Research Discussion Paper – RDP 2020-08 Start Spreading the News: News Sentiment and Economic Activity in Australia
December 2020
1. News, Sentiment and the Economy
Well, all I know is what I read in the Papers (American humourist, Will Rogers, The New York Times, 30 September 1923)
The large and immediate effect of the COVID-19 pandemic on consumer confidence, business sentiment and economic activity has highlighted the need for policymakers to develop better real-time indicators of the economy and to deepen their understanding of the links between the news, sentiment and economic activity.
In the popular press and the business community, there is a belief that sentiment (or ‘confidence’) has an important causal role in explaining changes in economic activity. But applied research on the topic generally finds that economic activity explains sentiment rather than vice versa (Roberts and Simon 2001). Some research concludes that sentiment is important for both forecasting and explaining business cycles, while other papers argue that it contains important information but has little causal effect, and some suggest it has no value even in forecasting (Barsky and Sims 2012).
To investigate these issues in more depth we develop a new real-time indicator for the Australian economy based on text analysis of news articles. A ‘news sentiment index’ (NSI) is constructed that measures the net balance of positive and negative words used by journalists in news articles about the economy. This indicator can be used to track economic conditions on a daily basis (Shapiro, Sudhof and Wilson 2017; Larsen and Thorsrud 2018). This new economic indicator allows us to explore the role of sentiment in the Australian business cycle with much greater precision and along more dimensions than previous work.
First, we demonstrate that the NSI is an important addition to the suite of real-time indicators of the economy. The NSI complements other high-frequency economic indicators in its ability to ‘nowcast’ the economy. But the NSI is timelier than almost all other indicators because it can be updated on a daily basis. This gives it a clear advantage in being able to pick turning points in the economy, which is important from a policy perspective.
Second, we provide some evidence of a causal role for news sentiment in driving survey-based measures of sentiment and, to a lesser extent, economic activity more generally. Not only is the NSI a very timely indicator, but changes in news-based sentiment precede movements in survey-based sentiment measures. This may be because, in making decisions, consumers and business managers rely on high-frequency information that is broadcast through the news media. On top of this, the NSI can predict changes in business conditions in the near future over and above survey-based sentiment indicators and measures of current business conditions (based on both local projection models and vector autoregressions). To the extent that the news media captures a broad audience the information could be more representative than survey data provided by professional data suppliers (Thorsrud 2020). But arguing against a causal interpretation, we find that the size of the effect of news sentiment on economic activity is small. We also do not find that changes in news sentiment can explain broad indicators of business conditions, such as GDP growth. This suggests the NSI provides limited information content at a lower (quarterly) frequency.
Third, we show that it is important to disentangle sentiment and uncertainty when trying to explain short-term fluctuations in economic activity.[1] There is now a very large literature exploring the links between uncertainty and the business cycle (e.g. Bloom 2014; Moore 2017; Shapiro et al 2017). But few studies look at both sentiment and uncertainty within the same framework. Along with the NSI, we construct a related measure of news uncertainty. We show that changes in news sentiment have larger and more persistent correlations with measures of economic activity than changes in news uncertainty. This may be because news sentiment is a better indicator of current economic conditions than news uncertainty.
Fourth, we construct an indicator capturing sentiment in news specifically relevant to monetary policy. This news indicator predicts changes in the cash rate even after accounting for other important determinants of the cash rate, such as the RBA's forecasts for the economy. One potential explanation is that the news contains useful information about the risks to the economic outlook to which the RBA is responding when setting interest rates (e.g. Sharpe, Sinha and Hollrah 2017). Alternatively, it may be that the forecasts are biased in some way. Either way, the evidence suggests that changes in news sentiment do not cause changes in monetary policy, but that the relationship is due to an omitted variable.
Finally, we provide evidence that monetary policy causes changes in sentiment in ways consistent with standard models. We identify the causal effect of changes in interest rates on news sentiment by conducting an event study in the days before and after monetary policy announcements. Standard models would predict that lowering interest rates should boost the outlook for the economy and hence be associated with increases in sentiment (Nakamura and Steinsson 2018a). Prior to the COVID-19 pandemic though, there were numerous media reports that falling interest rates were causing weaker sentiment amongst business and consumers (Kirchner 2020b). In line with standard models, however, we find that increases (decreases) in interest rates are generally associated with weaker (stronger) news sentiment. This is consistent with the findings of earlier studies that rely on lower-frequency measures of sentiment, which also found that lower interest rates led to higher sentiment on average (Roberts and Simon 2001).
One limitation of our analysis is that we cannot clearly disentangle the effect of shocks to news (fundamentals) from those of sentiment or ‘animal spirits’ (non-fundamentals) on the economy. Future research may be able to separate these two shocks through different types of news articles.
2. How Do We Measure News Sentiment?
Sentiment is hard to measure as it is not directly observed. Common survey-based measures of sentiment typically ask respondents about their beliefs about current economic conditions as well as expectations for future economic conditions. We take a different approach and construct a proxy for sentiment based on the language used by journalists in news reports on the economy.
There are two general approaches for quantifying sentiment in text. The dictionary-based approach relies on pre-defined lists of words with each word either classified as positive, negative, neutral, or indicating uncertainty. The machine learning approach predicts the sentiment of any given set of text after training models with a large set of text that has been assigned sentiment ratings by human readers. For example, models have been developed using social media data, such as Twitter, that provide text that is combined with user feedback to identify the sentiment of the posts. This approach is better able to capture the nuances in human language but it is more complex and less transparent.
We follow the simpler dictionary-based approach to construct our NSI. The NSI measures the net balance of words used by journalists that are considered to be ‘positive’ and ‘negative’. When journalists use more positive words and/or fewer negative words, this is an indicator that sentiment is rising in the economy. This type of index has been used before for other regions, such as the United States, Japan and Europe (see, for instance, Fraiberger (2016); Scotti (2016); Larsen and Thorsrud (2018) and Buckman et al (2020)).
The raw data used in constructing the NSI consist of daily news extracted from Dow Jones Factiva. Each article listed in the database includes metadata such as publication time, language, region and category. After removing duplicates and selecting only articles that are written in English by Australian media outlets to cover the Australian economy, the resulting dataset includes around 300,000 articles. The data span the period from September 1987 to June 2020 and the sample covers more than 600 newspapers, though The Australian, The Sydney Morning Herald and The Australian Financial Review are the main sources.
Common steps in the natural language processing literature are taken to clean the raw dataset before analysis: numbers, punctuation marks, white spaces and common stop words are removed from each article. All words are then reduced to their respective ‘stem’, which is the part of a word that is common to all of its inflections (for example, ‘performs’, ‘performing’, and ‘performed’ are reduced to ‘perform’).
To measure the sentiment of a set of text, that is, whether or not the news is positive or negative, the Loughran–McDonald dictionary is used. This is a word list specific to the domain of economics and finance (see Loughran and McDonald (2011) for more details). The NSI is constructed by counting the number of times that negative and positive words appear in the cleaned text of articles.[2] A news uncertainty index (NUI) is also constructed by counting the number of articles that contain uncertain words.[3] The most common positive, negative and uncertain words in March 2020 are shown in Figure 1.
