RBA Annual Conference – 2015 Housing Prices and Entrepreneurship: Evidence for the Housing Collateral Channel in Australia Ellis Connolly, Gianni La Cava and Matthew Read[*]
1. Introduction
Changes in housing prices can affect the Australian economy through several channels. One channel that has not been previously documented is how changes in housing prices could affect entrepreneurial activity in Australia. Small businesses make a significant contribution to the Australian economy, accounting for 43 per cent of employment in the private non-financial sector and a third of production in 2012/13. Under the ‘housing collateral channel’ of entrepreneurship, rising housing prices increase the housing equity of residential property owners. This increases the potential borrowing capacity of credit-constrained entrepreneurs, allowing them to finance more entrepreneurial activity by using their housing equity.[1]
This paper is motivated by at least two factors. First, studying the housing collateral channel may help us to better understand how monetary policy affects the real economy. For instance, lower real interest rates typically lead to higher housing prices; if the housing collateral channel is operating, then expansionary monetary policy may lead to more entrepreneurial activity, such as the formation of new businesses. Furthermore, it is generally believed that new businesses contribute disproportionately to employment and output growth, implying that this channel of monetary policy transmission could be quite strong.[2] Second, if there is a housing collateral channel, then it implies that some entrepreneurs are credit constrained, which has implications for the design of policies aimed at improving entrepreneurs' access to finance.
The existing literature implies that there is a single housing collateral channel but, in fact, there are at least three channels through which housing prices can affect entrepreneurship.
- Business loans. Higher housing prices increase the value of housing collateral against which entrepreneurs can directly secure business loans.
- Personal lending products. Higher housing prices increase the value of housing equity available for entrepreneurs to draw down on by using housing-related lending products (e.g. home equity loans and cash-out refinancing).
- Personal guarantees. Higher housing prices increase the value of personal guarantees, as this is a function of the value of the guarantor's assets (such as residential property).
The existence of a housing collateral channel has been a significant area of empirical research recently. Several papers find a positive relationship between changes in housing prices (or housing equity) and entrepreneurship, which they interpret as evidence of a housing collateral channel (Adelino, Schoar and Severino 2013; Corradin and Popov 2013; Fort et al 2013; Schmalz, Sraer and Thesmar 2013). However, these papers may have confounded a housing collateral channel with broader wealth effects that can also increase entrepreneurial activity. In particular, a rise in housing prices increases the net wealth of home owners, which may encourage them to take more risk (including starting a business) independently of any change in their borrowing capacity. To address this identification problem, Jensen, Leth-Petersen and Nanda (2014) exploit a natural experiment in which an exogenous mortgage reform in Denmark provided entrepreneurs with greater access to home equity lines of credit, thereby unlocking a home equity source of finance to start a business. They find evidence of a housing collateral channel, although the economic effect of the channel is relatively small. Similarly, Kerr, Kerr and Nanda (2014) find that housing collateral plays a role in business formation, but that wealth effects appear to be more important.
We explore the housing collateral channel in Australia using three approaches. First, we explore the issue from the ‘lender's perspective’ by drawing on liaison with lenders (carried out by the Reserve Bank of Australia), and examine the role of housing collateral in lenders' credit assessment frameworks (Section 2). Second, we take a ‘borrower's perspective’ and explore the evidence for the existence of a housing collateral channel using household survey data (Section 3). Third, we adopt a ‘combined perspective’ and examine the evidence for the channel using postcode-level information (Section 4). Finally, we draw together all our results and discuss the implications (Section 5).
2. Evidence from Liaison with Lenders
To deepen our understanding of the role of housing collateral in small business lending, we conducted a series of interviews with small business finance experts at a range of lending institutions. These institutions account for around 95 per cent of the lending to small and medium-sized enterprises in Australia. In this section of the paper, we outline the key themes that emerged from the interviews, focusing on the role of housing collateral in the decision to lend to small businesses and the conditions of that lending. We also consider whether collateral is important in the provision of finance to start-up businesses, either through business loans or personal lending products such as home loans. Where relevant, we supplement the liaison with evidence from aggregate statistics and survey-based information.
2.1 The role of housing collateral in small business lending
The interviews highlighted that the provision of housing collateral by small business borrowers has some influence on the lending decision, and has a significant effect on the terms of the lending.
2.1.1 How lenders make small business lending decisions
Most of the small business finance experts identified housing collateral as being relevant to the framework they use when making lending decisions, although few considered it to be the most important element. To place the role of collateral in context, the lenders described the key factors that they take into account when assessing small business loan applications. The factors highlighted were similar across the lenders, and were typically drawn from the traditional ‘5Cs’ model, which involves evaluating the borrower's character, capacity, collateral, capital and the broader conditions surrounding the loan. The lenders noted that they drew on a wide range of qualitative and quantitative information in making this assessment, and that no single factor was sufficient of itself to justify approving a loan application. The lenders noted that this approach was motivated by their internal risk controls, along with prudential regulatory requirements and their legal obligation to lend responsibly.
The character of a small business borrower is a primary focus of most institutions when they make lending decisions because it is a key determinant of the borrower's probability of defaulting on the loan. When lenders assess the borrower's character or reputation, they are looking for evidence of management or business experience, a well-developed business plan, a strong advisory team and a commitment to repay the loan. Gathering this evidence typically involves face-to-face interviews with the potential borrower, analysing the borrower's credit default history and drawing on the local knowledge of the lending institution's managers.
The capacity of the borrower – whether the borrower has sufficient income to service their debts on an ongoing basis – is critical to the lending decision. To determine this, lenders analyse the financial records of the business, the business' actual and projected cash flow, along with the potential borrower's personal financial history. Several of the small business finance experts noted that it is often difficult to extract evidence of the profitability of the business from the financial statements prepared by accountants, since the statements are dated and some may have been prepared with the objective of minimising tax. Instead, most of the lenders stated that they prefer to have access to a history of the borrower's transactions to assess their capacity, which is most easily obtained if the small business is already a customer. As a result, lenders have a natural preference to lend to existing customers with a well-established transactions history. Lenders typically analyse several years of data to abstract from the effect of temporary shocks on the small business.
In contrast to the first two factors, which the lenders uniformly agreed were crucial in lending decisions, there was some divergence regarding the importance of the collateral provided by the borrower, particularly in the form of residential housing. Some lenders downplayed the importance of collateral, arguing that it was just a ‘backstop’ that could reduce the loss for the lender in the event of default, without affecting the probability of a default occurring. In addition, some emphasised how costly and ‘undesirable’ it was to take possession of a business owner's home upon default. These lenders viewed taking possession of the home as a third and final line of defence, after the borrower's capacity to repay has been exhausted, and after any other collateral, such as commercial property or equipment, has been sold to recover the value of the debt.
In contrast, some of the lenders viewed housing collateral as essential, particularly for larger loans. These lenders highlighted that the provision of housing collateral was an indicator of the borrower's character; it provided the small business borrower with strong incentives to repay, with the borrower clearly having ‘skin in the game’. In this way, housing collateral was seen as not just reducing the loss given default, but also the probability of default. In addition, some lenders viewed home ownership as a positive signal of the borrower's ability to accumulate wealth and as an indicator of the entrepreneur's capacity to repay debt. Even if the home was not explicitly provided as collateral against a business loan, home ownership provides the entrepreneur with a channel for raising additional funds if business revenues fall.
Regardless of the differences in opinion between the lenders on the role of collateral, all the lenders indicated that a very high proportion of their small business lending books were in fact collateralised. Typically, at least three-quarters of small business lending was collateralised, and around one-half to two-thirds of this lending was secured by housing. Consistent with this, the lenders interviewed had only a limited appetite for unsecured lending.[3]
When asked whether the role of collateral had evolved over the past decade or so, the lenders all indicated that there had been no change in their preference for secured lending. While the lenders acknowledged that there had been a broad reassessment of risk during the 2007–09 global financial crisis, they did not believe that this had led to increased demand for collateral by lenders. This is in contrast to the experience in many other developed countries; the OECD (2013) found that small business lending conditions deteriorated in most of the developed countries surveyed between 2007 and 2011, partly due to greater demand for collateral from lending institutions. Some of the lenders suggested that any increase in collateral since 2007 was actually due to small business borrowers becoming more price sensitive and choosing to offer more collateral to obtain a lower interest rate.
