Research Discussion Paper – RDP 2024-01 Do Monetary Policy and Economic Conditions Impact Innovation? Evidence from Australian Administrative Data
February 2024
1. Introduction
Productivity growth is the key determinant of living standards over the medium term. Many factors can influence how quicky productivity grows, including technological change, competition, skilled labour, regulation, trade and tax policy (Aghion and Howitt 2008). But economists have traditionally assumed that productivity is unaffected by ‘cyclical’ factors such as current economic conditions and monetary policy, at least over the medium term.
More recently, though, there is a growing literature questioning this assumption. One stream of this literature argues that weaker economic conditions, particularly economic downturns, can lead to slower rates of innovation and technology adoption by businesses, which can in turn lead to persistent changes in productivity and economic output. These hysteresis effects are considered in Stadler (1990), Comin and Gertler (2006), Anzoategui et l (2019), Bianchi, Kung and Morales (2019) and Amador (2022) among others.
In a similar vein, there is evidence that contractionary monetary policy, which weakens economic conditions, can slow innovation and technology adoption, and therefore have persistent effects on productivity (Moran and Queralto 2018; Jordà, Singh and Taylor 2020; Ma 2023; Ma and Zimmerman 2023).[1] While the effects of expansionary and contractionary monetary policy are likely to cancel out over a cycle, such a finding highlights the potential for medium-run economic scarring to occur if policy is constrained by the effective lower bound on interest rates and therefore cannot offset economic downturns.
This has important implications for macroeconomic policy, but there is little evidence on these issues outside the United States. And the effects could differ substantially in a small open economy like Australia that typically imports innovation, compared to a large economy like the United States that pushes the technological frontier. Moreover, much of the evidence has focused on narrow measures of innovative activity like research and development (R&D) spending and patenting. These measures are likely to miss a large amount of innovative activity in the form of adoption of existing technologies and processes. Empirically, adoption is an important determinant of aggregate productivity growth (OECD 2015; Argente et al 2020; Majeed et al 2021) and features in theoretical models of the effect of monetary policy on productivity (Moran and Queralto 2018).
We explore the effects of monetary policy shocks on several different measures of innovation in Australia, including broad measures that will capture adoption of innovation developed elsewhere. Further, we explore whether the effects differ by firm size and other firm characteristics, using firm-level information on innovation and adoption, which has received relatively little focus to date. As well as providing interesting insights into the heterogeneous effects of monetary policy, this helps us to better understand the mechanisms through which monetary policy, and economic conditions, could affect innovation. The channels we explore include: weaker demand, which lowers incentives to innovate; and tighter credit conditions, which make it harder to finance investment and innovation. We find that both are important. We also consider the effect of US monetary policy shocks, which could be important for a small open economy like Australia, both because of standard spillovers from US policy to domestic conditions (e.g. Georgiadis 2016; Kearns, Schrimpf and Xia 2023), and because Australia imports innovation from the United States and US policy affects US innovative activity.
We find that contractionary (expansionary) monetary policy shocks reduce (increase) aggregate R&D spending and that changes in R&D spending have medium-run effects on productivity. However, we do not find any effect of monetary policy shocks on the number of patents filed, consistent with Australia tending to be an importer of new-to-world innovations rather than a producer (Majeed and Breunig 2023).
Turning to the firm-level data, we find that overall monetary policy shocks appear to have relatively little effect on broader survey measures of innovation and adoption. But this result hides offsetting impacts of monetary policy by firm size. Following a contractionary policy shock the share of small and medium enterprises (SMEs) innovating declines, while the share of large firms innovating increases. These heterogeneous responses appear consistent with SMEs and large firms having differing exposures to the channels though which monetary policy affects innovation. Monetary policy tends to weigh on innovative activity by tightening credit constraints (the credit constraint channel), particularly for smaller firms. Monetary policy also weighs on innovation by lowering domestic demand (the demand channel), and exporting firms, who tend to be larger, appear less exposed to this channel. This latter result is flipped for US shocks, which, if anything, weigh more heavily on the innovative activity of exporters, who are more exposed to international conditions.
Overall, our results suggest that monetary policy and economic activity can have medium-run effects on innovation and therefore productivity, though they paint a more complex and heterogeneous picture compared to previous work. These effects are likely to cancel out over a cycle. However, the results reinforce the importance of using macro stabilisation policy to avoid sharp economic downturns, given the potential for medium-run economic scarring, and highlight the costs of having such policy constrained (Barlevy 2004; Benigno and Fornano 2018; Ikeda and Kurozumi 2019; Garga and Singh 2021). The potential for monetary policy to affect innovation and productivity in the medium run may also slightly alter the trade-offs between stabilisation of output and inflation when faced with a supply shock, particularly if inflation expectations can be kept anchored (Queralto 2022; Fornaro and Wolf 2023). That said, the results do not suggest that central banks should focus mainly on output growth at the expense of inflation stabilisation given the risks of expectations de-anchoring, which could require a much larger response and correspondingly sharper economic downturn.
