Speech Economic Forecasting and Its Role in Making Monetary Policy

Glenn Stevens
Assistant Governor (Economic)
Address to Economic Society of Australia Forecasting Conference
Melbourne –
My first experience in making forecasts was in the early 1980s, when I worked in a section of the Reserve Bank that was responsible for forecasting growth in the M3 measure of money. In those days, of course, we had a target – officially called a ‘conditional projection’ – for M3, following the trend in many countries at that time. For forecasting, we used a device known as a formation table, which was basically an accounting identity which rearranged the balance sheets of the Reserve Bank and the private sector, so that M3 appeared at the bottom. Some key bits of behaviour had to be guessed, like how much banks would expand their loans and how much government debt would be taken up by the public and a few other things. We became very good at re-arranging numbers in the various columns and rows to produce various forecasts. (There were no spreadsheets then of course – forecasts were done using a hand calculator, or in one's head.) What was striking, as I (admittedly dimly) remember, was that we always ended up with a forecast that M3 would grow by 10 per cent! Every number in the table would change, except that one. Then, quite late in the financial year, as it became all too obvious that the result would be different, we came up with a different forecast.
The process of forecasting has perhaps moved on a bit since then. (We certainly do not make forecasts of M3 any more – possibly to the dismay of some who still think in quantity theory terms.) I've had the chance to make a lot more forecasts which were wrong, and to re-learn the lesson that no matter what the effort and apparent science that goes into filling out a table of numbers, it is all to no avail if the figure in the bottom line is wrong.
With a few scars from that background, I intend to avoid making a forecast today, and to try instead to say something of interest about the forecasting process itself, and how useful it is. In particular, I want to make some remarks about the usefulness of forecasts in the monetary policy decision process.
My comments are not particularly profound. They are prompted by a certain amusement (or occasionally frustration) at the almost obsessive focus on particular numbers in official forecasts, revisions to them and errors in them, which make up much of the public discussion at particular points during the year. ‘Are the Budget forecasts too low/high? Will they be revised? What will the Government do now?’ And so on. Often, I think this sort of discussion misses the point about the real role of forecasts in policy-making.
This is not to say that serious forecasting errors do not have consequences – they clearly do. Macroeconomic policies have to be framed in a forecast context, and if the forecasts are badly astray then there is a high chance that the policies so set will be less than ideal, and perhaps much less. But a lot of discussion of forecast mistakes is about errors which are well within the standard errors for these processes, and which really don't matter much for policy purposes. At the same time, one of the key objectives of good policy-making is to try to lessen the sensitivity of outcomes to forecast errors as much as possible, and in particular to minimise the probability of a really bad outcome.
In grappling with this I first want to talk about the various purposes for which forecasts might be made. I will then review what the economics profession has found about forecast accuracy. Next I will make some observations about forecasts for the purposes of monetary policy-making, and finally say something about the monetary policy decision process – which doesn't by any means follow automatically from a particular forecast.
Purposes of Forecasts
Forecasts are made for various reasons. Governments make economic forecasts for the purposes of framing a Budget. They want to know how much revenue is likely to be available within a given period, so that the process of allocating public resources can be conducted properly, requisite amounts of borrowing arranged and so on. In this context, a reasonable degree of accuracy for year-average outcomes for key parameters – output, wage incomes, prices – is most of what is needed. Whether the actual forecasts for particular expenditure sub-components are quite right is less important. Year-average outcomes, moreover, can often be forecast with a reasonable degree of accuracy once the outcomes for the preceding year are known, unless the economy reaches a sharp turning point early in the year being forecast. These sorts of forecasts are reasonably robust to minor fluctuations from quarter to quarter in the national accounts data, though they can be sensitive to substantial data revisions. In fact, in my experience one of the most important forces producing forecast revisions and errors for year-average forecasts is revisions to history, especially to the pattern of quarterly growth near the end of the year before the one being forecast.
Other forecasts are made with a view to selling a product, or a piece of advice. I hope you will forgive me for saying that, as far as I can tell, many forecasts made in the private sector are essentially of this variety. The forecaster has a story to tell in order to provide credibility to their employer's efforts to win business. There is nothing wrong with that – there's a market for advice and various other services, and competition within that market, and it's up to the purchasers of those services to decide how much to buy. The point is simply that these sorts of forecasts are made with a different objective than ‘official’ forecasts.