Consistent with lenders' strong appetite for collateral, they typically require small business owners to provide personal guarantees over the loans to the business. These guarantees result in the owners being liable to repay the loan in the event that the small business defaults. It was noted that personal guarantees are more prevalent on small business loans in Australia than in the United Kingdom or the United States. It was suggested that the information available in these jurisdictions about the character and capacity of the borrower – partly through positive credit reporting regimes – reduced the need for personal guarantees. Given this, the introduction of comprehensive credit reporting in Australia had the potential over time to improve the information available to lenders. If this additional information provides lenders with more confidence in borrowers' character and capacity, it has the potential to reduce the importance of personal guarantees (and therefore housing collateral) in small business lending.
The amount of capital or equity that the borrower has injected into the business is also important to the lending decision because it reduces the probability of default as well as the loss given default. As part of their small business lending criteria, several of the lenders apply a maximum loan-to-valuation ratio, taking into account all lending to the entrepreneur (including home loans). As a result of this, small business borrowers could potentially be constrained by the amount of housing equity they have, even if their home was not being offered as collateral in their small business borrowing.[4]
The broader conditions surrounding the loan – such as the macroeconomic climate and the conditions in the industry in which the small business is operating – are also relevant to the lending decision. In this context, the presence of housing collateral can be particularly important during macroeconomic downturns, since some of the lenders noted that their willingness to engage in residentially secured small business lending tends to be less cyclical than other lending products, such as overdraft facilities and equipment finance.
2.1.2 The terms of small business loans
Most of the lenders indicated that the provision of collateral was particularly important for the terms of small business loans, including the loan size and the interest rate payable. In contrast to their lending to large businesses, lenders typically do not rely on covenants in small business lending and do not ask for frequent updates on the financial performance of the business. Instead, lenders prefer to manage their small business loan book on a portfolio basis and only reassess a particular loan if a negative event occurs, such as the borrower missing a payment or the loan falling into arrears. Given this portfolio approach, lenders rely on the availability of collateral when determining the terms of the loan; collateralised loans are likely to be larger and charged a lower interest rate than unsecured loans.
The lenders identified the key factors determining the interest rates on small business loans as the expected losses on the loan, the cost of loan origination, the cost of funding the loan and prudential regulation, including capital requirements. The provision of collateral can influence several of these factors, by potentially reducing the expected losses, the origination cost and the amount of capital that is required to be held against the loan.
The lenders indicated that the expected loss on an unsecured business loan is significantly larger than when the loan is secured with residential property. As a result of this, the pricing of loans is heavily influenced by the provision of collateral. Many of the lenders noted that this effect is more important than the effect of collateral on the lending decision itself. In addition, several lenders noted that under prudential regulations, the amount of capital that a lender has to hold against the credit on its balance sheet is lower where the borrowers have provided residential property as security, reflecting the lower risk on these loans.
Nevertheless, there were divergent views regarding the extent to which housing collateral reduced loan losses in the event of default. Some lenders highlighted that residential property was a very effective form of security, because it is more likely to retain its value in the secondary market than equipment or even commercial property, where prospective buyers have more bargaining power when the seller is a mortgagee in possession. Given this, some lenders charge higher interest rates on small business loans that are secured by commercial property compared with loans secured against residential property.[5] In contrast, other lenders noted that residential property was a weaker form of security, particularly where there is a first mortgage on the property that has priority in the event of a distressed sale. These lenders considered residential property as a ‘secondary’ form of security, and had a preference for ‘primary’ forms of security, such as a mortgage over the assets of the small business itself.
The cost of loan origination can also potentially be reduced if the borrower provides collateral. Over the past decade or so, there has been a shift towards using automated tools in assessing lending applications to keep down origination costs; this is particularly the case at the major banks, which are allowed to manage the credit risk on their exposures to small businesses on a pooled basis where each exposure is less than $1 million (APRA 2008). These automated tools typically assess whether the borrower passes a set of tests based on characteristics such as business age, industry, debt serviceability, credit history and collateral. If the borrower fails one of these tests, then the application goes to a credit officer for manual assessment. On this basis, applications for unsecured borrowing typically require more manual assessment to determine whether there is sufficient evidence on loan serviceability.
2.1.3 Comparing liaison with survey evidence and aggregate lending data
The role of housing collateral in small business lending as described in the interviews appears to be broadly consistent with survey evidence and aggregate data on the distribution of lending. The Household, Income and Labour Dynamics in Australia (HILDA) Survey provides detailed data on household income and balance sheets, including for around 1,000 households that own small businesses.[6] When these business-owning households are broken into quintiles based on average business income over the preceding three years, there is a positive relationship between business income and the proportion that owe business debt (Figure 1, top panel). This would be consistent with lenders requiring evidence of capacity to repay debt (particularly past business income) before extending business lending products.
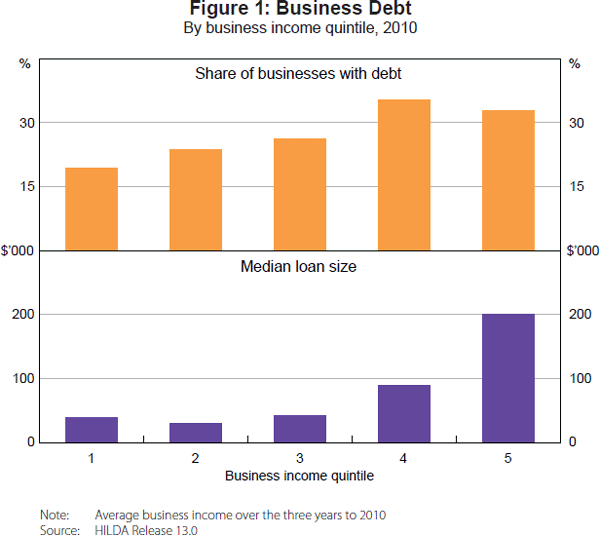
In contrast, when the business-owning households are broken up into quintiles based on housing equity, there does not appear to be a clear relationship between housing equity and the proportion owing business debt (Figure 2, top panel). This is consistent with housing equity not being as important as evidence of capacity in small business lending decisions, as indicated by most of the lenders interviewed.
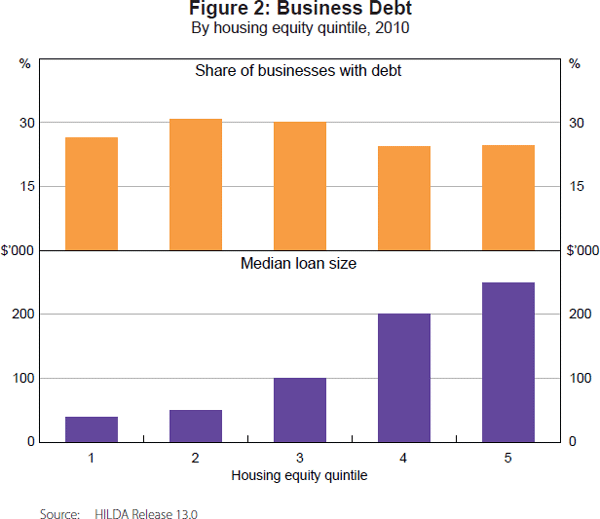
Focusing on the size of business loans for those households owing business debt, the median loan size is considerably larger for households with higher housing equity; this is also true for households with higher business income, although the relationship is not quite as strong (Figures 1 and 2, bottom panel). While this is only an unconditional correlation, it is consistent with there being some relationship between housing equity and the terms of small business lending.
In addition, aggregate lending data clearly highlight the importance of housing collateral in the pricing of small business loans. Data collected by the Reserve Bank on standard variable interest rates confirm that small business overdraft rates are significantly higher than residential-secured small business rates (Figure 3). Data on business borrowing by the size of the lending facility also indicate that a high proportion of larger facilities tend to be lent at around the residential-secured interest rate, while small facilities are more likely to be lent at higher rates consistent with unsecured lending (Figure 4). This suggests that the provision of housing collateral influences both the interest rate charged and the size of the loan.
