Research Discussion Paper – RDP 2024-06 Examining the Macroeconomic Costs of Occupational Entry Regulations
September 2024
1 Little is known about the economic costs of OER in Australia
1.1 OER are widespread in Australia
OER set requirements that workers must meet to practice in a particular occupation. A common example of such an entry regulation is a licence—a government regulation that requires a person to obtain permission to do the activities associated with an occupation (e.g. doing electrical work). The nature of these entry regulations differs across occupations, ranging from maintaining membership in professional organisations to formal education and training.
OER, and licensing in particular, are widespread. In Australia, around one in five workers were subject to occupational licensing requirements in 2011 (Commonwealth Productivity Commission 2015). Similar levels have been estimated in the European Union (von Rueden and Bambalaite 2020). In the United States, OER have become more widespread where around one in four workers need to hold an occupational licence, up from around one in twenty in the 1950s (Kleiner and Krueger 2008).
In Australia, OER are often applied inconsistently across states and territories. A Commonwealth Productivity Commission study of nearly 100 occupations found that over 70 were not consistently licensed in all jurisdictions (Commonwealth Productivity Commission 2008). Even where states and territories are broadly aligned on the use of an occupational licence, there can be significant differences in terms of minimum requirements, regulatory frameworks, legislative instruments, terminology, and the classes or categories of work that are regulated (Commonwealth Productivity Commission 2023).
1.2 Recent evidence indicates there are economic costs to OER
There is growing awareness about the economic costs of the high and growing prevalence of occupational licensing requirements and other OER. OER are intended to protect consumers by ensuring practitioners have at least a minimum standard of training (Leland 1979); however, evidence from a sample of European countries suggests they have raised barriers to entry and decreased competition, which has led to lower productivity and higher prices in the economy (e.g. Bambalaite et al. 2020). They also have the potential to make it harder for the economy to adjust to economic shocks and structural change, contributing to skills shortages and higher structural unemployment. These concerns have grown given the declines in productivity growth and measures of economic dynamism (Akcigit and Ates 2021), such as the entry and exit of businesses observed over recent decades.
Nevertheless, there has been little research that examines the potential economic costs of OER in Australia. Previous work has been limited to measuring the stringency of OER in Australia for a narrow range of occupations in New South Wales and Queensland (Barker 2022). While extremely valuable, such work must rely on international estimates of the costs of OER. Examining the economic costs of OER in an Australian context therefore remains important, as it is not a given that findings from other countries can be applied to Australia due to the differing nature of OER, labour markets, and other institutional factors (Commonwealth Productivity Commission 2023).
1.3 This paper explores the costs of OER in Australia
This paper helps fill this gap. First, it applies the indicator of OER stringency developed by the Organisation for Economic Co-operation and Development (OECD) to a wide range of occupations in New South Wales, Queensland, and Victoria.[1] This allows us to assess how the stringency of OER in Australia compares across states and to other countries, as well as considering what aspects of policy are contributing most to the stringency of OER.
We then explore the relationship between OER and several measures of business dynamism, specficially rates of firm entry and exit, and the rate at which labour flows from less to more productive firms. We focus on economic dynamism for three reasons:
- There are strong reasons to believe that OER make it more costly for new businesses to enter, and they can limit the drive for existing businesses to adjust staff numbers.
- Overseas analysis has found evidence of a relationship between OER and these metrics (Bambalaite et al. 2020).
- Considering the effects of OER offers an important avenue to help understand why measures of economic dynamism have been declining over recent decades in Australia, which has had negative implications for productivity and living standards (e.g. Andrews and Hansell 2021).
Finally, we explore the relationship between the stringency of OER and the intensity of skills shortages. This provides some sense as to whether OER could contribute to greater mismatch of demand for, and supply of, labour, and thereby lead to higher structural unemployment and more generally impinge on economic growth.
The intent of this analysis is not to necessarily argue OER are bad, or too stringent. It is simply to try to fill a key gap in our knowledge by measuring OER stringency, and their components, across a range of occupations and states in Australia. In addition, we quantify some of the economic costs associated with OER. This can provide an important input into future research and policymakers' decisions and allow for a more complete understanding of the relative costs and benefits of various OER arrangements.
In addition, our findings can help researchers understand how changes in OER stringency may have affected the Australian economy in the past or may affect it going forward. To that end, future work that tracks the stringency of OER over time could be valuable in better understanding whether changes in OER contributed to the slowdown of business dynamism and productivity in Australia.
2 OER have costs and benefits
OER have costs and benefits, that may need to be weighed up when creating them. The main benefits largely stem from the potential to improve or maintain minimum quality standards. This can help provide consumers greater confidence to engage services and protect them, and workers, from direct harms.
The costs of OER include the direct costs to workers in needing to pay for the additional licensing. In addition, OER also impose indirect costs that can lead to higher prices and less choice for consumers. This can stem from reduced competition as it is more difficult for new firms to enter and expand, and for workers to move to where there are opportunities. For similar reasons, OER can also make it harder for the economy, businesses, and workers to respond to shifts in demand or technological change. The NSW Productivity Commission and NSW Innovation and Productivity Council (2022) also found that overly stringent OER can perversely incentivise consumers to use unlicensed services. These economic costs are fairly indirect and diffuse, relative to the benefits. As a result, they may be hard to assess when setting OER.
2.1 OER help maintain minimum quality standards
OER are intended to ensure goods and services meet a minimum quality, by ensuring that the workers producing them meet a minimum standard in terms of skills and training (Leland 1979). As consumers are sure that goods and services will be of a high quality, it will inspire greater confidence, benefiting both consumers and producers. Workers may also benefit from OER by ensuring that only qualified individuals can perform certain tasks that may pose a risk to worker safety.
These benefits are likely to be particularly large where the consumer cannot effectively assess the quality of the goods or services due to a lack of expert knowledge. That is, where there are informational asymmetries between the consumer and the provider. While some of these information asymmetries can be overcome by repeated interactions, or reputational mechanisms (e.g. online reviews), this is not always the case, nor is it always appropriate. For example, in some cases it may not be obvious whether the goods or services were of high quality for some time (e.g. the workmanship of a builder). Similarly, where poor-quality goods and services could lead to significant harm for consumers, relying on repeated interactions and reviews may not be desirable.
Not all OER are necessarily effective in maintaining or improving quality standards. Minimum qualification requirements do not guarantee that a practitioner will meet standards or deliver high quality services. And numerous studies looking at the effects of lowering OER failed to find evidence of a subsequent reduction in the quality of goods and services (see Bambalaite et al. 2020, annex C for a detailed summary of this literature).
That said, the lack of evidence of a benefit does not indicate that OER serve no purpose as quality is inherently difficult to measure (Commonwealth Productivity Commission 2023). In addition, it can be difficult to extrapolate findings from incremental changes, which are more common and tend to be used in assessing benefits, to more substantive changes in OER, particularly given the incremental changes that occur are likely to be relatively ‘safe’.
2.2 But OER can also work against consumer interests
OER create barriers to entry for workers and businesses looking to enter a profession or industry. This can have several direct and indirect costs.
First, by creating barriers to entry into professions, OER can create and exacerbate skill shortages. Differences in licensing regulation across jurisdictions makes it difficult (and costly) for individuals to move between states, countries, or professions: a study in the United States found state-specific licensing reduced interstate mobility of workers by 36 per cent (Johnson and Kleiner 2017). And within jurisdictions, OER can make it harder for people to change occupations. Both sets of frictions make it harder for the economy to adjust to economic shocks or structural changes. This can potentially contribute to higher levels of skills shortages and structural unemployment (i.e. workers can't find jobs because their skills do not match the jobs available).