Yet other forecasts are made so as to enable decision-makers to make well-informed decisions or to take precautions. Forecasts of the weather perhaps fall into this category: if I am engaged in some sort of outdoor activity, a look at a forecast of rain may prompt me to take the precaution of carrying some different clothing or an umbrella, even if the weather is fine at present. If forecast rain does not arrive, I have suffered the minor inconvenience of carrying things I do not need; but the cost of not heeding a correct forecast might have been much higher. If the forecast is for extremely bad weather, I may change my plans completely and stay indoors – and still feel I have made a good decision even if the weather does not turn out quite as bad as forecast.
Forecasts made for the purposes of monetary policy have some of the last of these characteristics. The forecast can alert policy-makers to the need to consider precautionary policy changes. Where the analogy breaks down, of course, is that weather forecasters can't, by inducing people to carry an umbrella, reduce the chance of rain. But forecasts made for policy purposes might evoke a response from policy-makers which makes the outcome different from what was forecast. This adds an extra dimension to the process of making such forecasts, and can sometimes make comparison of those forecasts with others difficult.
How Good are Forecasts?
There is something of a literature on assessing forecasting accuracy. As far back as the early 1970s, US economists debated the accuracy of various kinds of model-based forecasts. During the 1980s, and again in the early 1990s, there was a series of studies looking at the accuracy of international forecasts, particularly, but not only, those of bodies such as the OECD and IMF. A tendency for more intense discussion of forecast quality to occur following serious recessions is quite discernible.[1]
A reasonable, though not exhaustive, summary of the main findings of these various studies is as follows:
- Forecasts were generally a bit better than simple extrapolation
- There appears to be no basis for claiming that any one forecaster is consistently superior (there is some evidence that using the average of all the forecasts is a superior strategy). One contrary finding by Romer and Romer (1996) is that the Federal Reserve's internal forecasts of inflation were consistently better than those of commercial forecasters.
- Extreme movements in variables were generally poorly forecast. It is not uncommon for outcomes to be well outside the range defined by the highest and lowest individual forecast.
- Forecasters do not do well around turning points, but it is not only cyclical turning points which are hard to handle. Large and persistent changes in trend growth or inflation have been hard for forecasters to cope with. As Macfarlane and Hawkins (1993) point out, the forecasting community seriously failed to predict the extent of the increase in inflation in the early and mid 1970s, or the duration of the recession in the late 1970s/early 1980s.
As a result of events of the 1990s, we have a few additional bits of evidence we can offer in support of the last of these findings. Few, if any, predicted accurately the chronic weakness of the Japanese economy through the 1990s, with an average rate of GDP growth of about 1 per cent, one-quarter of its average growth in the preceding 20 years. This ranks alongside the substantial slowdown in productivity growth in the western industrialised economies in the mid 1970s as a major shift in trend. Moreover, if the recent unexpected strength in Japanese GDP turns out to be a foretaste of even a modest expansion in Japanese economic activity during the next couple of years, then that will be another turning point which almost everyone missed.
Another example is the US economy's remarkable performance over recent years. That the growth was a surprise to most forecasters is illustrated clearly in Graph 1, which shows the annual outcomes for US growth, compared with forecasts published by Consensus Economics, with the shaded area being the range implied by the highest and lowest forecast each year. The forecasts are made at the end of the preceding calendar year in each case. Since the forecasts are on a year-average basis, the forecasters have a certain advantage, in that by the end of the previous year, a good deal of the annual average outcome for the forecast year is more or less in the bag, unless there are dramatic developments.
In most years, the forecasts were not too bad. It is clear that the growth in the past couple of years, however, has been higher than even the most optimistic forecast. The latest Consensus forecast for the US in 1999, moreover (not shown on the graph) is for growth at about the same pace as in 1998. If that turns out to be correct, it will be the third time in succession that growth has been outside the band shown here. Of course, some of these forecasts of reduced growth relied on assumed tighter US monetary policy. Interestingly, forecasts for US inflation made by the same group of forecasters have been closer to the mark – though one wonders how those forecasts might have been different had they known the actual outcomes for GDP growth and interest rates in advance.
It is also important to record that, while the forecasts of policy-makers in the US were not all that different from the private consensus, forecast errors do not seem to have led them into significant policy error, at least not as far as we can tell at this stage. This suggests that the US policy-makers, while I am sure using the forecasts in their deliberations, were not simply reacting automatically either to the forecasts or to the observed errors in forecasts – an important point to which I return shortly.