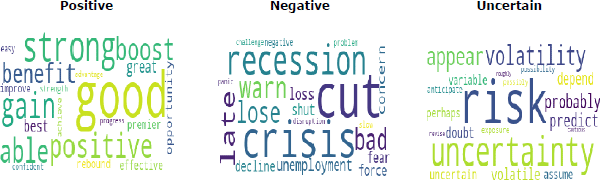
Sources: Authors' calculations; Dow Jones Factiva
To construct the time series of the NSI, the articles are sorted by date of publication and the data are divided into blocks of time, which could be a day. For each time period (t), we compute the sentiment index by subtracting the count of negative words from the count of positive words and then dividing by total word count:
Between September 1987 and March 2020 there are, on average, around two more negatives than positives for every 100 words in the articles, with a standard deviation of less than 1 word. We standardise the indicator to have a mean of zero and a standard deviation of one.
3. Is the News Sentiment Index Useful for Nowcasting?
The news sentiment index clearly moves with fluctuations in economic conditions at a monthly frequency, with sharp declines in economic downturns, such as the early 1990s recession, the 2008–09 global financial crisis (GFC) and the pandemic-led contraction more recently (Figure 2).
The NSI can also be constructed on a daily basis. Because of this, the NSI has the potential to identify turning points in economic activity before other partial indicators, such as survey-based sentiment measures that are available on a monthly basis. For example, based on a (one-sided) 30-day moving average, the NSI was pointing to a sharp drop-off in activity caused by the COVID-19 pandemic in early March, well before the public release of the business survey results at the end of March (Figure 3, bottom panel). Similarly, the one-sided 30-day moving average of the NSI appeared to pick the turning point in economic activity in February 2009, before there were clear signs of a recovery in sentiment based on the business surveys (Figure 3, top panel).[4]
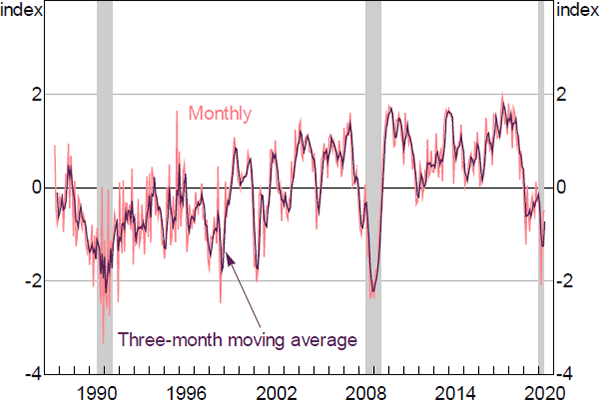
Note: Shaded areas represent the early 1990s recession, the 2008–09 global financial crisis and the COVID-19-led contraction
Sources: Authors' calculations; Dow Jones Factiva
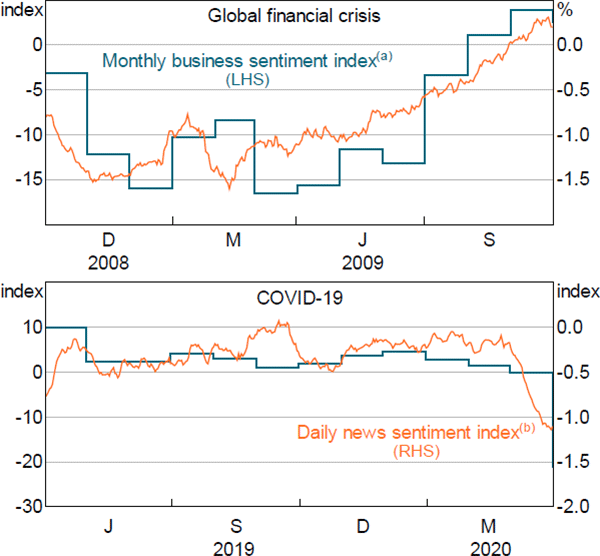
Notes:
(a) Based on NAB business conditions survey
(b) Thirty-day moving average
Sources: Authors' calculations; Dow Jones Factiva; NAB
4. News Sentiment versus Uncertainty
A measure of news uncertainty can also be constructed based on high-frequency information contained in news articles. The NUI is constructed in a slightly different fashion to the NSI. The NUI is estimated by calculating the share of articles that express uncertainty (through terms such as ‘risk’, ‘uncertainty’ and ‘volatility’), rather than the share of words that express uncertainty. This is due to the more limited number of times that words expressing uncertainty appear in news articles.
In March 2020, the NUI rose to a record high level indicating that the pandemic caused more economic uncertainty among Australian households and businesses than during the GFC (Figure 4). The NUI also strongly correlates with publicly available measures of economic uncertainty, such as the Economic Policy Uncertainty Index developed by Baker, Bloom and Davis (2016). Both uncertainty indicators have eased since April.
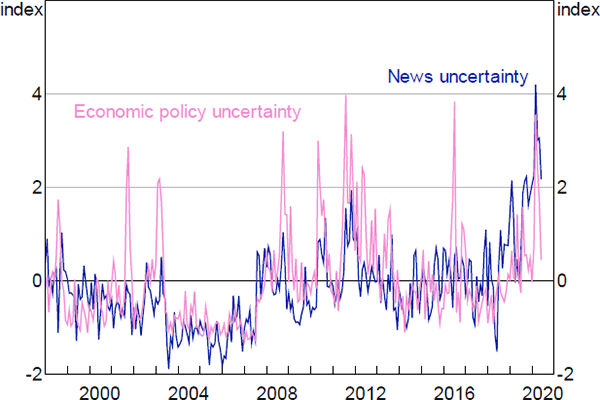
Sources: Authors' calculations; Baker, Bloom and Davis (2016) at www.PolicyUncertainty.com; Dow Jones Factiva
5. News Sentiment and the Economy
5.1 Identification
To identify the effect of changes in news sentiment on both consumer and business sentiment as well as other economic activity indicators, we loosely follow the model of Barsky and Sims (2012). In particular, we estimate impulse response functions using the local projections (LP) method of Jordà (2005). This is similar to the standard vector autoregression (VAR) approach but less restrictive. However, the non-parametric nature of LP comes at an efficiency cost and may suffer from excessive variability. Thus, we also estimate impulse responses using the B-splines smooth local projections (SLP) method proposed by Barnichon and Brownless (2019), which aims at striking a balance between the VAR and LP approaches. The SLP method preserves the flexibility of LP while offering efficiency gains by imposing that impulse responses are polynomial functions of the forecast horizons.
In effect, the identification strategy is the same as estimating a VAR with economic activity, consumer sentiment and news sentiment to consider the partial derivatives of the activity indicators at various horizons with respect to innovations in the news sentiment indicator. The result coincides with that of a recursive system with the news sentiment variable ordered last (Plagborg-Møller and Wolf 2019). In this setting, we define a temporary ‘shock’ as the change in news sentiment that is orthogonal to current and lags of all other variables in the regression. We are not able to distinguish shocks to ‘news’ (or fundamentals such as productivity) from shocks to ‘sentiment’ (or non-fundamental ‘animal spirits’).
Specifically, for each forecast horizon, h, we run a regression of the relevant activity indicator, , on contemporaneous and lagged values of all relevant economic variables including itself, Yt, and of the NSI:
where the lag length, Q, is chosen by a combination of information selection criteria. The impulse responses of the economic variable to a change in news sentiment are traced out by the estimates of the s in Equation (1). To construct the confidence bands, we adopt the Barnichon and Brownlees (2019) heuristic procedure and estimate the asymptotic variance of the B-splines parameters using the Newey-West estimator.