Second, OER can also lower the overall number of providers, which can reduce competition leading to higher prices for consumers. For example, in the United States, licensing is estimated to have reduced labour supply by an average of 17 to 27 per cent across several occupations (Blair and Chung 2018). Similarly, across a broad range of countries more stringent OER are correlated with lower rates of business entry and exit – or dynamism, the sum of the two (Figure 1).
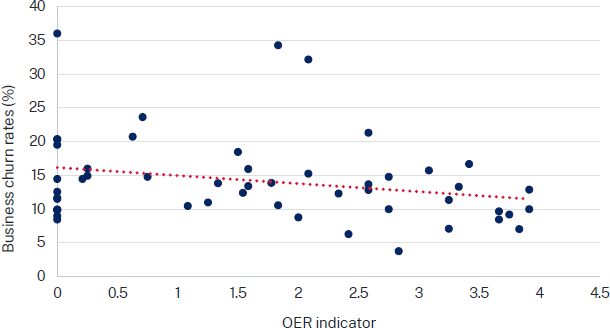
Note: Each dot represents one country-sector cell, where sectors correspond to one of the occupations covered by the OECD OER indicator (e.g. architectural activities in Spain). The indicator is scaled to take values between 0 (no regulation and 6 (fully regulated). Business churn is defined as the sum of enterprise births and enterprise deaths divided by the number of active enterprises, over the period 2014 to 2016. The set of countries used includes Belgium, Finland, France, Germany, Hungary, Italy, Portugal, Slovenia, Spain, Sweden, and the UK.
Source: Bambalaite et al. (2020).
Third, occupational licensing can also have implications for productivity growth as businesses may have reduced incentives to innovate. As discussed in Bambalaite et al. (2020), occupational licensing could cause individual businesses to be more or less productive. By ensuring that the average worker is better trained, it could raise the productivity of existing businesses. But at the same time, barriers to entry could weaken competitive pressures which can weaken businesses' incentives to improve (Andrews et al. 2022, Andrews and Hansell 2021). Bambalaite et al. (2020) find that firms' productivity growth tends to be lower where OER are more stringent, suggesting that the reduced incentive to innovate is the dominant channel.
Fourth, occupational licensing could also affect productivity by influencing the allocation of resources in the economy – that is, the extent to which resources such as labour are attached to the most productive businesses. One of the key drivers of productivity is the reallocation of resources from low- to high-productivity businesses, as high-productivity businesses thrive and grow and attract resources from lower-productivity businesses. Stringent OER could slow this process by making it harder for skilled professionals to move between industries or businesses, and by weakening competitive pressures and therefore the impetus for low-productivity firms to shrink and exit (e.g. De Loecker et al. 2021, Hambur 2023). Bambalaite et al. (2020) find evidence of such effects, with the flow of labour from low- to high-productivity firms tending to be slower in jurisdictions and occupations with more stringent OER.
Fifth, occupational licensing may affect productivity by limiting the ability of occupations to change and evolve as technology and consumer demand changes. Previous overseas research has demonstrated that the tasks required by narrowly defined occupations have changed significantly over time (Atalay et al. 2020). For example, bank tellers in the past primarily handled cash transactions but their role has shifted to include customer service, sales of financial products, and troubleshooting digital banking issues. By tightly defining the boundaries of occupations we may limit this natural transformation, which limits new and innovative industries and ways of working.
3 Measuring the stringency of OER
OER encompass a range of factors such as qualification pathways, licensing requirements and recognition of interjurisdictional requirements. Creating a single metric to quantify the stringency of OER is helpful in being able to readily compare OER across occupations and jurisdictions.
We adopt the approach laid out by the OECD in von Rueden and Bambalaite (2020) to create a single index to measure the stringency of OER. This provides a simple, single indicator of the stringency of OER that we can compare to other countries. The following sections describe the construction of the measures in more detail, as well as outlining the range of occupations to which we apply the index.
The OER indicator is constructed in two steps (see Box 1 for more details). First, we assess the burden imposed by the restriction on an occupation. The index captures three areas of the burden: the administrative burdens that need to be met, the qualification requirements, and any mobility restrictions across states or countries associated with the OER (e.g. Queensland licences are not automatically recognised in New South Wales, or vice versa). Each of these indicators is scored, then combined with an equal weight to construct an aggregate indicator for the occupation. This takes on a value between 0 (no regulation) and 6 (fully regulated).
In the second step, we scale the burden by a factor to reflect the type of OER used, as these differ in how binding they are. For example, licences are required to undertake certain protected activities, while certification is only needed to use a protected title. We scale down the OER indicator for the less binding types of OER.
Box 1: How the OER indicator is structured
Step 1: Collect and score information on regulatory entry barriers
The OER's burden is assessed along three regulatory areas (Table 1). Each area has several sub-indicators, which we score between 0 to 6 for each (see appendix A for the scoring index). Scores are then aggregated for each sub-indicator using equal weights, and then across the three areas using an equal weighting.
Regulatory area and weighting | Description | Sub-indicators |
---|---|---|
Administrative burden (33% weighting) |
Barriers to obtaining legal authorisation to practice |
|
Qualification requirements (33% weighting) |
Education requirements to enter an occupation |
|
Mobility restrictions (33% weighting) |
Barriers to labour mobility between jurisdictions |
|
Step 2: Assess the regulatory stringency by type of licence
The total score value of each regulated area is discounted based on the type of licence (Table 2). This reflects the fact that different types of arrangements are binding to different extents.
Licence (100%) | Supervisor licence (70%) | Certification (50%) | Unregulated (0%) |
---|---|---|---|
Practitioner must obtain legal authorisation to practice. E.g. only registered medical practitioners can use the protected title “Doctor” and undertake specialised activities under their registration. |
Practitioners can undertake the regulated activity under the supervision of a fully licensed professional. E.g. a tradesperson can undertake a regulated activity, such as carpentry, without a licence if they do so under the supervision of a licensed professional. |
Practitioners can voluntarily be certified to use a legally protected title, but no one is barred from practicing. E.g. a regulator only allows certified architects to use the protected title of “Architect” but anyone could theoretically carry out the activities associated with this profession. |
Practitioners can freely practice without any restriction. |
Source: von Rueden and Bambalaite (2020) adapted by NSW Treasury and the Reserve Bank of Australia. |
3.1 OER indicator estimated for a range of occupations in Australia's largest states
We calculate the OECD's OER measure for a range of occupation groups in New South Wales, Queensland, and Victoria. We start by focusing on the set of 15 OECD occupations considered in von Rueden and Bambalaite (2020) and Bambalaite et al. (2020) for New South Wales, Queensland, and Victoria (Table 3). This enables direct comparison of OER across Australia's largest states and with other OECD countries.