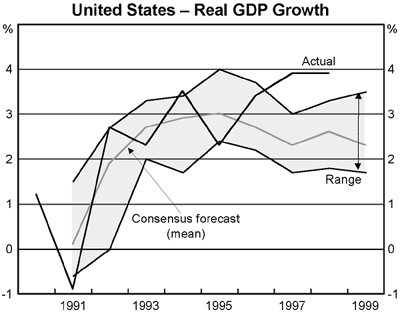
Generally speaking, then, forecasting is a difficult process. The evidence over a longish period is that economic forecasts do add some value, but as a profession we still have a fair bit to be modest about.
Forecasts for Monetary Policy
The above discussion suggests that the hazards involved in forecasting are such that perhaps the policy-makers could be forgiven for viewing them with considerable scepticism. And frequently they do.
But whether we like it or not, forecasts – however arrived at – must unavoidably be at the heart of the process of forming advice on monetary policy. The fact that forecasting is hard and often apparently unrewarding work does not absolve us from putting substantial effort into it. The most obvious reason is that the long lags associated with the full impact of monetary policy changes mean that policy changes today must be made with a view not just to what is happening now, but what is likely to be happening in a year's time and even beyond then.
We are often asked the simple question of what are the lags in the economy's responses to interest rate changes. Being economists, our answer is usually ‘it depends on what else is happening’. But the econometric evidence from modelling we have done, for what that is worth, suggests that the mean lag on the effect of interest rate changes on activity is about five or six quarters. That is, after a year and a half, we have seen half the full effect of the policy change. But effects are still occurring, albeit smaller ones, in the third year after a policy adjustment. These results are the average outcomes over a sample period of 15 years or so; in different episodes the speed and size of effects could obviously differ. But the point is that the lags are fairly long, and so making forecasts is an unavoidable element of the policy adviser's job.
The lags in the full effects on inflation are probably somewhat longer than those to activity. This heightens the need to think ahead – to make a forecast - especially in the case where policy is centred, as in Australia, around a numerical inflation target. This is why inflation targeting is, as Lars Svensson has made clear in the academic literature, inflation forecast targeting. The central bank makes a promise that it will adjust its instrument such that inflation in expectation is at the target at the end of a suitable horizon. That horizon has to take into account the policy lags: if we are significantly off target today, there is no point promising to be back there next quarter.
So we have to make and use forecasts, with due acknowledgment that forecasting is, to say the least, a very imprecise process. This much is, I think, well understood.
What perhaps needs better articulation is the nature of the decision-making process which uses those forecasts. Policy-making, whether it be inflation targeting or some other discretionary regime, is sometimes thought of as a process like the following:
- ask the advisers for a forecast based on a ‘no policy change’ assumption;
- assess the forecast deviation of outcomes from desired levels;
- ask the adviser what size shift in the instrument would be required to deliver an outcome consistent with what is desired; and
- make that change.
Everyone who has been involved in the policy process knows, however, that there is more to it than that. Decision-makers are not convinced merely by a forecast to jump immediately to a decision – partly because many of them used to be forecasters, and so they know how unreliable forecasts sometimes are! I have yet to see a policy argument won on the basis of the presentation of a printout from a forecasting exercise.[2]
Those involved in forecasting sometimes find this frustrating. But the reason why policy-makers often do not simply accept a forecast, and its associated message, at face value is not sheer stubbornness. It is uncertainty. Put simply, the policy-maker has to bear in mind the possibility that the forecast might be wrong. If it is wrong, a policy decision which is heavily based on the assumption that the forecast is correct might also be wrong. On occasions, that might have serious consequences for the economy.
Having heard a forecast, the policy decision-makers must then go another step. They must attach payoffs or penalties to the possible errors they could make in responding or not responding to that forecast. There are different types of errors. One error is to fail to act on the basis of a correct forecast; another is to act on the basis of a forecast which turns out to be wrong.
