Research Discussion Paper – RDP 2021-01 The Role of Collateral in Borrowing
January 2021
1. Introduction
Throughout history financial crises have been accompanied by credit crunches (Schularick and Taylor 2012). Credit market supply-side factors are important determinants (Bernanke and Lown 1991), but during crisis times, there is also a flight to quality – lenders become more reactive to borrower risk. As banks generally shift towards safe assets (Caballero, Farhi and Gourinchas 2017), lenders request better and more collateral (Bernanke, Gertler and Gilchrist 1996; Gorton and Ordoñez 2014; Benmelech, Kumar and Rajan 2020a, 2020b), and borrowers without collateral suffer (Rampini and Viswanathan 2010).
In this paper, we empirically analyse the effect of collateral on borrowing during crises. For identification, we focus on interbank loans during the 2008 crisis episode, as loans to banks are prone to the information problems that afflict credit more broadly (Morgan 2002; Dang et al 2017), and wholesale liquidity markets played a central role during this period.
We exploit transaction-level data on the Australian interbank markets for both collateralised and uncollateralised loans around a large exogenous shock – the Lehman Brothers failure. Interbank markets present an ideal laboratory for identification because collateralised and uncollateralised lending take place between the same borrower-lender pairs at the same time. Importantly, the collateral we analyse is liquid and homogeneous across borrowers, whereas in other markets such as real estate lending, collateral and borrower characteristics can be correlated in opaque ways. Australia presents an ideal setting because, in addition to data availability and one-way causality with the Lehman Brothers shock, collateralised lending (i.e. repo) is not guaranteed by a central counterparty – as in as in Europe, for example – and is not segmented from uncollateralised lending (i.e. unsecured) – as in the United States, for example.
Our main result is to robustly show that after the shock, ex ante riskier borrowers with sufficient high-quality collateral substitute from unsecured to repo borrowing. In the unsecured market, borrowing becomes negatively related to banks' measures of ex ante risk, and in the repo market, the largest pick-up is by the riskier banks with more high-quality collateral. We also present evidence of broad-based excess demand for high-quality collateral, as the repo market collateralised against second-best collateral (i.e. semis) expands, while interest rates against first-best collateral (i.e. Australian Government securities (AGS)) fall noticeably – over 100 basis points in our sample. This demand to hold liquid assets presents another means through which collateralisation can lift credit supply, separate from mitigating counterparty risk and information asymmetries, as banks lend more to acquire the best collateral, particularly to riskier borrowers substituting into the collateralised market. We find additional evidence consistent with this channel in the heterogeneity of behaviour across lenders and collateral types. Overall, our estimated effects are statistically and quantitatively significant, and do not appear in placebo regressions using 2006 data (i.e. normal times).
Our key contribution is to detail the role of collateral in borrowing using a robust identification strategy. The large credit channel literature has identified characteristics of credit crunches (e.g. Ivashina and Scharfstein 2010), but there are limitations in comparing collateralised and uncollateralised lending due to identification problems. There is some evidence that safer borrowers need less collateral when borrowing (e.g. Lian and Ma forthcoming) and that more conservative lenders prefer borrowers with less risk and better collateral (e.g. Jiménez et al 2014). However, identifying these effects is difficult because the collateral analysed tends to vary across borrowers, be highly illiquid, and be subject to asymmetric information problems (e.g. Benmelech and Bergman 2011). Moreover, in the literature on interbank and other wholesale markets, uncollateralised and collateralised lending are typically analysed in isolation from each other (e.g. for unsecured lending, see Afonso, Kovner and Schoar (2011); for repo lending, see Krishnamurthy, Nagel and Orlov (2014)). The two markets are analysed together by di Filippo, Ranaldo and Wrampelmeyer (2018); however, the collateralised market is intermediated by a central counterparty, which fundamentally alters the role that borrower collateral plays.
The rest of this section comprises a preview of the paper, followed by a review of the literature and how this paper contributes to it.
1.1 Preview of the Paper
Our empirical analysis centres around reactions to the failure of Lehman Brothers in mid September 2008, which triggered a global financial crisis with disruptions to wholesale funding liquidity around the world. The Australian interbank markets present an ideal platform for identifying these reactions. There exist supervisory data on the positions that each borrower had with each lender each day in both the repo and unsecured markets, including the collateral type for repos, and how much collateral was held by each counterparty. Importantly, the Australian repo and unsecured markets were both operating through the same form of infrastructure, and overlapped substantially in bank participation. These characteristics permit a ceteris paribus analysis of the implications of collateral. In addition, the global financial crisis was largely exogenous to the Australian economy and its interbank market. The real estate market did not crash, and the economic slowdown was modest compared to other advanced economies.
With these data, we answer the following questions. How does the presence of collateral affect credit markets' responses to system-wide stress? Does availability of high-quality collateral matter? Do banks substitute between collateralised and uncollateralised markets? With data at the lender-borrower-day-market level, we can identify the impact of the Lehman shock conditional on ex ante borrower risk and ex ante collateral holdings, while controlling for lender behaviour. Controlling for lender behaviour is important because matching between borrowers and lenders is endogenous. The theoretical literature shows that lenders may choose their borrowers in similar (for monitoring) or in different (for risk diversification) businesses and geographical areas (Rochet and Tirole 1996; Freixas and Rochet 2008; Allen and Gale 2000, 2009). We find evidence of endogenous matching in our sample, and, consistent with the credit channel literature, control for it by saturating the regressions with fixed effects (e.g. Khwaja and Mian 2008; Jiménez et al 2017), though given the quality of the data, we can take further steps. For example, when analysing substitution between the repo and unsecured markets, we can use borrower*lender*day fixed effects, which removes influences that are common to both markets at any level, such as borrower-lender relationships that evolve. We also assess how these controls for unobserveables – and thus the channels they shut off – affect the estimates, to understand different drivers. Finally, we run placebo tests on data from 2006, a year without financial distress, and find no effects.
We find the following robust results. At the market level, after the Lehman Brothers default, the repo market expands substantially alongside the rise in the US TED spread, while the unsecured market remains flat.[1] Reactions to financial stress also differ across markets at the borrower (conditional on lender) level. In the unsecured market, loan volume reactions are negatively related to borrowers' ex ante balance sheet weakness (i.e. borrower risk). This occurs mostly in the intensive margin, that is, in the quantities borrowed rather than in the number of counterparties borrowed from. In the repo market, however, loan volume reactions are positively related to borrowers' ex ante high-quality collateral holdings. Importantly, the positive effect of ex ante collateral holdings is increasing in the degree of borrower risk. In the repo market, moreover, there is more activity in the extensive margin (i.e. number of counterparties borrowed from), consistent with collateral alleviating information asymmetries and facilitating faster changes to the borrower-lender network.
Our central specification combines data from both markets and formally tests whether collateralisation affects reactions to stress. The results confirm substitution behaviour in line with the abovementioned results on individual markets. After the Lehman shock, ex ante riskier borrowers shift from the unsecured to the repo market. This effect has a significant interaction with ex ante high-quality collateral holdings, such that the strongest shifts are by risky borrowers with large holdings of high-quality collateral. These patterns occur in the intensive and extensive margins. Overall, our results indicate that riskier borrowers are rationed from uncollateralised borrowing, while those with sufficient collateral can switch to collateralised borrowing.
We also analyse patterns across lenders and across collateral types. We do not find evidence of lending contractions related to cash hoarding. Rather, there is evidence of lending expansions related to heightened demand for liquid (and safe) assets. Heightened demand for high-quality collateral is evident from the interest rate differential on collateralised loans across collateral types – rates for first-best collateral fall market-wide by over 100 basis points relative to second-best (but still high quality) collateral. This encourages the riskier banks substituting to collateralised borrowing to borrow against first-best collateral, if they hold it ex ante. At the same time, there is an increase in collateralised lending by some banks with low ex ante collateral lending. Therefore, when collateral is high quality, it can facilitate the flow of liquidity beyond the role that it plays in mitigating counterparty risk.
1.2 Contribution to the Literature
Our contribution is to identify the effect of collateral on borrowing, by analysing a large exogenous shock in a setting where the effect of collateralisation can be isolated from confounding factors. We contribute to the large literature on credit, and in particular, the fields related to credit crunches and collateral. The setting we analyse is interbank markets – uncollateralised and collateralised side by side – around the Lehman Brothers failure. We also make an important contribution to the literature on interbank markets, where, to date, most papers analyse collateralised or uncollateralised interbank markets in isolation. In the few studies that cover both markets (see below), there are key risk-related differences between the two markets aside from the presence of collateral; namely, intermediation by a central counterparty, which obscures the analysis of collateral in market transactions.
The credit channel literature shows that borrower and loan characteristics are important determinants of credit allocation during credit crunches (e.g. Goldstein and Pauzner 2005; Chava and Roberts 2008; Ivashina and Scharfstein 2010; Chava and Purnanandam 2011; Chodorow-Reich 2014; Ivashina, Laeven and Moral-Benito 2020). Borrowers' access to collateral is key among these, as demonstrated by the effects of collateral constraints being tightened or loosened (e.g. Kiyotaki and Moore 1997; Chaney, Sraer and Thesmar 2012; Adelino, Schoar and Severino 2015; Campello and Larrain 2016). Several papers have also shown that lenders become more willing to lend collateralised during downturns (Lian and Ma forthcoming; Liberti and Sturgess 2014; Benmelech et al 2020a, 2020b; De Jonghe et al 2020). However, it is difficult to analyse the effect of collateralisation on borrowers' access to credit, because available data typically do not permit controlling for endogenous factors. In particular, the studies mentioned tend to analyse illiquid collateral, which can be deeply related to borrower characteristics, for multiple reasons. First, it is idiosyncratic in opaque dimensions, which opens up omitted variable bias problems. Second, the higher liquidation costs mean that its purpose is often to signal borrower quality rather than reduce lenders' losses given default (Berger, Frame and Ioannidou 2016). Third, illiquid collateral can have fire-sale externalities on other borrowers that use similar types of collateral (Benmelech and Bergman 2011).
Interbank markets present a laboratory in which the role of collateral can be separated from borrower characteristics, because the collateral is liquid, transparent and homogeneous across borrowers. Interbank markets also play a central role in monetary policy and the banking system, with a separate devoted literature, particularly on the effects of financial system stress. In unsecured interbank markets after the Lehman Brothers failure, riskier borrowers experience a reduction in credit or worse terms on their credit (Afonso et al 2011; Angelini, Nobili and Picillo 2011). For repo markets, detailed data are less available (Adrian et al 2014). Existing studies of repo markets under stress show an expansion of credit against liquid collateral (Krishnamurthy et al 2014), and a contraction of credit against collateral whose underlying markets are disrupted (Gorton and Metrick 2012). Our study provides granular evidence behind these patterns, with the first loan-level analysis of both markets side by side where the two markets operate through the same infrastructure. This reveals new results on the role of collateral. In particular, collateral can allow riskier borrowers to substitute markets, and can generate ‘reverse cash hoarding’, with funding conditions eased for riskier borrowers due to heightened demand for the collateral they hold.
The few studies that combine repo- and unsecured-market data during stressed periods analyse over-the-counter (OTC) unsecured markets alongside centrally cleared repo markets, and without loan-level data that include bank identities. These studies present evidence of substitution from unsecured to repo markets under stress (Mancini, Ranaldo and Wrampelmeyer 2016; di Filippo et al 2018; Piquard and Salakhova 2019). Mancini et al (2016) conclude that the outcomes are driven more by the central counterparty (CCP) infrastructure than by the presence of collateral, consistent with interbank studies emphasising the importance of market infrastructure during crises (Martin, Skeie and von Thadden 2014). Mancini et al write ‘the CCP-based segment represents the sole resilient part of the euro repo market. This suggests that anonymous CCP-based trading is key for repo market resilience’ (p 1773). We contribute by isolating the role of collateral, by analysing repo and unsecured markets that both operate bilaterally. Several other papers theoretically model the two types of interbank markets (e.g. Freixas and Holthausen 2005; De Fiore, Hoerova and Uhlig 2018).
Our paper also contributes to the safe asset literature. We show that the safest collateral in Australia remained information insensitive throughout the peak stress in 2008, in line with the theory of Gorton and Ordoñez (2014) and Dang, Gorton and Holmström (2015). We also document a spike in demand for safe assets relative to supply, with rates for borrowing against the safest collateral falling heavily (i.e. prices increasing), consistent with studies that postulate a safe-assets shortage that was exacerbated by that period (Caballero, Farhi and Gourinchas 2016, 2017).
2. Institutional Background and Data
This section provides background on the markets that we analyse and describes our data. Section 2.1 details characteristics of Australian interbank markets. Section 2.2 summarises the financial environment in Australia during the period under analysis. Section 2.3 describes our data sources and Section 2.4 describes the variables in our regressions.
2.1 Interbank Markets in Australia
In Australia the repo and unsecured interbank markets are over-the-counter and settled bilaterally (more detail on the infrastructure is in Appendix A). Banks use both markets to manage short-term liquidity needs, and the repo market also acts as a source of funding for securities holdings (Hing, Kelly and Olivan 2016; Becker and Rickards 2017).