5.2 Response of Survey-based Sentiment to a News Sentiment Shock
Survey-based measures of sentiment are closely monitored by economic analysts and policymakers as timely indicators of economic activity (Hüfner and Schröder 2002; Aylmer and Gill 2003). Figure 5 plots the NSI along with the ANZ–Roy Morgan consumer sentiment index (CSI) over time, where both series are normalised to have a mean of zero and a standard deviation of one. The two series have a correlation coefficient of 0.52 over the full sample, and the correlation is only slightly weaker when economic downturns are removed from the sample.
We also show that news sentiment appears to lead the survey-based consumer sentiment measure by estimating Equation (1) where yi is the consumer sentiment index. We choose four lags for the weekly model and two lags for the monthly model, as suggested by a combination of information selection criteria. We find that a temporary one standard deviation increase in the weekly NSI (7 net positive words per 1,000 words) is associated with 3 to 4 per cent of a standard deviation increase in the CSI (roughly half an index point) in the following few weeks (Figure 6). Similarly, a one standard deviation increase in the monthly NSI is associated with 3 to 5 per cent of a standard deviation increase in the CSI over a similar timeframe. The responses get weaker as the horizon lengthens and become statistically insignificant after roughly 12 periods. So we find that the NSI can predict movements in consumer sentiment, though the size of the effect is small, which argues against a causal interpretation.
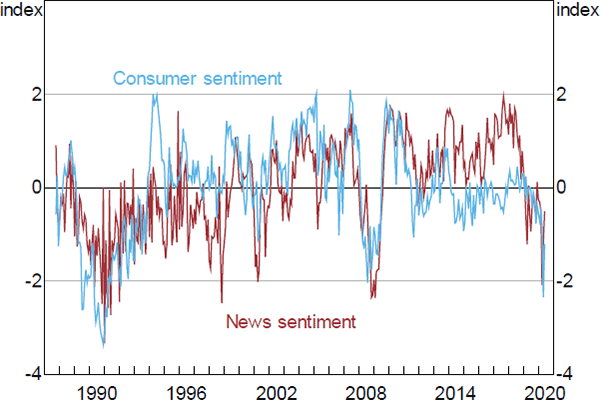
Sources: ANZ–Roy Morgan; Authors' calculations; Dow Jones Factiva
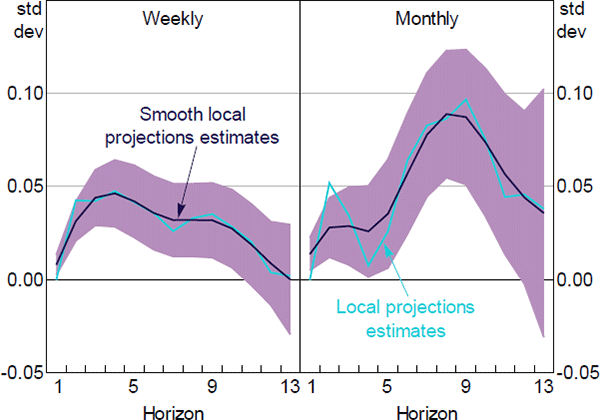
Note: Shaded areas are the 90 per cent confidence intervals for the smooth local projections estimates
Sources: ANZ–Roy Morgan; Authors' calculations; Dow Jones Factiva
5.3 Response of Economic Activity to News Sentiment Shock
To evaluate the effect of changes in news sentiment on economic activity, we estimate Equation (1) on a monthly basis with two lags as chosen by a combination of information selection criteria. The activity indicators included are the NAB measure of current business conditions (to capture overall economic activity), the NAB measure of capital spending (to proxy for business investment) and the change in the unemployment rate (to capture labour market conditions).[5] We also include the NAB survey-based measure of current business conditions as a control variable.[6]
Figure 7 presents the impulse responses of the monthly indicators to a temporary news sentiment shock, which are traced out by the estimates of the s in Equation (1). The results indicate that a temporary increase in news sentiment is associated with higher sentiment about business conditions, higher capital spending and a lower change in the unemployment rate.
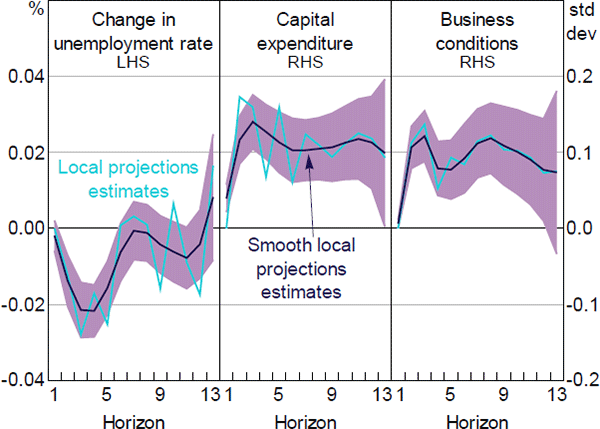
Note: Shaded areas are the 90 per cent confidence intervals for the smooth local projection estimates
Sources: Authors' calculations; Dow Jones Factiva; NAB
The effect of a NSI shock on capital spending is persistent, staying positive for almost 12 months, while the effect on unemployment is shorter lived. The effects are statistically significant even after controlling for the business conditions index, suggesting that the text-based sentiment measure contains information orthogonal to survey-based measures. While this is again suggestive of a causal role for the NSI, we cannot rule out the possibility that the NSI is simply a better measure of current economic activity than some of the other survey indicators.
In terms of economic magnitude, a one standard deviation shock to news sentiment is associated with capital spending rising by about 10 to 15 per cent of a standard deviation and the change in unemployment rate falling by 0.01 to 0.03 percentage points in the months following the shock. The effect on capital spending appears meaningful but the effect on the unemployment rate is very small. By comparison, these responses are faster (and about the same size) than the responses to a one standard deviation shock to business conditions (Figure 8).
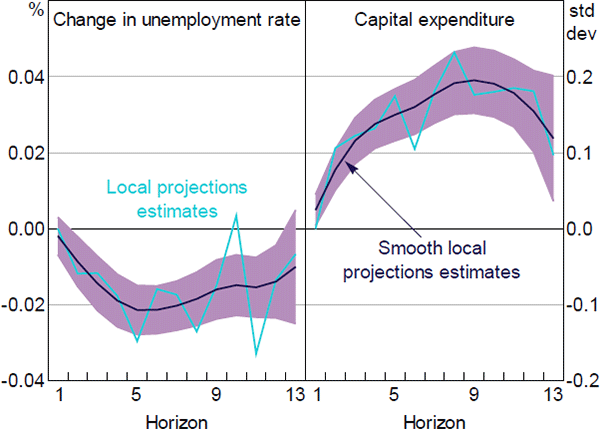
Note: Shaded areas are the 90 per cent confidence intervals for the smooth local projection estimates
Sources: Authors' calculations; Dow Jones Factiva; NAB
We also run similar but separate regressions to estimate the responses of economic indicators to news uncertainty shocks. Figure 9 shows that a temporary increase in the NUI is associated with lower business conditions, lower capital spending and higher unemployment. The responses to both NSI and NUI shocks remain generally unchanged having controlled for each other (Appendix A), suggesting that both news sentiment and uncertainty have independent roles to play in predicting economic activity in a timely fashion.