These errors need not have equal penalties. There can be times when they are decidedly unequal. Imagine a forecast which indicates strong growth and rising inflation. Taken at face value this suggests a tightening of policy. (I stress that this is for illustration only; there is no other message to be taken from this particular example.) The policy-makers can either believe or disbelieve the forecast, and the forecast can either be accurate or not. So there are four possible outcomes. Suppose the forecast is accurate, the policy-makers believe it, and tighten policy (in so doing causing an outcome different from the forecast!). This is the ideal outcome. If they do not believe it, and do nothing, then interest rates will be too low. But consider now the outcomes when the forecast is wrong to the extent that, in fact, growth slows and an easing of policy was actually required. The ‘do nothing’ strategy would still be wrong, but not as wrong as a strategy which believed the forecast and tightened. Hence in that case, if the policy-makers had reservations about the forecast, there might be a case for them to do nothing rather than respond automatically to the forecast, on the basis that this lessens the probability of a really serious error.
Now this example might be criticised as being somewhat contrived, because another possible forecast error is that the growth turns out stronger, and inflation higher, than forecast. In that event, to have done nothing with policy would have been a much worse decision than to have acted on the basis of a forecast which turned out to be too low. In essence, this is part of the reason why in simple theoretical models which take into account uncertainty about forecasts, the answer which usually emerges is that the policy-makers should behave as if they were certain that the forecast was correct –‘certainty equivalence’ in the jargon.[3] (This assumes that errors in forecasts are symmetric around the central forecast, and that the penalties the policy-makers attach to those outcomes also symmetric.) I should add that forecasts can also be ‘wrong’ in the sense that the outcomes are slightly different from what is expected, without being seriously misleading. Most of the time, forecasts which are roughly right are probably good enough.
But I think policy-makers would argue that there are times when the distribution of forecast errors is not symmetric, and certainly that the ‘penalties’ attached to them are not symmetric. The situation I described above, for example, might easily characterise the late stages of a long, strong business cycle upswing, where policy has tightened a good deal but where there was as yet little sign of slowing growth.
Alternatively, consider an economy which is growing well, but has very low inflation and considerable spare capacity. It is then hit by a contractionary shock of some kind. Forecasters tell the policy-makers that growth will decline, but will still be reasonable. In this environment, policy-makers might choose to make sure that they err, if at all, slightly on the expansionary side in setting policy. The reason is that if growth turns out to be stronger than forecast, the economy has ample capacity to cope with it in the short term, without causing problems on inflation, and in any event a slight rise in inflation from a very low starting point might be no real problem. On the other hand, if growth turns out lower than forecast, with inflation perhaps falling, that is clearly an inferior outcome – given the starting point in question – to the one where growth is stronger than expected. One could argue that this type of consideration has been a part of the policy-making environment in Australia over the past couple of years.
The decision-makers, then, need not only to ask ‘What is the forecast?’ They also need to ask ‘How much should we stake on this forecast being correct?’ They know that if they behave in a way the forecast suggests and the forecast turns out to be correct, they will have done well. But they must also give some thought to making as sure as they can that any mistake they might make is the lesser of the possible errors.[4]
Good forecasters, of course, know this and so they present not just a central forecast, but also an extensive discussion of ‘risks’ to the forecasts, and an assessment of where the balance of risks lies. This is, in fact, the most important and most useful part of a forecast which is made for policy purposes. Our own internal forecasting practices in recent years have tried to do much more of this. The central numbers written down are judged to be the most likely outcome, but there is an appreciation that other outcomes might also have a reasonably high likelihood. This idea has been shown most vividly in the Bank of England's celebrated ‘rivers of blood’ fan chart published in its Inflation Report. We have not gone to that extent in presentation of forecasts, and do not intend to, but the general idea of the forecast being a probability distribution of possible outcomes rather than a point estimate is, I think, a useful one.
In a sense, the numerical central forecast and the associated discussion of risks become a benchmark, which those involved in a discussion about the outlook can use to explore differences of view, and possible consequences of alternative outcomes. It is, to me, quite conceivable that there might be no-one who actually holds to every element of the numerical central forecast itself, but that, at the same time, all may be content to use it as the basis for discussion. Often, the value of that discussion lies less in its focus on particular numbers than in its identifying what the major forces are that might have a bearing on the economy over a particular period of time. This is a point made by Sir Alan Budd (1999) in a recent review of the experience of the Panel of Independent Forecasters in the UK in the early 1990s.[5] That sort of discussion itself is far more useful for policy-makers, even if forecasters cannot quite agree on how those forces might play out, than a bald statement of central forecasts which have resulted from a compromise between differing views. The policy-maker is then in a position to take a decision which is informed by forecasts, but which also allows for uncertainty.
