Research Discussion Paper – RDP 2024-09 The ‘Clean Energy Transition’ and the Cost of Job Displacement in Energy-intensive Industries
December 2024
1. Introduction
Across the OECD, economies are transitioning towards greater carbon- and resource-efficiency to mitigate the impacts of climate change, promote sustainable growth, and ensure long-term economic resilience. At the heart of these processes are energy-intensive industries (whether in terms of supply or demand), which will see profound restructuring to realize the “clean energy transition”. This includes a reduction in the use of fossil fuels for energy production and consumption, while incentivizing the development of cleaner sources of energy (energy supply), as well as the adoption of technologies that enhance energy-efficiency in energy-intensive processes in transport and production (energy distribution and demand).[1]
The transition towards cleaner energy systems, as part of the broader effort to combat climate change, e.g. the EU's “Fit for 55”, has put in motion significant changes in energy-intensive sectors. While this shift opens up new employment opportunities in renewable and nuclear energy activities (see e.g. Borgonovi et al. (2023[2])) as well as jobs associated with improved energy efficiency, it increases the risk of job displacement in energy-intensive and predominantly fossil-fuel-based industries, potentially affecting public support for climate policies. Some sectors and firms may be able to change their fuel mix and/or reduce the energy intensity of their production processes by changing the way they operate.[2] In other cases, such as fossil-fuel extraction, the impacts are likely to arise across firms and sectors, raising the risk of job displacement.
The main objective of this paper is to provide a comprehensive analysis of job displacement in energy-intensive industries (and other industries) using detailed linked employer-employee data for 14 OECD countries.[3] Displaced workers in energy-intensive industries may face particularly challenges because of the specific characteristics of firms and workers in these sectors and the limited availability of opportunities for moving to a similar jobs due to the decline in energy-intensive employment (Borgonovi et al., 2023[2]). A secondary objective is to provide additional background to OECD (2024[1]) on the methodology and the effects of job-displacement across subsectors (energy supply, heavy manufacturing, transport). Energy-intensive industries, as defined in this paper based on energy-intensity, correspond exactly to the set of high-emission sectors as defined in OECD (2024[1]) based on greenhouse gas intensity.
The remainder of the paper is structured as follows. Section 2 outlines the measurement of energy-intensive industries, presents the national data sources that are be used for the main analysis, and discusses the harmonization process to ensure their comparability across countries. In Section 3, we detail our framework for estimating the costs of job displacement across sectors. Section 4 presents our main results for various energy-intensive industries and the broader economy. Section 5 summarizes policy approaches that can reduce the costs of job displacement in energy-intensive industries and help to build support for the clean energy transition.
2. Data
In this paper, as well as in OECD (2024[1]), we utilise matched employer-employee data for a total of 14 OECD countries (Australia, Austria, Canada, Denmark, Estonia, Finland, Germany, Hungary, the Netherlands, Norway, Portugal, Spain, France, Sweden). This section defines energy-intensive industries, explains the harmonisation of matched employer-employee data across countries and introduces the empirical strategy for measuring and explaining the costs of displacement between sectors and countries. As such, it provides additional background to the less detailed exposition of the methodology in OECD (2024[1]).
Defining energy-intensive industries
For comparability purposes, we use a uniform definition of energy-intensive industries across countries.[4] We rely on Environmental Accounts data from the World Input-Output Database, covering 27 EU countries, the United Kingdom, Norway, Iceland and Switzerland, for the period 2000-2014. The Environmental Accounts include information on gross energy use in terajoules (TJ) and value added across NACE 2-digit industries (European Commission JRC, 2019[3]). This allows us to define the energy intensity of a sector in terms of energy use over value added and to differentiate between sectors with high-energy intensity and lower energy intensity.
In practice, we define energy-intensive industries as industries appearing in the top two deciles of the energy-intensity distribution across 2-digit NACE industries in at least 15 countries (Figure 1).[5] The definition of energy-intensive sectors comprises sectors with particularly high energy intensity, averaging about 181 terajoule (TJ) per million EUR of gross value added (in constant 2015 prices of the national currency), from sectors with lower energy intensity. The manufacturing of coke and refined petroleum products stands out in terms energy-intensity, with more than 1200 TJ per million EUR of gross value added, significantly driving up the average energy-intensity of energy-intensive industries. Without this sector, energy-intensive industries would average 64 TJ per million EUR of gross value added. Importantly, the definition of energy-intensive industries coincides with that of carbon-intensive sectors, as used in Barreto, Kril and Grundke (2023[4]) and with that of GHG-intensive sectors used in OECD (2024[1]).[6]
To provide more insight on the cost of job displacement across energy-intensive industries, we divide them into three separate sectors:
- Energy supply (“E”). This includes three sectors: i) electricity, gas and air conditioning; ii) coke and refined petroleum; iii) mining and quarrying. The NACE-2 digit level classification is not sufficiently granular to single out renewable energy generation which is expected to expand from fossil fuel energy generation (see e.g. Borgonovi et al. (2023[2])).
- Energy-intensive manufacturing sectors (“heavy manufacturing” or “M”). This includes many sectors commonly defined as “heavy manufacturing” (or sometimes “hard-to-abate”) such as manufacturing of basic metals, chemicals, pulp and paper, and non-metallic mineral products. We refer to this as “heavy manufacturing” (I).
- Energy-intensive service sectors (“transport” or “T”). This includes the part of services related to transport whether by air, sea or land transport. We will therefore refer to energy-intensive services as transport (T). Note that NACE classification does not allow excluding transport based on renewable energy sources such as electrified land transport.
Given the very different nature of these sectors, they are likely to be impacted differently by the clean-energy-transition. For example, energy supply is directly impacted by structural changes in the energy mix of many OECD countries, with a shift from fossil-fuel-based energy-generation to renewables. Heavy manufacturing is indirectly affected by the clean-energy-transition, as it faces changes in energy costs as well as regulatory requirements. The transport sector is affected through technological and infrastructure changes, but also faces increased energy costs as well as regulatory requirements. As such, a sectoral differentiation of the analysis in OECD (2024[1]) may shed additional light on the labour market effects of the clean-energy transition.[7]
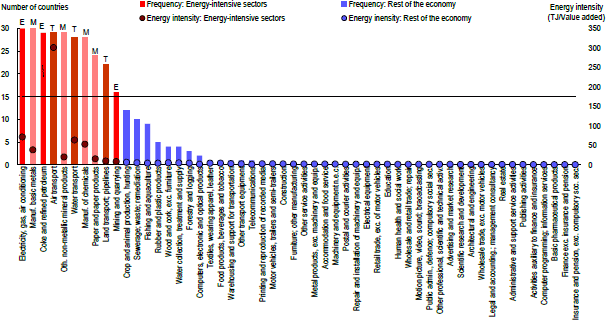
Note: The figure shows on the left axis the frequency with which a particular industry is ranked among the top 20% of most energy-intensive industries across European countries as well as on the right axis the average energy use across countries expressed in TJ per unit of value added. E refers to energy supply sectors, M refers to heavy manufacturing sectors and T refers to transport sectors.
Source: World Input-Output Database Environmental Accounts
Data sources and harmonisation
For the analysis of job displacement in energy-intensive industries, we make use of harmonised linked employer-employee data for 14 OECD countries: Australia, Austria, Canada, Denmark, Estonia, Finland, France, Germany, Hungary, the Netherlands, Norway, Portugal, Spain and Sweden (see Table 1). These data are derived from administrative records designed for tax or social security purposes or, in a few cases, mandatory employer surveys. Each of these datasets are panels, cover the universe of workers or a large random sample of workers, and contain identifiers for their employers and industries. Each of these features is indispensable to the analysis of job displacement within detailed industries. The resulting dataset typically covers the years 2000 to 2019, and thus avoids potential confounding effects resulting from the COVID-19 pandemic and subsequent energy-cost inflation. For some of the countries, the observation period begins later than 2000 or ends before 2019.
Country | Name | Earnings data source | Sample structure | Time coverage |
---|---|---|---|---|
Australia | Person Level Integrated Data Asset (PLIDA) | Tax administration | 10 % random sample of workers | 2002-2019 |
Austria | AMS-BMASK Arbeitsmarktdatenbank | Social security administration | Universe | 2000-2019 |
Canada | Canadian Employer-Employee Dynamics Database | Tax administration | Universe | 2001-2019 |
Denmark | Integrerede Database for Arbejdsmarkedsforskning (IDA) and other data from Statistics Denmark | Tax administration | Universe | 2000-2019 |
Estonia | Data from the Tax and Customs Board Register | Tax administration | Universe | 2003-2019 |
Finland | FOLK employment data from Statistics Finland, Employer Payroll Report from Tax Admin. | Tax administration | Universe | 2000-2019 |
France | Panel DADS | Social security administration | 1/12 % random sample of workers | 2002-2019 |
Germany | Integrierte Erwerbsbiographien (IEB) | Social security administration | 10% random sample of workers | 2000-2019 |
Hungary | ADMIN –2 – Panel of linked administrative data (OEP, ONYF, NAV, NMH, OH) | Social security administration | 50% random sample of workers | 2003-2017 |
Netherlands | CBS Microdata from Statistics Netherlands | Tax administration | Universe | 2006-2019 |
Norway | Arbeidsgiver- og arbeidstakerregister (Aa-registeret), Lønns- og trekkoppgaveregisteret (LTO) | Tax administration | Universe | 2002-2019 |
Portugal | Quadros de Pessoal | Mandatory employer survey | Universe | 2002-2019 |
Spain | Muestra Continua de Vidas Laborales con Datos Fiscales (MCVL-CDF) | Social security and tax administration | 4% random sample of workers | 2006-2019 |
Sweden | Longitudinell integrationsdatabas för sjukförsäkrings- och arbetsmarknadsstudier (LISA), Företagens ekonomi (FEK), Jobbregistret (JOBB) | Social security administration | Universe | 2002-2018 |
To ensure comparability across the 14 linked employer-employee panels, we standardize the data using a common data preparation procedure for each country. For each of the datasets, we select the primary employer, which is defined as the employer from which an employee receives the highest earnings in a given year.[8] Whenever possible, employers are identified based on establishment identifiers rather than those of the firm which may comprise several establishments, potentially operating in different industries and regions. In countries where there are no establishment identifiers are available, firm identifiers are used instead.[9] Worker tenure at these employers is measured as the duration of employment with the current employer in years.