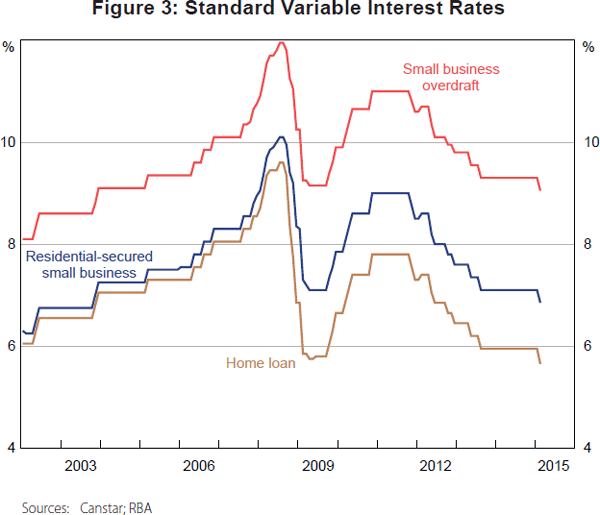
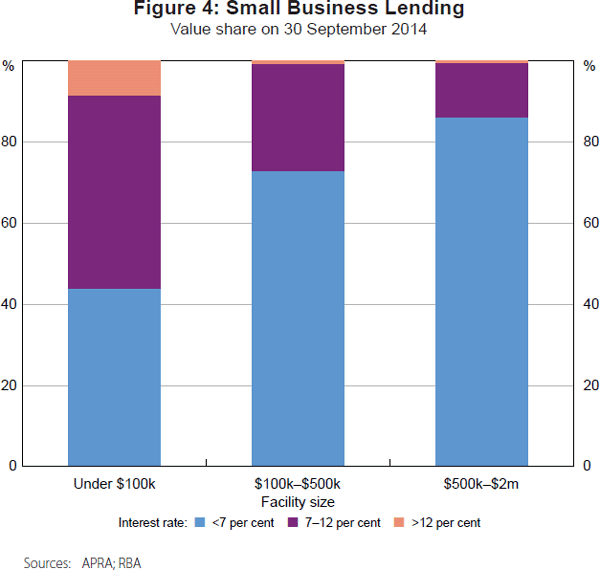
2.2 Start-up businesses and access to lending
Most of the small business finance experts acknowledged that it is relatively difficult for start-up businesses to access business lending products. This reflects the much higher risk of default on such lending, which can be around twice the risk of default on loans to established small businesses. Lenders expect potential borrowers to demonstrate their capacity to repay the loan from the cash flow of the business. Such evidence is inevitably absent for a start-up. Some institutions have built a threshold into their automated tools such that a business must have been operating for at least two years for an application to receive approval. As a result, applications from start-up businesses require more manual risk assessment, increasing the cost of originating the loan. The lenders noted that it was particularly unlikely that they would lend to a start-up in a new or emerging industry, as it was very difficult for the entrepreneur to adequately demonstrate loan serviceability.
The lenders all emphasised that the provision of collateral was not sufficient of itself to justify the approval of a business loan to a start-up. Rather, evidence of capacity to service the loan was much more important. Nevertheless, several lenders noted that the presence of collateral increases the likelihood of lending to a start-up, because the collateral serves as assurance to the lender that the loan is a responsible extension of credit. In particular, if the entrepreneur owns their home, some lenders impose a shorter minimum threshold period for the business to be operating before a loan application will be automatically approved.
Some of the lenders expressed concern that the high level of housing prices may make it more difficult for young entrepreneurs to enter the housing market. In turn, young entrepreneurs may find it more difficult than established business owners to post residential security, which could reduce their access to small business finance at a reasonable price.[7] In particular, the high personal debt and gearing levels required to achieve first home ownership can negatively affect a borrower's capacity to service additional debt, reducing the likelihood of receiving a small business loan approval. Consistent with this, the lenders acknowledged that their small business borrowers are typically older, well established and own property with significant equity. The vast majority of approved small business loan applications are for these existing customers to refinance their facilities.
Young entrepreneurs may also be more reliant on their housing equity to access small business lending products since lenders are increasingly reluctant to rely on guarantees from family members. Lenders are less likely to accept third-party guarantees due to the rigorous due diligence expected by the courts.[8] In addition, according to one lender, the tighter regulations under the National Consumer Credit Protection Act 2009 (NCCP) have made lenders more reluctant to rely on guarantees from family members when extending loans to first home buyers; this may delay home ownership for young entrepreneurs and reduce their capacity to build home equity.
The lenders suggested some alternative avenues for young entrepreneurs starting a business to access finance. Many of the lenders highlighted that equity rather than debt financing was more appropriate for start-ups given their risk profile. Equity could be obtained from family or friends through equity partnerships (as an alternative to debt guarantees), or through emerging channels such as small-scale venture capital or crowdfunding.
Where young entrepreneurs are seeking small business lending, lenders suggested that they start by opening a transaction account with the lending institution. This can be used to build up a transaction history as evidence of their capacity to service debt. Over time, the entrepreneur could migrate to more attractively priced lending products, such as equipment leasing and eventually term loans.
2.3 The use of home loans for business purposes
The use of home loan funds for business purposes is a separate channel through which housing collateral could influence entrepreneurship. The lenders widely acknowledged the intertwined nature of the entrepreneur's business and personal finances, particularly for sole traders and family businesses. In response to this, the lenders actively seek to analyse the finances of their small business customers on a consolidated basis, including their personal finances. Some lenders speculated that a share of their home loans to self-employed borrowers, and home equity loans for ‘investment purposes’, could be used in part to finance small businesses.
The distinction between borrowing for business purposes and personal purposes has been made clearer since the introduction of the NCCP. Financial institutions are effectively required to separately identify lending for personal as opposed to small business purposes, given the additional consumer protections that apply to personal lending. The lenders implement this by asking customers the purpose of the loan when they make a loan application or seek a redraw on a home loan. However, once a borrower has indicated that a loan is for a non-business purpose and the funds have been lent, it is not feasible for lenders to determine the extent to which the funds are used for business purposes. This is particularly the case for products such as home loans with offset accounts, because borrowers can draw funds by running down the offset account balance without being required to indicate the purpose.
The HILDA Survey highlights the widespread use of personal lending products by households that own small businesses, particularly start-ups (Table 1). These households were more likely than employee households in 2010 to owe residential-secured debt, particularly in the form of second mortgages, or property investor debt. Focusing on households owning young businesses (those owned in 2010 but not in 2006), these households were somewhat less likely than other business-owning households to owe business debt, and just as likely to owe home debt or credit card debt. This could be consistent with these households finding it harder to raise business debt and instead relying on personal lending products to fund their young business.
Employee households | Business-owning households | Households owning a young business(a) | |
---|---|---|---|
Business debt | 0 | 27 | 21 |
Home debt | 44 | 51 | 53 |
Of which: second mortgages |
6 | 11 | 8 |
Property investor debt | 12 | 18 | 17 |
Credit card debt | 33 | 36 | 38 |
Any debt | 82 | 85 | 87 |
Note: (a) Households owning a business in 2010 that did not own a business in 2006 Sources: Authors' calculations; HILDA Release 13.0 |
Many of the lenders suggested that the widespread use of personal lending products by entrepreneurs may be due to a lack of awareness of small business lending products and the tax benefits they can provide. One of the key financial benefits of small business lending products relative to personal loans is the capacity to deduct the interest paid from the small business' tax liability. However, the benefit of interest deductibility is only relevant for small businesses that actually have a tax liability. Data from the Australian Taxation Office (ATO) indicate that in 2011/12, only 44 per cent of micro businesses and 69 per cent of small businesses paid tax. Start-up businesses in particular may take some time to become profitable, largely negating the benefit of interest deductibility.
A final reason why entrepreneurs may prefer to draw on their home loan is that the interest rate tends to be lower than for residential-secured business loans. Data collected by the Reserve Bank on standard variable interest rates suggest that residential-secured small business rates are typically around 1 percentage point higher than home loan rates (Figure 3). The lenders indicated that residential-secured small business loan portfolios have historically experienced higher losses than home loan portfolios. Given this, lenders are required to hold more capital against residential-secured small business loans than home loans, and accordingly charge higher interest rates. Lenders also noted that the home loans of small business borrowers tend to be riskier than for other borrowers; small business borrowers pay their home loans at a slower rate, maintaining a high rate of gearing for a longer period, with the funds potentially being used to finance the business.[9]
3. Evidence from Household Survey Data
We now examine the evidence for a housing collateral channel from the ‘borrower's perspective’. We investigate the relationships between housing equity and several facets of entrepreneurial activity using household survey data. More specifically, we explore the effect of housing equity on: (1) business formation; (2) the use of business debt by new entrepreneurs; and (3) employment by new entrepreneurs.