This paper proceeds as follows. We first discuss the relevant literature on the determinants of innovation in Section 2 before giving an overview of our data and methodology in Section 3. We then look at the effects of monetary policy shocks on aggregate on innovative activity – patents, trademarks and R&D – and firm-level innovation in Section 4, before exploring the channels through which monetary policy may affect innovation in Section 5. We then consider the aggregate effect of changes in R&D spending on productivity in Section 6 before concluding.
2. Literature Review
Until recently, the prevailing wisdom was that current macroeconomic conditions and monetary policy have no effect on productivity over the medium term. For monetary policy, this assumption is reflected in the so called ‘neutrality’ of monetary policy. However, a growing number of papers and policymakers have begun to question this assumption, contributing to the broader literature on hysteresis. Much of this literature focuses on medium-run employment effects, stemming from labour market scarring for workers (e.g. Blanchard and Summers 1986; Ball 2009; Andrews et al 2020) or changes in the nature or number of startups (Ouyang 2009; Sedláček and Sterk 2017; Davis and Haltiwanger forthcoming).
More recently the scarring literature has begun to look at the medium-run implications for productivity, building on the existing evidence that economic and financial conditions can influence the amount of innovative activity undertaken (e.g. Brown, Fazzari and Petersen 2009; Jensen and Webster 2011; Ouyang 2011; Huber 2018). Early papers in this productivity hysteresis literature focused on economic downturns, and whether they could contribute to lower productivity in the medium run by weighing on the amount of innovation and technology adoption done during the downturn (Stadler 1990; Comin and Gertler 2006; Barlevy 2007; Anzoategui et al 2019; Bianchi et al 2019).[2] Focusing mostly on R&D spending as their measure of innovation, these papers tend to find evidence that innovation declines during downturns and that this decline can have medium-run effects on productivity and output. Different papers propose different mechanisms for the decline in innovation, including reduced incentives due to lower demand (Comin and Gertler 2006; Barlevy 2007; Anzoategui et al 2019) or tighter credit market conditions (Bianchi et al 2019; Queralto 2020). Some papers have also argued that innovation will increase during downturns, as weaker economic activity can free up resources and make innovation cheaper (Aghion et al 2012).
Recent papers have begun to explore whether monetary policy can influence innovation and technology adoption and, through this, have medium-run effects on productivity and economic output. Jordà et al (2020), using a cross-country dataset, provide empirical evidence that contractionary monetary shocks lead to lower productivity growth over the medium term. They build a New Keynesian model with endogenous total factor productivity (TFP) growth to motivate their empirical findings.
Moran and Queralto (2018) provide more evidence on the mechanisms for the United States. Using a vector autoregression (VAR) model, they find that contractionary monetary policy lowers innovative activity, as measured by R&D spending. In turn, lower R&D spending tends to lead to slower productivity growth and therefore lower economic output. They build these mechanisms into a New Keynesian model where weaker economic conditions, including those due to contractionary monetary policy, lower the returns to innovation and adoption, and therefore lead to slower innovation as well as slower adoption of innovations. Monetary policy can thus influence productivity and economic output in the medium term. Their model indicates that the medium-run effects of policy and conditions on productivity and output can be sizeable, and therefore of first order importance to policymakers. They estimate that US output and productivity would have been permanently 2 per cent higher had monetary policy not been constrained by the effective zero lower bound following the global financial crisis. Similarly, US productivity and output would have been permanently 1 per cent higher if the tightening of monetary policy from 2016 had been more gradual (though this may have had implications for inflation and inflation expectations).
Ma (2023) focuses on patenting as a measure of innovative activity, again for the United States. He finds that the value and number of patents rise following an expansionary monetary policy shock, which in turn can contribute to higher productivity. He finds that firms with higher liquidity are more responsive than firms with lower liquidity, suggesting that financing constraints play an important role. In a heterogeneous firm model, he shows how this financing constraint mechanism can contribute to longer-lived impacts of monetary policy shocks on productivity and output.
Ma and Zimmerman (2023) focus on similar measures to the two previously mentioned papers, such as R&D spending and patenting, as well as venture capital funding, and find similar results to the above papers for the United States. Similar to our paper, they explore the channels through which monetary policy can affect innovative activity. They find that R&D and patenting are more responsive to monetary policy shocks in cyclical industries, suggesting monetary policy affects innovation by influencing demand conditions – the demand channel. They also find evidence that venture capital funding falls following a contractionary monetary policy shock, which in turn is likely to limit the amount of funds available for innovative activity – the financial channel. Applying standard multipliers from innovative activity to output, they suggest that a 100 basis point contractionary shock could lead to output that is 1 per cent lower five years after the shock.
Amador (2022) focuses on cross-country measures of the take-up of general-purpose technologies, like electrification. He finds that contractionary policy leads to slower diffusion of these technologies, which can weigh on output in the medium term.