Earnings are defined broadly, encompassing regular wages, overtime, and bonuses, all before taxes and social security deductions.[10] In countries where earnings are censored above a certain ceiling (e.g. Germany), earnings are imputed following the procedure proposed by Card, Heining and Kline (2013[5]). Based on the earnings information, we compute a set of harmonized outcomes. Annual earnings are calculated as the total labour income for the respective year, potentially from multiple employers beyond the primary employment spell. Daily wages are derived by dividing annual earnings from the main employer by the number of days worked for the main employer that year, excluding marginal jobs with very low earnings.[11] Following the standard practice in the job displacement literature, we assign zero earnings and missing daily wages during periods of non-employment spells, that is, years without recorded earnings from any employer.
Finally, we apply common sample restrictions across all datasets. Our baseline analysis focuses on workers aged 18-50 years in the private sector, excluding apprentices and self-employed individuals While we do not consider mass-layoff events in the public administration and defence sectors, we retain employment spells in the final datasets to avoid overstating earnings losses when workers transition to the public sector after displacement (see the methodology section for a detailed definition of the mass layoff events).
Descriptive statistics
The average number of workers included in the final datasets is large in all countries and sectors, and its absolute number depends on the data structure (i.e. whether the source dataset is the universe or a random sample) (Table 2). The energy-intensive sector accounts about 9% of total employment in the included countries. Workers in this sector tend to predominantly be male, slightly older on average, and often boast longer tenures compared to workers in other sectors. Average length of tenure is especially high in energy supply and heavy manufacturing, while in transport it is not too different from the rest of the economy. Workers in energy supply and heavy manufacturing are also more frequently employed by high-paying firms. While employment in energy supply and heavy manufacturing has been on a declining trend, employment in the rest of the economy[12] and, in some countries also in the transport sector, has been growing.
As evident in Table 2, the number of workers displaced in mass layoff events – which we define further below – varies considerably across sectors. Among other factors, this is the result of differences in sample size and employment shares as well as country- and sector-level employment trends. Notably, the number of displaced workers tends to be smallest in energy supply. For this reason, estimates of job displacement in energy supply sectors should be interpreted with caution, especially for France and Spain. In practice, we exclude country-sector combinations with fewer than 100 individual layoffs and 10 firms experiencing mass layoffs over the analysis period.
Country | Average number of workers | Age | Share of women | Tenure (years) | Firm size | Log daily wage | AKM Firm FE | Number of displaced workers | Number of mass-layoff firms | Average share of sector in total employment | Employment change (first year – last year) |
---|---|---|---|---|---|---|---|---|---|---|---|
Panel A. Energy supply | |||||||||||
AUS | 15,405 | 38.69 | 19.83 | 3.42 | 441 | 5.58 | 0.40 | 776 | 367 | 2.43 | 35.62 |
AUT | 31,713 | 41.67 | 16.96 | 10.44 | 566 | 4.79 | 0.12 | 530 | 17 | 0.98 | 1.44 |
CAN | 321,768 | 41.17 | 22.15 | 7.68 | 4,449 | 11.23 | 0.41 | 10,345 | 40 | 3.22 | 20.87 |
DEU | 32,941 | 43.53 | 20.15 | 9.98 | 794 | 4.95 | 0.32 | 107 | 181 | 1.17 | −25.62 |
DNK | 12,556 | 45.00 | 22.78 | 5.92 | 115 | 7.08 | 0.15 | 33 | 18 | 0.60 | 2.07 |
ESP | 2,797 | 44.07 | 15.01 | 6.98 | 329 | 4.47 | 0.61 | 72 | 85 | 0.43 | −21.26 |
EST | 12,848 | 45.86 | 24.04 | 3.83 | 1,225 | 9.23 | 0.32 | 40 | 6 | 2.28 | −35.75 |
FIN | 10,530 | 43.11 | 19.55 | 7.33 | 1,395 | 4.72 | 0.09 | 396 | 44 | 0.98 | −17.81 |
FRA | 16,893 | 42.43 | 23.35 | 8.65 | 350 | 4.80 | 0.17 | 41 | 61 | 0.97 | −22.73 |
HUN | 17,239 | 43.32 | 24.40 | 5.43 | 1,666 | 9.35 | 0.55 | 339 | 18 | 1.05 | −27.19 |
NLD | 38,604 | 43.98 | 20.45 | 3.30 | 1,674 | 4.96 | 0.31 | 10 | 11 | 0.55 | 26.24 |
NOR | 65,377 | 43.25 | 19.76 | 5.51 | 5,128 | 7.54 | 0.43 | 1,043 | 14 | 2.68 | 57.53 |
PRT | 19,726 | 44.13 | 13.59 | 14.97 | 1,239 | 3.89 | 0.36 | 169 | 1 | 0.83 | −15.78 |
SWE | 36,275 | 44.91 | 22.80 | 7.17 | 949 | 7.05 | 0.10 | 225 | 20 | 1.00 | 22.20 |
Average | 45,334 | 43.22 | 20.35 | 7.19 | 1,451 | 6.40 | 0.31 | 1,009 | 63 | 1.37 | −0.01 |
Panel B. Heavy manufacturing | |||||||||||
AUS | 9,927 | 39.6 | 22.2 | 4.0 | 393 | 5.2 | 0.15 | 335 | 1,128 | 1.57 | −36.94 |
AUT | 106,058 | 39.5 | 18.9 | 7.3 | 536 | 4.7 | 0.20 | 3,047 | 194 | 3.27 | 2.95 |
CAN | 263,721 | 43.5 | 19.3 | 8.6 | 2,252 | 10.9 | 0.20 | 15,500 | 480 | 2.64 | −35.77 |
DEU | 94,626 | 43.0 | 19.6 | 11.0 | 2,067 | 4.7 | 0.23 | 955 | 2,327 | 3.37 | −15.55 |
DNK | 34,408 | 44.2 | 24.5 | 7.1 | 140 | 6.9 | 0.11 | 2,012 | 138 | 1.64 | −33.99 |
ESP | 12,751 | 43.0 | 18.0 | 7.2 | 355 | 4.2 | 0.36 | 501 | 932 | 1.94 | −25.81 |
EST | 8,372 | 41.8 | 30.0 | 3.7 | 230 | 9.1 | 0.20 | 363 | 25 | 1.48 | 14.26 |
FIN | 28,909 | 42.3 | 29.4 | 7.5 | 474 | 4.7 | 0.08 | 1,153 | 89 | 2.69 | −27.92 |
FRA | 37,400 | 42.5 | 24.7 | 7.6 | 434 | 4.6 | 0.17 | 836 | 715 | 2.14 | −38.20 |
HUN | 39,246 | 40.9 | 31.1 | 4.7 | 837 | 8.9 | 0.25 | 2,515 | 107 | 2.40 | −5.44 |
NLD | 106,617 | 45.3 | 15.2 | 4.3 | 1,172 | 4.7 | 0.19 | 2,461 | 123 | 1.52 | −12.16 |
NOR | 38,487 | 43.2 | 19.0 | 7.6 | 1,802 | 7.1 | 0.23 | 4,209 | 142 | 1.58 | −38.13 |
PRT | 66,232 | 41.6 | 26.6 | 11.6 | 208 | 3.5 | 0.11 | 2,613 | 139 | 2.78 | −14.98 |
SWE | 102,541 | 43.9 | 20.8 | 8.8 | 1,069 | 6.9 | 0.09 | 2,431 | 143 | 2.82 | −18.33 |
Average | 67,807 | 42.47 | 22.81 | 7.21 | 855 | 6.15 | 0.18 | 2,781 | 477 | 2.27 | −20.43 |
Panel C. Transport | |||||||||||
AUS | 17,833 | 40.46 | 24.36 | 3.31 | 372 | 5.06 | 0.09 | 282 | 2,454 | 2.82 | −9.23 |
AUT | 109,124 | 39.95 | 18.61 | 4.64 | 284 | 4.30 | −0.07 | 2,940 | 473 | 3.36 | 38.15 |
CAN | 359,332 | 43.80 | 24.19 | 7.40 | 4,509 | 10.61 | 0.01 | 6,275 | 650 | 3.59 | 29.44 |
DEU | 60,875 | 43.43 | 19.28 | 6.97 | 1,219 | 4.31 | −0.09 | 418 | 1,331 | 2.17 | 35.13 |
DNK | 74,950 | 43.81 | 18.87 | 4.03 | 59 | 6.82 | 0.05 | 1,910 | 278 | 3.58 | −12.45 |
ESP | 18,637 | 44.54 | 13.04 | 6.99 | 721 | 3.96 | 0.11 | 378 | 803 | 2.83 | −0.51 |
EST | 22,273 | 44.88 | 18.36 | 2.87 | 243 | 8.73 | −0.11 | 312 | 37 | 3.95 | 19.64 |
FIN | 86,761 | 40.88 | 28.47 | 6.81 | 318 | 4.72 | 0.04 | 1,229 | 149 | 8.09 | −18.10 |