Decision-making under Uncertainty
In trying to explain the process by which policy-makers might respond to forecasts, I have strayed into a much bigger field, namely decision-making under uncertainty. There are, of course, other types of uncertainty than just forecast uncertainty. In theory, forecast uncertainty is seen as one of the more straightforward areas to deal with, if that is the only uncertainty one has – ‘certainty equivalence rules’, as I noted above. But things get more complicated if, as in the case I outlined earlier, one finds oneself in a position where a greater significance is attached to outcomes on one side of the central expectation than on the other. They are also complicated by other uncertainties, like model uncertainty (including uncertainty about how much effect policy changes have on the economy). In many circumstances, this sort of uncertainty leads to policy caution – smaller moves in interest rates than would be suggested by looking simply at the forecast and ignoring uncertainty. The intuition is that if the policy-makers don't know accurately how much their actions affect the economy, then activist policy increases the expected variability of the economy – precisely the opposite of what policy-makers are seeking to achieve.
From there it is not hard to understand the decision rule proposed by Blinder (1996), which essentially is as follows:
- estimate the required change in policy (based on your forecast etc);
- do less; and
- watch developments – if things turn out as expected, continue down the planned track; otherwise, make a new plan.
I would also offer the conjecture that a lot of uncertainty about the outlook is really model uncertainty: that is, uncertainty about the process that is driving not only the forecast but also the current data. As Budd (1999) points out, a vast amount of time in both forecasting and policy discussions is taken up by trying to understand what recent data are actually saying, and in deciding how much weight to attach to the apparently contradictory findings which are inevitably thrown up by some of the statistics. Another way of putting this same point is that the process of analysing forecast errors – how the most recent data differ from what was expected – is important, with the key question whether the error is within the normal tolerances and to be seen as just noise, or whether it is large and persistent enough to be taken as signifying that the way the economy works has changed. The discussion that has been taking place in several countries over unexpectedly strong growth combined with unexpectedly low inflation, and whether this amounts to a ‘new economy’ and so on, falls into this category.
My final observation is that forecast uncertainty, from a policy-maker's perspective, may often be over how well a forecast has taken account of past changes in policy. A common feature of forecasts is that they tend to expect that what has been happening recently will continue, before giving way to a smooth return to ‘normality’ towards the end of the forecast horizon. As noted above, forecasts are notoriously bad at picking turning points. Yet it is at precisely those times when policy-makers need a good forecast most of all, and particularly one which takes account of the policy changes that they have already made. When policy-makers have made substantial shifts in policy already, forecasts that have not taken proper account of those moves are dangerous.
In my experience, policy-makers instinctively, and justifiably, get more cautious at such times, and more sceptical of particular forecasts, particularly if they suspect too much weight has been given to a continuation of current trends and too little to longer-run considerations. Both theory and experience suggest, moreover, that such caution is often well-advised. This is not to say that inertia in the policy process is advisable all the time; far from it. At key moments, policy-makers have to be prepared to move quickly, and plenty of mistakes have been made by being too slow to adjust policy.
These more general questions of policy-making under various kinds of uncertainty are worthy of more detailed consideration than we can manage today. There is some quite interesting work going on in various central banks on these issues, and they are certainly on our work program in the Economic Group of the RBA. But for now, it is time to conclude.
Conclusion
History is replete with classic examples of forecasts that went spectacularly astray. So I have always believed in the maxim that the first rule of forecasting is ‘don't’. Forecasting is, however, an occupational hazard of being an economist, particularly one involved in giving policy advice. Because of lags in the effect of policy, forecasts have to be made as part of the policy process.
But it is a mistake to think that getting policy right is simply a matter of getting the forecast right, and that everything flows easily from that. It would be foolhardy to operate on the assumption that forecasts will not, from time to time, go wrong. They will, despite our best efforts to improve forecasting accuracy. So forecasts are not, and cannot be, simply accepted at face value by policy-makers. Instead, the policy-makers must be informed by the forecasts, by the discussion of risks around the forecasts and of the forces the forecasters see at work in producing them. They must then take into account all the relevant uncertainties in calibrating their policy decision. Some forecast errors don't matter much, but some do. Part of the policy-makers' art is to develop a sense of when the consequences of forecast and associated policy error are likely to be greater, and to adjust their behaviour accordingly.