Previous studies have typically examined the relationship between changes in housing prices and business formation rates (or other facets of entrepreneurial activity), and have interpreted a positive correlation as indirect evidence of a housing collateral channel (see, for example, Adelino et al (2013) and Schmalz et al (2013)). However, these studies have lacked data on entrepreneurs' balance sheets, and so have been unable to provide direct evidence for the existence of such a channel. Instead, the correlation between housing price growth and business formation could reflect wealth effects, where the probability of starting a business is a function of wealth for reasons other than housing wealth facilitating access to finance. Furthermore, the existence of a housing collateral channel implies that entrepreneurs actually use their housing collateral to access business finance, but previous studies have been unable to demonstrate this. In contrast, the HILDA Survey provides data on both sides of household balance sheets. These data allow us to directly examine the relationship between housing equity and entrepreneurial activity, including new entrepreneurs' use of business loans. Importantly, we are also able to provide evidence that business formation responds to changes in housing wealth but not changes in other types of wealth, which suggests that we are identifying a housing collateral channel rather than wealth effects. Finally, the HILDA Survey data provide a rich set of control variables that are likely to be correlated with housing equity and entrepreneurial activity, such as age, education and income.
3.1 Data
Using person-level data from the HILDA Survey – an annual household-based longitudinal study – we identify entrepreneurs in each year from 2001 to 2013. An individual is classified as being an entrepreneur if they reported working in their own business in the week before the survey. On average over the sample period, around 10 per cent of individuals over the age of 15 years owned a business.[10] Of these entrepreneurs, over 90 per cent operated a ‘small’ business (i.e. with fewer than 20 employees) and around 60 per cent were sole traders (Figure 5).
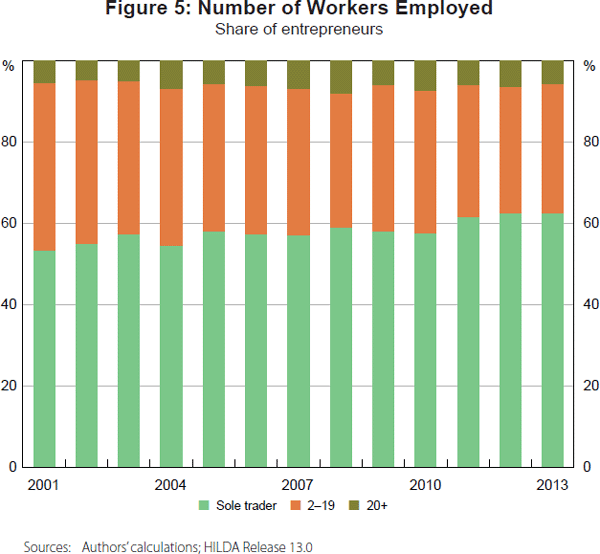
3.2 Econometric analysis
3.2.1 Business formation
A positive relationship between housing equity and business formation could provide evidence of a housing collateral channel. We identify an individual as starting a business if they transitioned into entrepreneurship between two consecutive surveys and were not identified as previously owning a business. The purpose of excluding previous business owners is to avoid a potential source of measurement error, which could be correlated with housing equity: some business owners appear to repeatedly transition into and out of self-employment.[11] On average over the sample period, around 1½ per cent of respondents started a business in the following year.
We employ probit models to quantify the effect of housing equity on the probability of business formation. The models are specified as follows:
where yigt is an indicator variable that is equal to one if individual i living in region g started a business between surveys t−1 and t, and Φ(.) is the standard normal cumulative distribution function. xi,t−1 is a vector of control variables measured at the time of the previous survey, including whether the individual owned property, educational attainment, labour force status, sex and self-assessed financial risk appetite.[12] Quadratic polynomials in the individual's age and disposable income are also included. The models include region fixed effects (δg), which control for the possibility that some regions tend to have higher business formation rates for reasons unrelated to the housing collateral channel, but which may be associated with housing equity; for example, persistent local demand shocks may increase business formation and also drive up housing prices.[13] The models also include time fixed effects (ωt), which control for factors such as the aggregate business cycle. The models are estimated using maximum likelihood, with standard errors clustered by individual.
Because the coefficients have limited economic relevance, we present average marginal effects of a change in the explanatory variables on the probability of business formation.[14]
We use two measures of the individual's housing equity at the time of the previous survey (HEi,t−1) – the key variable of interest in the models. In Model 1, home equity is the difference between the individual's self-assessed home value and the value of outstanding loans secured against the property, including mortgages taken out to purchase the home and second mortgages, such as home equity loans. In Model 2, total housing equity is the same as home equity but also includes equity in other residential property, such as investment property and holiday homes. Data on home equity are available each year; data on the broader measure of total housing equity are available only every four years in the survey's ‘wealth modules’.
The results from Model 1 indicate that the probability of starting a business is significantly positively correlated with the dollar value of home equity, which is consistent with the existence of a housing collateral channel. A $100,000 increase in the value of home equity is associated with the probability of starting a business increasing by about 0.07 percentage points (Table 2). An increase in home equity from the 25th percentile of the 2013 distribution of home equity ($0) to the 75th percentile ($450,000) is associated with the probability of starting a business increasing by about 0.3 percentage points. While this effect may seem relatively small, it is nontrivial relative to the unconditional probability of starting a business (about 1½ per cent).
Variable | Average marginal effect (ppt) | |
---|---|---|
Model 1 | Model 2 | |
Home equity ($'00,000s) | 0.07*** | na |
Total housing equity ($'00,000s) | na | 0.06*** |
Non-housing equity ($'00,000s) | na | −0.02 |
Owner-occupier | −0.41*** | −0.36* |
Owns other property | na | 0.44** |
Age (years) | −0.01*** | −0.02*** |
Couple | 0.57*** | 0.55*** |
Income ($'0,000s) | 0.06*** | 0.07* |
Education (base: university) | ||
Certificate | 0.10 | 0.35 |
High school | −0.27** | −0.33 |
Did not complete high school | −0.42*** | −0.10 |
Female | −0.58*** | −0.60*** |
Labour force status (base: full-time) | ||
Part-time | 0.83*** | 0.86*** |
Unemployed | 1.25*** | 1.57*** |
Not in the labour force | 0.87*** | 1.04*** |
Financial risk appetite (base: substantial) | ||
Above average | −1.27*** | −0.39 |
Average | −1.87*** | −1.00 |
Not willing | −2.19*** | −1.42* |
Observations | 106,066 | 24,576 |
Pseudo–R2 | 0.063 | 0.074 |
Notes: *, ** and *** denote statistical significance at the 10, 5 and 1 per cent levels, respectively; models include quadratic terms for age and income, and region and time fixed effects; models are estimated using maximum likelihood with standard errors clustered by individual; for continuous variables, the average marginal effect is for a one unit change; for discrete variables, the average marginal effect is for a change from the base category Sources: Authors' calculations; HILDA Release 13.0 |
The results from Model 2 – that is, using data on total housing equity and controlling for non-housing equity – are similar to the results from Model 1. Notably, the coefficient on non-housing equity in Model 2 is not significantly different from zero. This is consistent with lenders being less willing (or in some cases, such as superannuation, unable) to lend for business purposes against non-housing collateral.[15] It is also consistent with the estimated effect of total housing equity reflecting a housing collateral channel rather than wealth effects.
Nevertheless, the estimated correlation between housing equity and the probability of starting a business could, to some extent, reflect factors other than a housing collateral channel. For example, Hurst and Lusardi (2004) argue that business ownership is a luxury good, which implies that households with more housing equity are more likely to start a business because they are wealthier.[16] However, if this is the case, it is unclear why non-housing equity is an insignificant predictor of business formation. One possible explanation is that individuals have better information about the value of their housing wealth relative to other types of wealth, such as superannuation. More generally, there could be common unobserved factors that drive both housing equity and the probability of starting a business. For instance, less entrepreneurial individuals may prefer to pay their mortgage down rather than use savings to finance their own business; this would tend to attenuate the correlation between housing equity and entrepreneurship even if a housing collateral channel does, in fact, exist.
A number of the control variables are statistically significant in both models. For example, the probability of starting a business is: lower for owner-occupiers than for renters; lower for females than for males; higher for couples than for other individuals; and lower for full-time workers than for other individuals. Additionally, individuals that are less willing to take financial risk are less likely to start a business.