FRA | 61,343 | 41.45 | 18.98 | 5.91 | 933 | 4.43 | 0.06 | 729 | 1,320 | 3.52 | 37.39 |
HUN | 33,437 | 41.19 | 16.25 | 3.35 | 802 | 8.45 | −0.12 | 502 | 81 | 2.04 | 142.14 |
NLD | 214,825 | 44.50 | 19.62 | 3.29 | 3,361 | 4.49 | 0.07 | 2,360 | 320 | 3.06 | −2.96 |
NOR | 91,982 | 43.30 | 15.85 | 4.45 | 4,892 | 6.88 | 0.04 | 1,943 | 217 | 3.77 | −8.96 |
PRT | 85,156 | 41.72 | 13.42 | 8.16 | 937 | 3.45 | 0.07 | 2,241 | 171 | 3.58 | 37.11 |
SWE | 122,923 | 44.53 | 15.57 | 5.53 | 1,052 | 6.78 | −0.01 | 1,956 | 241 | 3.38 | 18.49 |
Average | 97,104 | 42.75 | 18.92 | 5.27 | 1,407 | 5.93 | 0.01 | 1,677 | 609 | 3.55 | 21.81 |
Panel D. Rest of economy | |||||||||||
AUS | 579,944 | 36.83 | 49.86 | 3.15 | 372 | 4.86 | −0.01 | 14,108 | 82,527 | 91.58 | 20.54 |
AUT | 2,973,153 | 38.18 | 48.14 | 5.41 | 379 | 4.34 | 0.00 | 79,992 | 10,104 | 91.65 | 38.51 |
CAN | 8,915,632 | 40.55 | 50.35 | 7.63 | 3,844 | 10.49 | −0.03 | 244,620 | 21,830 | 89.13 | 31.59 |
DEU | 2,600,800 | 41.39 | 47.05 | 7.59 | 819 | 4.41 | −0.01 | 26,034 | 78,629 | 92.52 | 19.47 |
DNK | 1,945,936 | 42.10 | 50.09 | 4.66 | 56 | 6.73 | 0.00 | 51,187 | 5,704 | 92.95 | 15.40 |
ESP | 579,140 | 41.88 | 46.45 | 5.45 | 555 | 3.86 | 0.02 | 7,907 | 31,695 | 88.02 | 6.25 |
EST | 508,767 | 41.44 | 54.03 | 2.98 | 641 | 8.84 | −0.01 | 8,419 | 1,059 | 90.16 | 8.40 |
FIN | 942,091 | 39.96 | 44.54 | 5.74 | 1,781 | 4.57 | −0.01 | 22,653 | 3,275 | 87.79 | 42.59 |
FRA | 1,611,195 | 39.51 | 48.76 | 4.91 | 596 | 4.34 | −0.01 | 17,930 | 33,628 | 92.39 | 40.82 |
HUN | 1,506,675 | 40.02 | 51.53 | 3.68 | 7,768 | 8.64 | 0.01 | 30,349 | 3,648 | 92.04 | 15.63 |
NLD | 6,598,902 | 40.95 | 47.52 | 3.18 | 7,496 | 4.32 | −0.01 | 79,300 | 9,595 | 93.86 | 10.76 |
NOR | 2,187,498 | 40.73 | 49.85 | 4.70 | 9,844 | 6.76 | −0.02 | 60,289 | 7,753 | 89.55 | 26.75 |
PRT | 2,170,234 | 38.85 | 47.52 | 7.13 | 1,048 | 3.29 | −0.01 | 55,924 | 5,482 | 91.15 | 35.83 |
SWE | 3,352,449 | 43.34 | 46.83 | 7.06 | 4,298 | 6.81 | 0.00 | 42,679 | 5,855 | 92.14 | 23.22 |
Average | 2,605,172 | 40.41 | 48.75 | 5.23 | 2,821 | 5.88 | −0.01 | 52,957 | 21,485 | 91.07 | 23.98 |
Note: Sample means and totals over the time coverage of each dataset. Source: National linked employer employee data, see Table 1 for details. |
3. Methodology
Determining the cost of job displacement for a given worker poses important methodological challenges. Ideally, we would like to compare outcomes for the same worker in two states of the world: first, a situation where the worker loses employment and second, a counter-factual situation where the same worker continues to be employed in that same job. As this is evidently infeasible, we follow the job displacement literature and match observationally identical displaced and non-displaced workers (Jacobson, Lalonde and Sullivan, 1993[6]; Lachowska, Mas and Woodbury, 2020[7]; Schmieder, von Wachter and Heining, 2023[8]). Following this procedure, we estimate displacement effects in an event study framework using the matched sample of displaced and non-displaced workers. The precise implementation of the event study approach follows Barreto, Grundke and Krill (2023[9]) and OECD (2024[1]).
Defining the treatment
The analysis focuses on job displacement as a result of mass-layoff events. This approach ensures that firm separations are plausibly exogenous, involuntary and unrelated to the performance of workers or their individual career plans. As such, it prevents unobservable differences in performance between workers remaining employed and those laid off from affecting the estimates of job displacement costs.
To identify mass layoff events in our datasets, we follow the displacement literature by defining mass layoffs as events in which employment in a establishments with at least 30 employees declines by at least 30% between one year to the next (Jacobson, Lalonde and Sullivan, 1993[6]).[13] Our definition of mass-layoff events includes complete plant closures. To avoid contaminating our measures of mass layoffs with restructuring events (e.g. mergers and acquisitions), we impose the restriction that no more than 30% of displaced employees move to the same establishment following the event (Hethey-Maier and Schmieder, 2013[10]). This tends to be particularly important in sectors that have experienced important pro-competitive reforms during the sample period, such as those in the transport sector of several included countries.
Having defined mass layoff events, we define treated workers as those who separate from their employer in the year a mass layoff takes place and are not recalled to the same employer over the six subsequent years. In practice, we focus on workers 18 to 50 years old at the time of displacement to limit the influence of early retirement programs.[14] To identify separations among workers who had stable employment trajectories in their original employer, we also restrict the analysis to workers with at least 2 years of tenure in the year of mass layoff. By considering only the first observed mass layoff event for each worker, treatment can happen only once over the sample period, consistent with the idea that displacement represents a permanent shock to labour market trajectories.
Balancing treatment and controls
In our main analysis, we compare the outcomes of workers who are displaced between one year and the next as a result of mass layoff (treated) with those of non-displaced workers (control) who satisfy the same restrictions in the year immediately before displacement. We allow non-displaced control workers to be co-workers of displaced workers and to separate from their employer in subsequent years for any reason except a mass layoff event.[15]
As displaced and non-displaced workers may differ in their observable characteristics, we match each displaced worker to an observationally identical non-displaced worker through a 2-step matching procedure (“statistical twinning”). First, we use exact matching by baseline year, industry (1-digit NACE) and energy-intensive sector and sex. This ensures that displaced workers are matched only with workers in the same (energy-intensive) sector, of the same sex, and from the same year. In the second step, we estimate a propensity score separately for each cell using a probit model of job displacement on observable characteristics, including log daily wages in the three years prior to displacement, log employer size, age, and tenure (all included contemporaneously). Based on the estimated propensity scores, we apply nearest neighbor one-to-one matching without replacement to assign each displaced worker to a unique control worker. After the implementing this matching procedure, matched treatment and control workers have similar observable characteristics as shown by standardized differences below a value of 0.1 (Austin, 2011[11]) (see 3).