In the unsecured market, the overnight interest rate is the Reserve Bank of Australia's (RBA's) monetary policy implementation target. The rate has historically displayed very little cross-sectional variance and has not been indicative of counterparty risk. The RBA keeps the rate at target by controlling the aggregate supply of reserve balances via RBA repo auctions each morning, and the lack of cross-sectional variance is due to strong commitment by the RBA combined with relatively low interbank default risk (although these dynamics have changed since COVID-19, see Kent (2020)). Statistics on market-wide rates outside of our sample reveal some instances of loans not at the target (Debelle 2007), although these appear to be driven by fund surpluses, with deviations mainly occurring below the target. Reactions to counterparty risk tend to occur through lending quantities (e.g. Brassil and Nodari 2018), consistent with OTC unsecured interbank markets in Europe (Abassi et al 2014) and the United States (Afonso et al 2011). Afonso et al (2011) write about the period around the Lehman Brothers default:
… interest rates in the fed funds markets did not become increasingly sensitive to bank performance metrics in a consistent manner ... Rather, the results suggest that lenders seem to be more likely to manage their risk exposure by the amount they lend to a particular bank or even whether they lend to the bank at all (p 1127).
The total value of RBA repos is large relative to the size of Australian interbank markets, typically representing more than half of the total repo value outstanding. However, there is some segmentation between RBA repos and interbank repos. RBA repos tend to have maturities ranging between one and several weeks, whereas the interbank market is focused on maturities up to one week. Also, RBA repos can be against private securities – and in our sample they mostly are – whereas the interbank repo market is heavily concentrated in AGS and securities issued by the Australian state governments (referred to as ‘semis’). AGS play a similar role in Australia to Treasuries in the United States, as the safest and most liquid assets, all rated AAA (or equivalent) in our sample, while semis are still considered very high quality, all rated AAA or AA+ (or equivalent) in our sample. AGS and semis are both included in the regulatory definition of high-quality liquid assets.
The unsecured and repo markets have strong overlap in participation – in our sample all of the banks participating directly in the repo market are also active in the unsecured market. Roughly three-quarters of the banks in the unsecured market and half of the banks in the repo market have foreign parents, which are mostly large international banks from the United States, the United Kingdom, Europe and Asia.[2] The Australian banks include all of the major Australian banks and some smaller Australian financial institutions.
2.2 The Australian Financial System Leading into the Crisis
The Australian financial system is not conspicuous among advanced economies. In 2007, Australia's bank assets were 114 per cent of GDP, relative to an average of 131 per cent across high-income OECD countries (Davis 2011). Australian banks were (and are) relatively concentrated in residential real estate loans, which in 2009 comprised 59 per cent of all loans, compared to, for example, 15 per cent in the United Kingdom and 38 per cent in the United States. Nonetheless, each of the major Australian banks had positive profits throughout the crisis, in part due to low investment in subprime mortgages relative to banks in other advanced economies. This helped Australia to avoid a severe downturn, with one quarter of negative GDP growth at end 2008.
Notwithstanding this, in the second half of 2008, funding conditions in Australia tightened substantially. The spread on Australian banks' short-term paper reached historical highs (Figure 1). In September banks' total bond issuance dropped to under a third of typical monthly issuance, and by November it had declined to almost zero. On 28 November, the Australian Government implemented a guarantee on banks' large deposits and wholesale debt (following similar guarantees in other countries), and a surge in bond issuance followed.
The funding tightness is also evident from the rapid rise of Exchange Settlement Account (ESA) balances to historical highs around when Lehman Brothers defaulted on 15 September (Figure 2). The expansion of ESA balances was driven by RBA repos against lower-quality securities, as banks preferred to hold onto their safest securities for other purposes, which the RBA deliberately facilitated through its auction allocations. On 8 October, immediately after our sample, the RBA expanded its eligible repo collateral to include a wider range of residential mortgage-backed securities and asset-backed commercial paper, and offered repos of extended maturities. On the same day, the RBA lowered its policy rate by 1 percentage point, the first change greater than half a percentage point in magnitude since 1992. This rapid easing occurred a day before the unscheduled and coordinated decisions by six other central banks to each cut policy rates by half a percentage point, including the Bank of England, the European Central Bank and the Federal Reserve.
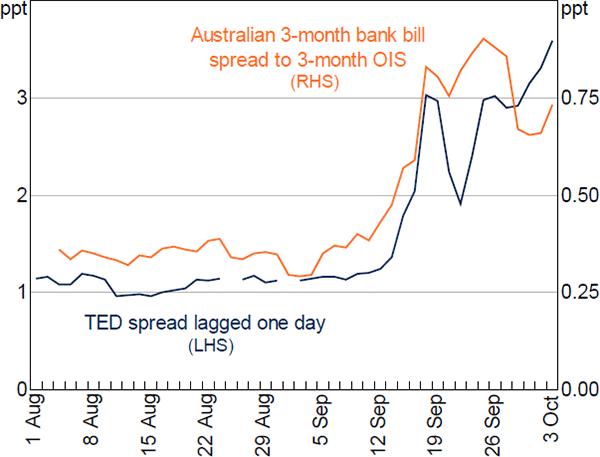
Sources: Federal Reserve Bank of St. Louis; RBA
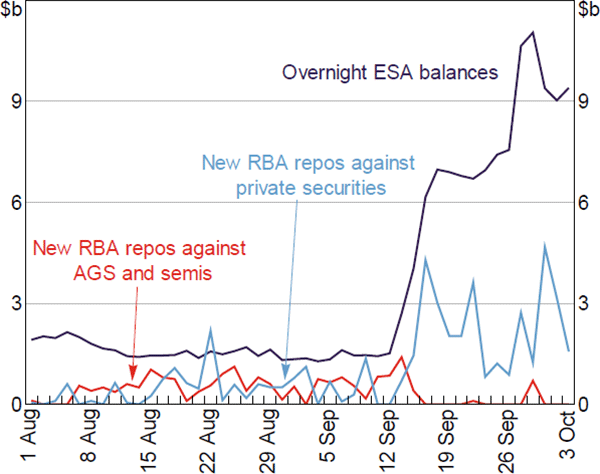
Source: RBA
2.3 Data Sources
Our transaction-level repo data, and data on participants' collateral holdings, come from two proprietary datasets that contain all overnight holdings and intraday changes in holdings (i.e. transactions) of most categories of debt securities held in Australia's debt securities infrastructure, Austraclear.[3] To identify the securities transactions that comprise repos, we use the algorithm from Garvin (2018), which detects pairs or groups of transactions that involve the same counterparties, the same type and face value of securities, and money quantities consistent with a feasible repo rate. We calibrate the algorithm to detect repos open for eight days or less with implied interest rates between 3 and 7.25 per cent, which the data indicate are realistic bounds.[4] The analysis in Garvin (2018) indicates that the algorithm detects repos with high accuracy.
The unsecured lending data come from a proprietary transaction-level dataset that contains all payments through the Reserve Bank Information and Transfer System (RITS). We use the algorithm in Brassil, Hughson and McManus (2016) to identify which payments are interbank loans. Brassil et al (2016) enhance the Furfine (1999) algorithm, which detects pairs of transactions that comprise interbank loans, to detect loans that involve more than two transactions. This picks up many otherwise-missed loans. Both our repo and unsecured datasets therefore capture loans whose principal is increased or decreased (or both) before it is fully repaid. Due to the transaction-level nature of the data, we (and other users of related algorithms) cannot explicitly distinguish between overnight loans that are subsequently rolled over without transacting, and loans that upon initiation are agreed to be for multiple days (i.e. short-maturity term loans). Our analysis groups these loan types together and, within the short-term category, puts little emphasis on maturities, instead focusing on quantity of loans outstanding at any point in time.
Data relating to entities' balance sheets are from S&P Global Market Intelligence (formerly from SNL Financial) or, if unavailable or problematic, from public financial reports. In Section 4.3 we also analyse banks' borrowing from the RBA. These data come from the securities transactions dataset, and are inferred from banks' transactions with the RBA's open market operations account.
2.4 Sample and Variables
We focus on loans outstanding during a four-week period from Monday 8 September to Friday 3 October 2008. By starting the sample shortly before the Lehman Brothers default, we can construct an ex ante (i.e. exogenous) measure of collateral holdings that remains relevant to the stressed period we examine. Our sample ends on Friday 3 October just prior to major RBA interventions that targeted interbank markets (see Section 2.2). The effect of these interventions on individual banks was likely related to the bank characteristics we use as explanatory variables, so we end the sample just before this period. For placebo regressions we also analyse a four-week sample of corresponding data covering Monday 11 September 2006 to Friday 6 October 2006.
Banks are grouped at the parent company level, in some instances aggregating across multiple Austraclear accounts. Some accounts banks use on behalf of clients – indicated by the account name – which we treat as entities separate from the bank holding the account, with missing observations for counterparty characteristics (which appropriately removes them from some regressions).[5] We remove one non-major Australian bank owing to in-sample corporate activity and a relatively inactive financial company with unavailable balance sheet data.
The key dependent variable is a balanced panel of outstanding loan balances at the lender-borrower-day-market level (LoanValueslbdm). These include loans of 8 days or less that were open at any point during the 20 business days from Monday 8 September to Friday 3 October 2008. To construct LoanValueslbdm, we sum all loans from lender l to borrower b that occurred in market m and were open at the end of day d. Usually m {unsecured, repo}; in Section 4.4 repo is separated into two markets by collateral type. If lender l never lent to borrower b in the sample or sub-sample analysed, the lb counterparty pair is excluded; if lender l lent to borrower b but not on day d in market m then LoanValueslbdm is zero. We measure outstanding loans in millions of AUD, add one, then take the natural logarithm; the raw distribution of loan sizes across entities is heavily skewed. The pre-log values of LoanValueslbdm are summarised in Panel A of Table 1. To separately analyse the extensive margin, we construct a Participationlbdm indicator variable equal to one if and only if LoanValueslbdm is greater than zero.
The key explanatory variables are collateral holdings ( Collaterali ), counterparty risk ( CPRiski ) and a Lehman default indicator variable ( LBd ).LBd is equal to 1 for all observations from Monday 15 September, the first business day after Lehman Brothers declared bankruptcy. Collateral holdings and counterparty risk are at the bank level, with banks indexed by i, which is replaced by l or b when included in regressions. In some instances collateral holdings are split into subcomponents (i.e. AGSi and Semisi). Collateral (or, where used, its subcomponents) and counterparty risk are standardised to have zero mean and unit variance. Panel B of Table 1 reports the means and standard deviations before standardisation.
Panel A: Loans outstanding at lender-borrower-day-market level, in AUD millions, pre-logs | ||||||||||
---|---|---|---|---|---|---|---|---|---|---|
Count | 25% | Median | Mean | 75% | 99% | Max | Std dev | |||
Repo | All | 8,100 | 0 | 0 | 11 | 0 | 206 | 2,591 | 79 | |
Non-zero | 1,124 | 10 | 29 | 79 | 70 | 928 | 2,591 | 198 | ||
Unsecured | All | 8,100 | 0 | 0 | 19 | 0 | 400 | 1,480 | 78 | |
Non-zero | 997 | 40 | 100 | 154 | 210 | 779 | 1,480 | 169 | ||
Panel B: Bank-level explanatory variables, before mean/variance standardisation | ||||||||||
Min | Mean | Max | Std dev | Min | Mean | Max | Std dev | |||
CPRiski | 0 | 1.15 | 3.49 | 0.97 | Assets (AUDtr) | 0.02 | 1.23 | 4.17 | 1.11 | |
Collaterali | 0 | 0.42 | 1.70 | 0.56 | Liabilities/assets | 0.82 | 0.94 | 0.98 | 0.03 | |
AGSi | 0 | 0.12 | 0.81 | 0.22 | Foreign (indicator) | 0.72 | ||||
Semisi | 0 | 0.36 | 1.69 | 0.50 | ||||||
Panel C: Number of unique lenders (borrowers) to each borrower (lender) across sample | ||||||||||
Lenders to each borrower | Borrowers to each lender | |||||||||
Min | Mean | Max | Std dev | Min | Mean | Max | Std dev | |||
Repo | 0 | 4.67 | 17 | 5.54 | 0 | 3.82 | 14 | 5.11 | ||
Unsecured | 0 | 8.31 | 22 | 6.26 | 0 | 6.80 | 18 | 5.33 | ||
Notes: Panel A reports statistics on the dependent variable LoanValueslbdm before log transformations. Lender-borrower pairs are included if they appear in any of the regressions and if one of the pair has observed counterparty characteristics (e.g. is not an unidentifiable client). Loans outstanding is zero if that lender has no open loans to that borrower on that day in that market. ‘All’ summarises all observations and ‘Non-zero’ summarises only the positive observations. Panel B reports statistics on bank-level variables. AGSi and Semisi correspond to the AGS and semis components of Collaterali , also in log AUD billions plus one. ‘Assets’ and ‘Liabilities/assets’ are from the bank's (parent company's) balance sheet at the same date as NPLi is measured. Foreign is an indicator variable for whether the bank (or its parent company) is foreign. The zero minimum CPRiski corresponds to a financial services company that reported zero provisions for loan losses in its 2007 annual report. See the Section 2.4 text for more details. Panel C reports how many different lender or borrower counterparties each bank had across the full sample, separated by market. All entities that appear in the sample are included. |
More specifically, Collaterali measures the total face value of AGS and semis that were held at both 1 September 2008 and 8 September 2008. Including only securities that were held at both dates minimises the influence of high-frequency changes in holdings prior to the sample start.[6] We measure this in billions of AUD, add one, then take the natural logarithm. Because collateral holdings is an ex ante measure, it is best interpreted as a soft constraint on repo borrowing. Banks could have acquired more collateral between the first week of September and the weeks in our sample, but it would have entailed transaction costs that were likely intensified by the tight liquidity conditions.