3.2.2 Business debt
The preceding analysis found a positive correlation between housing equity and the probability of starting a business. However, the existence of a housing collateral channel implies that new entrepreneurs actually make use of their housing equity to access finance. To explore this, we investigate how the use of business debt by new entrepreneurs – that is, individuals identified as having started a business – varies with home equity.
Using the sample of new entrepreneurs, we estimate a Tobit model where the dependent
variable is business debt (dit) in thousands of
dollars.[17]
We use a Tobit model because around 80 per cent of new entrepreneurs reported
having no business debt (i.e. there is a point mass at zero). Under the Tobit
framework, a latent variable determines whether individual i has business
debt and the value of business debt conditional on having debt:
Independent variables in the model include: home equity (HEi,t−1); controls measured at the time of the previous survey (xi,t−1); and characteristics of the business measured contemporaneously with business debt (zit), such as industry and incorporation status.
The coefficients in the model give the effects of a unit change in the explanatory
variables on the latent variable . The latent variable has limited economic relevance.
Therefore, we also present the average marginal effect of a change in the explanatory
variables on dit conditional on business debt being positive
(i.e. the effect on E(dit|dit > 0)).
Estimates from the Tobit model show a statistically significant positive relationship between home equity in the year before starting a business and the value of business debt at formation (Table 3). The estimated average marginal effect indicates that a $100,000 increase in home equity is associated with business debt being about $5,000 higher. This is consistent with housing collateral facilitating access to business finance, although the effect does not appear large. The relatively small effect could be because it is difficult to obtain a small business loan in the first year after formation, regardless of the value of housing collateral. Indeed, liaison with lenders (discussed in Section 2) suggests that they are unwilling to lend to start-ups.[18]
A number of control variables also have significant correlations with the use of business debt. Notably, of new entrepreneurs with business debt, unincorporated businesses have, on average, around $25,000 less business debt than incorporated businesses.[19] The use of business debt also tends to be higher for individuals that are willing to take more risk. Additionally, new entrepreneurs in the construction, business services or household services industries tend to have lower levels of business debt relative to new entrepreneurs in the agriculture industry.
Variable | Coefficient | Average marginal effect on E(dit|dit > 0) $'000s |
|
---|---|---|---|
Home equity ($'00,000s) | 0.24*** | 0.05*** | |
Owns home | −71.05 | −14.48 | |
Age (years) | 11.85 | 0.22 | |
Age squared | −0.13 | ||
Income ($'000s) | −3.25*** | −0.49*** | |
Income squared | 7.80 × 10−3** | ||
Unincorporated | −119.60*** | −24.81** | |
Labour force status (base: full-time) | |||
Part-time | −42.66 | −8.90 | |
Unemployed | −104.84 | −18.99 | |
Not in the labour force | −151.19*** | −24.64*** | |
Financial risk appetite (base: substantial) | |||
Above average | −204.38** | −60.34** | |
Average | −212.74*** | −61.86** | |
Not willing | −231.63*** | −65.07** | |
Industry (base: agriculture) | |||
Business services | −191.91** | −56.83* | |
Construction | −211.92** | −60.44** | |
Distribution | −127.07 | −42.42 | |
Household services | −304.45*** | −72.98* | |
Manufacturing | −125.22 | −41.94 | |
Constant | 136.29 | ||
Observations | 418 | ||
Of which: left-censored (i.e. zero) |
335 | ||
Pseudo–R2 | 0.05 | ||
Notes: *, ** and *** denote statistical significance at the 10, 5 and 1 per cent levels, respectively; model estimated using maximum likelihood; for continuous variables, the average marginal effect is for a one unit change; for discrete variables, the average marginal effect is for a change from the base category Sources: Authors' calculations; HILDA Release 13.0 |
3.2.3 Business employment
A positive relationship between housing equity and the number of workers employed
by new entrepreneurs could provide evidence that some entrepreneurs are credit
constrained, in that they would choose to employ more people if they had access
to sufficient finance. We construct a variable for the number of workers employed
by new entrepreneurs using responses to two survey questions. The first question
asks about the number of workers employed in the respondent's place of
work or business, while the second question asks about the total number of
workers employed at locations of this business throughout Australia. Responses
to both questions are categorical rather than continuous. The categories of
the constructed variable are ‘sole trader’ (i.e. 1 employee), ‘2–19
employees’ and ‘20+ employees’. Since this variable is categorical
with a natural ordering, we use an ordered probit model to investigate its
relationship with home equity. Under this framework, the number of employees
(Eit) is determined by the value of a continuous latent
variable relative to thresholds
µ1 and µ2:
The explanatory variable of interest is home equity at the time of the previous survey (HEi,t−1). Other explanatory variables include controls measured at the time of the previous survey (xi,t−1) and characteristics of the business measured contemporaneously with the number of employees (zit).
Table 4 presents the estimated coefficients from the model along with associated average marginal effects. The estimated coefficient on home equity is positive and statistically significant, although the marginal effects are relatively small. The estimated marginal effects suggest that, conditional on starting a business, new entrepreneurs with access to more home equity are less likely to be sole traders and more likely to have employees. For instance, a $100,000 increase in home equity is associated with: the probability of being a sole trader decreasing by about 0.7 percentage points; the probability of employing 2–19 employees increasing by 0.5 percentage points; and the probability of employing more than 20 employees increasing by about 0.3 percentage points. These results are consistent with the existence of a housing collateral channel, in that access to housing collateral allows new entrepreneurs to start businesses with a greater number of employees.
Variable | Coefficient | Average marginal effect (ppt) | ||
---|---|---|---|---|
Pr(Sole trader) | Pr(2–19) | Pr(20+) | ||
Home equity ($'00,000s) | 0.03*** | −0.70*** | 0.45*** | 0.25*** |
Owns home | 0.05 | −1.39 | 0.90 | 0.50 |
Age (years) | 0.02 | −0.06 | 0.04 | 0.02 |
Age squared | −2.17 × 10−4 | |||
Unincorporated | −0.97*** | 32.53*** | −19.46*** | −13.07*** |
Labour force status (base: full-time) | ||||
Part-time | −0.12 | 3.46 | −2.19 | −1.27 |
Unemployed | −0.57*** | 14.13*** | −9.65*** | −4.48*** |
Not in the labour force | −0.34*** | 9.19*** | −6.06*** | −3.13*** |
Industry (base: agriculture) | ||||
Business services | −0.44*** | 12.93*** | −7.93*** | −5.00*** |
Construction | −0.54*** | 15.25*** | −9.53*** | −5.73*** |
Distribution | −0.21 | 6.49 | −3.78 | −2.71 |
Household services | −0.24* | 7.40* | −4.34* | −3.06 |
Manufacturing | −0.22 | 6.91 | −4.04 | −2.87 |
µ1 | 0.04 | |||
µ2 | 1.06*** | |||
Observations | 1,845 | |||
Pseudo–R2 | 0.11 | |||
Notes: *, ** and *** denote statistical significance at the 10, 5 and 1 per cent levels, respectively; model estimated using maximum likelihood; for continuous variables, the average marginal effect is for a one unit change; for discrete variables, the average marginal effect is for a change from the base category Sources: Authors’ calculations; HILDA Release 13.0 |
4. Evidence from Postcode-level Data
In this section, we examine the correlation between housing prices and entrepreneurship at a more aggregated level. This serves as a useful crosscheck for the results obtained using household survey data in Section 3. We measure entrepreneurship in terms of the ‘company entry rate’ – the number of new companies formed (as a share of existing companies).
As a first pass, there is a reasonably strong positive correlation between housing price growth and the company entry rate across states and time (Figure 6).[20]
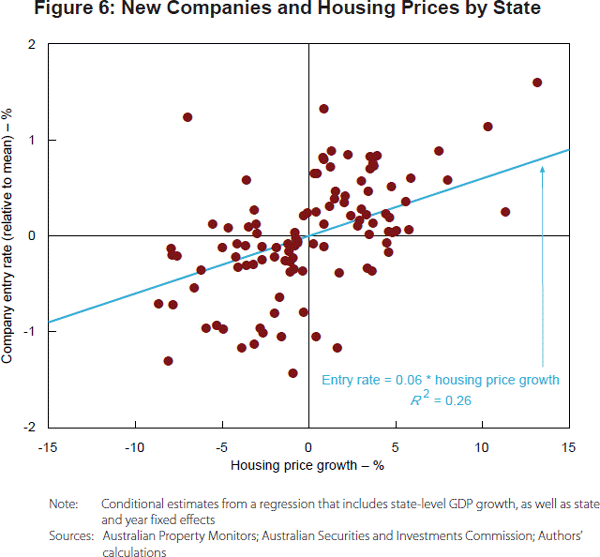
In the remainder of this section, we take this idea further and examine the correlation at the postcode level using a newly constructed dataset that links housing prices and company entry rates. The highly disaggregated nature of the dataset allows us to control for a wide range of factors in examining the link between housing prices and entrepreneurship.