Country | ||||||||||||||
---|---|---|---|---|---|---|---|---|---|---|---|---|---|---|
Variable | AUS | AUT | CAN | DEU | DNK | ESP | EST | FIN | FRA | HUN | NLD | NOR | PRT | SWE |
Panel A. Energy supply | ||||||||||||||
Log daily* wage (c-1) | 0.03 | 0.07 | 0.01 | −0.03 | 0.04 | −0.02 | 0.15 | −0.05 | 0.05 | −0.06 | −0.07 | −0.03 | 0.03 | 0 |
Log daily* wage (c-2) | 0.02 | 0.09 | −0.02 | 0 | −0.01 | −0.01 | 0.12 | −0.03 | 0.05 | −0.06 | 0.05 | −0.03 | 0.04 | 0.02 |
Log daily* wage (c-3) | 0 | 0.08 | −0.01 | 0 | −0.01 | 0.05 | 0.15 | −0.02 | 0.16 | −0.05 | −0.2 | −0.03 | 0.04 | 0.04 |
Age | 0 | −0.01 | 0 | 0.09 | 0.02 | −0.02 | −0.06 | 0.02 | 0.05 | 0.01 | −0.06 | −0.01 | 0.05 | −0.02 |
Job tenure | 0.01 | 0.01 | −0.02 | 0.05 | 0.02 | −0.05 | 0.14 | −0.04 | −0.03 | −0.03 | 0 | 0.02 | 0.03 | 0.06 |
Log employer size | 0.01 | 0.13 | 0.03 | 0.07 | 0 | 0.09 | 0.07 | 0.01 | 0 | 0 | 0.59 | 0.03 | 0.12 | 0.03 |
Observations | 776 | 530 | 10300 | 107 | 33 | 72 | 40 | 396 | 41 | 339 | 10 | 1,043 | 169 | 225 |
Panel B. Heavy manufacturing | ||||||||||||||
Log daily* wage (c-1) | −0.02 | −0.02 | 0.03 | −0.01 | 0.03 | 0.05 | 0.02 | 0.02 | −0.03 | 0 | 0.02 | −0.01 | 0 | 0.06 |
Log daily* wage (c-2) | −0.01 | −0.01 | 0.02 | −0.02 | 0.02 | 0.04 | 0.01 | 0.03 | −0.03 | 0.02 | 0.01 | −0.01 | 0 | 0.02 |
Log daily* wage (c-3) | −0.01 | 0 | 0.03 | −0.01 | 0.02 | 0.05 | 0.05 | 0.01 | −0.03 | 0.02 | 0.02 | 0 | 0 | 0.02 |
Age | 0 | −0.01 | 0.02 | 0.01 | 0 | −0.01 | 0 | 0 | 0.02 | 0 | 0 | −0.01 | 0.01 | 0 |
Job tenure | −0.04 | 0.01 | 0.04 | 0.03 | 0.01 | 0 | 0 | 0.02 | −0.01 | 0.01 | −0.01 | 0.02 | 0.01 | 0 |
Log employer size | 0.04 | 0.03 | 0.07 | 0.02 | 0.01 | −0.02 | 0.05 | 0.03 | −0.03 | 0.04 | 0.03 | 0.03 | 0.03 | 0.01 |
Observations | 335 | 3,047 | 15500 | 955 | 2,012 | 501 | 363 | 1,153 | 836 | 2,515 | 2,461 | 4,209 | 2,613 | 2,431 |
Panel C. Transport | ||||||||||||||
Log daily* wage (c-1) | −0.1 | −0.03 | 0.02 | 0.04 | −0.02 | 0.05 | 0 | 0 | −0.03 | 0.07 | 0.01 | −0.01 | −0.01 | 0.03 |
Log daily* wage (c-2) | −0.02 | −0.02 | 0.01 | 0.04 | −0.01 | 0.04 | 0.01 | −0.01 | −0.03 | 0.04 | 0.03 | −0.01 | 0.01 | 0.01 |
Log daily* wage (c-3) | −0.02 | −0.03 | 0.01 | 0.03 | 0.03 | 0.05 | −0.01 | −0.03 | 0 | 0.03 | 0.03 | −0.01 | 0 | 0.02 |
Age | 0.01 | −0.02 | 0.01 | −0.03 | 0.01 | 0.01 | −0.02 | −0.01 | 0 | 0 | 0 | −0.01 | 0 | 0 |
Job tenure | −0.06 | −0.02 | −0.01 | 0 | −0.01 | 0.05 | −0.03 | 0.01 | −0.05 | −0.04 | −0.03 | −0.03 | 0.03 | 0.01 |
Log employer size | 0.02 | 0.03 | 0.04 | −0.01 | 0.01 | 0 | 0.02 | 0.04 | 0.01 | 0.09 | 0.04 | 0.02 | 0.12 | 0.03 |
Observations | 282 | 2,940 | 6300 | 418 | 1,910 | 378 | 312 | 1,229 | 729 | 502 | 2,360 | 1,943 | 2,241 | 1,956 |
Panel D. Rest of the economy | ||||||||||||||
Log daily* wage (c-1) | −0.01 | −0.01 | 0 | 0 | 0 | 0 | −0.01 | 0 | 0 | 0 | −0.01 | −0.04 | −0.01 | 0 |
Log daily* wage (c-2) | −0.01 | 0 | −0.01 | 0 | 0 | −0.01 | −0.01 | 0 | 0 | 0 | −0.01 | −0.02 | −0.01 | 0 |
Log daily* wage (c-3) | −0.01 | 0 | −0.01 | 0 | 0 | 0 | −0.01 | 0 | 0 | 0 | −0.01 | −0.02 | −0.01 | 0 |
Age | −0.01 | 0 | 0 | 0 | 0 | 0.01 | 0 | 0 | 0 | 0 | 0 | −0.01 | 0 | −0.01 |
Job tenure | −0.01 | −0.01 | 0 | −0.01 | −0.01 | 0 | 0.05 | −0.01 | 0.01 | −0.02 | 0 | −0.01 | −0.01 | 0 |
Log employer size | 0.02 | 0.01 | 0 | 0.01 | 0.02 | 0.03 | 0.07 | 0.02 | 0.01 | 0.03 | 0.03 | 0.05 | 0.02 | 0.03 |
Observations | 14,10 8 | 79,99 2 | 24460 0 | 26,03 4 | 51,18 7 | 7,907 | 8,419 | 22,65 3 | 17,93 0 | 30,34 9 | 79,30 0 | 60,28 9 | 55,92 4 | 42,67 9 |
Note: Exactly matched characteristics (e.g. gender, sector) are omitted as balanced by construction. Source: National linked employer employee data, see Table 1 for details. |
Event study design
We rely on an event study design to compare the outcomes of displaced and non-displaced workers before and after displacement separately for each energy-intensive sector and the rest of the economy, using the equation below:
where yitc is the outcome of worker i belonging to cohort c of displaced workers and matched controls at time t. The coefficients of interest capture the change in outcome of displaced workers relative to the evolution of the respective outcomes for non-displaced workers in the same sector, where k indexes event time such that k=1 in the first post-displacement year and k=0 in the last year before displacement. The coefficients are normalized to k=-2, such that the effects are measured relative to that time. The worker fixed effect controls for time-invariant unobserved worker heterogeneity (see below), is a calendar year fixed effect, a time since event fixed effects and contains a cubic in age. Finally, ritc is the idiosyncratic error term. Standard errors are clustered at the worker level.
The outcomes considered are annual earnings relative to the pre-displacement average, a dummy for being employed, the number of days worked, the log daily wage, the firm wage premium, and various mobility outcomes, such as the likelihood of changing the pre-displacement sector, occupation and region. Annual earnings are defined as the sum of labour payments (potentially, from different employers) in a given year divided by average pre-displacement annual earnings. The employment dummy is equal to one if a worker has at least one day of dependent employment in a given year and zero otherwise. Recall that in the event a worker is not observed in dependent employment in a given year, zero earnings are imputed. This may overstate the actual costs of job loss to the extent that some displaced workers move to the public sector or become self-employed. Days worked are defined as the total number of days in dependent employment in a given year conditional being employed at least one day irrespective of hours worked. Log daily wages are constructed as the natural logarithm of annual earnings divided by days worked at the main employer. Firm wage premia measure the average wage premia paid to all employees in a firm net of worker characteristics and is estimated using an AKM two-way fixed effects model (Abowd, Kramarz and Margolis, 1999[12]).[16] Finally, the probability of changing sector, occupation or region is measured using a dummy which is equal to one if the observed value after displacement differs from its pre-displacement value and zero otherwise.
Decomposing earnings losses
To provide an indication of the different components behind displacement costs, expressed as differences in annual earnings between displaced and non-displaced workers, we also include a decomposition exercise into several components that make up annual earnings. These are i) being out of work for an entire year, (ii) fewer days worked conditional on being employed at some point during the year and (iii) lower daily wages upon re-employment. Fewer days worked conditional on being employed at least one day during the year may reflect a combination of the return to work during the year (as the first job after displacement starts after 1 January), the instability of employment following displacement (for example if workers are more likely to be re-employed on temporary contracts) or permanent exits during the year from employment (for example workers moving to inactivity or self-employment after 1 January). Lower wages upon re-employment, measured as daily wages, reflect a combination of hourly wages and working time. Daily instead of hourly wages are used to enhance the cross-country comparability of the results.
In practice, we decompose differences in annual earnings y for a given worker into the components that can be attributed to the probability of being employed in the year p, the number of days worked ND, and the daily wage w – mostly following Schmieder, von Wachter and Heining (2023[8]). Taking expectations over the samples of displaced and non-displaced workers, we can express the earnings losses of a displaced worker (D) related to the control group (S) in each time period after the event as:
Rearranging terms gives:
where the first term gives the contribution of changes in daily wages to changes in annual earnings relative to the control group, while the second and third term capture the contribution of days worked and employment probability, respectively. The contribution of the probability of being employed captures periods out of dependent employment lasting a full calendar year, in which annual earnings are imputed to be zero. The contribution of days worked captures periods of non-employment shorter than a full calendar year, which reflects a combination of non-employment and job instability. The contribution of daily wages captures changes in daily wages following displacement. Finally, the term is a residual which captures the change in the covariances between days worked, employment probability and daily wages and can be broadly interpreted as the selection into employment.
To shed light on the underlying drivers of wage losses, the role of daily wages for earnings losses can be further decomposed into a worker- and a firm-related component (Lachowska, Mas and Woodbury, 2020[7]). We do this by decomposing the treatment effect on wages into the sum of changes in firm wage-premia plus changes in worker-related components . While the former captures changes in the generosity of a firms' wage policies, the latter captures differences in human capital and match quality. The term is a residual which captures the change in the covariances between employment, days worked and daily wages which arise due to selection into employment. In practice, this component is very small and omitted for presentational purposes.