Endnotes
Reviews of international forecast accuracy include Llewellyn and Arai (1984), Artis (1988; 1996), Ballis (1989), Barrionuevo (1992), OECD (1993) and IMF (1998). For the UK, Pain and Britton (1992) review the National Institute forecasts, while Budd (1999) contains a fascinating account of the experience of the UK's Panel of Independent Forecasters. Romer and Romer (1996) compared Federal Reserve forecasts with others. Early Australian work on the topic included Pagan et al. (1982a; 1982b) and Macfarlane and Hawkins (1983). [1]
The following anecdote, from a recent book by Thomas Mayer about US monetary policy in the 1960s and 1970s, is of interest. In the early to mid 1960s, according to one Fed insider, Federal Reserve Chairman William McChesney Martin ‘had so little faith in economic forecasts that the staff was prohibited, on pain of being fired, from making forecasts other than flow-of-funds forecasts and primitive GNP forecasts connected with the flow-of-funds forecasts. And even these forecasts could not be made within the sacred premises of the Temple, but had to be made on Sunday mornings at the home of a senior staff economist…’ (Mayer 1999, p 19). These days central bank staff are at least allowed to develop their forecasts at the office! [2]
In slightly more technical language, with a linear model and a quadratic objective function, certainty equivalence is the optimal strategy. [3]
In a way, this is analogous to something which many in today's audience have something to do with as advisers, namely funds management. A funds manager has to listen to the prognosis for a particular market or instrument. He/she then has to ask ‘how much am I prepared to stake on this view?’ and ‘How much will I regret it if I take a position and turn out to be wrong?’ [4]
Having said that, Budd showed how people with diametrically opposed views on how the economy worked could sometimes come up with the same advice on policies! This almost turns the old joke about economists on its head. [5]
References
Artis, Michael J (1988), ‘How Accurateis the World Economic Outlook? A Post-Mortem on Short-Term Forecasting at the International Monetary Fund’, Staff Studies for the World Economic Outlook, IMF, July, pp 1–49.
Artis, Michael J (1996), ‘How Accurate are the IMF's Short-Term Forecasts? Another Examination of the World Economic Outlook’, International Monetary Fund Working Paper No. 96/89.
Ballis, B (1989), ‘A Post Mortem on OECD Short-Term Projections from 1982 to 1987’, OECD Economics and Statistics Department Working Paper No. 65.
Barrionuevo, José M (1992), ‘A Simple Forecasting Accuracy Criterion under Rational Expectations: Evidence from the World Economic Outlook and Time Series Models’, International Monetary Fund Working Paper No. 92/48.
Blinder, Alan S (1996), Central Banking in Theory and Practice, The Lionel Robbins Lectures, MIT Press, Cambridge, MA.
Budd, Alan (1999), ‘Learning from the Wise People’, The Manchester School, 67 (supplement), pp 36–48.
IMF (1992), ‘The Accuracy of World Economic Outlook Projections for the Major Industrial Countries’, Annex VIII, World Economic Outlook, May, pp 88–93.
Llewellyn, John and Haruhito Arai (1994), ‘International Aspects of Forecasting Accuracy’, OECD Economic Studies, 63, pp 73–117.
Macfarlane, IJ and JR Hawkins (1983), ‘Economic Forecasts and their Assessment’, Economic Record, 59 (167), pp 321–331.
Mayer, Thomas (1999), Monetary Policy and the Great Inflation in the United States: The Federal Reserve and the Failure of Macroeconomic Policy, 1965-79, Edward Elgar, Cheltenham, UK.
OECD (1993), ‘How Accurate are Economic Outlook Projections?’, OECD Economic Outlook, 53, June, pp 49–54.
Pagan, AR, PK Trivedi & TJ Valentine (1982a), ‘Assessment of Australian Economic Forecasts’, Australian Business Economists, April, Sydney.
Pagan, AR, PK Trivedi & TJ Valentine (1982b), ‘Assessment of Australian Economic Forecasts’, Australian Business Economists, September, Sydney.
Pain, Nigel and Andrew Britton (1992), ‘National Institute Economic Forecasts 1968 to 1991: Some Tests of Forecast Properties’, National Institute Economic Review, 141, August, pp 81–93.
Romer, Christina D & David H Romer (1996), ‘Federal Reserve Private Information and the Behavior of Interest Rates’, NBER Working Paper No.5692.
Svensson, Lars EO (1997), ‘Inflation Forecast Targeting: Implementing and Monitoring Inflation Targets’, European Economic Review, 41(6), pp 1111–1146.