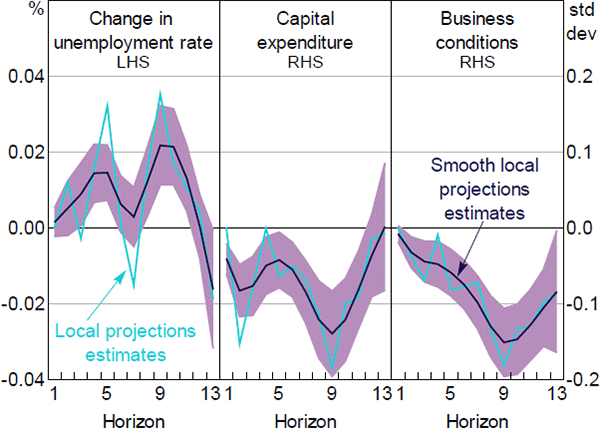
Note: Shaded areas are the 90 per cent confidence intervals for the smooth local projection estimates
Sources: Authors' calculations; Dow Jones Factiva; NAB
Taken together, we believe this analysis is suggestive of a causal interpretation in that changes in news sentiment drive survey-based measures of sentiment and economic activity more generally. In particular, changes in news-based sentiment precede movements in survey-based sentiment measures. This may be because consumers rely on high-frequency information that is broadcast through the news media. Also, the NSI predicts changes in business conditions over and above survey-based sentiment indicators and measures of current business conditions. So the information in news media may be capturing a broad audience and be more representative than survey data.
However, there are reasons to be cautious about a causal interpretation. For instance, the size of the effect of news sentiment on economic activity is small. Relatedly, in unreported results, we find that changes in news sentiment cannot explain broad indicators of business conditions, such as GDP growth. This suggests the NSI provides limited information content at a lower (quarterly) frequency. So, overall, it may be that changes in the NSI provide a very timely (and well measured) indicator of fluctuations in economic activity rather than causing changes in the behaviour of consumers and business managers. Future research could look to separate the effects of sentiment and news shocks to more directly test for causality.
6. News Sentiment and Monetary Policy
Next, we study the link between news sentiment and changes in monetary policy. This research extension is motivated by a couple of factors. First, it is important for a central bank to understand how its policy actions are reported in the news media and how this might affect beliefs amongst consumers about the outlook for the economy. For example, if the RBA wants to cut interest rates and lift confidence and economic activity then it may be important to understand how the policy action is typically communicated through the media. For this, we construct a measure of news sentiment that focuses specifically on news articles about monetary policy. Second, as suggested previously, news sentiment may be a high-frequency indicator of broader sentiment in the economy. If changes in monetary policy only affect sentiment within a few days of an interest rate change, then this effect may be captured by the NSI, but not the lower-frequency survey-based measures. So it allows us to study the causal effect of interest rates on sentiment more generally.
6.1 Monetary Policy News Sentiment Index
We first develop a monetary policy NSI for articles that mention terms specifically related to monetary policy, such as ‘monetary policy’, ‘cash rate’, and ‘RBA’. These articles account for about 15 per cent of total articles about the economy at any given time. The resulting index is quite volatile from month to month (Figure 10). Despite this, the index is significantly correlated with the stance of monetary policy as measured by ‘monetary policy shocks’ (for example, Romer and Romer (2004) for the United States, Bishop and Tulip (2017) and Beckers (2020) for Australia). These ‘shocks’ capture changes in the cash rate that are not systematically related to changes in the RBA's forecasts for economic activity and inflation.[7]
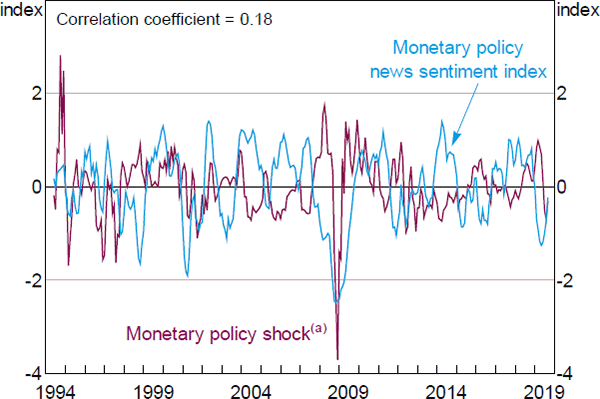
Note: (a) Measured by Beckers (2020)
Sources: Authors' calculations; Beckers (2020); Dow Jones Factiva
This correlation suggests that in setting monetary policy the RBA takes into account additional qualitative information about the future direction of the economy (over and above what is captured in the RBA's forecasts or financial markets), that is also evident in news articles; some of this information might be taken into account in the RBA's assessment of risks around the central forecasts, but not in the central forecasts themselves. Research for the United States similarly suggests that the tone of the narrative around the Federal Reserve's forecasts predicts their forecast errors for GDP growth and unemployment (and explains monetary policy decisions over and above the forecasts) (Sharpe et al 2018).
6.2 The Causal Effect of Monetary Policy on News Sentiment
We next look to establish direct evidence that unexpected changes in monetary policy cause changes in sentiment as expressed in the news media. Prior to the COVID-19 pandemic, there was extensive media coverage suggesting that lowering interest rates caused weaker sentiment amongst business and consumers (as discussed by Kirchner (2020a)). This positive co-movement of interest rates and sentiment contradicts the predictions of standard macroeconomic models. So it is important to weigh the evidence for these two competing predictions.
There is systematic variation in the language used by the news media in articles about monetary policy on the day just after a monetary policy announcement (Figure 11). For instance, the news media commonly uses the term ‘strong’ when the RBA raises the cash rate and the term ‘recession’ when the RBA lowers the cash rate. The terms ‘strong’, ‘unemployment’ and ‘volatility’ appear with similar frequency when the RBA leaves the cash rate unchanged. At face value, this suggests that the news media is discussing the balance of underlying risks to the economic outlook when reporting on monetary policy decisions. However, the term ‘crisis’ appears in the news when either the cash rate is increasing or decreasing, so the differences in language are not totally clear-cut for changes in monetary policy.[8]

Sources: Authors' calculations; Dow Jones Factiva
We test the direction of the relationship between interest rates and sentiment using an event study approach, estimating the change in news sentiment around the announcement of monetary policy decisions. Here, decisions include both announced changes to monetary policy and announcements of decisions to not change policy, so long as the market knew that a policy announcement would take place.
We exploit the fact we have a daily measure of news sentiment and examine how this sentiment measure changes in the days around monetary policy announcements. The identification strategy rests on the assumption that we can minimise the potential effect of any omitted variables by focusing on a narrow time window around monetary policy decisions. For instance, it is plausible that any change in news sentiment that occurs on the day after the monetary policy announcement will be due to changes in monetary policy and not to its actual effect on the economy. A similar identification strategy is developed by Lewis, Makridis and Mertens (2019) to identify the effect of monetary policy on consumer sentiment in the United States.