In a second step, wage losses upon re-employment are decomposed into a worker and a firm-related component. This is done by estimating the change in the firm-related component due to job displacement as measured by the firm fixed effect from an AKM two-way fixed effects model. The part of wage losses that is not firm-related may include worker-related wage losses due to the loss of human capital or wage losses due to a reduction in match quality.
Oaxaca-Blinder decomposition
The analysis of job displacement examines the outcomes of displaced workers compared to those who are not displaced but have similar characteristics within the same energy-intensive sector. Nevertheless, the characteristics of displaced workers in energy-intensive industries may still differ from those in other parts of the economy and from displaced workers in similar sectors in other analysed countries. Consequently, differences in the cost of job displacement across industries and countries might reflect variation in the composition of displaced workers or differences in the cost of job displacement for similar workers. Using Oaxaca-Blinder decompositions, we aim to measure the impact of worker composition on the differences in job displacement costs between industries and countries.
For this, we start by denoting the individual-level difference-in-differences estimate as:
where indicates the average outcome for after job displacement (1 to 4 years) and the corresponding average outcome before job displacement (-3 to -1 years). The estimate individual-level difference-indifferences can in turn be characterised as a linear model of the observable characteristics of displaced and non-displaced workers in each sector:
where is a vector of worker and firm characteristics measured before displacement (i.e. in the baseline period at k=0) for energy-intensive industries (ERI) and the rest of the economy (ROE) and is an error term. Naturally, this analysis extends to specific energy-intensive industries, i.e. energy supply, heavy manufacturing and transport, in place of ERI.
Using equations (1) and (2), the Oaxaca-Blinder decomposition of the difference in the difference-indifferences estimate between energy-intensive industries and the rest of the economy can be written as:
where the first component on the right-hand side captures the role of composition effect, i.e. the part that is explained by differences in observable characteristics between displaced workers in energy-intensive industries and the rest of the economy, and the second component captures the structural effect, i.e. unexplained differences in the cost of job displacement between energy-intensive industries and the rest of the economy holding composition constant. A similar decomposition can be used to shed light on the drivers of differences in the cost of job displacement in energy-intensive industries between countries.
4. Results
The cost of job displacement in energy-intensive industries on average across countries
On average across the countries considered in our analysis, workers displaced from energy-intensive industries have significantly higher earnings losses than those displaced in the rest of the economy (Figure 2). In the initial year following mass layoff, displaced workers in the rest of the economy lose about 52% of earnings compared to similar non-displaced workers, while workers displaced in energy-intensive industries lose 58% on average. Earnings losses partly converge with those of non-displaced workers in subsequent years, but differences in earnings losses between workers energy-intensive industries and the rest of the economy remain persistent. Even six years post-displacement, earnings losses are 26% in energy-intensive sectors compared with 18% in low energy-intensive sectors. On average over the six years following displacement, earnings losses are 7 percentage points higher in energy-intensive sectors than in the rest of the economy (which corresponds to a 24% difference in proportional terms).
However, there are important differences within the energy-intensive sector between sub-sectors. For example, the largest losses are observed in heavy manufacturing, where workers lose 38% of their earnings relative to non-displaced workers on average over the six years following job loss. In contrast, in transport the average earnings losses over the six years following displacement are similar to those in the rest of the economy. While the average losses following displacement in the energy supply sector are also larger than in the rest of the economy, they are not statistically. This partly reflects larger confidence intervals due to small sample sizes (see Figure 2) and partly heterogeneity in the direction and size of earnings losses across countries (see the next sub-section).
Overall, our aggregate results across countries suggest that displacement in energy supply and heavy manufacturing is associated with substantially higher costs than those in other sectors, including transport. These results are mirror those in OECD (2024[1]) and echo findings from Barreto, Grundke, & Krill (2023[9]), which also demonstrate that workers displaced from carbon-intensive industries in Germany, overlapping entirely with energy-intensive industries used here, experience higher costs of job displacement compared to other sectors. For energy-supply workers, the results are in line with previous findings for displaced workers in the coal sector. For example, Haywood, Janser, & Koch (2023[13]), Andrews, Dwyer and Vass (2023[14]) and Rud et al. (2022[15]) find elevated earnings losses for displaced coal workers in respectively Germany, Australia and the United Kingdom.
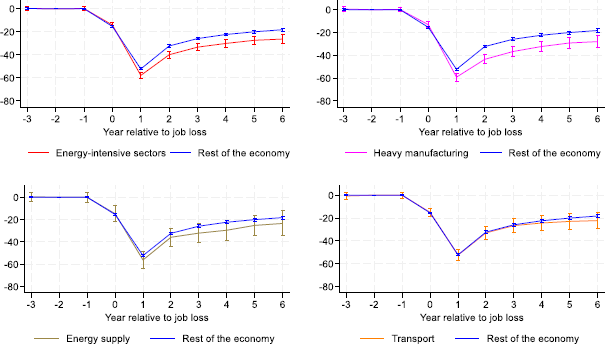
Note: Average across countries of earnings losses of displaced workers relative to observationally comparable non-displaced workers. “Rest of the economy” refers to non-energy-intensive sectors. The point estimates show the impact of job loss on earnings in event time, where workers are displaced between time 0 and time 1, such that time 1 is the first post-displacement year. The reference period for earnings losses is k=-2. Point estimates and 90% confidence intervals (clustered at the worker level) from country-level regressions are averaged assigning each country an equal weight. *The countries included are: Australia, Austria, Canada, Denmark, Estonia, Finland, Germany, Hungary, the Netherlands, Norway, Portugal, Spain, France, Sweden. For the energy supply sector, the number of laid off workers in Denmark, Estonia, France, the Netherlands and Spain falls below the 100-person threshold, in Portugal below the 10-firm threshold and these countries are therefore excluded from the energy supply average.
Source: National linked employer employee data, see Table 1 for details.
The cost of job displacement in energy-intensive industries by country
Differences in the size of earnings losses between workers in energy-intensive industries and those in the rest of the economy can differ across countries and specific energy-intensive industries. For ease of exposition, the size of the effects is compared across countries and industries by focusing on the average effects of job displacement during the six years following job displacement. The results are summarised in Figure 3.
There is wide variation across countries in the difference in average earnings losses between energy-intensive sectors and the rest of the economy.[17] For example, workers in the energy-intensive industries of Spain have on average about 15 percentage points higher earnings losses than workers from the rest of the economy, closely followed by France with a difference of 13 p.p.. With less than 5 p.p., differences in the earnings losses over six years following displacement are particularly small in Australia, Canada, Germany, the Netherlands, Portugal and Sweden.
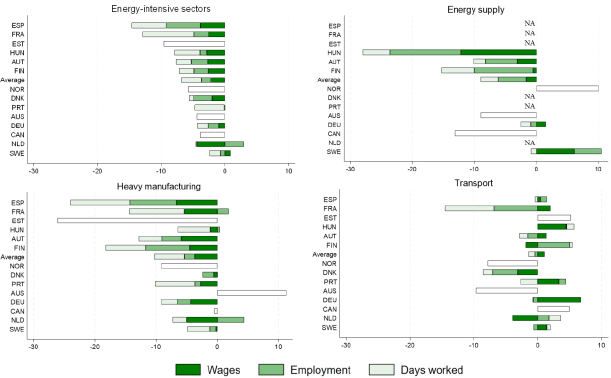
Note: Bars represent the average earnings loss six years after displacement, decomposed into the contribution of changes in daily wages, employment probability and days worked. The residual component is negligible and thus omitted for presentational purposes. Finland is missing from this figure due to missing information at the time of this draft. For Denmark, Estonia, France, the Netherlands and Spain, the number of laid off workers energy supply sectors falls below the 100-person threshold, in Portugal below the 10-firm threshold and results for this sector are therefore not presented. Average across the countries shown.
Source: National linked employer employee data, see Table 1 for details.
There are also important differences in the pattern of earnings losses across energy-intensive industries between countries. For instance, in energy supply, earnings losses are 28 p.p. larger in Hungary than the rest of the economy, but about 10 p.p. smaller in Norway and Sweden. This may in part reflect particularities in the national energy mix, as Sweden pre-dominantly produces energy through renewables, whereas Hungary relies predominantly on energy generation through fossil fuels (IEA, 2024[16]).[18] In heavy manufacturing, earnings losses for displaced workers are 26 p.p. higher than the rest of the economy in Estonia, while in Australia they are 11 p.p. lower. Even though our previous aggregate findings suggest that there are no elevated earnings losses in the transport sector, there are notable differences within specific countries. For example, transport workers in France face earnings losses nearly 13 p.p. higher than those in in the rest of the economy, whereas in Germany, displaced transport workers face about 6 p.p. lower losses than those in the rest of the economy.
The sources of earnings losses in energy-intensive industries by country
Earnings losses following displacement stem from different underlying causes, that can vary in importance by country and sector. Decomposing the earnings losses after job displacement into their contributing factors – namely (i) being out of work for an entire year, (ii) fewer days worked, conditional on being employed at some point during the year,[19] and (iii) lower daily wages upon re-employment – can thus shed light on the underlying drivers of job displacement costs for workers in energy-intensive industries and the rest of the economy. Figure 3 provides a decomposition of average earnings losses in energy-intensive sectors over a six-year period compared to the rest of the economy.