4.1 Data
We construct our postcode-level dataset using monthly information from the Australian Securities and Investments Commission (ASIC) company registry (obtained via Dun and Bradstreet). The registry provides information for each individual company registered in Australia including:
- the name of the company
- the registration date
- the deregistration date (if any)
- the postcode in which the company's headquarters are located
- the four-digit Australian and New Zealand Standard Industrial Classification (ANZSIC) code.
Using Australian company number identifiers, we match this registry with information for each company on the number of employees provided by Dun and Bradstreet. From this matched dataset we construct annual estimates of the total number of companies, as well as entry and exit rates, by postcode across six separate size classes (from sole traders to very large companies) and for each 1-digit ANZSIC industry division (e.g. manufacturing, mining, retail trade, etc). We aggregate the data at the 1-digit industry division because the sample sizes based on finer industry splits are too small for regression analysis. We focus on the period from 1999/2000 to 2010/11 because we do not have the relevant information on postcode location and employment before or after this time.
We match the company entry and exit data to (hedonically adjusted) housing prices at the postcode level. These data are provided by Australian Property Monitors (APM) and have been used in several recent Reserve Bank publications (e.g. Genesove and Hansen 2014; Read, Stewart and La Cava 2014; Windsor, La Cava and Hansen 2014). The housing price data are only available for Sydney, Melbourne and Brisbane, so this limits the sample of postcodes.
Summary statistics for some of the key variables used in the regression modelling are shown in Table 5.
Mean | Std dev | P25 | Median | P75 | |
---|---|---|---|---|---|
Company entry rate (%) | 25.0 | 29.4 | 7.1 | 14.3 | 33.3 |
Housing prices (log level) | 12.3 | 0.7 | 11.8 | 12.3 | 12.8 |
Average personal income (log level) | 10.8 | 0.3 | 10.6 | 10.8 | 11.0 |
Population size (log level) | 9.2 | 0.7 | 8.9 | 9.3 | 9.7 |
Sources: APM; ASIC; ATO; Authors' calculations |
4.2 Identification
Our identification strategy exploits variation in the level of housing prices and entrepreneurial activity across postcodes and over time. The tests of the housing collateral channel are similar in spirit to those used by Adelino et al (2013). To begin, we estimate the baseline regression:
The dependent variable (ENTRY) is the company entry rate (the number of company entries during the year divided by the stock of companies at the start of the year) for company size category i in postcode p in industry division s in financial year t. The key independent variable of interest is the log level of housing prices (ln(HP)) for each postcode and year.[21] The specification includes a set of control variables (Xpt) for each postcode and year, such as the average level of persona income and population size. These data are obtained from the ATO. The model also includes several fixed effects:
- Size fixed effects (α) control for time-invariant factors that drive entry decisions for different company size categories (e.g. the fixed costs of starting a company).
- Postcode fixed effects (μ) control for time-invariant factors that might explain company entry rates across different postcodes (e.g. distance from CBD, zoning regulations).
- Industry fixed effects (π) control for time-invariant factors that determine entry rates across different industries (e.g. capital intensity).
- Time fixed effects (θ) control for national cycles in business, housing and financial conditions.
If a housing collateral channel is in force, we expect a positive coefficient on housing prices (β > 0). However, it is not enough to demonstrate a positive correlation between the level of housing prices and the company entry rate to establish a causal link between housing prices and entrepreneurship. Moreover, even if we could establish a causal relationship, higher local housing prices could affect entrepreneurship through mechanisms other than an increase in potential borrowing capacity. First, and as noted in Section 3.2.1, an increase in housing prices will increase the wealth of local home owners, which could encourage them to engage in more risk-taking activities, such as starting a business (‘the wealth channel’). This could be because wealthier individuals are more willing to take risk or because entrepreneurship is a ‘luxury good’ (Hurst and Lusardi 2004).[22] Second, the increase in housing wealth could encourage home owners to spend more and the increase in local demand could, in turn, encourage local residents to start businesses (‘the local demand channel’).
To partly address these challenges, we tweak the model to allow housing prices to have differential effects on the entry rates of small and large companies:
Specifically, in addition to the control variables and fixed effects included previously, this model includes a dummy variable (SMALL) that equals one if the company size category is small (less than 20 employees) and zero otherwise.[23] More importantly, it also includes an interaction between the dummy variable for small companies and the level of housing prices (SMALL*ln(HP)). If a collateral channel is in force and small companies are more credit constrained than large companies, the coefficient on the interaction term should be positive and significant (γ > 0).
Furthermore, if the housing collateral channel exists, the correlation between housing prices and entry rates should be particularly strong for small companies in industries that are typically dependent on housing collateral. To test this, we add further interaction terms to the specification:
The model now includes a dummy variable (DEPEND) that equals one if the industry typically relies on housing collateral for start-up capital and zero otherwise. It also includes the interaction with the level of house prices (DEPEND*ln(HP)), as well as the small company indicator dummy and level of house prices (DEPEND*SMALL*ln(HP)).
We define ‘collateral-dependent’ industries as those that have a relatively high share of small businesses dependent on home equity loans for start-up capital. This information comes from publicly available unit-record data in a US Census Bureau survey of small business owners.[24] In the survey, small business owners are asked how they originally financed their business (e.g. bank debt, personal guarantee, etc). A loan collateralised by home equity is one of the options for respondents. Respondents are also asked about the industry in which they operate. For each industry, we calculate the share of small business owners that report using a home equity loan for start-up capital. The classification of industries according to the reliance on housing collateral is shown in Figure 7.[25] We denote the top five industries as ‘collateral dependent’. These are: accommodation and food services; manufacturing; wholesale trade; retail trade; and rental, hiring and real estate services.[26] Admittedly, the choice of industries is ad hoc. Moreover, it is not clear if the industry pattern for the United States necessarily corresponds to the industry pattern for Australia. Nevertheless, we have experimented with different classifications and get very similar results.[27]
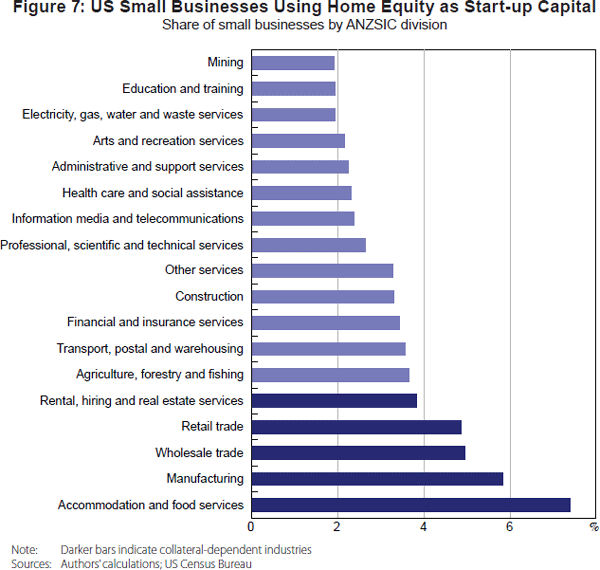
Finally, if the local demand channel is important, then the effect of a rise in housing prices on start-up activity should be stronger for businesses in ‘non-tradeable’ industries that are particularly exposed to local demand, such as restaurants, cafes and other small retail outlets. We re-specify the model as:
This model includes a dummy variable (LOCAL) that equals one if the industry is non-tradeable and typically focused on local demand and zero otherwise. It also includes the interaction with the level of house prices (LOCAL*ln(HP)) and both the small business indicator dummy and level of house prices (LOCAL*SMALL*ln(HP)). We define ‘non-tradeable’ industries as industries within the retail trade, accommodation and food services or business and property services sectors. This model specification is inspired by a similar test used by Mian and Sufi (2014).
To summarise, we test four separate hypotheses:
- there is a positive correlation between the level of housing prices and company entry rates
- the correlation is stronger for small companies than for large companies
- the correlation is stronger for small companies in industries that are dependent on housing collateral to start a business than for small companies in other industries
- the correlation is stronger for ‘non-tradeable’ small companies than for other small companies.
