RDP 2018-03: The Effect of Zoning on Housing Prices 7. Geographic Distribution of Zoning Effects within Cities
March 2018
- Download the Paper 1,488KB
Figures 3–6 show estimates of the zoning effect for the average property in local government areas (LGAs). We split property prices into structure values and land values in each LGA, using the CoreLogic data on property prices and the valuer general estimates for land values for each LGA (as this allows structure value to vary across LGAs for reasons other than just floor area).[19] We then estimate physical land values by running separate hedonic regressions for each LGA of the form of our large regression in Section 4.[20] Our estimate of the zoning effect by LGA is then the remaining portion of land value not accounted for by the physical value.
If zoning restrictions were relatively uniform in nature across a city, we would expect the zoning effect to be largest (in dollar terms) where underlying demand to develop land is the highest, which is likely to be close to the CBD, or perhaps in coastal areas (Kulish et al 2011). Our results are broadly consistent with this expected pattern. Figures 3–6 also indicate a high degree of spatial correlation in our estimates – neighbouring LGAs tend to have similar zoning effects. Spatial correlation could reflect similarity in zoning restrictions and demand to develop land, but it could also arise because supply restrictions in one location spill over to neighbouring locations that are close substitutes.
Section 3 of the Online Appendix contains maps of the zoning effect as a share of average property value in each LGA. Patterns in these maps are less clear, as there is considerable variation in structure values and physical land values (mostly reflecting average lot sizes) between neighbouring LGAs.[21] The Online Appendix also contains maps showing physical land values by LGA. As Fujita, Krugman and Venables (1999) discuss, the demand for land increases with proximity to the city centre.
We have not investigated how, or if, certain characteristics of LGAs systematically contribute to the patterns we observe (e.g. distance from CBD/coast, population density, demographics, minimum lot sizes, maximum building heights), but this could be a possible avenue for future research.
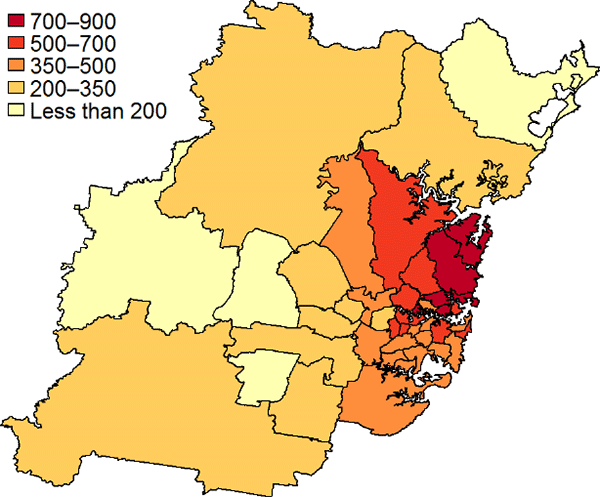
Note: The 2011 edition of the ABS's LGA boundaries are used for Sydney
Sources: ABS; Authors' calculations; CoreLogic
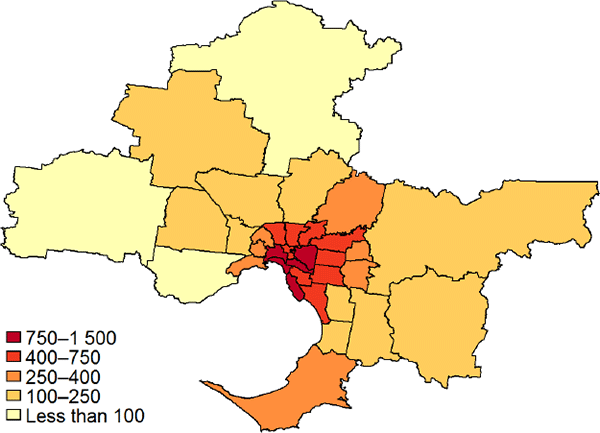
Sources: ABS; Authors' calculations; CoreLogic
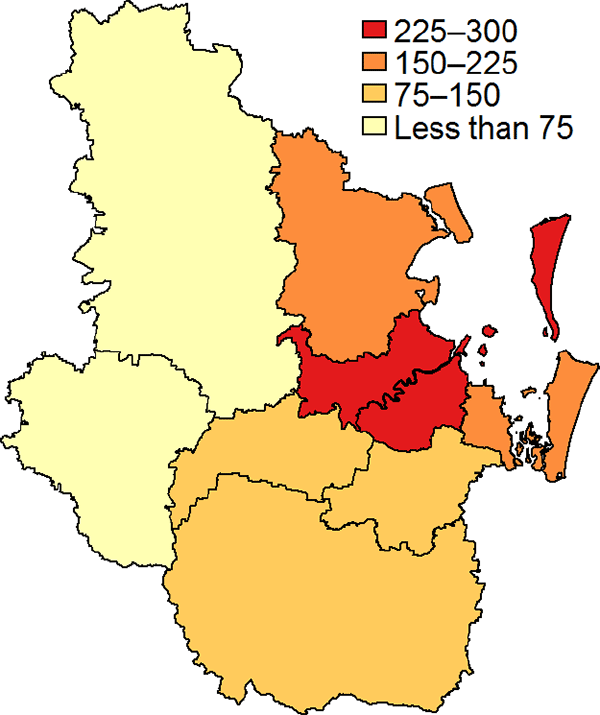
Sources: ABS; Authors' calculations; CoreLogic
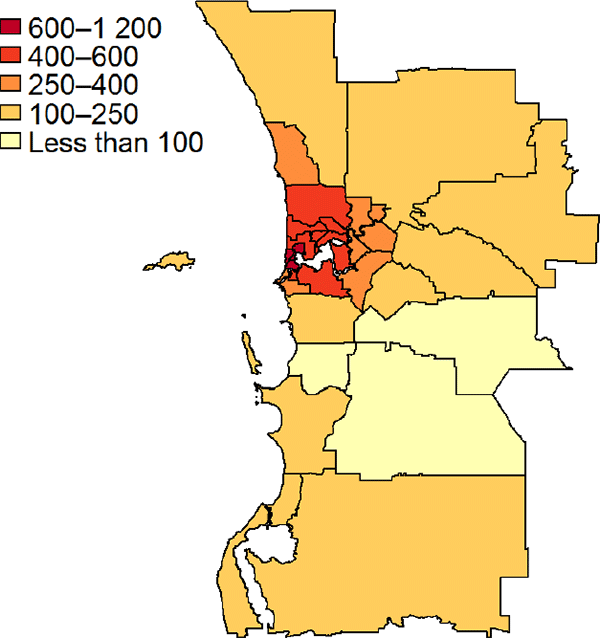
Note: Peppermint Grove LGA was combined with Mosman Park LGA for estimation purposes
Sources: ABS; Authors' calculations; CoreLogicFootnotes
As shown in Table 5, valuer general structure estimates imply a higher zoning effect estimate for Brisbane and Perth and lower for Sydney than would an average of the three valuation approaches. [19]
One difference is that we include sales from both 2015 and 2016 to estimate our hedonic equation, as the sample size for some LGAs was very small using a single year, making estimation of the coefficients difficult. However, we apply the estimated log land area coefficient only to the average sale price and land area of houses sold in 2016. [20]
Sunding and Swoboda (2010) argue that the ‘correct’ level of aggregation is the metropolitan area, because although different localities within the area have different regulations, if households are mobile then the zoning effect across the area will tend to equalise. [21]