Larger earnings losses due to job displacement in energy-intensive sectors mainly reflect weaker re-employment outcomes, notably differences in the number of days worked and re-employment wages rather than differences in the likelihood of being in employment. However, these margins differ considerably across countries. In France, significant differences in earnings losses are primarily driven by differences in days worked, whereas in Austria and Spain, all three components contribute more or less equally to the elevated in earnings losses in energy-intensive industries. The Netherlands stands out as differences in employment probabilities reduce rather than increase the gap in earnings losses between displaced workers in energy-intensive and the rest of the economy.
The sources of differences in earnings losses differ to some degree across energy-intensive sectors. In energy-supply, earnings losses are on average driven in equal parts by differences in employment, days worked, and re-employment wages, though there is considerable variation across countries. For example, days worked explain virtually nothing of the differences in earnings losses in Sweden. In heavy manufacturing, the contribution of employment, days worked and re-employment wages is of roughly equal to that in energy-intensive industries as a whole, while re-employment wages play a relatively small role in the differences in earnings losses in Denmark, Hungary, and Sweden. In transport, there is strong variation in the contributing factors to differences earnings losses across countries. For example, transport workers in France experience earnings losses that are for the most part driven by differences in employment and days worked, while German transport workers see higher re-employment wages after displacement.
Firm- and worker-related wage losses in energy-intensive industries by country
Wage losses in re-employment are an important part of the differences in earnings losses between energy-intensive sectors and the rest of the economy, contributing on average to about a quarter of the overall difference in earnings losses. The origin of these wage losses can stem from firm-related losses due to foregone firm wage premia and worker-related losses due to human capital depreciation and lower match quality (Lachowska, Mas and Woodbury, 2020[7]). The results are summarised in Figure 4.
Higher wage losses in energy-intensive sectors tend to be firm-related. This may indicate that workers in energy-intensive sectors may undergo larger earnings losses in part because they were displaced from higher paying firms. These may be either firms that are more productive, capture more rents, or compensate workers for physically demanding working conditions by paying higher wages (Card et al., 2018[17]; Sorkin, 2018[18]; Hirsch and Mueller, 2020[19]). Differences in worker-related wage losses either tend to be small or negative (i.e. lower in energy-intensive industries). Only in Finland and Portugal, are larger wage losses in energy-intensive industries mainly worker-related.
The importance of firm-related wage losses to differences in re-employment wages in energy-intensive industries tends to be driven by heavy manufacturing, while in energy supply, wage losses tend to reflect worker-related rather than firm-related factors. In transport, wage losses due to job displacement tend to be smaller than in the rest of the economy due to smaller worker-related wage losses. While the pattern for heavy manufacturing is strikingly consistent across countries, the patterns for energy supply and transport are more mixed.
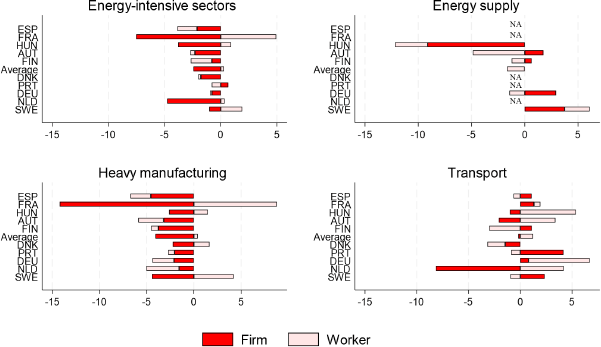
Note: Bars represent the average wage loss six years after displacement, decomposed into the contribution of firm wage premia changes, and worker-related (i.e. match quality and human capital changes) following Lachowska et al. (2020). The chart excludes countries for which daily wages cannot be calculated. For Denmark, Estonia, France, the Netherlands and Spain, the number of laid off workers energy supply sectors falls below the 100-person threshold, in Portugal below the 10-firm threshold and results for this sector are therefore not presented. Average across the countries shown.
Source: National linked employer employee data, see Table 1 for details
The effects of job displacement on job mobility in energy-intensive industries
The clean energy-transition and its potential impact on employment in energy-intensive industries may mean that displaced workers find it harder to find another job in the same sector, occupation or region. However, switching sector or occupation may entail significant additional costs due to the loss of occupation- and sector-specific human capital (Huckfeldt, 2022[20]; Barreto, Grundke and Krill, 2023[9]). Changing region, by contrast, may enhance employment opportunities, potentially mitigating earnings losses after displacement (Arntz, Ivanov and Pohlan, 2022[21]). Regional mobility from rural to urban areas in particular may also help mitigate the earnings losses of job displacement (Huttunen, Moen and Salvanes, 2018[22]; Meekes and Hassink, 2019[23]). To understand how mobility patterns interact with the cost of job loss in energy-intensive sectors, Figure 5 presents the effect of job loss on the likelihood of switching sectors, occupations and regions in energy-intensive and non-energy-intensive industries on average over six years following job loss.
Workers in energy supply and heavy manufacturing are more likely to change sector and occupation after displacement than workers in non-energy-intensive industries (Figure 5). This may reflect the structural decline in employment in those sectors. The higher incidence of switching sectors and occupations and the associated loss of sector-specific human capital may help explain why displaced workers in those sectors tend to suffer larger earnings losses and weaker re-employment outcomes. In contrast, workers displaced in transport are less likely to change occupations and sectors than workers displaced in non-energy-intensive industries as well as the other energy-intensive industries. This indicates that displaced workers in transport find jobs that are similar to those from which they were displaced and in turn may explain why they experience lower earnings losses. Strikingly, regional mobility does not significantly differ across energy-intensive industries and the rest of the economy.
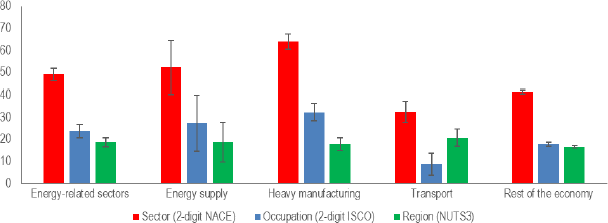
Note: Average across Austria, Portugal, Germany, Spain, Estonia, Hungary, Finland, France, Sweden. For occupational and regional changes, the figure excludes Austria and Estonia. 90% confidence intervals clustered at the worker level.
Sources: National linked employer employee data, see Table 1 for details.
Potential mechanisms behind within-country differences in earnings losses
Although the costs of job displacement are estimated by comparing observationally comparable displaced and non-displaced workers before and after mass-layoff events, there can still be significant differences between displaced workers from energy-intensive industries and those from other sectors in the economy. This raises the question to what extent differences in the composition of firms and workers between industries contribute to the variation in job displacement costs. To determine the extent of these composition effects on the differences in job displacement costs within countries, Figure 6 presents an Oaxaca-Blinder decomposition that takes into account both individual and firm characteristics.
The composition of workers and firms is a key determinant for the differences in the costs of job displacement between each of the energy-intensive sectors and the rest of the economy, explaining at least half of the differences earnings losses. In each of the energy-intensive industries, displaced workers tend to be older, have lower levels of portable skills (as measured by the worker-related component of wages) and tend be employed in firms that offer high wages, conditional on worker skills, which are lost upon re-employment. The main reason why displaced workers in transport do not experience higher earnings losses than displaced workers in the rest of the economy appears to be related to tenure. Whereas displaced workers in heavy manufacturing and energy supply tend to have somewhat higher tenure than displaced workers in the rest of the economy, contributing to higher earnings losses, in transport displaced workers tend to have shorter tenures relative to other energy-intensive sectors as well as the rest of the economy, reducing earnings losses.
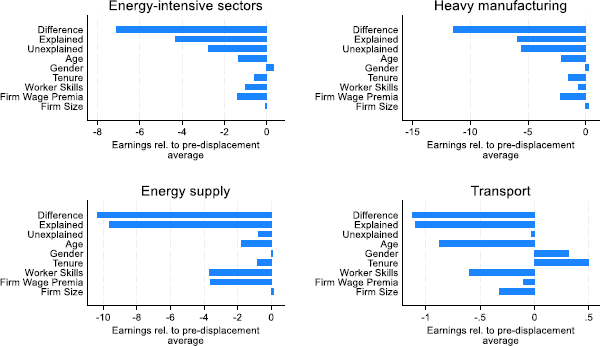
Note: The figure refers to within-country differences in job displacement costs between workers in each energy-intensive industry and the rest of the economy (reference group_ and decomposes the difference in mean earnings losses within a country between sectors net of year effects. Worker skills are measured by the worker-related wage component, whereas firm wage premia are measured by the firm-related wage component. Both are estimated using two-way fixed effects wage models (Abowd, Kramarz and Margolis, 1999[12]). The decomposition excludes Australia and Canada as it is not possible to estimate two-way fixed effects for firms and workers due to data limitations. For Denmark, Estonia, France, the Netherlands and Spain, the number of laid off workers energy supply sectors falls below the 100-person threshold, in Portugal below the 10-firm threshold and results for this sector are therefore not included in the average.