CPRiski measures the ratio of non-performing loans over total loans in percentage points (NPL), as at end 2007 or the closest available reporting date to end 2007. This is a common measure of counterparty risk in interbank markets, used by, for example, Cocco, Gomes and Martins (2009) and Afonso et al (2011). NPL has several advantages as a measure of counterparty risk in this setting:
it measures banks' assets at the lower tail of the quality distribution, of which subprime mortgages are likely to be a component; it depends directly on balance sheet outcomes, without determination by market speculation or credit rating agencies, which can provide biased forward-looking information before a crisis (Bolton, Freixas and Shapiro 2012); and it is available for all banks in our sample. Section 5 presents further evidence that NPL is a suitable measure of counterparty risk. For a small number of entities, non-performing loans are not reported, in which case we replace non-performing loans with the closest available measure to debt assets that are 90 days or more past due.
The sample includes 30 borrowers and 31 lenders with observations of Collaterali and CPRiski (i.e. banks) and 42 lenders and 48 borrowers in total. In each market, there are typically about 20 active on any day, and around 50 to 60 active counterparty pairs. Panel C of Table 1 provides summary statistics for how many counterparties each bank dealt with in each market.
3. Empirical Strategy
Our objective is to compare how collateralised and uncollateralised markets respond to system-wide stress, and how the responses depend on borrowers' and lenders' counterparty risk and collateral availability. To achieve this we regress interbank activity at the lender-borrower-day-market ( lbdm ) level in each market, and the difference in activity across markets, on an interaction between market-wide stress and ex ante bank characteristics. The idea is that following unexpected system-wide stress, differences in risk characteristics and available collateral levels lead to unanticipated differences in access to credit in the two markets. The within-market aspect of this approach is similar to studies of heterogeneous effects of monetary policy changes (e.g., Kashyap and Stein 2000; Jiménez et al 2014) and to analysis of US interbank markets around the Lehman Brothers default (Afonso et al 2011). With the cross-market dimension, the strategy can be interpreted as a triple differences-in-differences with heterogeneous treatment effects.
Identification rests on an assumption that the measure of market stress is exogenous, that is, it is not driven by the bank behaviour that we analyse. We have high confidence that conditions in Australian interbank markets had no material contribution to the Lehman Brothers default, owing to the difference in size and global centrality between the US and Australian financial systems. This is an advantage of focusing on the Australian interbank market. To maintain exogeneity of borrower (and lender) counterparty risk and collateral holdings, they are measured at the start of the sample or earlier. By focusing the sample on a relatively tight window around the Lehman Brothers default, we ensure that the pre-sample measure of collateral holdings – a characteristic that can endogenously change relatively quickly – is both relevant and exogenous.
We first examine three ‘individual’ markets: repo, unsecured, and treating both as a single market (i.e. summing lbdm -level observations across markets to produce lbd -level variables). The explanatory variables of interest are the interactions between the system-wide stress measure and the counterparty characteristics represented by Xi , where
To ensure that estimated coefficients are not biased by endogenous counterparty selection, we include counterparty*day fixed effects (e.g. when analysing borrower characteristics, lender*day).
The theoretical literature argues that monitoring incentives and diversification motives are important for interbank transactions (Rochet and Tirole 1996; Freixas and Rochet 2008; Allen and Gale 2009), which implies that lenders choose their borrowers in similar (for monitoring) or in different (for risk diversification) businesses and geographical areas. These fixed effects ensure that, for example, if a lender's behaviour reacts to system-wide stress, it is not attributed to the borrowers that it lends to. When analysing borrower characteristics, we also include borrower fixed effects (and similar for lenders), so that all time-invariant bank-level unobservables are controlled for. Our individual-markets regression specification for analysing borrower characteristics is
To analyse lender characteristics, the l and b subscripts are swapped.
Next we formally compare the difference between the repo and unsecured markets. The variables of interest are triple interactions between an indicator variable for whether the market is collateralised ( 1s ), market stress ( LBd ), and counterparty characteristics ( Xi ). Following the credit channel literature, we saturate the regressions with fixed effects to control for unobserved variation (e.g. Khwaja and Mian 2008; Jiménez et al 2017); in our case lender*borrower*day fixed effects. This isolates the difference between the two markets, removing common variance driven by, for example, time-varying counterparty relationships. The coefficients on these triple interactions tell us whether, in reaction to market stress, the allocation of borrowing between repo and unsecured markets changes more for certain types of borrowers than others. In other words, whether substitution between markets is determined by bank-level characteristics. To analyse borrower characteristics, the regression specification is
where is the coefficient vector of interest. Again, to analyse lender characteristics, the l and b subscripts are swapped.
The regressions are also repeated with the dependent variable replaced by Participationlbdm using a linear probability model. This specification reveals whether changes in LoanValueslbdm also took place at the extensive margin, that is, whether borrowers (lenders) changed the number of lenders (borrowers) they dealt with. Specifically, if a borrower borrows from N different lenders throughout the sample (summarised in Panel C of Table 1), an estimated coefficient of x implies a change in the number of lenders on a given day by xN.[7]
As a placebo test, the regressions are re-run on the corresponding 2006 sample, using 2008 values for the Lehman Brothers default treatment variable, in line with the suggestion of Roberts and Whited (2013). All loan-related and bank-characteristic variables are constructed as described in Section 2.4, but using 2006 data. The sample also starts on the second Monday in September, running for four weeks until Friday 6 October (with 19 days owing to a public holiday in this period).
This also demonstrates robustness against seasonal factors such as quarter-end or time-of-month effects.
4. The Repo and Unsecured Markets' Reactions to Stress
The paths of aggregate activity in the repo and unsecured markets diverge after the shock to market-wide stress. When the TED spread rises following the Lehman Brothers default, the unsecured market remains relatively flat (Figure 3, left panel). In contrast, the repo market expands, with the pick-up in activity moving roughly in line with the TED spread.[8] The aggregates from the 2006 placebo sample demonstrate that these patterns are particular to 2008 (Figure 3, right panel); this is examined formally in Section 5. Mancini et al (2016) find that stress generated negative correlations in trading volumes across the European repo and unsecured markets. The data in Figure 3 show a similar pattern. The cross-market correlations in the 2008 and 2006 samples are −0.36 and 0.46, respectively.
The Australian repo market expansion is consistent with the resiliency documented in US and European repo markets against high-quality securities (Copeland, Martin and Walker 2014; Mancini et al 2016), and in contrast to the tightening observed in US repo markets against private collateral (Gorton and Metrick 2012). Using the terminology of Dang et al (2015), collateral in the Australian market remained information insensitive.
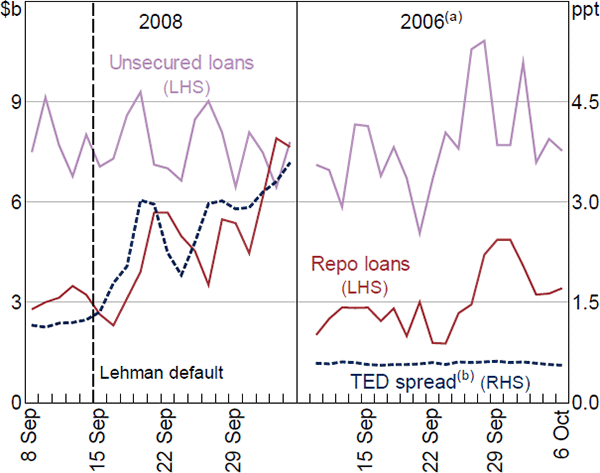
Notes:
(a) Monday 2 October 2006 was a public holiday, loans outstanding taken from the previous business day
(b) Lagged one day to account for time zones
Sources: ASX; Authors' calculations; Federal Reserve Bank of St. Louis; RBA
4.1 Heterogeneity across Borrowers
Previous research establishes that unsecured borrowers react heterogeneously to stress, and that repo and unsecured markets respond differently in aggregate (see Section 1.1). In this section we fill the gap between these sets of findings: how does borrower heterogeneity differ between repo and unsecured markets? The results show that borrower default risk plays a stronger role in the unsecured market, while borrower collateral holdings are more important in the repo market.
First the difference in reactions is visualised by plotting unsecured borrowing against repo borrowing for sub-samples of interest (Figure 4). Each scatterpoint represents the demeaned log borrowing of a bank on a given day in the repo (X-axis) and unsecured (Y-axis) markets, excluding banks that did not borrow in both markets.[9] A positive relationship indicates that banks are spreading their fluctuating liquidity needs across both markets. A negative relationship suggests that they are substituting between the two markets, on any given day concentrating their borrowing in only one.
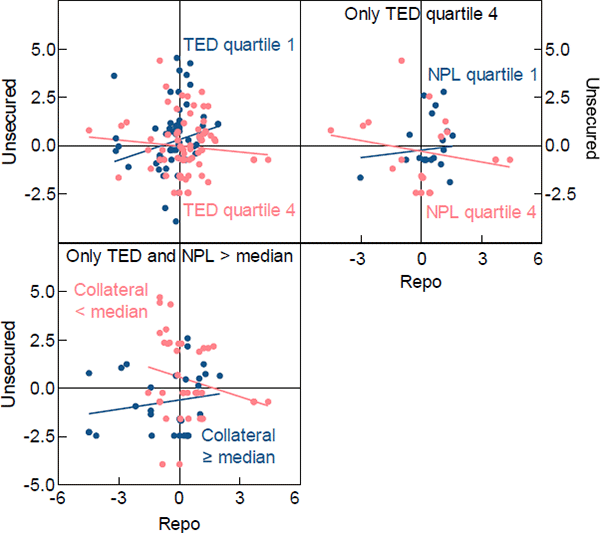
Notes: Natural logarithm of borrower-day-market level observation in AUD millions plus one, with borrower-market mean subtracted; fitted lines for sub-samples using OLS
Sources: ASX; Authors' calculations; RBA
The slopes of the fitted lines, each representing particular subsets of days and borrowers, fit the following description. First, on high-stress days banks face tighter borrowing constraints, which generates greater cross-market substitution than on low-stress days (top-left panel). Second, on high-stress days, these constraints are more likely to bind for riskier borrowers (top-right panel). Third, among the riskier borrowers, it is those with the most collateral that are most capable of substituting across markets (bottom-left panel).[10]
Our empirical strategy controls for potentially confounding factors in these relationships. One of these factors is that counterparties are not randomly matched with respect to the characteristics we examine. We demonstrate this with a modification of specification (1) that examines whether interactions between borrower and lender characteristics explain interbank activity (Table 2). In both markets, high counterparty-risk lenders tend to lend to high counterparty-risk borrowers (first row, columns (a) to (f)), and, in the unsecured market, high counterparty-risk borrowers tend to borrow from high-collateral lenders (third row, columns (a) and (b)). The causes of these correlations are not entirely clear – Jaremski and Wheelock (2020) argue that there is still much to understand about interbank network formation, and Brassil and Nodari (2018) show that shocks can have long-lasting impacts on interbank network structures. Nonetheless, the patterns demonstrate the importance of controlling for the behaviour of counterparties. In regression specifications (1) and (2), we achieve this with counterparty*day fixed effects.
Market: | Unsecured | Repo | Total | |||||
---|---|---|---|---|---|---|---|---|
Regressand: | LoanValueslbd | Participationlbd | LoanValueslbd | Participationlbd | LoanValueslbd | Participationlbd | ||
(a) | (b) | (c) | (d) | (e) | (f) | |||
CPRiskb * CPRiskl | 0.065 (0.039) |
0.014*** (0.005) |
0.042 (0.044) |
0.031 (0.018) |
0.119** (0.048) |
0.033*** (0.010) |
||
Collateralb * Collaterall | 0.078 (0.048) |
0.017 (0.011) |
−0.010 (0.100) |
−0.009 (0.020) |
0.092 (0.075) |
0.015 (0.015) |
||
CPRiskb * Collaterall | 0.080*** (0.018) |
0.016*** (0.003) |
0.056 (0.092) |
0.023 (0.020) |
0.052 (0.067) |
0.010 (0.013) |
||
Collateralb * CPRiskl | −0.050 (0.058) |
−0.010 (0.010) |
0.001 (0.064) |
0.004 (0.019) |
−0.018 (0.069) |
0.000 (0.014) |
||
Lower-level interactions | Yes | |||||||
Fixed effects | Borrower and Lender and Day | |||||||
Observations | 5,340 | 5,340 | 2,840 | 2,840 | 7,000 | 7,000 | ||
R-squared | 0.095 | 0.074 | 0.168 | 0.118 | 0.138 | 0.120 | ||
Notes: *, ** and *** denote significance at the 10, 5 and 1 per cent levels, respectively. This table contains results from regressing loans outstanding, in log millions plus one ( LoanValueslbd ), and an indicator variable for whether LoanValueslbd is positive ( Participationlbd ), on interactions between borrower and lender characteristics. Columns (a) and (b) use the unsecured sample, columns (c) and (d) use the repo sample, and columns (e) and (f) treat the two markets as a single combined market. The pre-interacted explanatory variables are each standardised to zero mean and unit variance. The full variable definitions are in Section 2.4. Standard errors are clustered at the borrower and lender and day levels. |
The borrower results from specification (1) are in Table 3. In the unsecured market, market stress causes a relative decline in riskier banks' borrowing, irrespective of how much collateral they hold (columns (a) to (d)). Afonso et al (2011) find a similar negative interaction between the Lehman Brothers default and counterparty risk on unsecured borrowing activity.[11] To interpret the effect size implied by the −0.247 coefficient in column (b), consider a representative scenario, with banks A and B that each have $150 million outstanding unsecured loans per engaged lender:
bank A has mean; CPRiskb and
bank B has CPRiskb one standard deviation above the mean.