Personal services | Professional services |
---|---|
Aesthetician | Accountant |
Baker | Architect |
Butcher | Civil engineer |
Taxi driver | Lawyer |
Driving instructor | Real estate agent |
Electrician | |
Hairdresser | |
Painter/decorator | |
Plumber | |
Nurse | |
Note: The OECD defines personal services as those provided directly to individuals. Professional services meanwhile refer to specialised services provided by professionals with specific expertise to clients or businesses. Source: von Rueden and Bambalaite (2020) adapted by NSW Treasury and the Reserve Bank of Australia. |
We extend the analysis, measuring regulatory stringency for the top 100 most common occupations in New South Wales (see appendix B for the full list). This provides a broader view of the coverage and stringency of OER throughout the New South Wales economy, as well as the key components that feed into OER.
Finally, we conducted industry-specific analysis by measuring the regulatory stringency of 14 select occupations in the building and construction industry in New South Wales, Queensland, and Victoria. We do a deep dive into this sector given it consistently reports acute skill shortages, which has important implications for our ability to meet growing demand for housing and infrastructure (Housing Industry Association 2023). Moreover, it is a highly cyclical industry, meaning that OER may be particularly binding, preventing new workers from entering and exiting the construction sector as demand ebbs and flows.
A summary of the occupation groupings and states covered in the analysis is contained in Table 4. We use data available as at 2023.
Occupation group | New South Wales | Queensland | Victoria |
---|---|---|---|
1. OECD's 15 occupations | Applied | Applied | Applied |
2. 15 selected building and construction occupations | Applied | Applied | Applied |
3. Top 100 most common occupations in New South Wales | Applied | Not applied | Not applied |
Source: NSW Treasury and the Reserve Bank of Australia. |
The OER indicator rates the stringency of OER on a scale of 1 to 6, which enables us to assess how regulatory stringency compares across states and internationally. Table 5 provides an example of how the OER indicator was used to measure the stringency of occupational requirements for registered nurses across Australia under the nationally harmonised registration scheme. The scoring criteria and weighting for each regulated area and sub-indicator is outlined in appendix A. The scores reveal that registered nurses have an overall OER score of 2.33, with qualification requirements being the main contributed to this score.
Regulated Area | Sub indicator | Score |
---|---|---|
Administrative Burden (33%) | Limitation to number of authorisations granted | 0 |
Restrictions on territorial validity of professional qualification | 0 | |
Compulsory membership or registration in professional association | 6 | |
Regulated Area Weighted Score | 0.67 | |
Qualification Requirements (33%) |
Number of pathways to obtain qualification | 3 |
Requirement of university degree or vocational course | 3 | |
Requirement of compulsory practice |
6 | |
Mandatory state exam | 0 | |
Regulated Area Weighted Score | 1.00 | |
Mobility Restrictions (33%) |
Process for recognising qualifications gained abroad | 0 |
Local exam required to practice | 6 | |
Nationality or citizenship required to practice | 0 | |
Regulated Area Weighted Score | 0.67 | |
Total Weighted Score | 2.33 | |
Source: NSW Treasury and the Reserve Bank of Australia. |
3.2 OER indicator has some innate limitations
The OER indicator also has several limitations:
- Simplification: The regulatory areas are weighed equally, in line with the OECD method, to enable comparison. In reality, one might be a bigger constraint on another, and their relative importance might vary between occupations and jurisdictions.
- Coverage: OER measure does not consider the number of specific tasks that are restricted to each occupation, which means the actual stringency of regulations may not be accurate where reserved activities differ.
- Data quality and availability: Construction of the OER indicator is time-consuming and data availability issues may limit the extent to which the indicator can be estimated over time and across jurisdictions. The OER indicator may be mismeasured if data is limited or outdated.
For example, the typical four-year apprenticeship to become an electrician in New South Wales may present a bigger barrier for potential entrants than the mobility restrictions, such as the process for recognising qualifications gained abroad. The scope of work allowed for electricians can also vary across jurisdictions. Some areas may combine electrical and air conditioning and refrigeration work under a single licence, while others may require separate licences for each activity. Further, it can be challenging to find information on how mobility restrictions on electricians have changed over time.
In addition, the OER indicator cannot be used in isolation to determine whether certain requirements should be kept or removed. This assessment requires an evaluation of the costs and benefits, which is beyond the scope of a single indicator.
4 Documenting the stringency of OER across jurisdictions
This section examines patterns in OER across occupations and jurisdictions to better understand the nature of OER in Australia, and in New South Wales in particular. Reform opportunities can be identified by examining whether quality/standards have been comparable in other jurisdictions that have less stringent OER. These reform opportunities require further analysis that assesses the benefits of various OER, which is beyond the scope of this paper.
4.1 Australia's OER are more stringent for select occupations than other OECD countries
OER in Australia's largest states are more restrictive compared to the least stringent OECD countries, such as Sweden and Switzerland (Figure 2).[3] This suggests that there may be scope to reduce the stringency of OER in Australia if other jurisdictions have been able to achieve comparable or even better quality/safety outcomes.
Relative to the OECD average level, OER in Australia's three largest states tend to be more stringent in personal services – those provided directly to individuals, such as a baker or electrician – and less stringent for professional services occupations – specialised services provided by professionals with specific expertise to clients or businesses, such as a lawyer or accountant.
There are also differences across states, with OER tending to be more stringent in New South Wales, compared to Queensland and Victoria.
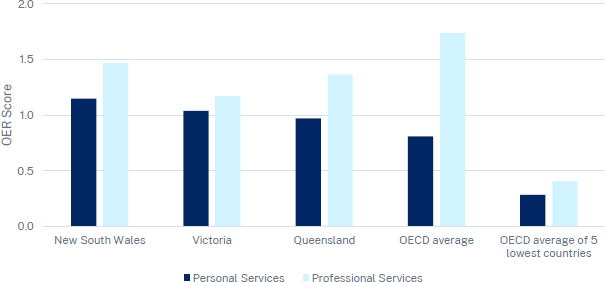
Note: Scores reflect the average aggregate scores for the ten personal service and five professional service occupations listed in Table 3.
Source: NSW Treasury and the Reserve Bank of Australia, von Rueden and Bambalaite (2020).
Focusing on occupations in New South Wales, OER are more stringent for hairdressers, electricians, driving instructors, architects, and real estate agents compared to other jurisdictions (Figure 3). This reflects more stringent qualification requirements and more onerous mobility restrictions across states and countries (Figure 4).
Ongoing efforts are underway to reduce mobility restrictions across states in Australia through the automatic mutual recognition (AMR) of licences. This means if someone is licensed to perform an activity in one state or territory, they can perform the same activity in other participating states where those activities are recognised and licensed. However, the potential benefits of AMR have not been realised as many occupations in New South Wales have been exempt from the scheme, which commenced in 2021. Exemptions have been granted due to the perceived risk to the health and safety of workers or the public.
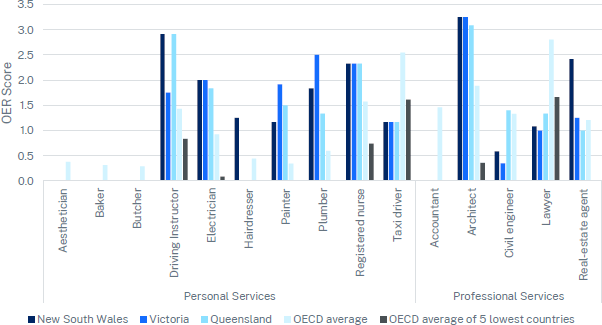
Note: The OECD average of 5 lowest countries refers to those countries that score the lowest within each respective occupation.
Source: NSW Treasury and the Reserve Bank of Australia, data: von Rueden and Bambalaite (2020).