Source: National linked employer employee data, see Table 1 for details
5. Policy implications
While the clean energy transition is crucial for a sustainable future, it poses significant employment challenges, in part through significant and persistent job displacement costs in energy-supply and industrial-demand sectors. Strategic long-term planning can help to address the labour market challenges posed by the clean energy transition, yet some level of displacement is unavoidable. Consequently, developing and implementing comprehensive labour market policies that reduce the costs of job displacement is essential for assisting displaced workers in sectors and occupations at risk and ensuring transitions to new and emerging sectors and occupations, for example occupations in sectors relying on clean energy. However, it is also critical for securing public support for the clean energy transition (see OECD (2024[1]) for a comprehensive overview of policy approaches).
Income support during periods of non-employment after displacement plays a central role in cushioning earnings losses after job loss. Unemployment insurance (UI) in particular serves as a crucial safety net, supporting consumption and allowing time for displaced workers to find suitable employment (OECD, 2023[]). However, the effectiveness of UI depends on careful design to balance income security with the incentives for job search. In some countries, severance pay plays an important role in offsetting earnings losses following displacement, especially for workers with longer tenures that previously earned relatively high wages (OECD, 2018[]). While early retirement schemes can in principle can mitigate adverse labour market outcomes by offering a swift transition to pensions, they negatively impact aggregate labour supply and public finances (OECD, 2018[]), and are therefore not a strategically sound approach for the clean energy transition.
As a significant part of earnings losses following displacement in energy-intensive industries stems from a decline in re-employment wages, an additional avenue in supporting displaced workers is through in-work income supports, such as in-work benefits (see e.g. Immervoll and Pearson (2009[])) and wage insurance schemes. The latter, which replaces a significant part of the differences to previous wages, has already shown to be a particularly effective tool to mitigate job displacement costs. In the United States, for example, it has led to faster re-employment and increased cumulative long-run earnings of trade-displaced workers, while paying for itself through reduced public expenditure on UI and increased tax receipts (Hyman, Kovak and Leive, 2023[]; Hyman et al., 2021[]). Whether such policies should be targeted to support workers specifically affected by the clean energy transition depends on the country context.
To support transition from declining sectors to new and emerging industries, which may require re- or upskilling, active labour market policies (ALMPs) and lifelong learning initiatives play a significant role, e.g. Causa et al (2024[]). Public employment services are instrumental in this context, providing job search assistance and identifying relevant training opportunities based on systematic skills assessment and anticipation, ensuring workers can adapt to new job requirements in an evolving labour market (OECD, 2023[]). Such approaches may be most effective when implemented as early intervention that offer training and job search advice already during notice periods and before effective job loss (OECD, 2018[]).
References
[12] Abowd, J., F. Kramarz and D. Margolis (1999), “High Wage Workers and High Wage Firms”, Econometrica, Vol. 67/2, pp. 251-333, https://doi.org/10.1111/1468-0262.00020.
[14] Andrews, D., E. Dwyer and L. Vass (2023), “At the coalface: What happens to workers displaced by”, e61 Micro Notes, Vol. 11, https://e61.in/wp-content/uploads/2023/10/the_coal_face-5.pdf.
[21] Arntz, M., B. Ivanov and L. Pohlan (2022), “Regional structural change and the effects of job loss”, IAB-Discussion Paper, Vol. 17.
[11] Austin, P. (2011), “An Introduction to Propensity Score Methods for Reducing the Effects of Confounding in Observational Studies”, Multivariate Behavioral Research, Vol. 46/3, pp. 399-424, https://doi.org/10.1080/00273171.2011.568786.
[9] Barreto, C., R. Grundke and Z. Krill (2023), “The cost of job loss in carbon-intensive sectors: Evidence from Germany”, OECD Economics Department Working Papers, Vol. No. 1774.
[4] Barreto, C., Z. Kril and R. Grundke (2023), “Displacement Effects in Carbon-Intensive Sectors”, OECD Economics Department Working Papers, Vol. No. 1774, https://doi.org/10.1787/6f636d3b-en.
[2] Borgonovi, F. et al. (2023), “The effects of the EU Fit for 55 package on labour markets and the demand for skills”, OECD Social, Employment and Migration Working Papers, No. 297, OECD Publishing, Paris, https://doi.org/10.1787/6c16baac-en.
[33] Callaway, B. and P. Sant'Anna (2021), “Difference-in-Differences with multiple time periods”, Journal of Econometrics, Vol. 225/2, pp. 200-230, https://doi.org/10.1016/j.jeconom.2020.12.001.
[17] Card, D. et al. (2018), “Firms and Labor Market Inequality: Evidence and Some Theory”, Journal of Labor Economics, Vol. 36/S1, pp. S13-S70, https://doi.org/10.1086/694153.
[5] Card, D., J. Heining and P. Kline (2013), “Workplace Heterogeneity and the Rise of West German Wage Inequality*”, The Quarterly Journal of Economics, Vol. 128/3, pp. 967-1015, https://doi.org/10.1093/qje/qjt006.
[35] Causa, O., M. Nguyen and E. Soldani (2024), “A new measurement approach for identifying high-polluting jobs across European countries”, OECD Economics Department Working Papers, No. 1795, OECD Publishing, Paris, https://doi.org/10.1787/f5127e4c-en.
[37] Causa, O., M. Nguyen and E. Soldani (2024), “Lost in the green transition? Measurement and stylized facts”, OECD Economics Department Working Papers, No. 1796, OECD Publishing, Paris, https://doi.org/10.1787/dce1d5fe-en.
[29] Causa, O. et al. (2024), “Labour markets transitions in the greening economy: Structural drivers and the role of policies”, OECD Economics Department Working Papers, No. 1803, OECD Publishing, Paris, https://doi.org/10.1787/d8007e8f-en.
[39] D'Arcangelo, F., T. Kruse and M. Pisu (2023), “Identifying and tracking climate change mitigation strategies: A cluster-based assessment”, OECD Economics Department Working Papers, No. 1786, OECD Publishing, Paris, https://doi.org/10.1787/a23b43c5-en.
[32] de Chaisemartin, C. and X. D'Haultfœuille (2020), “Two-Way Fixed Effects Estimators with Heterogeneous Treatment Effects”, American Economic Review, Vol. 110/9, pp. 2964-2996, https://doi.org/10.1257/aer.20181169.
[3] European Commission JRC (2019), World input-output database environmental accounts: update 2000-2016, https://data.europa.eu/doi/10.2760/024036.
[13] Haywood, L., M. Janser and N. Koch (2023), “The welfare costs of job loss and decarbonization – evidence from Germany's coal phase out”, Journal of the Association of Environmental and Resource Economists, https://doi.org/10.1086/726425.
[10] Hethey-Maier, T. and J. Schmieder (2013), Does the Use of Worker Flows Improve the Analysis of Establishment Turnover? Evidence from German Administrative Data, National Bureau of Economic Research, Cambridge, MA, https://doi.org/10.3386/w19730.
[19] Hirsch, B. and S. Mueller (2020), “Firm Wage Premia, Industrial Relations, and Rent Sharing in Germany”, ILR Review, Vol. 73/5, pp. 1119-1146, https://doi.org/10.1177/0019793920917105.
[20] Huckfeldt, C. (2022), “Understanding the Scarring Effect of Recessions”, AMERICAN ECONOMIC REVIEW, Vol. 112/4.
[22] Huttunen, K., J. Moen and K. Salvanes (2018), “Job Loss and Regional Mobility”, Journal of Labor Economics, Vol. 36/2.
[27] Hyman, B., B. Kovak and A. Leive (2023), “Wage Insurance for Displaced Workers”, Working Paper.
[28] Hyman, B. et al. (2021), “Wage Insurance and Labor Market Trajectories”, AEA Papers and Proceedings, Vol. 111, pp. 491-495, https://doi.org/10.1257/pandp.20211093.
[16] IEA (2024), Energy Statistics Data Browser, https://www.iea.org/data-and-statistics.
[26] Immervoll, H. and M. Pearson (2009), “A Good Time for Making Work Pay? Taking Stock of In-Work Benefits and Related Measures across the OECD”, OECD Social, Employment and Migration Working Papers, No. 81, OECD Publishing, Paris, https://doi.org/10.1787/225442803245.
[6] Jacobson, L., R. Lalonde and D. Sullivan (1993), “Earnings Losses of Displaced Workers”, American Economic Review, Vol. 83, pp. 685-709.
[38] Keese, M. and L. Marcolin (2023), “Labour and social policies for the green transition: A conceptual framework”, OECD Social, Employment and Migration Working Papers, No. 295, OECD Publishing, Paris, https://doi.org/10.1787/028ffbeb-en.
[34] Krolikowski, P. (2017), “Choosing a Control Group for Displaced Workers”, ILR Review, Vol. 71/5, pp. 1232-1254, https://doi.org/10.1177/0019793917743707.
[7] Lachowska, M., A. Mas and S. Woodbury (2020), “Sources of Displaced Workers' Long-Term Earnings Losses”, American Economic Review, Vol. 110/10, pp. 3231-3266, https://doi.org/10.1257/aer.20180652.
[23] Meekes, J. and W. Hassink (2019), “The role of the housing market in workers′ resilience to job displacement after firm bankruptcy”, Journal of Urban Economics, Vol. 109, pp. 41-65, https://doi.org/10.1016/J.JUE.2018.11.005.
[1] OECD (2024), Job Displacement in High-Emission Industries: Implications for the Net-Zero Transition, OECD.