The riskier bank B is estimated to reduce borrowing (from each lender) by $33 million more than bank A. This is illustrated in Figure 5 by the light bars. The effect of counterparty risk on borrowers' participation – that is, number of lender counterparties – is also statistically significant (columns (c) and (d)), but not large. Consider again the scenario above. If both banks borrow unsecured from 10 lenders throughout the sample (see Table 1, panel C), the coefficient of −0.049 implies that borrower B has 0.5 fewer counterparties on an average day after the Lehman Brothers default, relative to borrower A. The effect of counterparty risk is therefore mostly in the intensive margin – banks reduced the quantities lent to risky borrowers, rather than cutting lending altogether.
In the repo market, borrowers' collateral holdings are stronger determinants of reactions to stress than their counterparty risk (Table 3, columns (e) to (h)). Borrower counterparty risk does not have a significant effect for a borrower with mean collateral holdings. In contrast, collateral holdings determine borrowing directly as well as through the interaction with counterparty risk (columns (f) and (h)). To interpret the coefficient sizes, consider another representative scenario, with banks A, B and C that each have $80 million repo loans outstanding per engaged lender:
bank A has mean CPRiskb and mean Collateralb;
bank B has mean CPRiskb and Collateralb one standard deviation above the mean;
bank C has CPRiskb one standard deviation above the mean and Collateralb one standard deviation above the mean.
In this scenario, the coefficients in column (f) estimate that bank B increases borrowing by $25 million per lender more than the lower collateral bank A. However, for bank C, whose CPRiskb is also one standard deviation above the mean, the effects of high counterparty risk and high collateral holdings compound, and its borrowing is estimated to increase by $70 million more than bank A. Alternatively, if bank C's CPRiskb was one standard deviation below the mean, the effects of high collateral and low counterparty risk offset each other, and the change in borrowing is estimated to be roughly equal to bank A. These estimated effects are illustrated in Figure 5 by the dark bars.
Market: | Unsecured | Repo | Total | ||||||||||||||
---|---|---|---|---|---|---|---|---|---|---|---|---|---|---|---|---|---|
Regressand: |
LoanValueslbd | Participationlbd | LoanValueslbd | Participationlbd | LoanValueslbd | Participationlbd | |||||||||||
(a) | (b) | (c) | (d) | (e) | (f) | (g) | (h) | (i) | (j) | (k) | (l) | ||||||
LBd * | |||||||||||||||||
CPRiskb | −0.166*** (0.053) |
−0.247** (0.090) |
−0.038*** (0.009) |
−0.049** (0.019) |
−0.171 (0.134) |
−0.019 (0.117) |
−0.026 (0.045) |
0.023 (0.032) |
−0.178* (0.085) |
−0.141 (0.103) |
−0.033 (0.020) |
−0.013 (0.024) |
|||||
Collateralb | 0.108 (0.087) |
0.019 (0.108) |
0.013 (0.018) |
0.001 (0.023) |
0.053 (0.095) |
0.267*** (0.082) |
0.015 (0.027) |
0.084*** (0.020) |
0.109 (0.071) |
0.149 (0.091) |
0.021 (0.016) |
0.043* (0.024) |
|||||
CPRiskb * Collateralb | −0.151 (0.127) |
−0.021 (0.030) |
0.358*** (0.097) |
0.117*** (0.014) |
0.073 (0.122) |
0.039 (0.029) |
|||||||||||
Fixed effects | Borrower and Lender * Day | ||||||||||||||||
Observations | 5,780 | 5,780 | 5,780 | 5,780 | 3,020 | 3,020 | 3,020 | 3,020 | 7,660 | 7,660 | 7,660 | 7,660 | |||||
R-squared | 0.227 | 0.227 | 0.219 | 0.219 | 0.248 | 0.251 | 0.218 | 0.222 | 0.224 | 0.224 | 0.215 | 0.216 | |||||
Notes: *, ** and *** denote significance at the 10, 5 and 1 per cent levels, respectively. This table reports estimates from lender-borrower-day level regressions on sub-samples containing a particular interbank market (i.e. specification (1)). We regress loans outstanding, in log millions plus one ( LoanValueslbd ), and an indicator variable for whether LoanValueslbd is positive ( Participationlbd ), on interactions between borrower characteristics and market stress. Columns (a) to (d) use the unsecured sample, columns (e) to (h) use the repo sample, and columns (i) to (l) treat the two markets as a single combined market. The pre-interacted explanatory variables are borrowers' counterparty risk ( CPRiskb ), borrowers' pre-sample high-quality collateral holdings ( Collateralb ) and an indicator variable equal to one after the Lehman Brothers default ( LBd ). CPRiskb and Collateralb are standardised to zero mean and unit variance. The full variable definitions are in Section 2.4. Standard errors are clustered at the borrower and lender and day levels. |
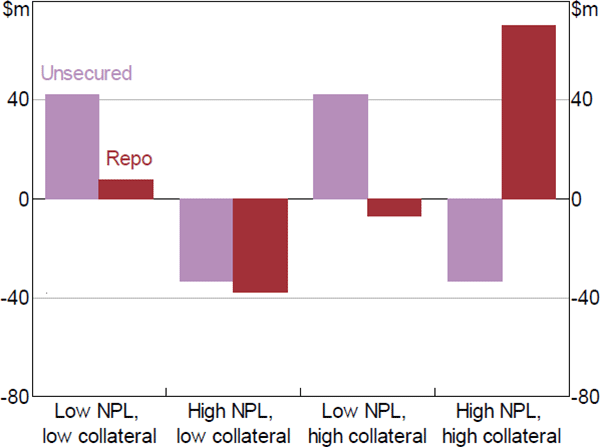
Krishanmurthy et al (2014) find that after the Lehman Brothers default, dealers' repo borrowing from money market funds becomes negatively correlated to how much of the dealers' borrowing is against lower-quality collateral. They conclude that this was driven by the collateral that these dealers held. Our formal results show that collateral holdings are indeed a significant determinant of reactions to stress, in support of their conclusion. di Filippo et al (2018) find a positive effect of counterparty risk on repo borrowing, in a specification without collateral holdings. We do not find a similar positive effect, but show that counterparty risk increases the positive effect of collateral holdings on repo borrowing.
The estimated effect of collateral holdings on repo borrowing is through both the intensive and extensive margins. Consider the same scenarios discussed two paragraphs earlier, with the three banks each borrowing repo from ten different lenders throughout the sample (see Table 1, panel C). Bank C is estimated to borrow from two additional lenders after the shock, relative to bank A.
The result that extensive margin responses are more pronounced in the repo market than the unsecured market is consistent with theory that collateral reduces information frictions. If the collateral is high enough quality, banks can trade with additional counterparties without first seeking information about expected default losses (Dang et al 2015). Relative to unsecured lending, collateralisation should therefore facilitate faster changes in the network of lending relationships. In line with this interpretation, the number of active counterparty pairs in the repo market picks up by 16 per cent in our sample, from a mean of 50 before the Lehman Brothers default to 58 afterward, while in the unsecured market, the corresponding means change by half that amount, from 53 to 49.[12]
When repo and unsecured borrowing are combined into a single measure of interbank borrowing, we do not find a robust significant net effect of counterparty risk (columns (i) to (l) of Table 3). However, collateral holdings are a significant determinant of cross-sectional differences in extensive-margin reactions, driven by the repo market behaviour discussed above.
4.2 Borrowers' Substitution between the Repo and Unsecured Markets
Specification (2) formally tests whether borrowers' reactions to stress differ across the collateralised and uncollateralised markets. We reject the hypothesis that collateralisation has no effect on interbank market functioning (Table 4), consistent with Section 4.1. Riskier borrowers shift towards the repo market. For riskier borrowers with large collateral holdings, the shift is significantly larger. Note also that aggregate repo borrowing is growing while unsecured borrowing is relatively flat (Figure 3), which suggests that substitutions into the repo market would have likely more than offset any decline in unsecured borrowing.
Regressand: | LoanValueslbdm | Participationlbdm | |||
---|---|---|---|---|---|
(a) | (b) | (c) | (d) | ||
1s * LBd * | |||||
CPRiskb | 0.091*** (0.020) |
0.166*** (0.032) |
0.026** (0.009) |
0.047*** (0.012) |
|
Collateralb | −0.023 (0.057) |
0.061 (0.070) |
0.005 (0.016) |
0.029* (0.015) |
|
CPRiskb * Collateralb | 0.151** (0.061) |
0.043*** (0.015) |
|||
Lower-level interactions | Yes | ||||
Fixed effects | Borrower * Lender * Day | ||||
Observations | 15,600 | 15,600 | 15,600 | 15,600 | |
R-squared | 0.531 | 0.533 | 0.504 | 0.505 | |
Notes: *, ** and *** denote significance at the 10, 5 and 1 per cent levels, respectively. This table reports estimates from lender-borrower-day-market level regressions on a sample containing the unsecured and repo markets (i.e. specification (2)). We regress loans outstanding, in log millions plus one ( LoanValueslbdm ), and an indicator variable for whether LoanValueslbdm is positive ( Participationlbdm ), on interactions between borrower characteristics, market stress and an indicator variable for whether the observation is on the repo market. The pre-interacted explanatory variables are borrowers' counterparty risk ( CPRiskb ), borrowers' pre-sample high-quality collateral holdings ( Collateralb ), an indicator variable equal to one after the Lehman Brothers default ( LBd ), and the repo-market indicator ( 1s ). CPRiskb and Collateralb are standardised to zero mean and unit variance. The full variable definitions are in Section 2.4. Standard errors are clustered at the borrower and lender and day levels. |
To analyse the robustness of this key result to model specification and omitted variables, we re-estimate specification (2) for a wide range of fixed effects combinations, focusing on the coefficient of interest on 1s × LBd × CPRiskb × Collateralb. Oster (2019) demonstrates that as controls are added to a regression, the ratio of the change in coefficients to the change in R-squared is indicative of the degree of omitted variable bias remaining. As we add fixed effects, the coefficient estimates are stable (slightly increasing), while the explained variance ranges from 3 per cent to 70 per cent (Table 5). This is strong evidence that our results are not being overstated by omitted variable bias. The coefficient stability also addresses potential concerns about fixed effects narrowing the variance of interest to unrepresentative sub-samples (Miller, Shenhav and Grosz 2019).
