RDP 2021-02: Star Wars at Central Banks 1. Introduction
February 2021
- Download the Paper 1,553KB
How credible is central bank research? The answer matters for the policymakers who use the research and for the taxpayers who fund it.
We investigate an aspect of credibility by searching for evidence of researcher bias, which is a tendency to use undisclosed analytical procedures that raise measured levels of statistical significance (stars) in artificial ways. For example, a researcher might try several defensible methods for cleaning a dataset and favour those that yield the most statistically significant research results. In doing so, the researcher exaggerates the strength of evidence against their null hypothesis, potentially via inflated economic significance. The bias need not be malicious or even conscious.
Earlier research has investigated researcher bias in economics, but has focused mainly on journal publications. Christensen and Miguel (2018) survey the findings, which suggest the bias is common, and partly the result of journal publication incentives. More recent work by Blanco-Perez and Brodeur (2020) and Brodeur, Cook and Heyes (2020) corroborates these ideas. The literature uses several bias-detection methods. For our paper, we use the p-curve, from Simonsohn, Nelson and Simmons (2014), as well as the z-curve, from Brodeur et al (2016).[1] We choose these methods because they suit investigations into large bodies of research that cover a mix of different topics. But the methods also have shortcomings: the p-curve generates a high rate of false negatives and the z-curve requires strong assumptions.
Applying these methods to central bank research is a useful contribution to the literature because the existing findings about journals need not generalise. On the one hand, formal incentives for central bankers to publish in journals are often weak; much of their long-form research remains in the form of discussion papers. On the other hand, some central bank research does appear in journals, and there might be other relevant incentive problems to worry about, such as pressures to support house views (see Allen, Bean and De Gregorio (2016) and Haldane (2018)). Depending on how central bankers choose research topics, pressures to support house views could plausibly encourage findings of statistical significance.
To our knowledge, investigating researcher bias at central banks has been the topic of just one other empirical paper, by Fabo et al (2020). Focusing solely on research about the effects of quantitative easing, they find that central banks tend to estimate more significant positive effects than other researchers do. This is a worrisome finding because central bankers are accountable for the effects of quantitative easing, and positive effects of quantitative easing are likely to form part of the house view. Our work complements Fabo et al (2020) as our methods free us from having to benchmark against research from outside of central banks, benchmarks that the survey by Christensen and Miguel (2018) suggests would be biased. Our methods also allow us to study a wider body of central bank research at once. To conduct our search, we compile a dataset on 2 decades of discussion papers from the Federal Reserve Bank of Minneapolis (the Minneapolis Fed), the Reserve Bank of Australia (RBA) and the Reserve Bank of New Zealand (RBNZ). Where possible, we include parallel analysis of top economic journals, using the dataset from Brodeur et al (2016).
Another of our contributions to the literature is to conduct a new investigation into the merits of the z-curve assumptions. We test the assumptions using a placebo exercise, looking for researcher bias in hypothesis tests about control variables. We also investigate problems that we think could arise from applying the z-curve to research containing transparent use of data-driven model selection techniques.
Our headline findings are mixed:
- Using the p-curve method, we find that none of our central bank subsamples produce evidence of researcher bias.
- Using the z-curve method, we find that almost all of our central bank subsamples produce evidence of researcher bias.
- However, our placebo exercise and investigations into data-driven model selection cast doubt on the z-curve method.
A related finding – but one we are unable to explain – is that central banks produce results with patterns different from those in top journals, there being less bunching around the 5 per cent significance threshold of |z| = 1.96 (Figure 1). We offer some speculative explanations for the difference, but ultimately leave it as a puzzle for future work.
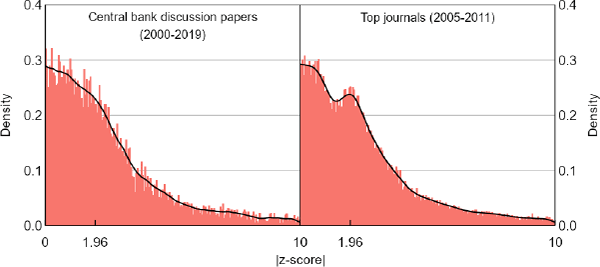
Notes: We plot the absolute values of de-rounded t-statistics (very close to z-score equivalents) for results that are discussed in the main text of a paper. We use the term ‘Top journals’ as loose shorthand for The American Economic Review, the Journal of Political Economy, and The Quarterly Journal of Economics.
Sources: Authors' calculations; Brodeur et al (2016); Federal Reserve Bank of Minneapolis; Reserve Bank of Australia; Reserve Bank of New Zealand
Footnote
Brunner and Schimmack (2020) and Bartoš and Schimmack (2021) offer another method, also called the z-curve. It aims to understand whether the findings in a body of work are replicable, whereas our objective is solely to understand researcher bias. [1]