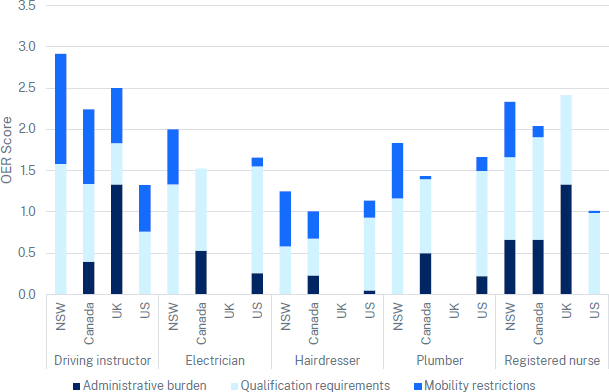
Source: NSW Treasury and the Reserve Bank of Australia, data: von Rueden and Bambalaite (2020).
4.2 New South Wales imposes stricter OER for several construction-related occupations compared to Queensland and Victoria
The building and construction industry is highly regulated and employs a significant number of workers in New South Wales and Australia. In recent years, licensing arrangements in the building and construction sector have become more stringent across most Australian states, typically driven by higher entry requirements (Commonwealth Productivity Commission 2023). The industry is also facing significant shortages of skilled workers that is impacting the delivery of infrastructure and housing projects. The National Skills Commission found that all construction trades occupations are in shortage across Australia (National Skills Commission 2021). While occupational regulation may aim to minimise the risks of consumer detriment, particularly building defects, it may also restrict the adaptability of the workforce.
We measure the stringency of OER across 14 select occupations in the building and construction sector in New South Wales, Queensland, and Victoria ( Figure 5). OER are notably more stringent in New South Wales compared to other states for air conditioning and refrigeration (AC&R) mechanics. Likewise, New South Wales is considerably more stringent for project builders compared to Queensland.
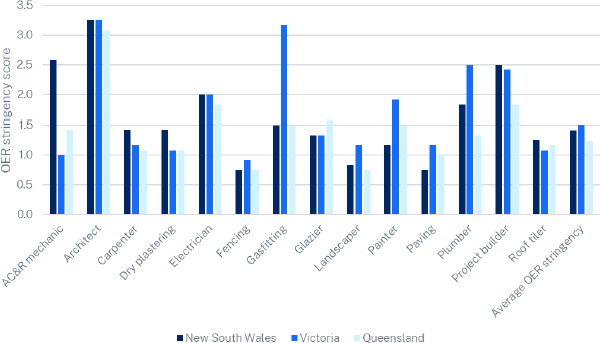
Sources: NSW Treasury and the Reserve Bank of Australia.
4.3 OER are prevalent across the most common occupations in New South Wales
OER are commonplace across the NSW economy. Of the 100 most common occupations in New South Wales, 43 of them had some form of OER, with formal licences or mandatory education requirements being the most common form (Figure 6).[4] OER tended to be higher in the health sector, and the building and construction sector. Both are somewhat unsurprising, given the potential for consumer harm stemming from poor provision.
Qualification requirements were the greatest contributor to the stringency of OER for most of the top 100 occupations subject to occupational regulation. Further work could investigate whether the qualification requirements for occupations is effective and proportional in improving the quality of services provided. For example, the NSW Productivity Commission (2023) found that increasing the qualification requirements for aspiring teachings from a one-year Graduate Diploma of Education to a two-year Master of Teaching degree was not effective. The change had a negligible impact on student achievement but imposed considerable costs on aspiring teachers.
Mobility restrictions are also consistently an important contributor, adding to the OER indicator for four out of five regulated occupations. Of these 34 occupations, half of them had limited ability to recognise skills gained abroad, and more than half required local exams to be taken for qualifications gained outside of New South Wales. This highlights the large number of potential occupations that could benefit from the automatic mutual recognition of licences.
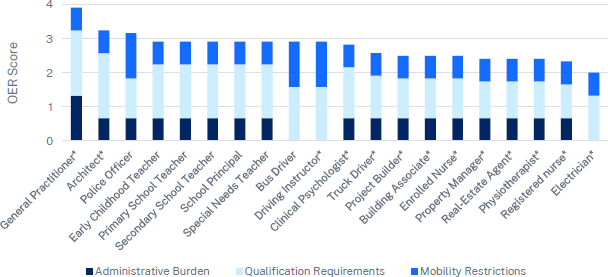
Note:
Scores for bus drivers and driving instructors appear unusually high due to requirements for drivers to obtain a local driving licence, in addition to further qualification and registration requirements. Scores would appear lower if it was assumed that an aspiring individual already holds a local driving licence.
*Indicates occupation is regulated by a licensing scheme.
Source: NSW Treasury and the Reserve Bank of Australia.
5 Examining the effects of OER
As discussed above, occupational licences are intended to protect consumers, ensuring that goods and services meet a minimum quality by requiring providers to have a certain level of training. At the same time, occupational licences also impose economic costs. This stems from the greater difficulty for workers to switch and enter occupations with more stringent OER, which must be weighed against their potential benefits.
We provide the first empirical evidence for Australia on some of the potential costs of OER. We focus on two different aspects. First, whether more stringent OER reduce economic dynamism – that is the rate at which businesses enter and exit, or the rate at which the most productive businesses expand, which are important contributors to growth in productivity. Second, whether more stringent OER could be contributing to skills shortages that make it harder for those unemployed to secure employment.
5.1 Stringent OER make businesses less dynamic
We focus on economic dynamism for three reasons. First, there is a strong basis for a relationship. OER reflect a cost for workers to enter an occupation, making entry of new businesses more costly. Such barriers should also weaken the relationship between firm growth and productivity (Decker et al. 2020). Second, Bambalaite et al. (2020) find evidence of a relationship between OER and measures of dynamism. And third, measures of economic dynamism have been declining in recent years, with negative implications for productivity and living standards (e.g. Andrews and Hansell 2021). As such, understanding OER's relationship with these measures is of particular interest, as it may help us to better understand the slowdown in productivity observed over recent decades.
For this analysis we follow Bambalaite et al. (2020) and focus on a subset of the OECD occupations that can be easily mapped to commonly used industry classifications (ANZSIC – Australian and New Zealand Standard Industrial Classification). This mapping is shown below in Table 6.[5] Our sample includes observations for New South Wales, Victoria, and Queensland.
Occupation | ANZSIC industry code |
---|---|
Baker | 1172, 1174 |
Butcher | 4121 |
Electrician | 3232 |
Hairdresser | 9511 |
Painter | 3244 |
Plumber | 3231 |
Taxi driver | 4623 |
Accountant | 6932 |
Architect | 6921 |
Civil engineer | 3101, 3109, 6923 |
Lawyer | 6931 |
Real estate agent | 6711, 6712, 6720 |
Source: NSW Treasury and the Reserve Bank of Australia, data: ABS (2006). |
5.1.1 OER reduce business entries and exits
Occupational restrictions represent a barrier to entry for firms, creating costs for business owners in terms of their own training and potentially limiting the pool of qualified labour they can draw from. As such, we may expect entry rates to be lower where OER are more stringent. Exit rates may also be expected to be lower where OER are more stringent, given incumbents are protected from competition from entrants.