These results also add support to our choice of specification (2). The marked change in R-squared demonstrates that unobservables contribute substantially to interbank activity, which appears common in analysis of interbank lending quantities (e.g. Cocco et al 2009; Afonso et al 2011; di Filippo et al 2018), and is consistent with theory that random liquidity shocks are the main driver of activity (e.g. Allen, Carletti and Gale 2009; Freixas, Martin and Skeie 2011). Fixed effects saturation is therefore appropriate. Moreover, these results show that the most influential level of fixed effects is borrower * lender * day (see columns (i) and (j), Table 5), supporting our choice for specification (2).[13]
(a) | (b) | (c) | (d) | (e) | (f) | (g) | (h) | (i) | (j) | (k) | (l) | (m) | |
---|---|---|---|---|---|---|---|---|---|---|---|---|---|
Regressand: LoanValueslbdm | |||||||||||||
1s * LBd * CPRiskb * Collateralb | 0.151 | 0.151 | 0.151 | 0.151 | 0.151 | 0.151 | 0.151 | 0.151 | 0.151 | 0.151 | 0.151 | 0.151 | 0.172 |
Statistical significance | ** | ** | ** | ** | ** | ** | ** | ** | ** | ** | ** | * | *** |
R-squared | 0.023 | 0.044 | 0.045 | 0.088 | 0.079 | 0.112 | 0.192 | 0.122 | 0.168 | 0.533 | 0.533 | 0.668 | 0.706 |
Regressand: Participationlbdm | |||||||||||||
1s * LBd * CPRiskb * Collateralb | 0.043 | 0.043 | 0.043 | 0.043 | 0.043 | 0.043 | 0.043 | 0.043 | 0.043 | 0.043 | 0.043 | 0.043 | 0.047 |
Statistical significance | ** | ** | ** | ** | *** | ** | ** | *** | *** | *** | *** | ** | *** |
R-squared | 0.025 | 0.044 | 0.045 | 0.080 | 0.078 | 0.112 | 0.158 | 0.113 | 0.163 | 0.505 | 0.505 | 0.660 | 0.702 |
Lower-level interactions | yes | yes | yes | yes | yes | yes | yes | yes | yes | yes | yes | yes | yes |
Fixed effects | |||||||||||||
Borrower | no | yes | yes | yes | . | no | . | . | . | . | . | . | . |
Day | no | no | yes | yes | . | . | no | . | . | . | . | . | . |
Lender | no | no | no | yes | no | . | . | yes | . | . | . | . | . |
Secured | no | no | no | no | no | no | no | no | no | no | yes | . | . |
Borrower * Day | no | no | no | no | yes | no | no | yes | yes | . | . | . | . |
Lender * Day | no | no | no | no | no | yes | no | no | yes | . | . | . | . |
Borrower * Lender | no | no | no | no | no | no | yes | no | no | . | . | . | . |
Borrower * Lender * Day | no | no | no | no | no | no | no | no | no | yes | yes | yes | yes |
Secured * Borrower * Lender | no | no | no | no | no | no | no | no | no | no | no | yes | yes |
Secured * Lender * Day | no | no | no | no | no | no | no | no | no | no | no | no | yes |
Notes: *, ** and *** denote significance at the 10, 5 and 1 per cent levels, respectively. This table reports the primary coefficient of interest from specification (2), i.e. the interaction between borrower counterparty risk, borrower collateral, market stress and the repo market indicator, across varying levels of fixed effects. The dependent variables are loans outstanding, in log millions plus one ( LoanValueslbdm ), and an indicator variable for whether LoanValueslbdm is positive ( Participationlbdm ). The pre-interacted explanatory variables are borrowers' counterparty risk ( CPRiskb ), borrowers' pre-sample high-quality collateral holdings ( Collateralb ), an indicator variable equal to one after the Lehman Brothers default ( LBd ), and the repo market indicator ( 1s ). CPRiskb and Collateralb are standardised to zero mean and unit variance. The full variable definitions are in Section 2.4. Standard errors are clustered at the borrower and lender and day levels. |
4.3 Heterogeneity across Lenders
This section explores how responses to stress vary across lender characteristics. A reduction in unsecured lending could be consistent with banks building up liquidity buffers to protect against future illiquidity (e.g. Acharya and Skeie 2011; Gale and Yorulmazer 2013). Two potential manifestations in our sample are the following. First, banks with more solvency risk could reduce unsecured lending due to fear of funding runs. Second, banks could reduce unsecured lending because they hold less collateral, and therefore have less capacity to handle a future shock. In a high-quality collateral repo market, however, it is a priori unclear how liquidity hoarding would relate to lending activity. Each lender's reduction in cash is offset by an increase in liquid assets, with little net effect on the bank's access to liquidity.
We explore lending motives by applying specification (1) to lender characteristics, and also by exploring how lenders' characteristics determine their repo borrowing from the RBA. RBA repos are informative about interbank lending because banks sometimes fund their interbank lending by borrowing from the RBA. RBA repos are less relevant for the borrower analysis in Section 4.1 because they are not a direct substitute for interbank borrowing (see Section 2.1).
There is little supporting evidence for liquidity hoarding in the unsecured market (Table 6, columns (a) to (d)). The coefficients on CPRiskl are small and not significant, and the coefficients on Collaterall are negative. Similarly, Afonso et al (2011) conclude that there is little evidence of liquidity hoarding in the US unsecured market during the same period.
The results in Table 6 also suggest a relative pick-up in repo lending by: (i) safer banks with low collateral holdings, and (ii) riskier banks with high collateral holdings (i.e. the significant coefficients in columns (f) and (h)). It is likely that type (i) banks are lending to acquire liquid asset holdings, potentially funded by the pick-up in unsecured borrowing documented in Section 4.1, which would imply an overall net inflow of liquidity. Comparing these results with banks' repo borrowing from the RBA, in open market operations (OMO), sheds some light on the pick-up in repo lending by type (ii) banks. Type (ii) banks increase borrowing from the RBA against AGS and semis (column (n)), and Section 4.1 shows that they also increase interbank repo borrowing. With both sources of incoming funds, it is plausible that the pick-up in lending is to signal their strong liquid asset position to other banks that might otherwise treat them as risky. Afonso et al (2011) speculate similar motives for unexplained patterns in unsecured lending behaviour.
Market: | Unsecured | Repo | Total | AGS/semis collateral | Private collateral | |||||||||||||||||
---|---|---|---|---|---|---|---|---|---|---|---|---|---|---|---|---|---|---|---|---|---|---|
Regressand: |
LoanValueslbd | Participationlbd | LoanValueslbd | Participationlbd | LoanValueslbd | Participationlbd | OMOld | |||||||||||||||
(a) | (b) | (c) | (d) | (e) | (f) | (g) | (h) | (i) | (j) | (k) | (l) | (m) | (n) | (o) | (p) | |||||||
LBd * | ||||||||||||||||||||||
CPRiskl | −0.065 (0.102) |
−0.023 (0.116) |
−0.012 (0.023) |
−0.006 (0.025) |
−0.034 (0.125) |
0.106 (0.112) |
−0.014 (0.039) |
0.017 (0.039) |
−0.055 (0.079) |
0.039 (0.088) |
−0.010 (0.020) |
0.008 (0.022) |
−0.072 (0.577) |
0.435 (0.362) |
1.054** (0.391) |
1.009* (0.524) |
||||||
Collaterall | −0.297** (0.126) |
−0.189 (0.123) |
−0.054** (0.024) |
−0.040 (0.027) |
−0.014 (0.153) |
0.409 (0.276) |
−0.011 (0.057) |
0.082 (0.083) |
−0.135 (0.131) |
0.094 (0.096) |
−0.017 (0.035) |
0.027 (0.028) |
−0.406 (0.549) |
1.031* (0.493) |
1.067* (0.538) |
0.938 (1.018) |
||||||
CPRiskl * Collaterall | 0.162 (0.153) |
0.022 (0.030) |
0.613** (0.279) |
0.136* (0.074) |
0.344* (0.172) |
0.066 (0.041) |
2.319*** (0.619) |
−0.207 (1.026) |
||||||||||||||
Fixed effects | Lender and Borrower * Day | Lender and Day | ||||||||||||||||||||
Observations | 5,360 | 5,360 | 5,360 | 5,360 | 3,060 | 3,060 | 3,060 | 3,060 | 7,240 | 7,240 | 7,240 | 7,240 | 380 | 380 | 380 | 380 | ||||||
R-squared | 0.229 | 0.230 | 0.217 | 0.218 | 0.240 | 0.246 | 0.205 | 0.210 | 0.218 | 0.219 | 0.204 | 0.206 | 0.611 | 0.646 | 0.687 | 0.687 | ||||||
Notes: *, ** and *** denote significance at the 10, 5 and 1 per cent levels, respectively. Columns (a) to (l) contain estimates from regressing loans outstanding, in log millions plus one ( LoanValueslbd ), and an indicator variable for whether LoanValueslbd is positive ( Participationlbd ), on interactions between lender characteristics and market stress. Columns (a) to (d) use the unsecured sample, columns (e) to (h) use the repo sample, and columns (i) to (l) treat the two markets as a single combined market. The pre-interacted explanatory variables are banks' counterparty risk ( CPRiskl ), banks' pre-sample high-quality collateral holdings ( Collaterall ) and an indicator variable equal to one after the Lehman Brothers default ( LBd ). CPRiskl and Collaterall are standardised to zero mean and unit variance. The full variable definitions are in the Section 2.4. Standard errors are clustered at the borrower and lender and day levels. Columns (m) to (p) report regressions using a different dependent variable. The dependent variable is, at the lender-day level, each lender's daily outstanding borrowing of RBA repos, in log AUD millions ( OMOld ), against two separate collateral categories: AGS and semis combined (i.e. government debt securities); and private, which includes everything else. The OMO data excludes RBA repos against discount securities, which, unlike for interbank repos, may have been a non-negligible portion of total RBA repos in the sample (e.g. Kearns 2009). Changes in outstanding RBA repo balances are inferred from transactions between banks and the RBA's OMO account in the Austraclear data. The starting values of these repo positions is pinned down by assuming that the minimum sample value of each bank's outstanding position is AUD 1 million. Standard errors are clustered at the lender and day levels. |
4.4 The Role of Collateral Type in the Repo Market
This section compares how the repo market segments against different collateral types reacted to the market stress surrounding the Lehman Brothers default. Most collateral in the Australian repo market is issued by either the national or state governments (AGS and semis, respectively). Both categories are widely considered to be low risk and are included in the regulatory definition of high-quality liquid assets. Notwithstanding, AGS have higher average credit ratings and are more liquid than semis, related to the fact that the national government is a higher authority than the state governments.
Differences in market behaviour around the Lehman Brothers default are clear from plots of the market sizes and interest rates (Figure 6). The aggregate repo market expansion discussed at the start of Section 4 comprises repos against semis, while the total value of AGS repos remains flat. At the same time, AGS repo spreads (to the cash rate) fall heavily while spreads on repos against semis are relatively stable. Together, these patterns indicate a scarcity of AGS alongside a demand for funding that was satisfied in the semis repo market. Indeed, at the time, the Australian Government had very little debt outstanding, and the face value of semis on issue was greater than that of AGS.
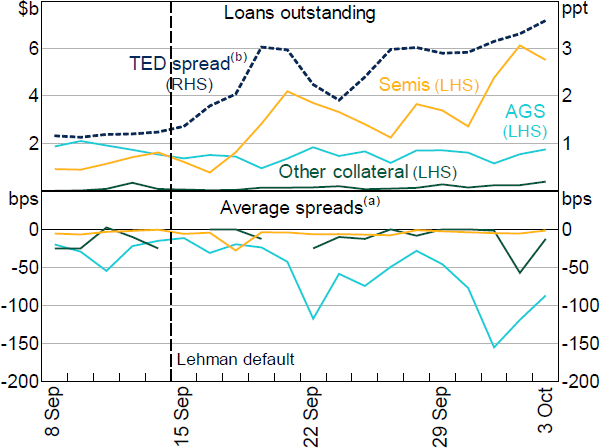
Notes:
(a) Spreads are to the cash rate, measured for loans initiated that day; to construct market-wide average, counterparty pairs are weighted equally and loans within a pair are value weighted
(b) Lagged one day to account for time zones
Sources: ASX; Authors' calculations; Federal Reserve Bank of St. Louis; RBA
The heavy decline in AGS repo rates likely included special repos. Special repos are repos driven by demand for the specific securities received, with the cash side acting as collateral for the loan of securities. They can be difficult to distinguish from other repos (i.e. general collateral, or ‘GC’, repos), both conceptually and observationally in our data.[14] Demand for particular securities can push down interest rates on special repos, particularly when those securities are scarce. Nevertheless, rates on GC AGS repos would also be expected to fall when there is a pick-up in general demand for holding liquid assets. Indeed, Bartolini et al (2011) show that during periods of (non-crisis) heightened liquidity demand in the United States, noticeable spreads opened up between GC Treasury repos and GC agency repos, driven by declining GC Treasury rates. Our data indicate that the decline in AGS repo rates was at least partly driven by GC repos: the largest declines involved AGS ISINs that were relatively frequently used as collateral in the second half of the sample.
To examine the bank-level behaviour within the aggregate volumes, we estimate specification (1) on each of the AGS and semis repo markets, also separating the two types of banks' collateral holdings (i.e. Collateralb is replaced by AGSb and Semisb ). The results show that the risk–collateral interaction reported in Section 4.1 is significant for both collateral types (in their respective markets), but that riskier banks with large holdings of both types of collateral tend to borrow against AGS rather than semis (Table 7). That is, the counterparty risk–semis interaction coefficients increase from around zero only when AGS holdings are controlled for (columns (c), (d), (g) and (h)), while the counterparty risk–AGS interaction coefficients are uniformly significant with and without semis controls (columns (a), (b), (e) and (f)). Similarly, high counterparty-risk borrowers with large AGS holdings shift out of the semis market (columns (g) and (h)), but there is no such shift in the opposite direction (columns (e) and (f)).
The tendency for high counterparty-risk banks to increase borrowing against AGS rather than semis has plausible explanations on both the lender and borrower sides. Lenders may have been less willing to lend to high counterparty-risk borrowers against semis, given that AGS are higher quality. Alternatively, borrowers with high counterparty risk, substituting from the unsecured market (Section 4.2), may have been taking advantage of low interest rates in the AGS market (Figure 6, bottom panel).
To test these potential explanations we re-estimate the regressions in Table 7 with repo rates as a dependent variable. The results (in Appendix B) show that borrowers that substituted into the AGS market were receiving good terms – in the AGS market, the counterparty risk–AGS interaction coefficients are negative and significant at 90 per cent (Table B1). This runs against the supply-side explanation that riskier borrowers that had both types of collateral were pushed to borrow against AGS. Rather, it suggests that riskier borrowers chose to borrow against AGS. That is, the heightened demand for liquid assets eased funding conditions for riskier borrowers, by lowering borrowing rates.
Aside from this, the repo rate regressions mostly produce null results. Overall, counterparty risk does not appear to be a strong determinant of repo rates, consistent with findings of Krishnamurthy et al (2014), and with findings for unsecured loans (discussed in Section 2.1).