We estimate the response of daily changes in news sentiment to monetary policy shocks in a narrow window of time around RBA board meetings through local projections. Specifically, we estimate the following equation:
where NSIt+h denotes the news sentiment index on day t, NSIt−1 is the value of the index on the day prior to the announcement and st is the monetary policy shock.[9] We estimate the equation by ordinary least squares for each daily horizon h in a range of 3 days before and 10 days after the monetary policy shock. The resulting series of coefficient estimates gives us the conditional average NSI relative to the average value for the day before the monetary policy announcement.
Given the daily volatility in the NSI we also consider smooth local projections:
where all variables are as before, except that an indicator function is included for whether the projection horizon is equal to zero (I(h = 0)) or greater than zero (I(h > 0)).[10]
In gauging the effect on sentiment, we consider several alternative monetary policy shock estimates. First, we look at Romer and Romer-style estimates for Australia that are sourced (and updated) from Bishop and Tulip (2017) and Beckers (2020). Second, we consider monetary policy shocks that are identified in high-frequency event studies by isolating the surprise component of a change in monetary policy using changes in market interest rates (e.g. Kearns and Manners 2006). The interest rate surprise is calculated as the change in the 1-month overnight indexed swap rate (OIS) from the close of the day prior to the close of the day of the monetary surprise.
The local projection estimates indicate that an unexpected tightening of monetary policy of 100 basis points is associated with news sentiment declining by about one-half of a standard deviation in the days just after the policy announcement, irrespective of the policy shock measure used (Figure 12). The effect is not particularly strong given that a typical monetary policy shock will be much smaller at around 10 to 20 basis points. The relatively small economic effects of monetary policy on economic activity indicators, such as sentiment, is common when using high-frequency shocks (Nakamura and Steinsson 2018b). The effect is consistently negative for at least two weeks after the announcement for both the Bishop and Tulip (2017) and the Beckers (2020) shock (left-hand and middle panels of Figure 12). In contrast, in the case of the 1-month OIS series, the effect of the shock on sentiment is not significantly different from zero (right-hand panel of Figure 12). At the very least, we find little evidence that falling interest rates cause lower sentiment as measured in the news media.[11]
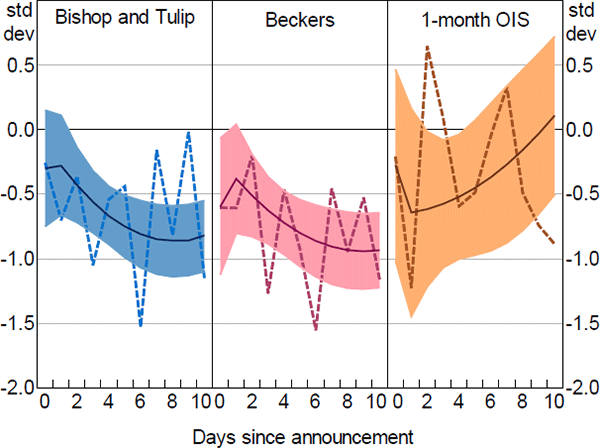
Notes: Effect of a 100 basis point shock to monetary policy; solid lines show smooth local projections, dashed lines show non-smoothed local projections; shaded areas are 90 per cent confidence intervals; standard errors are robust
Sources: Authors' calculations; Beckers (2020); Bishop and Tulip (2017); Dow Jones Factiva
7. Robustness Tests and Extensions
7.1 Responses of Economic Activity to News Sentiment Shock Using VAR
We test for the robustness of estimated impulse responses in Section 5.2 using a multivariate VAR model which includes the current and lags of change in unemployment rate, NAB capital expenditure, NAB business condition index, and the NSI[12]. A lag length of two months is chosen by a combination of information selection criteria. To identify the impulse responses of economic activity to news sentiment shocks we use a Cholesky decomposition with the NSI ordered last. This assumption imposes the restriction that shocks to economic activity affect the news sentiment contemporaneously, but news sentiment only affects economic activity with a lag. We see this as a conservative assumption that makes it more difficult to find a ‘causal’ effect of the news sentiment on the other variables. In Appendix B we present estimates that have the NSI ordered first. Similarly, we run a VAR model to estimate the impulse responses to a news uncertainty shock. Impulse responses of economic measures to a one standard deviation shock in news sentiment and news uncertainty using VAR and LP are both qualitatively and quantitatively comparable (Figures 13 and 14).
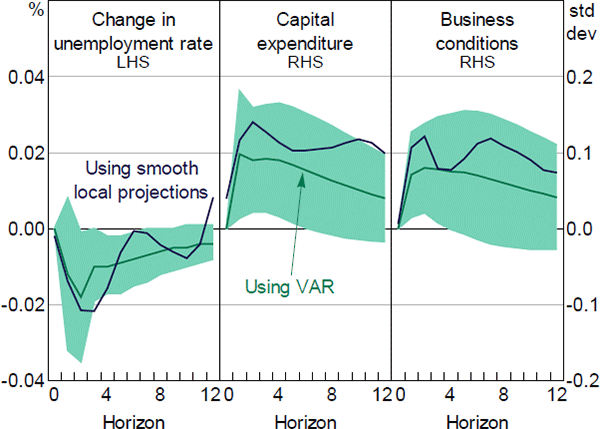
Note: Shaded areas are the 90 per cent confidence intervals for the responses using VAR
Sources: Authors' calculations; Dow Jones Factiva; NAB
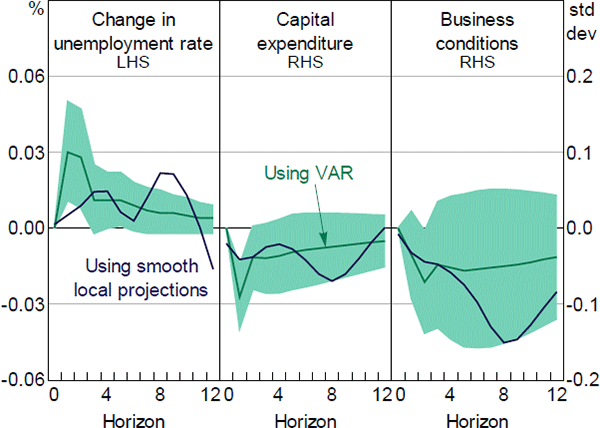
Note: Shaded areas are the 90 per cent confidence intervals for the responses using VAR
Sources: Authors' calculations; Dow Jones Factiva; NAB
7.2 Alternative Measures of News Sentiment
7.2.1 Using different lexicons
We first check the robustness of our empirical findings by using two other lexicons to construct the NSI: the Harvard General Inquirer (GI) Dictionary (Heston and Sinha 2015) and the Hu and Liu (2004) lexicon (HL). Since the Loughran and McDonald (LM) lexicon is developed specifically for the economics and finance domain, it is generally less covered in news corpus compared with the other lexicons, thus potentially resulting in fewer matched words. We find that the preferred index using the LM lexicon has a stronger correlation with the NAB business conditions index (coefficient of 0.5) than either of the NSIs using GI and HL (coefficients of 0.2 and 0.4 respectively). This suggests that context matters and that it is important to rely on lexicons designed for an economics context.