Figure 7 provides some initial evidence, plotting average entry rates over the period 2003 to 2019 for each industry-by-state pairing, alongside their OER.[6] In doing so we abstract from the average rates for each industry (remove industry means). This allows us to focus on differences across states with differing OER, rather than across industries where other factors may also influence entry and exit rates. We see that entry rates are lower the more stringent an occupation's OER.
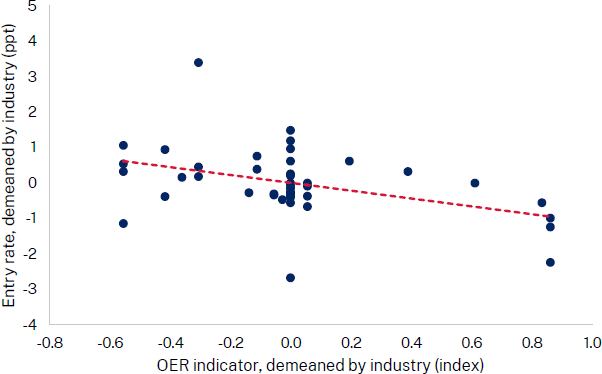
Note: Each dot represents state-industry pairing. Entry rates are average from 2003 to 2019. Both entry rates and OER have mean level for industry (across states) removed. As such, chart focuses on differences in OER and entry rates across states, once accounting for average industry outcomes. Set of industry outlined in Table 6.
Source: NSW Treasury and the Reserve Bank of Australia.
To test this more formally we adopt a simple regression framework. The equation takes the form:
where Ratei,s is our measure of economic dynamism (i.e., entry and exit rates) in industry i, state s on average over 2003 to 2019, OER is the relevant OER measure, and captures industry fixed effects.[7] The coefficient of interest is , which captures whether entry and exit rates are higher or lower when OER are more stringent.
Table 7 shows the results. Our preferred model just focuses on how entry and exit rates and OER within each industry differ across states. So it just captures whether, for example, entry rates for hairdressers tend to be lower in New South Wales, which has more stringent OER for hairdressers, than Victoria and Queensland. But it will ignore the fact that OER scores and entry rates might tend to be low for hairdressers across all states on average. We find that a one-unit increase in the OER is associated with a 1.1 percentage point decrease in the entry rate and a 0.5 percentage point decrease in the exit rate. This is consistent whether we focus on all firms, or just employing ones.
All firms | Employing firms | |
---|---|---|
Entry rate | −1.11*** | −1.13*** |
(Standard error) | (0.38) | (0.40) |
Industry controls | ||
Observations | 42 | 42 |
R-squared | 0.798 | 0.862 |
Exit rate | −0.50* | −0.54* |
(Standard error) | (0.25) | (0.29) |
Industry controls | ||
Observations | 42 | 42 |
R-squared | 0.898 | 0.903 |
Note: (a) Standard errors in parentheses, ***P < 0.01; **P < 0.05; *P < 0.1. Standard errors are robust to heteroskedasticity. Coefficients represent percentage point increase in entry/exit rates for one unit rise in OER index. Source: NSW Treasury and the Reserve Bank of Australia. |
To put the numbers in context, the OER index for hairdressers in New South Wales is 1.25 units higher than Victoria and Queensland. This is estimated to have reduced the entry rate of hairdressers in New South Wales by around 1.4 percentage points and exit rates by 0.6 percentage point. This is equivalent to 168 fewer entrants and 76 fewer exits in 2023.
To estimate the potential costs of OER, we assess how much higher entry and exit rates would be if each industry had their OER lowered to the lowest rate observed in Bambalaite et al. (2020). This assessment, based on the estimated coefficients above, provides a plausible benchmark on how low OER can be while still providing consumer and worker protections. An alternative benchmark could consider the increase in entry and exit rates if OER were reduced to zero; however, this was not pursued as the benchmark is likely to be unrealistic in practice.
Figure 8 shows the outcomes. On average across the industries covered by our analysis, annual entry rates would be around one percentage point higher if OER rates were lowered to OECD lows. To put this in context, entry rates for employing businesses fell by around five percentage points from the mid-2000s to mid-2010s. So lowering OER could offset around one-fifth of the decline in economic dynamism observed over the past two decades, at least in those industries considered.
Similarly, exit rates would be around 0.5 percentage point higher. This is obviously a very simple, back-of-the-envelope partial equilibrium approach to quantifying the effect and should be interpreted in that context. But the results do suggest that reducing OER could have a notable effect on the entry and exit of businesses, at least in those industries considered.
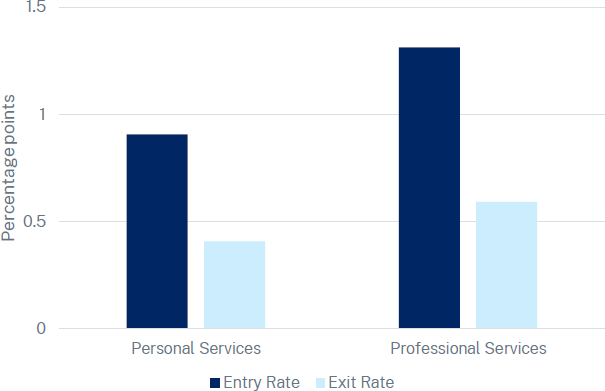
Note: OECD lowest is average of five lowest scores across sample in OECD paper. Unweighted average across states and occupations.
Source: NSW Treasury and the Reserve Bank of Australia, von Rueden and Bambalaite (2020).
We can do a similar back-of-the-envelope exercise for individual industries, using the coefficient from the aggregate model and applying them to each sector (which may be a strong assumption). Aligning OER in New South Wales to those of the least stringent OECD countries would have the largest effects on entry and exit in the architecture and real estate industries (Figure 9). The entry rates for these occupations would be expected to increase 3.2 percentage points and 2.7 percentage points respectively. Across personal services, measures to relax OER would see greater business entry and exit among electricians, hairdressers, painters, and plumbers. This is noteworthy as New South Wales faces labour shortages in each of these occupations (National Skills Commission 2022). New South Wales has relatively more lenient occupational entry requirements for taxi drivers and lawyers, so there are no benefits to aligning these regulations to countries in the OECD.
This exercise is not arguing that OER should be loosened in these industries, as any assessment would need to weigh up these costs against the potential benefits, which is beyond the scope of this paper. It is simply trying to provide estimates and frameworks that can be helpful in any such assessments, and more generally to understand the effects of OER on the macroeconomy.
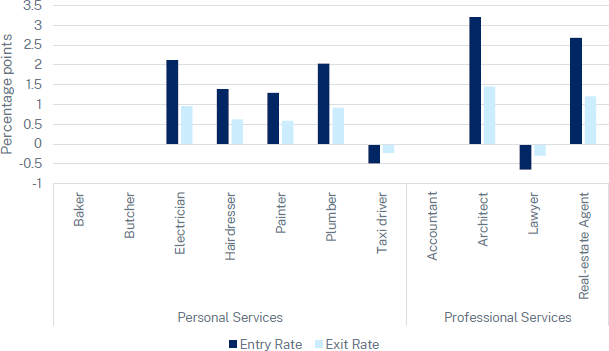
Note: OECD lowest is average of five lowest scores across sample in OECD paper. Unweighted average across states and occupations.
Source: NSW Treasury and the Reserve Bank of Australia.