[36] OECD (2024), OECD Employment Outlook 2024: The Net-Zero Transition and the Labour Market, OECD Publishing, Paris, https://doi.org/10.1787/ac8b3538-en.
[24] OECD (2023), “Income support for jobseekers: Trade-offs and current reforms”, Employment, Labour and Social Affairs Policy Briefs, OECD, Paris, https://www.oecd.org/employment/Income-support-for-jobseekers-Trade-offs-and-current-reforms.pdf.
[30] OECD (2023), Job Creation and Local Economic Development 2023: Bridging the Great Green Divide, OECD Publishing, Paris, https://doi.org/10.1787/21db61c1-en.
[25] OECD (2018), Good Jobs for All in a Changing World of Work: The OECD Jobs Strategy, OECD Publishing, Paris, https://doi.org/10.1787/9789264308817-en.
[31] OECD (2018), OECD Employment Outlook 2018, OECD Publishing, Paris, https://doi.org/10.1787/empl_outlook-2018-en.
[15] Rud, J. et al. (2022), “Job Displacement Costs of Phasing Out Coal”, IZA DIscussion Papers, Vol. 15581, https://docs.iza.org/dp15581.pdf.
[8] Schmieder, J., T. von Wachter and J. Heining (2023), “The Costs of Job Displacement over the Business Cycle and Its Sources: Evidence from Germany”, American Economic Review, Vol. 113/5, pp. 1208-1254, https://doi.org/10.1257/aer.20200252.
[18] Sorkin, I. (2018), “Ranking Firms Using Revealed Preference*”, The Quarterly Journal of Economics, Vol. 133/3, pp. 1331-1393, https://doi.org/10.1093/qje/qjy001.
Annex A. Copyright and Disclaimers Notices
Australia
The following Disclaimer Notice refers to data and graphs sourced from the Australian Bureau of Statistics' BLADE (Business Longitudinal Analysis Data Environment) database.
The results of these studies are based, in part, on data supplied to the ABS under the Taxation Administration Act 1953, A New Tax System (Australian Business Number) Act 1999, Australian Border Force Act 2015, Social Security (Administration) Act 1999, A New Tax System (Family Assistance) (Administration) Act 1999, Paid Parental Leave Act 2010 and/or the Student Assistance Act 1973. Such data may only be used for the purpose of administering the Census and Statistics Act 1905 or performance of functions of the ABS as set out in section 6 of the Australian Bureau of Statistics Act 1975. No individual information collected under the Census and Statistics Act 1905 is provided back to custodians for administrative or regulatory purposes. Any discussion of data limitations or weaknesses is in the context of using the data for statistical purposes and is not related to the ability of the data to support the Australian Taxation Office, Australian Business Register, Department of Social Services and/or Department of Home Affairs' core operational requirements.
Legislative requirements to ensure privacy and secrecy of these data have been followed. For access to MADIP and/or BLADE data under Section 16A of the ABS Act 1975 or enabled by section 15 of the Census and Statistics (Information Release and Access) Determination 2018, source data are de-identified and so data about specific individuals has not been viewed in conducting this analysis. In accordance with the Census and Statistics Act 1905, results have been treated where necessary to ensure that they are not likely to enable identification of a particular person or organisation.
Acknowledgements
This work was carried out under the supervision of Stefano Scarpetta (Director of the OECD Directorate for Employment, Labour and Social Affairs) and Stéphane Carcillo (Head of the OECD Jobs and Income Division).
The paper benefitted from insights and helpful comments from Stefano Scarpetta, Stéphane Carcillo, Andrea Bassanini and Andrea Garnero (OECD Directorate for Employment, Labour and Social Affairs), Emilia Soldani (OECD Economics Department) and several other OECD colleagues. The authors are grateful to delegates to the OECD Working Party on Employment and participants of the OECD Workshop on Greening Labour Markets (20 October 2023), the IEA workshop on Measuring Affordability and the Social Impacts of Clean Energy Transitions (28-29 March 2024), IZA/OECD workshop on Applications Using Linked Employer-Employee Data (9-10 April 2024) and IZA/OECD workshop on Climate Change and the Labour (25 April 2024).
The paper provides additional background to a chapter in OECD (2024[1]) on job displacement in high emission industries with respect to the methodology and the effects of job-displacement across energy-intensive sub-sectors (energy supply, heavy manufacturing and transport). It is part a broader OECD project that mobilises linked employer-employee data for cross-country research and policy analysis (LinkEED 2.0). For more details, please visit: https://www.oecd.org/en/about/projects/linkeed-200.html.
Footnotes
Recent OECD work on the labour market effects of the transition towards greater carbon- and resource-efficiency and related labour and social policy responses include OECD (2024[]; 2023[]), Keese and Marcolin (2023[]), Borgonovi et al. (2023[2]), Causa et al. (2024[]) and Causa, Nguyen and Soldani (2024[]; 2024[]). [1]
Some of this restructuring will take place within a given sector (and even firm), with changes in power generation technologies being an obvious example. [2]
As discussed in more detail below, job displacement in the present paper refers to workers separations from firms engaged in mass layoff events. [3]
For a discussion of the measurement of polluting and energy-intensive occupations, see OECD (2024[]) and Causa, Nguyen and Soldani (2024[]). [4]
This approach is comparable to those using GHG-intensity in OECD (2024[1]) and carbon-intensity in Barreto, Krill and Grundke (2023[4]) with the exception that we use a threshold of appearing in the top two deciles of the energy-intensity distribution in at least 15 countries as compared to 10 in the other two classifications. This ensures that the sewerage and waste-collection sector as well as the agricultural sector are omitted from the list of energy-related industries, as these are expected to grow and/or are essential for the clean-energy transition (see also OECD (2024[1])). [5]
Using the same approach for GHG-intensive sectors instead yields two differences. The sewerage and waste collection sector and the agriculture sector are both characterised by relatively high emissions but low energy-intensity. However, in the final classification of high emission sectors in OECD (2024[1]) they are omitted as they are not expected to decline. This partly reflects the role of policies since sewerage and waste collection is very needed for the net-zero transition (D'Arcangelo, Kruse and Pisu, 2023[]). [6]
It is important to note that the present classification is based exclusively on energy demand but – partly by coincidence – also captures key aspects of energy supply. This is most obvious in the case of energy supply. Coke and refined petroleum is energy-intensive because it uses high levels of energy per unit of valued and not because its main activity is energy generation. To a certain extent the same applies to also to energy-intensive manufacturing and services. For example, non-metallic mineral products are energy-intensive in production, but are also potentially relevant for energy supply, e.g. through manufacturing of relevant products derived from critical minerals. Similarly, the definition of energy-intensive service sectors also incorporates parts of the economy directly related to energy supply in the form of distribution (e.g. pipelines in land transport, oil freight in sea transport). [7]
In the event a worker has several jobs with identical total earnings, the spell that occupies most of the worker's time is selected. If total time also identical or no information on working time is not available, the primary job is based on a random draw among those with the highest total earnings (or time worked). [8]
Countries where firm identifiers are used are Australia, Canada, Estonia, the Netherlands and Portugal. [9]
All monetary values are expressed in real terms using the Consumer Price Index with 2015 as the base year. [10]
We account for implausibly low and high earnings by removing employment spells with earnings falling below 20% of median earnings and winsorising earnings at the top 0.1% of the distribution. This adjustment is done based on daily wages, whenever available, annual earnings otherwise. Daily wages are used instead of hourly wages to maximize country coverage. [11]
Note that when we refer to “rest of the economy” we refer to non-energy-related sectors throughout this paper. [12]
We use an employment threshold of 30 instead of 50 as done by Jacobson et al. (1993) to allow for a sufficiently large sample in each of the sub-sectors. [13]
OECD (2024[1]) presents a robustness check including workers aged 50-60. The results are qualitatively similar, with slightly larger earnings losses in both energy-intesive industries and the rest of the economy, while the diffience between the sectorsremain the same. For this reason, and in order to minimize the impact of differences in early retirement schemes across countries, our baseline sample includes workers up to 50 years of age at separation. [14]
The latter prevents “forbidden comparisons” of treated units with units that were treated in earlier periods (de Chaisemartin and D'Haultfœuille, 2020[]; Callaway and Sant'Anna, 2021[]) while the former avoids overestimating displacement effects when restricting the control group to workers who remain continuously employed with the same employer (Krolikowski, 2017[]). [15]
In practice, we estimate the following AKM model: , where wit is the log wage of worker i in year t. Worker fixed effects for each worker i are captured by while captures the firm fixed effects which reflect employer-specific wage premia in each establishment (firm) J of worker i in year t. Year fixed effects are captured through Yt, while Xit includes a cubic in age interacted with gender dummies. In the estimation of this model, we exclude post-displacement observations of treated and matched control units to avoid these transitions from impacting the estimation of firm effects. [16]
A negative bar indicates that earnings losses are on average higher for those in energy-related industries over a 6-year period. [17]
A higher share of renewables in the energy mix may mean that there is less of a reason for mass layoffs in the clean energy transition. In contrast, a larger share of fossil fuels in the energy mix would require a stronger degree of restructuring of energy systems to realize the clean energy transition, leading to more job displacement and potentially adverse labour market outcomes compared to workers in non-energy-related industries. [18]
Differences in days worked in the first year after displacement are mainly related to the return to employment later in the year (i.e. after 1 January), whereas differences in subsequent years mainly reflect lower job stability upon re-employment. [19]