Market: | AGS | Semis | AGS | Semis | |||||||
---|---|---|---|---|---|---|---|---|---|---|---|
Regressand: |
LoanValueslbd | Participationlbd | LoanValueslbd | Participationlbd | LoanValueslbd | Participationlbd | LoanValueslbd | Participationlbd | |||
(a) | (b) | (c) | (d) | (e) | (f) | (g) | (h) | ||||
LBd * | |||||||||||
CPRiskb | 0.254* (0.119) |
0.055 (0.040) |
−0.247** (0.093) |
−0.010 (0.023) |
0.295** (0.133) |
0.066 (0.043) |
−0.276* (0.134) |
−0.015 (0.017) |
|||
AGSb | 0.377*** (0.099) |
0.080** (0.031) |
0.326*** (0.104) |
0.054 (0.037) |
−0.298*** (0.058) |
−0.069*** (0.007) |
|||||
CPRiskb * AGSb | 1.084*** (0.189) |
0.220*** (0.058) |
1.044*** (0.199) |
0.187*** (0.057) |
−0.755** (0.256) |
−0.169*** (0.042) |
|||||
Semisb | 0.019 (0.089) |
0.033 (0.026) |
0.135** (0.048) |
0.046 (0.038) |
0.321*** (0.106) |
0.103** (0.037) |
|||||
CPRiskb * Semisb | −0.018 (0.053) |
0.061*** (0.017) |
0.045* (0.025) |
0.041 (0.037) |
0.401*** (0.060) |
0.157*** (0.023) |
|||||
Fixed effects | Borrower and Lender * Day | ||||||||||
Observations | 2,660 | 2,660 | 1,640 | 1,640 | 2,660 | 2,660 | 1,640 | 1,640 | |||
R-squared | 0.273 | 0.242 | 0.264 | 0.236 | 0.274 | 0.242 | 0.269 | 0.240 | |||
Notes: *, ** and *** denote significance at the 10, 5 and 1 per cent levels, respectively. This table reports estimates from lender-borrower-day level regressions separating the repo market by collateral type (using specification (1)). We regress loans outstanding, in log millions plus one ( LoanValueslbd ), and an indicator variable for whether LoanValueslbd is positive ( Participationlbd ), on interactions between borrower characteristics and market stress. Columns (a), (b), (e) and (f) examine repos collateralised by AGS and columns (c), (d), (g) and (h) examine repos collateralised by semis. The pre-interacted explanatory variables are borrowers' counterparty risk ( CPRiskb ), borrowers' pre-sample AGS holdings ( AGSb ), borrowers' pre-sample semis holdings ( Semisb ) and an indicator variable equal to one after the Lehman Brothers default ( LBd ). CPRiskb , AGSb and Semisb are standardised to zero mean and unit variance. The full variable definitions are in Section 2.4. Standard errors are clustered at the borrower and lender and day levels. |
5. Further Robustness
This section reports the results from several additional robustness tests. We first run a set of placebo regressions. These repeat the main parts of the analysis in Section 4 on a 2006 sample, when markets were calm, covering four weeks from the second Monday in September (see Figure 3 for the market aggregates). The placebo regressions produce null results (Table B2). This addresses two potential concerns. First, it shows our results are not driven by patterns in interbank markets that occur on a regular basis, such as time of month, time of quarter, or time of year effects. Second, it shows that our results are not driven by random variability.
To test whether counterparty risk is exogenous to omitted variables, we regress borrowers' NPL on their size, leverage, domicile (an indicator equal to one if the bank is not Australian) and quantity of interbank borrowing in the week prior to our sample. The coefficient on pre-sample borrowing tests whether counterparty risk is endogenous to interbank activity prior to the peak-stress period. Only domicile is statistically significant (Table B3) – its p-value is 0.075, while the next lowest p-value is 0.324, on leverage. To test whether the counterparty risk coefficients in our main regressions are instead picking up domicile effects, we repeat the regressions after replacing counterparty risk with the domicile dummy variable. The borrower results are largely null (Table B4), which further supports our use of NPL as a measure of counterparty risk.
Next, to test the robustness of LBd as a measure of stress, we repeat the main regressions after replacing LBd with the TED spread, lagged one day to account for the time zone difference. The results have similar signs and significance levels (Table B5). Finally, we re-run the main regressions using different standard error clustering levels. The regressions in Section 4 cluster at the borrower and lender and day levels, which tends to make the minimum number of clusters around 15 to 20. To ensure that these are not presenting unconverged estimates, we re-run the regressions such that none have fewer than 30 clusters. For the specification (1) regressions, we cluster at the borrower*lender and borrower*day and lender*day levels simultaneously, and for the specification (2) regressions, we cluster at the lender and borrower levels. The results have similar significance levels (Table B6).
6. Conclusion
The credit contractions that occur during financial crises have lasting adverse consequences. These contractions have been studied in detail (e.g. Schularick and Taylor 2012), and the presence of collateral has been put forward as an important determinant (e.g. Lian and Ma forthcoming). However, the effect of collateral on credit is difficult to identify, in part because retail credit markets tend to have illiquid and idiosyncratic collateral that is correlated with other borrower characteristics. To identify the role of collateral during crises, we focus on Australian interbank markets around the Lehman Brothers default. In this setting, the collateral is liquid and homogenous across borrowers, the shock is large and exogenous (unlike in regions where banks failed), and, with loan-level data, we can control for endogenous borrower-lender matching.
Our regulatory dataset includes loan-level collateralised and uncollateralised interbank borrowing, and the collateral held by each counterparty. With these data, we examine how banks' ex ante risk and collateral-holding characteristics determine how their borrowing reacts to the Lehman Brothers default. We control for endogenous borrower-lender matching by adopting fixed effects at the lender*time level or higher. Our study is also a contribution to the interbank markets literature. As far as we know, we are the first loan-level examination of collateralised and uncollateralised interbank markets that operate side by side without intermediation by a central counterparty.
We show that after the shock, uncollateralised borrowing by ex ante riskier borrowers declines, collateralised borrowing by banks with ex ante more high-quality collateral rises, and riskier borrowers with sufficient high-quality collateral substitute from uncollateralised to collateralised borrowing. We also find evidence of broad-based excess demand for safe collateral, as the repo market collateralised against second-best collateral expands, while rates on the safest collateral fall heavily (over 100 basis points in our sample). Patterns across lenders and collateral types indicate that this demand to hold liquid/safe assets facilitates credit flows, resembling the opposite of cash hoarding. Overall, our estimated effects are statistically and quantitatively significant, and do not appear in placebo regressions using 2006 data (i.e. normal times).
Appendix A: Interbank Market Infrastructure
In Australia the repo and unsecured interbank markets are over-the-counter and settled bilaterally. Counterparties negotiate loans directly with each other, and settle them by transferring cash and securities to each others' accounts in the Australian payments and settlements infrastructure. In the unsecured market, lending takes place via payments between banks' Exchange Settlement Accounts (ESAs) held in RITS, which is the RBA's interbank high-value payments settlement system. All banks licensed to operate in Australia must have an ESA. During 2008 there were around 50 banks with RITS ESAs, comprising Australian banks and subsidiaries and/or branches of foreign banks.
While an unsecured loan takes place through a series of cash payments in RITS, a repo loan resembles a series of securities transactions. In Australia these transactions are mostly settled through Austraclear, Australia's settlement system and central securities depository for debt securities (a subsidiary of the publicly listed company ASX). Austraclear has capability to feed payments instructions into RITS, which permits account holders to instruct Austraclear to simultaneously transfer, in opposite directions, debt securities across Austraclear accounts and cash across RITS accounts. The international regulatory term for this simultaneous transfer – a key protection against settlement risk – is delivery-versus-payment (DvP) settlement. In September 2008, there were roughly 360 active Austraclear accounts belonging to around 180 separate entities. Entities that hold an Austraclear account but not an ESA execute DvP securities settlements by having another bank with an ESA transfer cash through RITS on their behalf.
Appendix B: Additional Regressions and Robustness Tests
Market: | AGS | Semis | AGS | Semis | |||
---|---|---|---|---|---|---|---|
(a) | (b) | (c) | (d) | ||||
LoanValueslbd | 0.138** (0.053) |
0.020*** (0.003) |
0.142** (0.054) |
0.020*** (0.003) |
|||
LBd * | |||||||
CPRiskb | −0.015 (0.089) |
0.008 (0.006) |
0.020 (0.074) |
0.030 (0.026) |
|||
AGSb | −0.130 (0.076) |
−0.210* (0.102) |
−0.075 (0.101) |
||||
CPRiskb * AGSb | −0.197* (0.098) |
−0.343* (0.181) |
−0.081 (0.112) |
||||
Semisb | 0.007 (0.005) |
0.075 (0.109) |
0.090 (0.109) |
||||
CPRiskb * Semisb | 0.007 (0.005) |
0.203 (0.193) |
0.092 (0.113) |
||||
Fixed effects | Borrower and Lender * Day | ||||||
Observations | 744 | 496 | 615 | 342 | |||
R-squared | 0.078 | 0.043 | 0.482 | 0.725 | |||
Notes: *, ** and *** denote significance at the 10, 5 and 1 per cent levels, respectively. This table reports estimates from lender-borrower-day level regressions of repo interest rates, separating the repo market by collateral type (using specification (1)). On days with multiple repos in the same lbd unit, the dependent variable is a loan-size weighted average of the rates. Observations for which there are no loans outstanding are excluded. Columns (a) and (c) examine repos collateralised by AGS and columns (b) and (d) examine repos collateralised by semis. The pre-interacted explanatory variables are borrowers' counterparty risk ( CPRiskb ), borrowers' pre-sample AGS holdings ( AGSb ), borrowers' pre-sample semis holdings ( Semisb ) and an indicator variable equal to one after the Lehman Brothers default ( LBd ). CPRiskb , AGSb and Semisb are standardised to zero mean and unit variance. Loans outstanding, in log millions plus one ( LoanValueslbd ) is included in each regression as a control (as suggested by Garvin (2018)). The full variable definitions are in Section 2.4. Standard errors are clustered at the borrower and lender and day levels. |
Analysis: | Borrower characteristics | Lender characteristics | Borrower substitution | ||||||||||||
---|---|---|---|---|---|---|---|---|---|---|---|---|---|---|---|
Market: | Unsecured | Repo | Unsecured | Repo | Full sample | ||||||||||
Regressand: |
LoanValueslbd | Participationlbd | LoanValueslbd | Participationlbd | LoanValueslbd | Participationlbd | LoanValueslbd | Participationlbd | LoanValueslbdm | Participationlbdm | |||||
(a) | (b) | (c) | (d) | (e) | (f) | (g) | (h) | (i) | (j) | ||||||
LBd * | (1s * explanatory variables) | ||||||||||||||
CPRiski | −0.006 (0.105) |
−0.010 (0.020) |
0.105 (0.339) |
0.073 (0.090) |
0.033 (0.074) |
0.013 (0.020) |
0.136 (0.159) |
0.043 (0.042) |
−0.020 (0.175) |
0.051 (0.030) |
|||||
Collaterali | 0.158 (0.321) |
0.015 (0.068) |
−0.122 (0.609) |
0.100 (0.147) |
0.565 (0.365) |
0.085 (0.080) |
0.921 (0.554) |
0.232 (0.152) |
0.045 (0.067) |
0.027* (0.016) |
|||||
CPRiski * Collaterali | 0.126 (0.301) |
0.006 (0.061) |
0.026 (0.557) |
0.115 (0.139) |
0.830 (0.498) |
0.140 (0.110) |
0.967 (0.682) |
0.211 (0.163) |
0.038 (0.181) |
0.053 (0.043) |
|||||
Lower-level interactions | . | yes | |||||||||||||
Fixed effects | Borrower and Lender * Day | Lender and Borrower * Day | Lender * Borrower * Day | ||||||||||||
Observations | 5,073 | 5,073 | 2,413 | 2,413 | 4,560 | 4,560 | 2,565 | 2,565 | 16,454 | 16,454 | |||||
R-squared | 0.208 | 0.188 | 0.268 | 0.241 | 0.199 | 0.190 | 0.241 | 0.220 | 0.524 | 0.510 | |||||