5.1.2 OER make it harder for the most productive businesses to expand
OER could also affect the allocation of resources, creating frictions that hinder the rate at which highly productivity firms tend to grow. This is an important channel of productivity growth that can reduce the living standards of citizens. To examine the effects of OER and the flow of resources to more productive firms, we follow Bambalaite et al. (2020) and adopt the regression framework put forward by Decker et al. (2020).
This framework is motivated by standard models of firm dynamics which predict that, conditional on initial size, more productive firms should grow more quickly than less productive firms. But frictions that make it more costly for labour to flow between firms, or that weaken competition and therefore weaken the impetus for high-productivity firms to grow and make this gap in growth between high- and low-productivity firms smaller. This can lead to lower aggregate productivity growth as, all else equal, high-productivity firms will tend to be smaller and low-productivity firms larger.
The regression takes the form:
where is the employment growth of firm f in industry i, in state s, at time t, and Productivityf,s,c,t–1 is their labour productivity in the previous period. Xf,c,s,t contains a number of firm-level controls, including their size, and sales growth. are state and industry time fixed effects to capture the effects of the economic cycle on employment outcomes. As we include these industry controls, we are accounting for average growth and productivity in the industry, and so effectively are only comparing outcomes across high- and low-productivity firms within each industry, rather than capturing reallocation between more and less productive industries.
The coefficient of interest is , which captures whether the relationship between firm growth and productivity is stronger (>0) or weaker (<0) where OER are higher. In order to focus on the effects of the OER itself, we include an interaction between productivity and state unemployment. This allows us to abstract from the fact that the relationship between growth and productivity could vary depending on the strength of the local economy.[8]
In our preferred specification, similar to our entry and exit regressions above, we also control for the average strength of the relationship in each industry by interacting a dummy variable for each industry with the productivity term. This helps us to abstract from the possibility that some industries may, for some unrelated reasons, tend to have high OER and low rates of reallocation (e.g. due to high job-specfic human capital). Instead, we focus on how the rate of reallocation differs within industries across states, based on the differing OER level across states.
Table 8 shows the results. Focusing on our preferred specification in column 1, we see that more productive firms grow more quickly, but that this relationship is weaker where the OER is higher. To put numbers to this, take the case of a high- and low-productivity firm in an industry, where we define high and low productivity as one standard deviation above and below the industry mean (equating to around one log point either side of the mean). If we focused on an industry with an OER score of zero, the more productive firm would be expected to grow 10 percentage points faster – every year – than the low productivity firm. However, were the OER score to increase by one unit, this gap in growth falls to around nine percentage points.[9]
Industry-by-productivity control | Industry-by-productivity control, since 2012 | |
---|---|---|
(1) | (2) | |
Productivity | 4.71*** | 3.76*** |
(Standard error) | (1.12) | (1.03) |
Productivity*OER | −0.37** | −0.51** |
(Standard error) | (0.15) | (0.22) |
Controls | ||
Productivity*lndustry | Y | Y |
Observations | 708,591 | 406,903 |
R-squared | 0.061 | 0.058 |
Note: (a) Standard errors in parentheses, ***P < 0.01; **P < 0.05; *P < 0.1. Standard errors clustered at the industry*state level. All regressions control for lagged firm sales growth and size, industry*state*year fixed effects, and productivity*state unemployment. Coefficients represent percentage point increase in employment growth for a one unit increase in log productivity. Source: NSW Treasury and the Reserve Bank of Australia. |
As with the entry and exit analysis, we can again ask: if OER were lowered to align with the lowest levels in the OECD, how much more quickly would labour flow from low- to high-productivity firms? Based on the regressions above, the predicted gap in growth between a high- and low-productivity firm given the observed OER was 7.5 percentage points. After lowering the OER that gap would increase to around 8.5 percentage points, a moderate increase for those industries analysed.
To put these in context, Andrews and Hansell (2021) find that, on average across the entire non-resource, non-financial private sector, the employment growth gap between high- and low-productivity businesses fell from around nine percentage points in the mid-2000s, to around seven percentage points in the mid-2010s. Moreover, they find that this had a substantial effect on productivity growth, lowering it by around 0.25 percentage point. So taking this admittedly very simple approach to quantificiation, it would suggest that we could offset around half of this decline in productivity and dynamism by lowering OER to OECD lows, at least for those industries considered here.
5.2 Stringent OER may be contributing to skill shortages
OER can dissuade workers from entering an occupation by creating a barrier to entry. This has the potential to lead to a mismatch between the demand for certain occupations and skills and their supply, both in the shorter term as demand for certain skills ebbs and flows over an economic cycle, and potentially over the longer term as the economy undergoes structural change. Such skills shortages and mismatches have the potential to raise the structural level of unemployment and impinge on economic growth more generally. The NSW Productivity Commission and NSW Innovation and Productivity Council (2022) also found that overly stringent OER can lower the quality of services by reducing competition in the labour market or perversely incentivising consumers to use unlicensed services.
The relationship between OER and skills shortages is examined using occupation-level metrics provided by the National Skills Commission's Skill Priority List (2022). This approach is less rigorous than the firm-level analysis above, as we cannot rule out the possibility that high OER and high rates of skill shortages are both driven by some third factor, such as the nature of the training required for certain occupations and how it is provided. For example, the need to learn a lot of content and skills. However, this approach still provides suggestive evidence of the effect of OER stringency on skill shortages.
For this analysis we focus first on those sectors that are regulated by OER (i.e. have an OER indicator above zero). We focus on this subset to abstract from the variation in outcomes for unregulated sectors, which will be entirely driven by other factors. Overall, OER tend to be more stringent for occupations facing skill shortages than those not facing shortages (Figure 10). This suggests that OER may exacerbate skill shortages and could be contributing to higher levels of structural unemployment.
Considering occupations with OER, they are substantially experiencing skill shortages, compared to those without OER. For example, among the top 100 most common NSW occupations, only 30 per cent of the unregulated occupations were found to be facing skill shortages, compared to 75 per cent of the regulated occupations. Taken together these results provide some tentative evidence that more stringent OER may contribute to skill shortages.
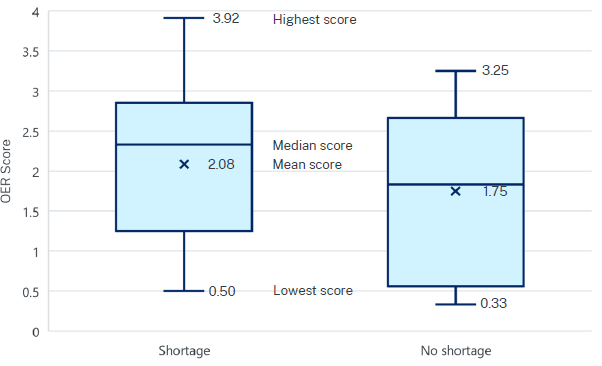
Note: The chart examines OER stringency for 40 of the top 100 most commonly regulated occupations in New South Wales (i.e. all occupations with an OER stringency score > 0). The chart segregates OER stringency scores by occupations experiencing skill shortages and no skill shortages based on data from the National Skills Commission.
Sources: NSW Treasury and the Reserve Bank of Australia.
6 Conclusion and further work
OER is an important regulatory tool that has important costs and benefits. This paper fills a gap in our understanding of OER by documenting their stringency across several states and occupations and assessing their costs in terms of economic dynamism.
We find evidence that OER in Australia are slightly more stringent than the average OECD country, particularly for personal services, but are significantly more stringent than the least stringent countries in the OECD. There are also differences across states, with OER tending to be more stringent in New South Wales, compared to Queensland and Victoria.