7.2.2 Using newspaper fixed effects
We follow Shapiro et al (2017) to construct an alternative measure of the NSI by estimating the time effects from the following regression over articles (indexed by a):
where sa is the net positivity score for article a and ft(a) is a sample month (t) fixed effect. We also control for the article newspaper j and the article type p (online or printed). Thus, fp(a), j(a) is the newspaper and type fixed effect. Since the tone of articles can differ between online and printed versions within the same newspaper, it's important to make sure that the NSI is independent of the trend over time in the composition of the sample across newspapers and types of articles. The monthly NSI is then the estimated monthly fixed effects from Regression (4). Figure 15 suggests that the NSI constructed using this approach is virtually the same as the original one.
7.2.3 Using machine learning
Another approach is to use the machine learning algorithm ‘word2vec’ developed by Mikolov et al (2013), which counts not only exact matches but also words that are semantically similar to the pre-defined list of words in the sentiment dictionaries. For example, if ‘downturn’ were not in the negative dictionary, its semantic similarity to ‘recession’, which is in the dictionary, ensures that it gets picked up by this method. As such, this method has the advantage of potentially capturing the context of sentiment beyond a pre-defined list of words. The algorithm uses a pre-labelled training dataset to construct the linguistic context of words. It takes as input a large corpus of words and produces a vector space in which words that share common contexts in the corpus are located close to each other. For example, the terms ‘recession’ and ‘crisis’ would be placed in close proximity. We test this method using the pre-trained dataset and find the resulting NSI to be very similar to the NSI constructed using the LM lexicon (Figure 15).
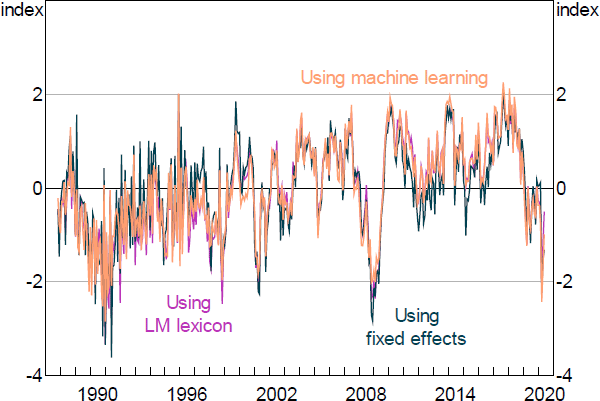
Sources: Authors' calculations; Dow Jones Factiva
8. Conclusion and Directions for Future Research
We construct a news sentiment index and find that it is useful both to understand the current state of the economy and to help explain economic conditions in the near term. Some related indicators, such as the news uncertainty index, are also useful real-time indicators of the economy.
We also present novel estimates of monetary policy-related news sentiment, which can predict changes in the cash rate even after accounting for the RBA's forecasts for the economy. This suggests that the news contains useful information about the risks to the economic outlook to which the RBA is responding when setting interest rates. We conduct an event study in the days around monetary policy announcements and find evidence that increases (decreases) in interest rates are associated with weaker (stronger) news sentiment. These results are consistent with monetary policy having its intended effects and we find no evidence of any counterproductive effects of monetary policy on news sentiment.
This paper focuses on the ability of the news sentiment indicators to explain high-frequency indicators of economic activity. We do not find any evidence that fluctuations in news sentiment explain economic activity at a longer frequency, such as quarterly measures of GDP growth and consumer price inflation. It may be worth looking at this more closely in future given the relative importance that policymakers place on these specific measures of activity. Relatedly, we have applied a very simple text analysis method in constructing our preferred news sentiment indicators. It may be worth looking at more sophisticated machine learning measures in future research (e.g. Bybee et al 2020).