A rejection of the null hypothesis for each of the first three hypotheses is evidence consistent with the housing collateral channel. A rejection of the fourth null hypothesis would be evidence in favour of the local demand channel (and against the housing collateral channel). However, none of these hypotheses are able to directly test the collateral channel against the wealth channel.
4.3 Results
The results of estimating Equations (4) to (7) are shown in Table 6. The baseline results from estimating Equation (4) indicate that the level of housing prices is positively correlated with the company entry rate. An increase in housing prices from the 25th to the 75th percentile of the distribution (which roughly implies a trebling of housing prices) is associated with the company entry rate increasing by about 260 basis points. This would be associated with the company entry rate rising from a median of 14.3 per cent to about 16.9 per cent, on average.
Equation (4) | Equation (5) | Equation (6) | Equation (7) | |
---|---|---|---|---|
Housing prices | 0.025* (1.69) |
−0.029 (−1.36) |
−0.029 (−1.32) |
−0.025 (−1.19) |
Small company x housing prices |
0.056*** (3.60) |
0.059*** (3.60) |
0.056*** (3.58) |
|
Collateral-dependent industry x housing prices |
−0.015 (−1.52) |
|||
Collateral-dependent industry x small company x housing prices |
0.003 (1.40) |
|||
Non-tradeable industry x housing prices |
−0.032*** (−6.68) |
|||
Non-tradeable industry x small company x housing prices |
0.002 (0.74) |
|||
Average personal income | −0.028 (−1.01) |
−0.026 (−0.94) |
−0.027 (−0.98) |
−0.021 (−0.77) |
Population size | −0.023** (−2.11) |
−0.021** (−1.99) |
−0.022** (−2.06) |
−0.023** (−2.14) |
Observations | 38,640 | 38,640 | 38,640 | 38,640 |
R2 | 24.3% | 24.3% | 24.4% | 24.6% |
Notes: *, ** and *** denote statistical significance at the 10, 5 and 1 per cent levels, respectively; t-statistics are reported in parentheses; standard errors are clustered by postcode; all specifications include postcode, industry, year and size category fixed effects (which are all omitted from the table) Sources: ABS; APM; ASIC; ATO; Authors' calculations; US Census Bureau |
The results of estimating Equation (5) imply that the positive correlation between entry rates and housing prices is solely due to small companies. This is shown by the positive interaction effect and the insignificant (negative) main effect. Together, these results are consistent with a housing collateral effect if small companies are more likely to be credit constrained.[28] The positive association between the level of housing prices and the small company entry rate is more than twice as large as for all companies. Moreover, the economic effect seems quite large compared with the results obtained from the household survey model. This may reflect the fact that we are not directly controlling for wealth shocks that may vary across postcodes and over time. In other words, the postcode-level estimates could be affected by omitted variables bias.
The results of estimating Equation (6) indicate that the positive correlation between housing prices and small company entry rates is slightly stronger in collateral-dependent industries. This is shown by the positive coefficient on the interaction term for collateral-dependent small businesses. However, the difference is not statistically significant and, therefore, provides only tentative support for the collateral channel. The lack of statistical significance could reflect the fact that the split between collateral-dependent and independent industries is somewhat arbitrary.
Finally, the results of estimating Equation (7) indicate that the positive correlation between housing prices and entry rates is not specific to non-tradeable industries that are dependent on local demand. This finding supports the collateral channel to the extent that it does not provide strong support for the competing hypothesis of the local demand channel.
For completeness, we find that entry rates are higher in areas with smaller populations, on average. There is no correlation between entry rates and average personal income levels.
5. Conclusions
This paper has explored the relationship between housing collateral and entrepreneurship in Australia from two angles: first, we interviewed small business finance experts at a range of lending institutions to better understand the role of collateral in small business lending; and second, we econometrically tested the relationship between measures of housing collateral and entrepreneurial activity using two different datasets.
The key messages we took away from the liaison with lenders was that the provision of housing collateral influences small business lending decisions. While the lenders naturally place much more focus on the capacity of the potential borrower to service the loan, providing housing collateral is viewed by some lenders as evidence that the borrower has the capacity to build wealth and has strong incentives to repay the loan. Where the presence of housing collateral appears to be particularly important is in determining the terms of the loan, with lenders willing to lend larger amounts at lower interest rates against residential security. Consistent with these facts, all the lenders indicated that a high proportion of their small business lending was collateralised.
The liaison also highlighted the challenges that start-up businesses face in accessing intermediated lending products. Start-ups face a high hurdle to demonstrate their capacity to repay the loan without a cash-flow history. While the lenders emphasised that the provision of collateral was not sufficient of itself to justify lending to a start-up without evidence of serviceability, it nevertheless increased the likelihood of lending. Looking ahead, young entrepreneurs may find it more difficult to access debt finance given the high level of house prices relative to incomes, which could restrict their capacity to build the housing equity necessary to access residential-secured small business lending products.
Econometric models estimated using Australian household survey data yield evidence consistent with the existence of a housing collateral channel, although the estimated effects are relatively small. Specifically, the dollar value of housing equity is positively correlated with: business formation; the use of business debt conditional on business formation; and the number of workers employed by new entrepreneurs. One result that further supports the existence of a housing collateral channel is that, unlike housing equity, non-housing equity seems to be insignificant in predicting business formation. This is also consistent with lenders being less willing to lend for business purposes against non-housing collateral. However, we cannot discount the possibility that our results reflect wealth effects. This might be the case if, for instance, entrepreneurial risk appetite is a function of housing wealth but not non-housing wealth.
We find supporting evidence of a positive correlation between housing prices and entrepreneurship using a completely different dataset based on Australian company registrations. In particular, we find a clear and robust positive correlation between the level of housing prices and the share of new companies measured across postcodes. The correlation is only apparent for small companies and appears to be relatively broad based across industries.
In the absence of a natural experiment (as in Jensen et al (2014)), it is difficult to disentangle the housing collateral channel from wealth effects. Nevertheless, our results are relevant for policy. In terms of understanding the monetary policy transmission mechanism, a decrease in interest rates may have less impact through the housing collateral channel if lenders' balance sheets were to become impaired or if their lending were to become constrained by capital requirements. If a housing collateral channel exists, it too may have distributional effects because entrepreneurs that do not own their own homes are adversely affected by a rise in housing prices relative to those entrepreneurs that own their homes. This should be taken into account when designing policies aimed at influencing home ownership rates or entrepreneurs' access to business finance.
There are many avenues for further research on the housing collateral channel in Australia. While this paper has focused on the relationship between housing collateral and business formation, we have not explored the effect on the outcomes for existing businesses. While the effects found in this paper seem reasonably small in general, this is consistent with the liaison with lenders, which confirmed that new businesses have more difficulty accessing finance, regardless of their housing collateral. It may be the case that the housing collateral channel is more important for the activity of existing businesses. As the time series from the HILDA Survey grows, it would be worthwhile examining the effect of housing collateral over the life cycle of a small business.