We also find evidence that OER do impose some macroeconomic costs. More stringent OER are associated with less business entry and exit, which potentially has implications for competition and therefore consumer prices. More stringent OER are also associated with slower flows of labour from low- to high-productivity firms, and therefore lower productivity growth. And finally, there is some tentative evidence that more stringent OER are associated with skill shortages.
These findings provide a useful input for regulators when assessing changes to OER. They also help us better understand the macroeconomic effects of past, or future, changes in OER. To this end, future work documenting changes in OER over time could be useful in understanding whether changes in OER contributed to the decline in economic dynamism and productivity growth observed in Australia over the 2010s.
7 References
Akcigit, U. and Ates, S. (2021) “Ten Facts on Declining Business Dynamism and Lessons from Endogenous Growth Theory”, American Economic Journal: Macroeconomics, no. 13 (1): 257-98. https://www.aeaweb.org/articles?id=10.1257/mac.20180449
Andrews, D., Hambur, J., Hansell, D., and Wheeler, A. (2022) “Reaching for the stars: Australian firms and the global productivity frontier”, No. 2022-01. Treasury Working Paper. https://treasury.gov.au/sites/default/files/2022-02/p2022-243535.pdf
Andrews, D. and Hansell, D. (2021) “Productivity‐Enhancing Labour Reallocation in Australia†”, Economic Record, The Economic Society of Australia, vol. 97 (317): 157-169, June.
Atalay, E., Phongthiengtham, P., Sotelo, S. and Tannenbaum, D. (2020) “The Evolution of Work in the United States”, American Economic Journal: Applied Economics, no. 12 (2), pp. 1-34. https://www.aeaweb.org/articles?id=10.1257/app.20190070
ABS. (2006) ‘Australian and New Zealand Standard Industrial Classification’, revision 2.0, rev 26 June 2013. https://www.abs.gov.au/statistics/classifications/australian-and-new-zealand-standard-industrial-classification-anzsic/2006-revision-2-0
Bambalaite, I., Nicoletti, G. and von Rueden, C. (2020) “Occupational entry regulations and their effects on productivity in services: Firm-level evidence”, OECD Economics Department Working Papers, no. 1605. https://doi.org/10.1787/c8b88d8b-en
Barker, A. (2022) ‘Skills Recognition 2022’, Submission to the Employment White Paper, Committee for Economic Development of Australia, Melbourne. https://cedakenticomedia.blob.core.windows.net/cedamediacontainer/kentico/media/attachments/c eda-skills-recognition.pdf
Blair, P. and Chung, B. (2018) “How much of barrier to entry is occupational licensing?”, NBER Working Paper, no. 25262, rev December 2018. https://www.nber.org/papers/w25262
Commonwealth Productivity Commission. (2008) ‘Review of Australia's Consumer Policy Framework’, Vol. 2, Inquiry Report no. 45, Canberra. https://www.pc.gov.au/inquiries/completed/consumer-policy/report/consumer2.pdf
Commonwealth Productivity Commission. (2015) ‘Mutual Recognition Schemes’, Research report, Canberra. https://www.pc.gov.au/inquiries/completed/mutual-recognition-schemes/report/mutual-recognition-schemes.pdf
Commonwealth Productivity Commission. (2023) ‘5-year Productivity Inquiry: A more productive labour market’, Vol. 7, Inquiry Report no. 100, Canberra. https://www.pc.gov.au/inquiries/completed/productivity/report/productivity-volume7-labour-market.pdf
De Loecker, J., Eeckhout, J. and Mongey, S. (2021) “Quantifying Market Power and Business Dynamism in the Macroeconomy”. NBER Working Paper, no. w28761. https://www.nber.org/papers/w28761
Decker, R., Haltiwanger, J., Jarmin, R., and Miranda. J. (2020) “Changing Business Dynamism and Productivity: Shocks versus Responsiveness”, American Economic Review, no. 110 (12): 3952-90. https://www.aeaweb.org/articles?id=10.1257/aer.20190680
Hambur, J. (2023) “Product Market Competition and its Implications for the Australian Economy†”. Economic Record, 99(324), pp. 32-57. https://doi.org/10.1111/1475-4932.12707
Housing Industry Association. (2023) “Shortage of skilled trades remains acute, despite return of skilled migration”, Media release, 28 April 2023.
Johnson, J. and Kleiner M. (2017) “Is Occupational Licensing a Barrier to Interstate Migration?”. NBER Working Paper, no. 24107. https://www.nber.org/papers/w24107
Kleiner, M. and Krueger, A. (2008) “The Prevalence and Effects of Occupational Licensing”. NBER Working Paper Series, no. 14308. https://www.nber.org/system/files/working_papers/w14308/w14308.pdf
Leland, H. (1979). “Quacks, Lemons, and Licensing: A Theory of Minimum Quality Standards”. Journal of Political Economy, no. 87(6), 1328–1346. http://www.jstor.org/stable/1833335
National Skills Commission. (2021) ‘Skills Priority List Findings: Construction Trades Workers: ANZSCO Sub-major Group 33’, Skills Priority List Occupation Report. https://www.jobsandskills.gov.au/sites/default/files/2022-01/33%20Construction%20Trades%20Workers.pdf
National Skills Commission. (2022) ‘National Skills Commission, Skills Priority List, 2022’. Available at: https://immi.homeaffairs.gov.au/employer-subsite/files/national-skills-commission-skills-priority-list-2022.pdf
NSW Productivity Commission and NSW Innovation and Productivity Council. (2022) ‘Adaptive NSW: how embracing tech could recharge our prosperity’, NSW Productivity Commission and NSW Innovation and Productivity Council, Sydney. 20221117-nsw-productivity-commission_adaptive-nsw_how-embracing-tech-could-recharge-our-prosperity.pdf
NSW Productivity Commission. (2023) ‘The economic impacts of longer postgraduate initial teacher education.’ NSW Productivity Commission. https://www.productivity.nsw.gov.au/sites/default/files/2023-03/20230125_1-the-economic-impacts-of-longer-postgraduate-initial-teacher-education.pdf
von Rueden, C. and Bambalaite, I. (2020) “Measuring occupational entry regulations: A new OECD approach”. OECD Economics Department Working Papers, no. 1606. https://doi.org/10.1787/296dae6b-en
Copyright and disclaimer notices
Blade disclaimer
The results of these studies are based, in part, on data supplied to the ABS under the Taxation Administration Act 1953, A New Tax System (Australian Business Number) Act 1999, Australian Border Force Act 2015, Social Security (Administration) Act 1999, A New Tax System (Family Assistance) (Administration) Act 1999, Paid Parental Leave Act 2010 and/or the Student Assistance Act 1973. Such data may only be used for the purpose of administering the Census and Statistics Act 1905 or performance of functions of the ABS as set out in section 6 of the Australian Bureau of Statistics Act 1975. No individual information collected under the Census and Statistics Act 1905 is provided back to custodians for administrative or regulatory purposes. Any discussion of data limitations or weaknesses is in the context of using the data for statistical purposes and is not related to the ability of the data to support the Australian Taxation Office, Australian Business Register, Department of Social Services and/or Department of Home Affairs' core operational requirements.