Notes: *, ** and *** denote significance at the 10, 5 and 1 per cent levels, respectively. This table reports estimates from regressions using the 2006 sample from Monday 11 September to Friday 6 October. The placebo market-stress explanatory variable ( LBd ) is equal to 0 in the first week and 1 afterwards. Columns (a) to (h) report estimates from lender-borrower-day level regressions on sub-samples containing either the unsecured or repo interbank market (i.e. specification (1)), and columns (i) and (j) report lender-borrower-day-market level regressions on the full sample containing both markets (i.e. specification (2)). We regress loans outstanding, in log millions plus one ( LoanValueslbd or LoanValueslbdm ), and an indicator variable for whether LoanValues is positive ( Participationlbd or Participationlbdm ), on interactions between borrower or lender characteristics and market stress. The other pre-interacted explanatory variables are banks' counterparty risk ( CPRiski ), banks' pre-sample high-quality collateral holdings ( Collaterali ) and, in columns (i) and (j), the repo market indicator ( 1s ). CPRiski and Collaterali are standardised to zero mean and unit variance. The full variable definitions are in Section 2.4. Standard errors are clustered at the borrower and lender and day levels. |
Constant | 6.553 (6.444) |
---|---|
Sizeb | 0.132 (0.194) |
Leverageb | −6.585 (6.545) |
Foreignb | 0.867* (0.467) |
FirstWeekBorrowingb | 0.035 (0.070) |
Observations | 30 |
R-squared | 0.367 |
Notes: *, ** and *** denote significance at the 10, 5 and 1 per cent levels, respectively. This table reports estimates from regressing borrower NPL (i.e. CPRiskb ) on other borrower characteristics. Sizeb is the log of balance sheet asset value in AUD trillions plus one; Leverageb is balance sheet liabilities over assets; Foreignb is a dummy for whether the bank's ultimate parent is Australian; and FirstWeekBorrowingb is the sum of the bank's interbank borrowing transactions in the week prior to our sample (1 to 5 September 2008), in AUD millions logged plus one. The variables are not standardised. Standard errors are OLS. |
Analysis: | Borrower characteristics | Lender characteristics | Borrower substitution | ||||||||||||
---|---|---|---|---|---|---|---|---|---|---|---|---|---|---|---|
Market: | Unsecured | Repo | Unsecured | Repo | Full sample | ||||||||||
Regressand: |
LoanValueslbd | Participationlbd | LoanValueslbd | Participationlbd | LoanValueslbd | Participationlbd | LoanValueslbd | Participationlbd | LoanValueslbdm | Participationlbdm | |||||
(a) | (b) | (c) | (d) | (e) | (f) | (g) | (h) | (i) | (j) | ||||||
LBd * | (1s * explanatory variables) | ||||||||||||||
Foreigni | 0.141 (0.271) |
0.004 (0.065) |
0.269 (0.249) |
0.103 (0.070) |
−0.091 (0.176) |
−0.022 (0.039) |
−0.206 (0.240) |
−0.075 (0.087) |
0.132 (0.195) |
0.057 (0.052) |
|||||
Collaterali | 0.325** (0.152) |
0.041 (0.039) |
0.116 (0.116) |
0.030 (0.035) |
−0.294 (0.174) |
−0.055 (0.035) |
−0.341*** (0.088) |
−0.097* (0.049) |
−0.087 (0.083) |
−0.003 (0.026) |
|||||
Foreigni * Collaterali | −0.249 (0.244) |
−0.027 (0.055) |
0.120 (0.191) |
0.035 (0.053) |
0.014 (0.218) |
0.006 (0.044) |
0.805** (0.294) |
0.208** (0.098) |
0.137 (0.110) |
0.028 (0.033) |
|||||
Lower-level interactions | . | . | yes | ||||||||||||
Fixed effects | Borrower and Lender * Day | Lender and Borrower * Day | Lender * Borrower * Day | ||||||||||||
Observations | 5,800 | 5,800 | 3,020 | 3,020 | 5,940 | 5,940 | 3,100 | 3,100 | 15,640 | 15,640 | |||||
R-squared | 0.225 | 0.216 | 0.249 | 0.220 | 0.213 | 0.199 | 0.248 | 0.213 | 0.537 | 0.509 | |||||
Notes: *, ** and *** denote significance at the 10, 5 and 1 per cent levels, respectively. This table reports estimates from specifications that replace banks' counterparty risk with banks' domicile. Columns (a) to (h) report estimates from lender-borrower-day level regressions on sub-samples containing either the unsecured or repo interbank market (i.e. specification (1)), and columns (i) and (j) report lender-borrower-day-market level regressions on the full sample containing both markets (i.e. specification (2)). We regress loans outstanding, in log millions plus one ( LoanValueslbd or LoanValueslbdm ), and an indicator variable for whether LoanValues is positive ( Participationlbd or Participationlbdm ), on interactions between borrower or lender characteristics and market stress. The pre-interacted explanatory variables are market stress ( LBd ), equal to 0 in the first week and 1 afterwards, banks' domicile ( Foreigni ), equal to 0 if the bank's parent company is Australian and 1 otherwise, banks' pre-sample high-quality collateral holdings ( Collaterali ) and, in columns (i) and (j), the repo market indicator ( 1s ). Collaterali is standardised to zero mean and unit variance. The full variable definitions are in Section 2.4. Standard errors are clustered at the borrower and lender and day levels. |
Analysis: | Borrower characteristics | Lender characteristics | Borrower substitution | ||||||||||||||
---|---|---|---|---|---|---|---|---|---|---|---|---|---|---|---|---|---|
Market: | Unsecured | Repo | Unsecured | Repo | Full sample | ||||||||||||
Regressand: |
LoanValueslbd | Participationlbd | LoanValueslbd | Participationlbd | LoanValueslbd | Participationlbd | LoanValueslbd | Participationlbd | LoanValueslbdm | Participationlbdm | |||||||
(a) | (b) | (c) | (d) | (e) | (f) | (g) | (h) | (i) | (j) | ||||||||
TEDd * | (1s * explanatory variables) | ||||||||||||||||
CPRiski | −0.082* (0.039) |
−0.015 (0.009) |
−0.052 (0.057) |
0.003 (0.015) |
0.026 (0.054) |
0.005 (0.011) |
0.030 (0.046) |
0.001 (0.016) |
0.040** (0.018) |
0.015** (0.006) |
|||||||
Collaterali | 0.020 (0.037) |
0.003 (0.008) |
0.136** (0.052) |
0.049*** (0.010) |
−0.051 (0.081) |
−0.010 (0.018) |
0.145 (0.095) |
0.027 (0.029) |
0.029 (0.024) |
0.016** (0.006) |
|||||||
CPRiski * Collaterali | −0.045 (0.049) |
−0.003 (0.012) |
0.136** (0.061) |
0.050*** (0.009) |
0.166* (0.086) |
0.030 (0.018) |
0.223* (0.128) |
0.046 (0.034) |
0.050*** (0.015) |
0.016** (0.006) |
|||||||
Lower-level interactions | . | . | yes | ||||||||||||||
Fixed effects | Borrower and Lender * Day | Lender and Borrower * Day | Lender * Borrower * Day | ||||||||||||||
Observations | 5,780 | 5,780 | 3,020 | 3,020 | 5,360 | 5,360 | 3,060 | 3,060 | 15,600 | 15,600 | |||||||
R-squared | 0.226 | 0.218 | 0.253 | 0.225 | 0.233 | 0.211 | 0.244 | 0.208 | 0.533 | 0.505 | |||||||
Notes: *, ** and *** denote significance at the 10, 5 and 1 per cent levels, respectively. This table reports estimates from specifications that replace the binary market stress measure with the TED spread. Columns (a) to (h) report estimates from lender-borrower-day level regressions on sub-samples containing either the unsecured or repo interbank market (i.e. specification (1)), and columns (i) and (j) report lender-borrower-day-market level regressions on the full sample containing both markets (i.e. specification (2)). We regress loans outstanding, in log millions plus one ( LoanValueslbd or LoanValueslbdm ), and an indicator variable for whether LoanValues is positive ( Participationlbd or Participationlbdm ), on interactions between borrower or lender characteristics and market stress. The pre-interacted explanatory variables are the TED spread ( TEDd ), in percentage points and lagged one day, banks' counterparty risk ( CPRiski ), banks' pre-sample high-quality collateral holdings ( Collaterali ) and, in columns (i) and (j), the repo market indicator ( 1s ). TEDd , CPRiski and Collaterali are standardised to zero mean and unit variance. The full variable definitions are in Section 2.4. Standard errors are clustered at the borrower and lender and day levels. |
Analysis: | Borrower characteristics | Lender characteristics | Borrower substitution | |||||||||||
---|---|---|---|---|---|---|---|---|---|---|---|---|---|---|
Market: | Unsecured | Repo | Unsecured | Repo | Full sample | |||||||||
Regressand: |
LoanValueslbd | Participationlbd | LoanValueslbd | Participationlbd | LoanValueslbd | Participationlbd | LoanValueslbd | Participationlbd | LoanValueslbdm | Participationlbdm | ||||
(a) | (b) | (c) | (d) | (e) | (f) | (g) | (h) | (i) | (j) | |||||
LBd * | (1s * explanatory variables) | |||||||||||||
CPRiski | −0.247** (0.101) |
−0.049** (0.021) |
−0.019 (0.127) |
0.023 (0.033) |
−0.023 (0.100) |
−0.006 (0.021) |
0.106 (0.118) |
0.017 (0.034) |
0.166** (0.066) |
0.047** (0.019) |
||||
Collaterali | 0.019 (0.098) |
0.001 (0.022) |
0.267** (0.119) |
0.084*** (0.032) |
−0.189 (0.136) |
−0.040 (0.030) |
0.409** (0.192) |
0.082 (0.055) |
0.061 (0.089) |
0.029 (0.019) |
||||
CPRiski * Collaterali | −0.151 (0.131) |
−0.021 (0.028) |
0.358** (0.156) |
0.117*** (0.038) |
0.162 (0.147) |
0.022 (0.032) |
0.613*** (0.230) |
0.136** (0.058) |
0.151** (0.069) |
0.043** (0.016) |
||||
Lower-level interactions | . | . | yes | |||||||||||
Fixed effects | Borrower and Lender * Day | Lender and Borrower * Day | Lender * Borrower * Day | |||||||||||
Observations | 5,780 | 5,780 | 3,020 | 3,020 | 5,360 | 5,360 | 3,060 | 3,060 | 15,600 | 15,600 | ||||
R-squared | 0.227 | 0.219 | 0.251 | 0.222 | 0.230 | 0.218 | 0.246 | 0.210 | 0.533 | 0.505 | ||||
Notes: *, ** and *** denote significance at the 10, 5 and 1 per cent levels, respectively. This table reports estimates from specifications that use different standard error clustering. Columns (a) to (h) cluster at the borrower * lender, borrower * day and lender * day levels, and columns (i) and (j) cluster at the borrower and lender levels. Columns (a) to (h) report estimates from lender-borrower-day level regressions on sub-samples containing either the unsecured or repo interbank market (i.e. specification (1)), and columns (i) and (j) report lender-borrower-day-market level regressions on the full sample containing both markets (i.e. specification (2)). We regress loans outstanding, in log millions plus one ( LoanValueslbd or LoanValueslbdm ), and an indicator variable for whether LoanValues is positive ( Participationlbd or Participationlbdm ), on interactions between borrower or lender characteristics and market stress. The pre-interacted explanatory variables are market stress ( LBd ), equal to 0 in the first week and 1 afterwards, banks' counterparty risk ( CPRiski ), banks' pre-sample high-quality collateral holdings ( Collaterali ) and, in columns (i) and (j), the repo market indicator ( 1s ). CPRiski and Collaterali are standardised to zero mean and unit variance. The full variable definitions are in Section 2.4. |
References
Abbassi P, F Bräuning, F Fecht and J-L Peydró (2014), ‘Cross-Border Liquidity, Relationships and Monetary Policy: Evidence from the Euro Area Interbank Crisis’, Deutsche Bundesbank Discussion Paper No 45/2014.
Acharya VV and D Skeie (2011), ‘A Model of Liquidity Hoarding and Term Premia in Inter-Bank Markets’, Journal of Monetary Economics, 58(5), pp 436–447.
Adelino M, A Schoar and F Severino (2015), ‘House Prices, Collateral, and Self-Employment’, Journal of Financial Economics, 117(2), pp 288–306.
Adrian T, B Begalle, A Copeland and A Martin (2014), ‘Repo and Securities Lending’, in M Brunnermeier and A Krishnamurthy (eds), Risk Topography: Systemic Risk and Macro Modeling, National Bureau of Economic Research Conference Report, University of Chicago Press, Chicago, pp 131–148.
Afonso G, A Kovner and A Schoar (2011), ‘Stressed, Not Frozen: The Federal Funds Market in the Financial Crisis’, The Journal of Finance, 66(4), pp 1109–1139.
Allen F, E Carletti and D Gale (2009), ‘Interbank Market Liquidity and Central Bank Intervention’, Journal of Monetary Economics, 56(5), pp 639–652.
Allen F and D Gale (2000), ‘Financial Contagion’, Journal of Political Economy, 108(1), pp 1–33.
Allen F and D Gale (2009), Understanding Financial Crises, Paperback edn, Clarendon Lectures in Finance, Oxford University Press, Oxford.
Angelini P, A Nobili and C Picillo (2011), ‘The Interbank Market after August 2007: What Has Changed, and Why?’, Journal of Money, Credit and Banking, 43(5), pp 923–958.
Bartolini L, S Hilton, S Sundaresan and C Tonetti (2011), ‘Collateral Values by Asset Class: Evidence from Primary Securities Dealers’, The Review of Financial Studies, 24(1), pp 248–278.
Becker C and P Rickards (2017), ‘Secured Money Market Transactions: Trends in the Australian Repo Rate’, JASSA The Finsia Journal of Applied Finance, 2017(1), pp 13–21.
Benmelech E and NK Bergman (2011), ‘Bankruptcy and the Collateral Channel’, The Journal of Finance, 66(2), pp 337–378.
Benmelech E, N Kumar and R Rajan (2020a), ‘The Decline of Secured Debt’, NBER Working Paper No 26637.
Benmelech E, N Kumar and R Rajan (2020b), ‘Secured Credit Spread’, NBER Working Paper No 26799.
Berger AN, WS Frame and V Ioannidou (2016), ‘Reexamining the Empirical Relation between Loan Risk and Collateral: The Roles of Collateral Liquidity and Types’, Journal of Financial Intermediation, 26, pp 28–46.