Footnotes
The authors are from the Reserve Bank of Australia. The authors would like to thank Jon Cheshire and Ani Yadav for their outstanding contribution to the liaison with lenders undertaken for this paper. The authors would also like to thank David Hargreaves, Angus Moore and John Simon for useful feedback. [*]
A similar mechanism underlies some macroeconomic models with financial frictions (for example, see Bernanke and Gertler (1989) and Kiyotaki and Moore (1997)). [1]
To the best of our knowledge, there are no Australian studies that quantify the contribution of either small or young businesses to job creation and output growth. For evidence for the United States, see Haltiwanger, Jarmin and Miranda (2013). [2]
Collateral is particularly important in rural lending, with virtually all rural lending collateralised. This partly reflects the fact that the quality of the farmland offered as collateral also directly affects the productivity and profitability of the business, influencing the entrepreneur's capacity to repay. [3]
The application of relatively conservative loan-to-valuation ratios was particularly prevalent in lending to rural businesses, where loan-to-valuation ratios were often capped at 30–50 per cent. [4]
Rural lending specialists also noted that the value of land as collateral was particularly important for pricing rural loans given that the volatility of cash flows increases the risk of lending to the sector. [5]
For more details on the HILDA Survey, see Section 3.1 below. [6]
This concern was also raised by entrepreneurs at the small business finance roundtable hosted by the Reserve Bank in 2012. For more details, see RBA (2012). [7]
For instance, see Commonwealth Bank of Australia v Doggett [2014] VSC 423 and Fast Fix Loans Pty Ltd v Samardzic [2011] NSWCA 260. [8]
Lenders noted that there is an element of cross-subsidisation inherent in the home loan portfolio that actually favours small business borrowers, given that there is little differentiation between the interest rates charged on home loans to small business entrepreneurs compared with other households. [9]
For comparison, ABS data indicate that around 6 per cent of individuals aged over 15 years owned their own business in 2013. [10]
These individuals possibly work multiple jobs and alternate between reporting their own business and another (employee) job as their primary job. Including previous business owners results in housing equity having a larger estimated marginal effect on the probability of business formation. [11]
The financial risk appetite variable is not available in 2005, 2007 and 2009. For these years, financial risk appetite is imputed using responses (where available) from the neighbouring surveys. [12]
The region fixed effects are at the Statistical Division (SD) level. The SD is an Australian Standard Geographical Classification area, of which there are around 60. The results are similar when using a linear probability model and region-time fixed effects, which should be better able to control for transitory local demand shocks. We were not able to estimate a probit version of this model because of issues relating to numerical estimation caused by the very large number of dummy variables representing the fixed effects. [13]
Average marginal effects use the observed values of the covariates in the sample to calculate marginal effects for each individual. These are averaged over the sample to yield average marginal effects. For discussions of this approach to calculating marginal effects, see Wooldridge (2010) and Greene (2012). [14]
Splitting non-housing wealth into superannuation assets and other non-housing non-superannuation wealth yields: an essentially unchanged marginal effect for total housing equity; a statistically significant and negative marginal effect for superannuation assets; and an insignificant marginal effect for non-housing non-superannuation wealth. [15]
To test whether the effect of housing equity on business formation differs across the wealth distribution, we estimated a version of Model 2 where housing equity is interacted with a set of dummy variables representing the quintiles of total household wealth. There were no significant differences between the coefficients on the interaction terms, suggesting that the response of business formation to housing equity does not vary over the wealth distribution. [16]
The sample is restricted to new entrepreneurs in 2002, 2006 and 2010 because data on business debt are only collected in the wealth modules. Note that inferences based on models estimated using the sample of new entrepreneurs are conditional on selection into that sample. Future work could potentially consider the effect of sample selection on the results. [17]
Rather than taking out a business loan, new entrepreneurs may finance their business by directly drawing down on housing equity using housing-related lending products, such as home equity loans. A linear regression of the change in housing debt between consecutive surveys on a dummy variable for whether the individual started a business (and other controls) suggests that the change in housing debt is, on average, about $5,000 larger for new entrepreneurs than for other individuals, although the difference is not significant. [18]
This may be a measurement issue, since an unincorporated business is intrinsically more intertwined with the personal finances of the entrepreneur than an incorporated business. [19]
The scatterplot shows the conditional correlation between the entry rate and housing price growth. This is based on a regression of the company entry rate on growth in housing prices and growth in GDP by state and time. The regression also includes state and year fixed effects to control for factors such as state-level business regulations and monetary policy. To draw the figure, we use the Frisch-Waugh theorem and estimate two separate regressions – one of the entry rate on GDP growth, state and year fixed effects and then another of housing price growth on the same control variables. We take the residuals from these two regressions and draw them against each other in the scatterplot. [20]
We also experimented with the growth rate of housing prices as the key independent variable. None of the key results were affected by this change in model specification. [21]
Wealthier individuals can afford to ‘purchase’ the non-pecuniary benefits associated with business ownership, such as decision-making power and flexible time schedules. [22]
This dummy variable drops out of the specification because it is perfectly collinear with the size fixed effects, leaving just the interaction dummy variable. [23]
Kleiner (2015) uses these data to examine the effect of house price growth on entrepreneurial finance in the United States. [24]
Conveniently, the classification of NAICS 1-digit industry divisions is essentially equivalent to the ANZSIC 1-digit industry divisions, which makes it easy to map directly to the Australian data. [25]
We also used the US unit-record data to estimate a linear probability model for whether the owner initially financed their business with home equity or not. The regression model included many owner characteristics that might drive this decision (e.g. age, gender, race, geographic location) and, most importantly, industry dummies. The coefficients on these dummies provide estimates of how dependent each industry is on home equity, conditional on other factors. This conditional industry classification was very similar to the unconditional industry classification. [26]
Two Australian banks – CBA and Westpac – provide information on the industry portfolio of their small business lending (and whether it is collateralised by residential property or not). This information is available in their Pillar 3 reports. Broadly speaking, the Australian data supports the chosen industry classification based on the US data. [27]
However, large companies may also have multiple locations and this geographic diversification may lower the sensitivity of their entry rate decision to local housing prices. [28]
References
Adelino M, A Schoar and F Severino (2013), ‘House Prices, Collateral and Self-Employment’, NBER Working Paper No 18868.
APRA (Australian Prudential Regulation Authority) (2008), ‘Prudential Standard APS 113: Capital Adequacy: Internal Ratings-Based Approach to Credit Risk’. Available at <http://www.apra.gov.au/adi/Documents/cfdocs/Final-APS-113-November-2007.pdf>.
Bernanke B and M Gertler (1989), ‘Agency Costs, Net Worth, and Business Fluctuations’, The American Economic Review, 79(1), pp 14–31.
Corradin S and A Popov (2013), ‘House Prices, Home Equity and Entrepreneurships’, European Central Bank Working Paper Series No 1544.
Fort TC, J Haltiwanger, RS Jarmin and J Miranda (2013), ‘How Firms Respond to Business Cycles: The Role of Firm Age and Firm Size’, NBER Working Paper Series No 19134.
Genesove D and J Hansen (2014), ‘Predicting Dwelling Prices with Consideration of the Sales Mechanism’, RBA Research Discussion Paper No 2014-09.
Greene WH (2012), Econometric Analysis, 7th edn, Prentice Hall, Upper Saddle River, pp 696–699.
Haltiwanger J, RS Jarmin and J Miranda (2013), ‘Who Creates Jobs? Small Versus Large Versus Young’, The Review of Economics and Statistics, 95(2), pp 347–361.
Hurst E and A Lusardi (2004), ‘Liquidity Constraints, Household Wealth, and Entrepreneurship’, Journal of Political Economy, 112(2), pp 319–347.
Jensen TL, S Leth-Petersen and R Nanda (2014), ‘Housing Collateral, Credit Constraints and Entrepreneurship – Evidence from a Mortgage Reform’, NBER Working Paper Series No 20583.
Kerr SP, WR Kerr and R Nanda (2014), ‘House Money and Entrepreneurship’, Paper presented at Fordham Graduate School of Business, Center for Research in Contemporary Finance, Finance Research Seminar, New York, 21 November.
Kiyotaki N and J Moore (1997), ‘Credit Cycles’, Journal of Political Economy, 105(2), pp 211–248.
Kleiner K (2015), ‘Does Collateral Drive Access to Formal Lending Markets? Evidence from Home Equity Loans’, Unpublished manuscript, Indiana University, Kelley School of Business. Available at <http://kelley.iu.edu/kleinerk/Home_Equity_Kleiner.pdf>.
Mian A and A Sufi (2014), ‘What Explains the 2007–2009 Drop in Employment?’, Econometrica, 82(6), pp 2197–2223.
OECD (Organisation for Economic Co-operation and Development) (2013), Financing SMEs and Entrepreneurs 2013: An OECD Scoreboard, OECD Publishing, Paris.
RBA (Reserve Bank of Australia) (2012), ‘Small Business Finance Roundtable: Summary of Discussion’, RBA Bulletin, June, pp 91–94.
Read M, C Stewart and G La Cava (2014), ‘Mortgage-Related Financial Difficulties: Evidence from Australian Micro-Level Data’, RBA Research Discussion Paper No 2014-13.
Schmalz MC, DA Sraer and D Thesmar (2013), ‘Housing Collateral and Entrepreneurship’, NBER Working Paper Series No 19680.
Windsor C, G La Cava and J Hansen (2014), ‘Home Price Beliefs in Australia’, RBA Research Discussion Paper No 2014-04.
Wooldridge JM (2010), Econometric Analysis of Cross Section and Panel Data, 2nd edn, MIT Press, Cambridge, pp 577–578.