Finally, we are unable to clearly disentangle the effects of shocks to news (fundamentals) from those of sentiment or ‘animal spirits’ (non-fundamentals) on the economy. Future research may be able to separate these two shocks through different types of news articles. For example, the language used in editorials and opinion articles is plausibly more associated with changes in sentiment and the language used in standard news reports may be more associated with changes in fundamentals.
Appendix A: LP and SLP Estimates Using Control Variables
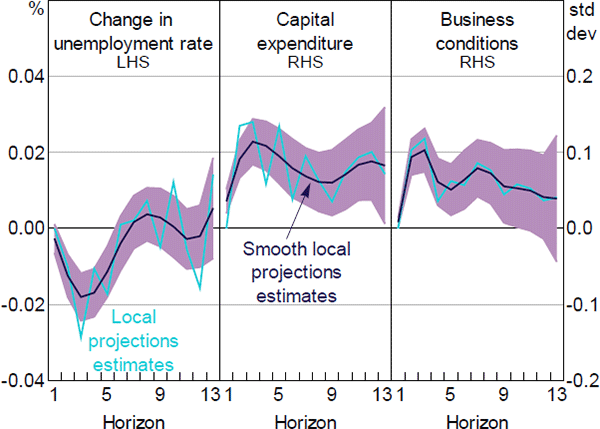
Note: Shaded areas are the 90 per cent confidence intervals for the smooth local projections estimates
Sources: Authors' calculations; Dow Jones Factiva; NAB
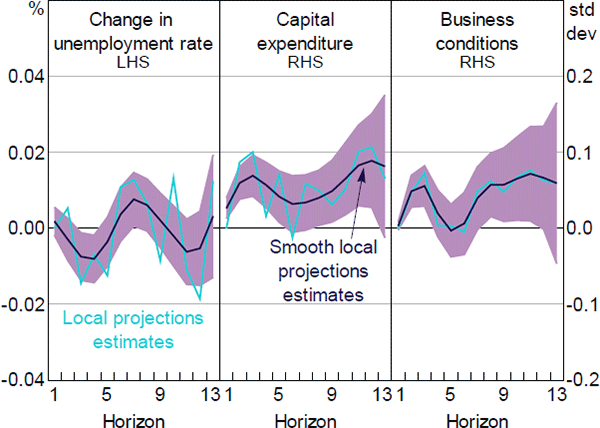
Note: Shaded areas are the 90 per cent confidence intervals for the smooth local projections estimates
Sources: Authors' calculations; Dow Jones Factiva; NAB
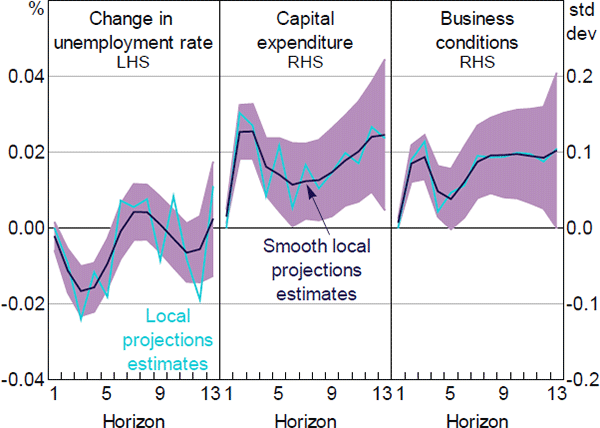
Note: Shaded areas are the 90 per cent confidence intervals for the smooth local projections estimates
Sources: Authors' calculations; Dow Jones Factiva; NAB
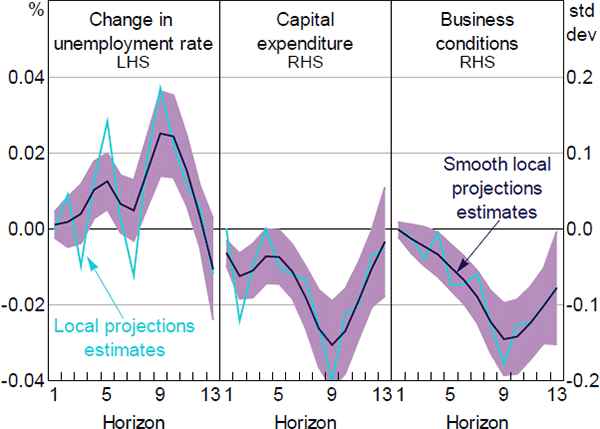
Note: Shaded areas are the 90 per cent confidence intervals for the smooth local projections estimates
Sources: Authors' calculations; Dow Jones Factiva; NAB
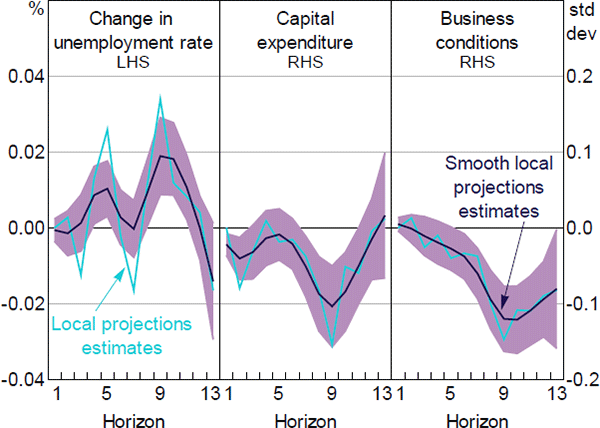
Note: Shaded areas are the 90 per cent confidence intervals for the smooth local projections estimates
Sources: Authors' calculations; Dow Jones Factiva; NAB
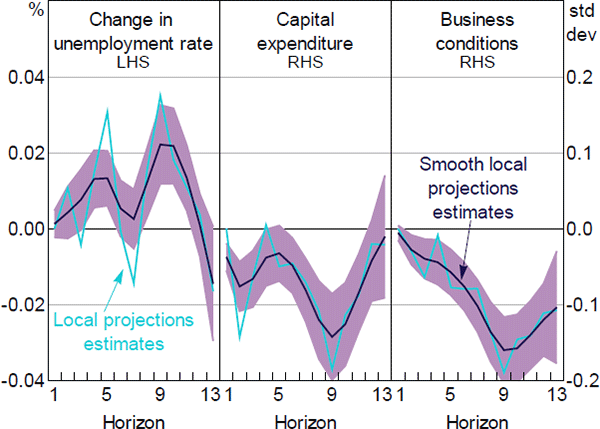
Note: Shaded areas are the 90 per cent confidence intervals for the smooth local projections estimates
Sources: Authors' calculations; Dow Jones Factiva; NAB
Appendix B: VAR Estimates with Different Ordering
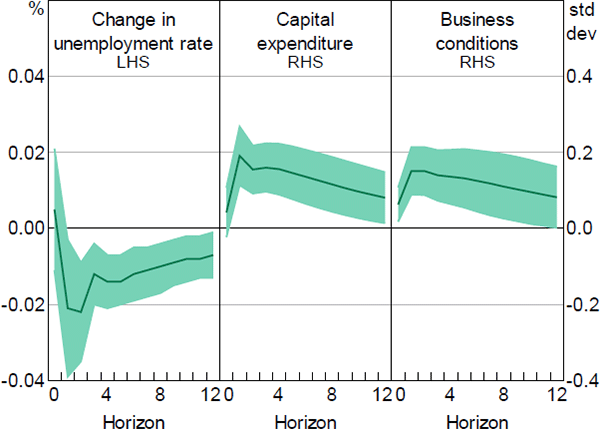
Note: Shaded areas are the 90 per cent confidence intervals
Sources: Authors' calculations; Dow Jones Factiva; NAB
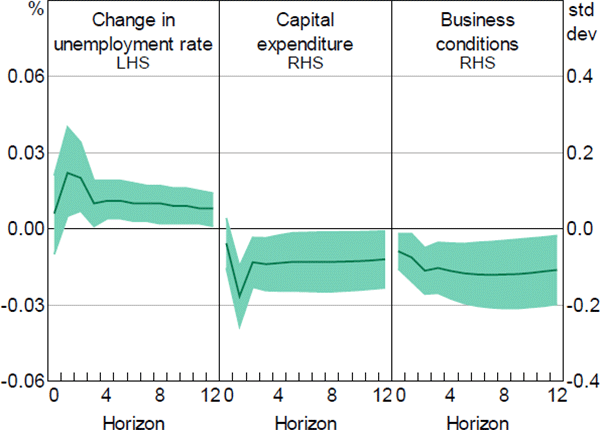
Note: Shaded areas are the 90 per cent confidence intervals
Sources: Authors' calculations; Dow Jones Factiva; NAB
Appendix C: Granger-causality Tests
Equation variable | Excluded variable | Lags | Marginal significance level |
---|---|---|---|
Weekly | |||
ANZ-Roy Morgan Consumer Sentiment Index | NSI | 4 | 0.00 |
ANZ-Roy Morgan Consumer Sentiment Index | NUI | 4 | 0.00 |
Monthly | |||
Change in unemployment rate | NSI | 2 | 0.00 |
NAB Capital Expenditure Index | NSI | 2 | 0.00 |
NAB Business Conditions Index | NSI | 2 | 0.01 |
Change in unemployment rate | NUI | 2 | 0.03 |
NAB Capital Expenditure Index | NUI | 2 | 0.00 |
NAB Business Conditions Index | NUI | 2 | 0.00 |
References
Aylmer C and T Gill (2003), ‘Business Surveys and Economic Activity’, RBA Research Discussion Paper No 2003-01.
Baker SR, N Bloom and SJ Davis (2016), ‘Measuring Economic Policy Uncertainty’, The Quarterly Journal of Economics, 131(4), pp 1593–1636.
Barnichon R and C Brownlees (2019), ‘Impulse Response Estimation by Smooth Local Projections’, The Review of Economics and Statistics, 101(3), pp 522–530.
Barsky RB and ER Sims (2012), ‘Information, Animal Spirits, and the Meaning of Innovations in Consumer Confidence’, The American Economic Review, 102(4), pp 1343–1377.
Beckers B (2020), ‘Credit Spreads, Monetary Policy and the Price Puzzle’, RBA Research Discussion Paper No 2020-01.
Bishop J and P Tulip (2017), ‘Anticipatory Monetary Policy and the “Price Puzzle”’, RBA Research Discussion Paper No 2017-02.
Bloom N (2014), ‘Fluctuations in Uncertainty’, Journal of Economic Perspectives, 28(2), pp 153–176.
Buckman SR, AH Shapiro, M Sudhof and DJ Wilson (2020), ‘News Sentiment in the Time of COVID-19’, FRBSF Economic Letter No 2020-08.
Bybee L, BT Kelly, A Manela and D Xiu (2020), ‘The Structure of Economic News’, NBER Working Paper No 26648.
Fraiberger SP (2016), ‘News Sentiment and Cross-Country Fluctuations’, in D Bamman, AS Doğruöz, J Eisenstein, D Hovy, D Jurgens, B O'Connor, A Oh, O Tsur and S Volkova (eds), EMNLP 2016 Workshop on Natural Language Processing and Computational Social Science: Proceedings of the Workshop, Association for Computational Linguistics, Stroudsburg, PA, pp 125–131.
