-banks, supports this assumption.[11]
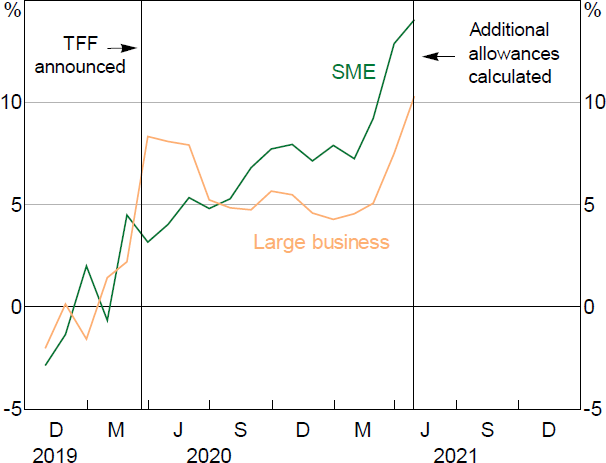
Note: Unweighted average of the cumulative growth in business credit relative to the average of the three months to January 2020 for banks that report data on business lending by business size; excludes two outliers.
Sources: APRA; RBA

Note: Unweighted average of the cumulative growth in business credit relative to the average of the three months to January 2020 for institutions reporting on ARF 720_1 A/B; excludes institutions that don't have data for all months.
Sources: APRA; RBA
The triple-difference regression considers the differing trends between lending to SMEs and to large businesses, comparing this difference between banks and non-banks. This regression assumes that this difference, SME lending less large business lending, would have evolved similarly for banks and non-banks in the absence of the TFF. However, prior to the pandemic, this differential was increasing for non-banks relative to banks, which could suggest a weakness in this analysis (Figure 8). In part, this could be due to the smaller sample size of non-banks which report outstanding credit by business size.
Our instrumental variable analysis implicitly assumes that total business credit growth would have evolved similarly for banks that had self-securitised assets and those that did not. These data are volatile, although a statistical test fails to reject the null hypothesis that these pre-trends were the same (Figure 9).
In general, the pre-trends period should be interpreted with caution because the EFS data collection only began in mid-2019 and there may have been data quality issues during the first few months of reporting.[12] In addition, we use non-seasonally adjusted data so month-to-month variations may reflect seasonality.
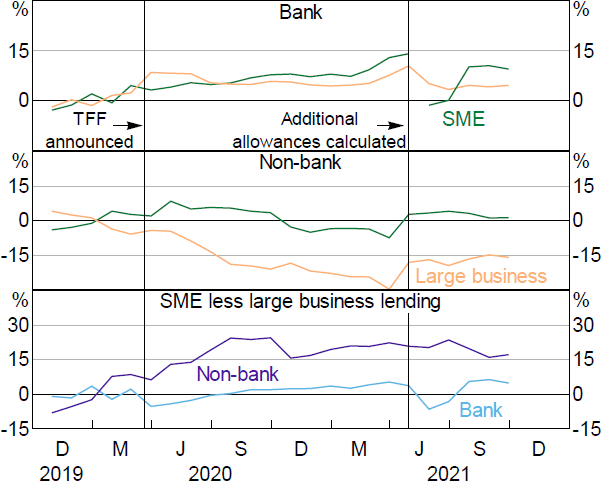
Note: Unweighted average of the cumulative growth in business credit relative to the average of the three months to January 2020 for institutions reporting business lending by business size; excludes three outliers.
Sources: APRA; RBA
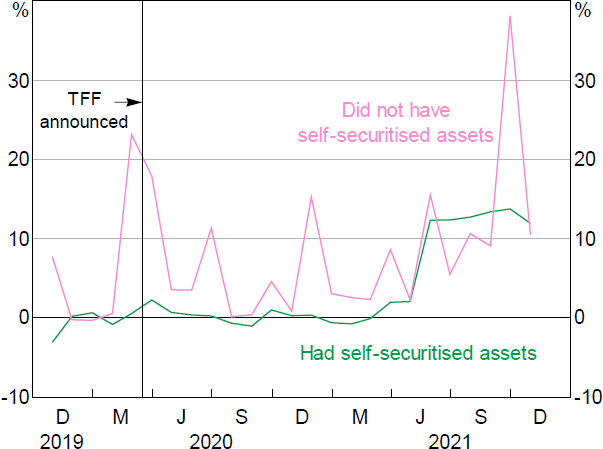
Note: Unweighted average of the cumulative growth in business credit relative to the average of the three months to January 2020 for banks reporting on ARF 720_1 A/B; excludes institutions that don't have data for all months.
Sources: APRA; RBA
Footnotes
While we capture the broader range of channels through which the TFF may affect business lending, our approach does not capture general equilibrium effects. [9]
In the very short-term, some businesses did access additional credit to manage cash flow challenges associated with the JobKeeper payment being paid monthly in arrears. [10]
We run a test of prior trends, which uses a simple difference-in-difference model. In this model, our dependent variable is log credit and our coefficient of interest is the interaction between the relevant group variable (SME borrowers, lender type and lenders that accessed the TFF) and a linear period variable. We run this model using only data from before the treatment period (before April 2020) for our three base models, and generate coefficients that are not significantly different from zero. This suggests that our pre-trends are not materially different. [11]
Prior to the EFS collection, there was limited data on business credit outstanding by size reported by banks permitted to use an internal ratings-based approach for credit risk management. These data suggested that large business lending had been growing but SME lending had been flat for several years. [12]