Legislative requirements to ensure privacy and secrecy of these data have been followed. For access to MADIP and/or BLADE data under Section 16A of the ABS Act 1975 or enabled by section 15 of the Census and Statistics (Information Release and Access) Determination 2018, source data are de-identified and so data about specific individuals has not been viewed in conducting this analysis. In accordance with the Census and Statistics Act 1905, results have been treated where necessary to ensure that they are not likely to enable identification of a particular person or organisation.
Appendix A: Top 100 most common occupations in New South Wales
- Sales Assistant (General)
- General Clerk
- Aged or Disabled Carer
- Accountant (General)
- Primary School Teacher
- Secondary School Teacher
- Storeperson
- Truck Driver (General)
- Commercial Cleaner
- Child Care Worker
- Electrician (General)
- Carpenter
- Accounts Clerk
- Kitchenhand
- Marketing Specialist
- Lawyer
- Chef
- Checkout Operator
- Management Consultant
- Motor Mechanic (General)
- Software Engineer
- Delivery Driver
- Bookkeeper
- Medical Receptionist
- Waiter
- Receptionist (General)
- Nursing Support Worker
- Teachers' Aide
- Shelf Filler
- Fitter (General)
- Personal Assistant
- Forklift Driver
- Plumber (General)
- Information Officer
- Bank Worker
- Project Builder
- Registered Nurse (Aged Care)
- Bar Attendant
- Police Officer
- ICT Customer Support Officer
- Developer Programmer
- General Practitioner
- Fast Food Cook
- Hairdresser
- Builder's Labourer
- Barista
- Property Manager
- University Lecturer
- Bus Driver
- Registered Nurse (Critical Care and Emergency)
- Credit or Loans Officer
- Building Associate
- Pharmacy Sales Assistant
- Recruitment Consultant
- Cafe Worker
- Security Officer
- Domestic Cleaner
- Cook
- Miner
- Insurance Consultant
- Welfare Worker
- Painting Trades Worker
- Graphic Designer
- Human Resource Adviser
- Metal Fabricator
- Handyperson
- Data Entry Operator
- Civil Engineer
- Call or Contact Centre Operator
- Enrolled Nurse
- Early Childhood (Pre-primary School) Teacher
- Vocational Education Teacher
- Fitness Instructor
- Real-estate Agent
- Retail Supervisor
- Payroll Clerk
- School Principal
- Physiotherapist
- Courier
- Personal Care Assistant
- Registered Nurse (nec)
- Beef Cattle Farmer
- ICT Business Analyst
- Sales Representative (Personal and Household Goods)
- Despatching and Receiving Clerk
- Community Worker
- Financial Investment Adviser
- Social Worker
- Gardener (General)
- Landscape Gardener
- Dental Assistant
- Concreter
- Special Needs Teacher
- Registered Nurse (Perioperative)
- Beauty Therapist
- Mixed Crop and Livestock Farmer
- Clinical Psychologist
- Airconditioning and Refrigeration Mechanic
- Registered Nurse (Medical)
- Architect
Appendix B: NSW Treasury Scoring Index
Question | Weight | Coding of data | |||||||
---|---|---|---|---|---|---|---|---|---|
I Administrative burdens (0.33 OECD / 0.25 NSW PC) | |||||||||
1. Limitation to number of authorisations | 0.33 | Yes 6 |
No 0 |
Missing | |||||
2. Restrictions on territorial validity of professional qualification | 0.33 | Yes 6 |
No 0 |
Missing | |||||
3. Compulsory membership or registration in professional association | 0.33 | Yes 6 |
N 0 |
Missing | |||||
II. Qualification requirements (0.33 OECD / 0.25 NSW PC) | |||||||||
4. Number of pathways to obtain qualification | 0.25 | One pathway 6 |
Two pathways 3 |
Three or more pathways 0 |
Missing | ||||
5. Requirement of university degree or vocational course | 0.25 | No 0 |
Yes, up to 1 year 1 |
Yes, more than 1 year to 2 years 2 |
Yes, more than 2 years to 3 years 3 |
Yes, more than 3 years to 4 years 4 |
Yes, more than 5 years to 5 years 5 |
Yes, more than 5 years 6 |
Missing |
6. Requirement of compulsory practice | 0.25 | Yes 6 |
No 0 |
Missing | |||||
7. Mandatory state exam | 0.25 | Yes 6 |
No 0 |
Missing | |||||
III. Mobility restrictions (0.33 OECD / 0.25 NSW PC) | |||||||||
8. Laws or regulations establish a process for recognising qualifications g | 0.33 | Yes 0 |
No 6 |
Missing | |||||
9. Local exam required to practice | 0.33 | Yes 6 |
No 0 |
Missing | |||||
10. Nationality or citizenship required to practice | 0.33 | Yes 6 |
No 0 |
Missing | |||||
IV. Licensing costs (0.25 NSW PC) | |||||||||
11. Licence application & registration fee to avgerage yearly income ratio | 0.33 | 0% 0 |
0.01% to 0.19% 1 |
0.2% to 0.39% 2 |
0.4% to 0.59% 3 |
0.6% to 0.79% 4 |
0.8% to 0.99% 5 |
1% and over 6 |
|
12 Licence renewal period | 0.33 | Yes, up to 1 year 5 |
Yes, more than 1 year to 2 years 4 |
Yes, more than 2 years to 3 years 3 |
Yes, more than 3 years to 4 years 2 |
Yes, more than 4 years to 5 years 1 |
No renewal 0 |
Missing | |
13. Mandatory insurance | 0.33 | Yes 6 |
No 0 |
Missing |
Acknowledgements
The views expressed in this paper are those of the authors and not necessarily those of NSW Treasury or the Reserve Bank of Australia. Any errors are our own.
We are grateful to Dan Andrews, Andrew Barker, Tom Carr and Anthony Rush for helpful comments and suggestions. We acknowledge important contributions from John Dundas for editorial and publication assistance.
Footnotes
Countries included in the analysis include Austria, Belgium, Canada, Finland, France, Germany, Hungary, Iceland, India, Israel, Italy, Poland, Portugal, Slovenia, South Africa, Spain, Sweden, Switzerland, United Kingdom, United States. [1]
Where registrations or licences are not automatically recognised between jurisdictions, these are considered to be territorial restrictions. [2]
These tend to include countries such as Sweden, Finland, Switzerland, and in some cases Great Britain, the United States, and Spain. [3]
The list of occupations was based on the 2021 Census and excludes executive and managerial positions (see appendix A). Note the executive and managerial positions were excluded where their roles were too vague to identify specific occupational regulations, for example, Chief Executive Officer. [4]
Relative to Bambalaite et al. (2020) we exclude driving instructors, as they are included in ANZSIC group 8219 ‘Community and Other Education’, which is very broad. [5]
For this analysis we exclude civil engineers as our sample is dominated by the mining boom, which will have substantively increased demand for and entry of such businesses in Queensland. [6]
Note that the evidence is weaker if we focus on the shorter sample since 2021. However, given volatility in entry and exit rates during the sample, our preferred approach is to focus over a longer period where these may average out. [7]
Unlike the entry regressions, we include civil engineering in these regressions as the inclusion of state by time, and state unemployment*productivity controls allow us to better account for the effect of local cycles. That said, results are very similar if civil engineering is excluded. [8]
More precisely, given the inclusion of the industry*productivity and unemployment*productivity variables, the numbers referred to are for a base industry, when unemployment is zero. Changing these parameters would scale up or down the level but would not change the effect of moving the OER. [9]