Haddow A, C Hare, J Hooley and T Shakir (2013), ‘Macroeconomic Uncertainty: What Is It, How Can We Measure It and Why Does It Matter?’, Bank of England Quarterly Bulletin, 53(2) Q2, pp 100–109.
Heston SL and NR Sinha (2016), ‘News versus Sentiment: Predicting Stock Returns from News Stories’, Board of Governors of the Federal Reserve System Finance and Economics Discussion Series No 2016-048.
Hu M and B Liu (2004), ‘Mining and Summarizing Customer Reviews’, in R Kohavi, J Gehrke, W DuMouchel and J Ghosh (eds), KDD-2004 Proceedings of the Tenth ACM SIGKDD International Conference on Knowledge Discovery and Data Mining, The Association for Computing Machinery, New York, pp 168–177.
Hüfner FP and M Schröder (2002), ‘Forecasting Economic Activity in Germany – How Useful are Sentiment Indicators?’, Centre for European Economic Research, ZEW Discussion Paper No 02-56.
Jordà Ò (2005), ‘Estimation and Inference of Impulse Responses by Local Projections’, The American Economic Review, 95(1), pp 161–182.
Kearns J and P Manners (2006), ‘The Impact of Monetary Policy on the Exchange Rate: A Study Using Intraday Data’, International Journal of Central Banking, 2(4), pp 157–183.
Kirchner S (2020a), ‘The Effect of Changes in Monetary Policy on Consumer and Business Confidence’, The Australian Economic Review, 53(1), pp 118–125.
Kirchner S (2020b), ‘The Evidence Suggests Reserve Bank Rate Cuts Don't Hurt Confidence’, The Conversation (online), 4 February, viewed 18 July 2020. Available at <https://theconversation.com/the-evidence-suggests-reserve-bank-rate-cuts-dont-hurt-confidence-130799>.
Larsen VH and LA Thorsrud (2018), ‘Business Cycle Narratives’, Norges Bank Working Paper 3|2018.
Lewis DJ, C Makridis and K Mertens (2019), ‘Do Monetary Policy Announcements Shift Household Expectations?’, Federal Reserve Bank of New York Staff Report No 897, rev January 2020.
Loughran T and B McDonald (2011), ‘When Is a Liability Not a Liability? Textual Analysis, Dictionaries, and 10-Ks’, The Journal of Finance, 66(1), pp 35–65.
Mikolov T, I Sutskever, K Chen, G Corrado and J Dean (2013), ‘Distributed Representations of Words and Phrases and Their Compositionality’, in Proceedings of the 26th International Conference on Neural Information Processing Systems – Volume 2 (NIPS' 13), Curran Associates Inc, Red Hook, pp 3111–3119.
Moore A (2017), ‘Measuring Economic Uncertainty and Its Effects’, Economic Record, 93(303), pp 550–575.
Nakamura E and J Steinsson (2018a), ‘High-Frequency Identification of Monetary Non-Neutrality: The Information Effect’, The Quarterly Journal of Economics, 133(3), pp 1283–1330.
Nakamura E and J Steinsson (2018b), ‘Identification in Macroeconomics’, Journal of Economic Perspectives, 32(3), pp 59–86.
Plagborg-Møller M and CK Wolf (2019), ‘Local Projections and VARs Estimate the Same Impulse Responses’, Unpublished manuscript, 10 July. Available at <https://economics.indiana.edu/documents/local-projections-and-VARs-estimate-the-same-impulse-responses.pdf>.
Roberts I and J Simon (2001), ‘What Do Sentiment Surveys Measure?’, RBA Research Discussion Paper No 2001-09.
Romer CD and DH Romer (2004), ‘A New Measure of Monetary Shocks: Derivation and Implications’, The American Economic Review, 94(4), pp 1055–1084.
Scotti C (2016), ‘Surprise and Uncertainty Indexes: Real-Time Aggregation of Real-Activity Macro-Surprises’, Journal of Monetary Economics, 82, pp 1–19.
Shapiro AH, M Sudhof and D Wilson (2017), ‘Measuring News Sentiment’, Federal Reserve Bank of San Francisco Working Paper 2017-01, rev March 2020.
Sharpe SA, NR Sinha and CA Hollrah (2017), ‘What's the Story? A New Perspective on the Value of Economic Forecasts’, Board of Governors of the Federal Reserve System Finance and Economics Discussion Series 2017-107, rev August 2018.
Thorsrud LA (2020), ‘Words are the New Numbers: A Newsy Coincident Index of the Business Cycle’, Journal of Business & Economic Statistics, 38(2), pp 393–409.
Acknowledgements
For helpful comments and suggestions we thank Benjamin Beckers, James Bishop, Luci Ellis, Richard Evans, Luke Hartigan, Roman Kovalenko, John Simon, Susan Slocum, Kaja Stojkov and Benjamin Wong. The views expressed in this paper are those of the authors and do not necessarily reflect the views of the Reserve Bank of Australia. The authors are solely responsible for any errors.
Footnotes
Broadly speaking, ‘sentiment’ captures people's beliefs about the mean of the distribution of future economic outcomes (the first moment) while ‘uncertainty’ captures the variance of people's beliefs (the second moment) (Haddow et al 2013).[1] [1]
The individual words are not weighted by the degree of positivity or negativity.[2]
The uncertainty index is therefore measured on a different basis to the sentiment index. This is mainly due to practical reasons – the terms in the uncertainty dictionary do not appear very frequently within articles. This approach to measuring uncertainty using text analysis is equivalent to that used by others in the literature (e.g. Moore 2017).[3]
The NAB survey typically surveys respondents in the last week of the month, so the NSI has the advantage of picking[4] turning points earlier in any given month because of this timing difference. [4]
Apart from the unemployment rate, these measures are probably best thought of as capturing changes in nominal economic conditions rather than real activity. [5]
Estimates using other controls such as the consumer sentiment index and the business confidence index yield similar results and can be found in Appendix A. [6]
The Beckers (2020) shock captures non-systematic changes in the cash rate accounting for the RBA's forecasts and credit spreads. [7]
After a cash rate increase, the term ‘crisis’ often appears as a reference to the post-crisis economic recovery. Following a cash rate decrease, the term ‘crisis’ often appears in articles discussing the crises related to the Australian bushfires and COVID-19 pandemic in 2020. [8]
Many news articles on the day of the monetary policy announcement are actually written the night before. Unfortunately, we are not able to separately identify these articles. [9]
Unlike the fully non-parametric projections, the smooth local projections estimate the responses at all horizons simultaneously. [10]
The relationship between unexpected changes in monetary policy and news sentiment is not affected by the presence of widely-reported economic releases in the week of the monetary policy decision. We find very similar effects in the weeks in which the national accounts, inflation and labour force statistics are released.[11]
In Appendix C, we present the Granger-causality results of the equations with key macroeconomic measures as dependent variables and NSI or NUI as explanatory variables. The marginal significance levels are reported for the hypothesis that all lags of the given right-hand side variable can be excluded. In other words, the excluded variable does not Granger-cause the equation variable. At the 5 per cent level of statistical significance, all null hypotheses are rejected, suggesting that the NSI and the NUI Granger-cause the key macroeconomic measures.[12]