Bernanke BS, M Gertler and S Gilchrist (1996), ‘The Financial Accelerator and the Flight to Quality’, The Review of Economics and Statistics, 78(1), pp 1–15.
Bernanke BS and CS Lown (1991), ‘The Credit Crunch’, Brookings Papers on Economic Activity, 1991(2), pp 205–239, 247.
Bolton P, X Freixas and J Shapiro (2012), ‘The Credit Ratings Game’, The Journal of Finance, 67(1), pp 85–111.
Brassil A, H Hughson and M McManus (2016), ‘Identifying Interbank Loans from Payments Data’, RBA Research Discussion Paper No 2016-11.
Brassil A and G Nodari (2018), ‘A Density-Based Estimator of Core/Periphery Network Structures: Analysing the Australian Interbank Market’, RBA Research Discussion Paper No 2018-01.
Caballero RJ, E Farhi and P-O Gourinchas (2016), ‘Safe Asset Scarcity and Aggregate Demand’, The American Economic Review, 106(5), pp 513–518.
Caballero RJ, E Farhi and P-O Gourinchas (2017), ‘The Safe Assets Shortage Conundrum’, Journal of Economic Perspectives, 31(3), pp 29–46.
Campello M and M Larrain (2016), ‘Enlarging the Contracting Space: Collateral Menus, Access to Credit, and Economic Activity’, The Review of Financial Studies, 29(2), pp 349–383.
Chaney T, D Sraer and D Thesmar (2012), ‘The Collateral Channel: How Real Estate Shocks Affect Corporate Investment’, The American Economic Review, 102(6), pp 2381–2409.
Chava S and A Purnanandam (2011), ‘The Effect of Banking Crisis on Bank-Dependent Borrowers’, Journal of Financial Economics, 99(1), pp 116–135.
Chava S and MR Roberts (2008), ‘How Does Financing Impact Investment? The Role of Debt Covenants’, The Journal of Finance, 63(5), pp 2085–2121.
Chodorow-Reich G (2014), ‘The Employment Effects of Credit Market Disruptions: Firm-Level Evidence from the 2008–9 Financial Crisis’, The Quarterly Journal of Economics, 129(1), pp 1–59.
Cocco JF, FJ Gomes and NC Martins (2009), ‘Lending Relationships in the Interbank Market’, Journal of Financial Intermediation, 18(1), pp 24–48.
Copeland A, A Martin and M Walker (2014), ‘Repo Runs: Evidence from the Tri-Party Repo Market’, The Journal of Finance, 69(6), pp 2343–2380.
Dang TV, G Gorton and B Holmström (2015), ‘The Information Sensitivity of a Security’, Unpublished manuscript, Yale University, March.
Dang TV, G Gorton, B Holmström and G Ordoñez (2017), ‘Banks as Secret Keepers’, The American Economic Review, 107(4), pp 1005–1029.
Davis K (2011), ‘The Australian Financial System in the 2000s: Dodging the Bullet’, in H Gerard and J Kearns (eds), The Australian Economy in the 2000s, Proceedings of a Conference, Reserve Bank of Australia, Sydney, pp 301–348.
Debelle G (2007), ‘Open Market Operations and Domestic Securities’, Address to Australia Securitisation Forum, Sydney, 29 November.
De Fiore F, M Hoerova and H Uhlig (2018), ‘Money Markets, Collateral and Monetary Policy’, NBER Working Paper No 25319.
De Jonghe O, H Dewachter, K Mulier, S Ongena and G Schepens (2020), ‘Some Borrowers Are More Equal Than Others: Bank Funding Shocks and Credit Reallocation’, Review of Finance, 24(1), pp 1–43.
di Filippo M, A Ranaldo and J Wrampelmeyer (2018), ‘Unsecured and Secured Funding’, Unpublished manuscript, April. Available at <https://papers.ssrn.com/sol3/papers.cfm?abstract_id=2822345>.
Foley-Fisher N, B Narajabad and S Verani (2016), ‘Securities Lending as Wholesale Funding: Evidence from the U.S. Life Insurance Industry’, NBER Working Paper No 22774.
Freixas X and C Holthausen (2005), ‘Interbank Market Integration under Asymmetric Information’, The Review of Financial Studies, 18(2), pp 459–490.
Freixas X, A Martin and D Skeie (2011), ‘Bank Liquidity, Interbank Markets, and Monetary Policy’, The Review of Financial Studies, 24(8), pp 2656–2692.
Freixas X and J-C Rochet (2008), Microeconomics of Banking, 2nd edn, MIT Press, Cambridge.
Furfine CH (1999), ‘The Microstructure of the Federal Funds Market’, Financial Markets, Institutions & Instruments, 8(5), pp 24–44.
Gale D and T Yorulmazer (2013), ‘Liquidity Hoarding’, Theoretical Economics, 8(2), pp 291–324.
Garvin N (2018), ‘Identifying Repo Market Microstructure from Securities Transactions Data’, RBA Research Discussion Paper No 2018-09.
Goldstein I and A Pauzner (2005), ‘Demand–Deposit Contracts and the Probability of Bank Runs’, The Journal of Finance, 60(3), pp 1293–1327.
Gorton G and A Metrick (2012), ‘Securitized Banking and the Run on Repo’, Journal of Financial Economics, 104(3), pp 425–451.
Gorton G and G Ordoñez (2014), ‘Collateral Crises’, The American Economic Review, 104(2), pp 343–378.
Hing A, G Kelly and D Olivan (2016), ‘The Cash Market’, RBA Bulletin, December, pp 33–41.
Ivashina V, L Laeven and E Moral-Benito (2020), ‘Loan Types and the Bank Lending Channel’, NBER Working Paper No 27056.
Ivashina V and D Scharfstein (2010), ‘Bank Lending during the Financial Crisis of 2008’, Journal of Financial Economics, 97(3), pp 319–338.
Jaremski M and DC Wheelock (2020), ‘The Founding of the Federal Reserve, the Great Depression and the Evolution of the U.S. Interbank Network’, The Journal of Economic History, 80(1), pp 69–99.
Jiménez G, S Ongena, J-L Peydró and J Saurina (2014), ‘Hazardous Times for Monetary Policy: What Do Twenty- Three Million Bank Loans Say about the Effects of Monetary Policy on Credit Risk- Taking?’, Econometrica, 82(2), pp 463–505.
Jiménez G, S Ongena, J-L Peydró and J Saurina (2017), ‘Macroprudential Policy, Countercyclical Bank Capital Buffers, and Credit Supply: Evidence from the Spanish Dynamic Provisioning Experiments’, Journal of Political Economy, 125(6), pp 2126–2177.
Kashyap AK and JC Stein (2000), ‘What Do a Million Observations on Banks Say about the Transmission of Monetary Policy?’, The American Economic Review, 90(3), pp 407–428.
Kearns J (2009), ‘The Australian Money Market in a Global Crisis’, RBA Bulletin, June, pp 15–27.
Kent C (2020), ‘The Stance of Monetary Policy in a World of Numerous Tools’, Keynote speech to the IFR Asia Australia Debt Capital Markets Roundtable 2020, Online, 20 October.
Khwaja AI and A Mian (2008), ‘Tracing the Impact of Bank Liquidity Shocks: Evidence from an Emerging Market’, The American Economic Review, 98(4), pp 1413–1442.
Kiyotaki N and J Moore (1997), ‘Credit Cycles’, Journal of Political Economy, 105(2), pp 211–248.
Krishnamurthy A, S Nagel and D Orlov (2014), ‘Sizing up Repo’, The Journal of Finance, 69(6), pp 2381–2417.
Lian C and Y Ma (forthcoming), ‘Anatomy of Corporate Borrowing Constraints’, The Quarterly Journal of Economics.
Liberti JM and J Sturgess (2014), ‘Uncovering Collateral Constraints’, Unpublished manuscript, February. Available at <https://papers.ssrn.com/sol3/papers.cfm?abstract_id=2407959>.
Mancini L, A Ranaldo and J Wrampelmeyer (2016), ‘The Euro Interbank Repo Market’, The Review of Financial Studies, 29(7), pp 1747–1779.
Martin A, D Skeie and E-L von Thadden (2014), ‘Repo Runs’, The Review of Financial Studies, 27(4), pp 957–989.
Miller DL, N Shenhav and MZ Grosz (2019), ‘Selection into Identification in Fixed Effects Models, with Application to Head Start’, NBER Working Paper No 26174.
Morgan DP (2002), ‘Rating Banks: Risk and Uncertainty in an Opaque Industry’, The American Economic Review, 92(4), pp 874–888.
Oster E (2019), ‘Unobservable Selection and Coefficient Stability: Theory and Evidence’, Journal of Business & Economic Statistics, 37(2), pp 187–204.
Piquard T and D Salakhova (2019), ‘Secured and Unsecured Interbank Markets: Monetary Policy, Substitution and the Cost of Collateral’, Banque de France Working Paper Series No 730.
Rampini AA and S Viswanathan (2010), ‘Collateral, Risk Management, and the Distribution of Debt Capacity’, The Journal of Finance, 65(6), pp 2293–2322.
Roberts MR and TM Whited (2013), ‘Endogeneity in Empirical Corporate Finance’, in GM Constantinides, M Harris and RM Stulz (eds), Handbook of the Economics of Finance: Volume 2A Corporate Finance, Handbooks in Economics, Elsevier, Amsterdam, pp 493–572.
Rochet J-C and J Tirole (1996), ‘Interbank Lending and Systemic Risk’, Journal of Money, Credit and Banking, 28(4, Part 2), pp 733–762.
Schularick M and AM Taylor (2012), ‘Credit Booms Gone Bust: Monetary Policy, Leverage Cycles, and Financial Crises, 1870–2008’, The American Economic Review, 102(2), pp 1029–1061.
Acknowledgements
We would like to thank Chris Becker, Anthony Brassil, Falko Fecht, Xavier Freixas, Fred Malherbe, John Simon and seminar participants at the RBA, Universitat Pompeu Fabra, University of New South Wales, University of Sydney and University of Technology Sydney for helpful comments and feedback. We would also like to thank Mark Manning and ASX staff Tim Hogben and Wayne Jordan for help with the data. This paper is not intended to represent the views of the RBA. Any errors are our own.
Footnotes
The TED spread is between the 3-month LIBOR based on USD and the 3-month US Treasury bill rate. [1]
In Section 5 we assess the relevance of domicile to our research question. [2]
Discount securities issued by non-government entities – that is, private securities without coupon payments – are the only debt securities not present in the datasets. Prudential data indicate these could comprise up to a quarter of the interbank repo market, but the RBA's liaison with financial institutions suggests they are much less. The transaction dataset would also miss transactions that take place within a single Austraclear account (and therefore require no movement of securities). Garvin (2018) discusses the prevalence of such transactions, which include any repo market segment transacted through foreign infrastructure. [3]
Specifically, this is the widest interest rate range at which repos are detected with the following characteristics: the implied interest rate is the same when rounded to four and five decimal places; there are at least two other detected repos at the same rate (rounded to four decimal places); and the repo is not between two ‘client’ designated accounts. [4]
Accounts are assumed to be client accounts if the words ‘nominee’, ‘custodian’ or ‘client’, or any abbreviation of these, appear in the account name. Where multiple client accounts are owned by a single organisation, those client accounts are grouped into a single ‘client’ entity. [5]
Specifically, for each International Securities Identification Number (ISIN) – the lowest level at which securities are identifiable – we take the minimum of the face value held at 1 September and at 8 September, and then sum these minima across all AGS and semis ISINs held by that bank (or for AGSi or Semisi , only the bank's AGS or semis holdings). [6]
That is, there are N observations for that borrower in that market each day, and x is the change in probability of each observation being one rather than zero. [7]
Behind these aggregates, the repo market expansion mostly reflects borrowing by a subset of entities, although repo activity by other entities also picks up by a similar proportion. Aside from the aggregate plots of outstanding loans, all the analysis in this paper maintains representativeness across entities. [8]
The subtracted means are bank and market specific, across days. [9]
Most of the fitted lines become steeper when outliers are removed. [10]
They find this result for the large banks in their sample. Most of our sample comprises the largest Australian banks and prominent international banks, so it is more comparable to their large-bank sub-sample than their small-bank sub-sample. [11]
Pairs of banks require a bilateral Global Master Repurchase Agreement (GMRA) in place before they can engage in repo with each other. The extensive margin change that we find is most likely between counterparty pairs that have pre-existing GMRAs, but that prior to the shock do not regularly engage each other, and therefore may not have up-to-date credit risk beliefs about each other. [12]
The unreported specification with borrow-lender, borrower-day and lender-day fixed effects explains 24 to 27 per cent of the variation in the dependent variables. [13]
Australian haircut data demonstrate the conceptual difficulty in distinguishing special and GC repos. In the United States, the amount of cash in a special repo (also called a securities loan) typically exceeds the value of the securities (Foley-Fisher, Narajabad and Verani 2016). This reflects that the loan is driven by demand from the securities receiver, so the securities receiver overcollateralises the securities provider (whereas in a GC repo the cash receiver overcollateralises the cash provider). To an onlooker who thought it was a GC repo, this would appear as a negative haircut. Garvin (2018) shows that haircuts on Australian repos tend to be continuously and randomly distributed around zero, with no clear gap between positive and negative haircuts. [14]