Finally, our paper also touches on the large literature exploring global spillovers from US monetary policy. While this literature tends to focus on the effects of US policy on interest rates, investment or output in other countries (e.g. Georgiadis 2016; Arbatli-Saxegaard et al 2022; Kearns et al 2023), we extend this to consider firms' innovative activity.
3. Data and Methodology
3.1 Data
We focus on four different measures of innovative activity.
The first three are narrow measures of innovative activity that have been considered in the earlier literature.
- The (log) flow of new patents filed in Australia by Australian residents from IP Australia's IPLORD database.[3] This is a fairly narrow measure of innovative activity that is more likely to capture the creation of ‘new-to-world’ innovation and which has previously been linked to economic growth (Atun, Harvey and Wild 2007).
- The (log) flow of new trademarks filed in Australia by Australian residents from IP Australia's IPLORD database. This is a slightly broader measure of innovation that will likely capture ‘new-to-firm’ innovation (Mendonça, Pereira and Godinho 2004; Malmberg 2005).
- Aggregate (log) R&D spending data from the Australian Bureau of Statistics (ABS) national accounts. R&D has been linked to both higher novelty of innovation and adoption of innovation (D'Este, Amara and Olmos-Peñuela 2016; Majeed and Breunig 2023). As such, this is a slightly broader measure of innovative activity that will capture spending on the creation of new-to-world innovation, as well as potentially some spending to adopt innovations.
All three variables are observed quarterly from March 1994 through December 2019.
Our fourth measure of innovative activity is a survey measure of innovation collected in the ABS Business Characteristics Survey (BCS). This is a broad measure of innovation based on the Oslo Manual (OECD and Eurostat 2018), the OECD benchmark for innovation measurement. It captures around 8,000 firms each year from 2005/06 to 2019/20. Each year firms are asked whether they introduced new or significantly improved: goods or services; operational processes; organisational/managerial processes; or marketing methods. This measure includes adoption of existing technologies or processes, which is an important mechanism in models such as that of Moran and Queralto (2018). It is also particularly important in Australia, which tends to be an importer of innovation: around 5 in 100 firms in the survey report introducing a new-to-world innovation, whereas 50 in 100 firms report some form of innovative activity.
The BCS is a census of firms with more than 300 employees and a stratified random sample for firms with less than 300 employees. Stratification by industry and business size is implemented to produce data that are representative of Australian businesses. The ABS do not provide the sample weights so we use unweighted data. As such, when reporting results across all firms, rather than by size, large firms will be somewhat overweighted.
Firms with fewer than 300 employees are included on a rotating five-year basis. This could create some biases in our results, particularly if small and large firms respond differently to shocks, as the share of large firms in our sample will be larger as we consider longer time horizons. Taking any given year as a base period, only two-fifths of small firms will still be in the sample in four years' time. Splitting the sample into small and large firms helps to limit any potential bias.[4]
Another potential bias could come from attrition. If monetary policy shocks cause firms to exit, and if the remaining firms are more likely to be innovative, it may appear that the share of firms innovating has increased, but this result would just reflect a positive survivorship bias. To avoid this issue, our main regressions focus on firms with at least five observations. These are firms who do not exit during their period in the sample. Our firm-level results thus measure the intensive margin effect of monetary policy on firm innovation only. That said, the results are very similar if we do not impose this restriction. We also directly test whether monetary policy affects the probability of firm exit, and whether the effect differs by innovator status. We do not detect any difference between innovators and non-innovators in terms of exit post-shock.
We exclude microbusinesses from our study by removing all firms with one full-time equivalent (FTE) employee or less. Excluding micro firms is standard practice in the literature.
The BCS data are available at the firm level and are linked to the ABS Business Longitudinal Analysis Data Environment (BLADE), which contains demographic and tax data from administrative sources. This allows us to model innovation at the firm level, accounting for firm-level covariates and potential heterogeneous effects across firm types. See Tables A1 and A2 for descriptive statistics for the firm-level sample.
3.2 Methodology
We do not explicitly develop our own theoretical model for the analysis but the channels of monetary policy that we consider are those of the models developed in other papers such as Moran and Queralto (2018) and Ma (2023). Our econometric methodology is motivated by testing the implications of such models.
3.2.1 Monetary policy shocks
An inherent difficulty in examining the effect of monetary policy on innovation is that the official cash rate will be endogenous. That is, innovation activity and monetary policy are co-determined by other factors. For example, the central bank is likely to raise rates if it expects economic activity and inflation to increase. But improvements in economic activity might also spur further innovative activity. As such, it might appear that higher interest rates lead to more innovation when both are moving with economic conditions.
To get around this endogeneity issue we use a monetary policy shock measure developed in Beckers (2020).[5] This is a Romer and Romer (2004)-style shock, in that it measures shocks as divergences of the observed policy rate from what would be expected based on an estimated policy reaction function. This approach is widely used in the literature (Ramey 2016).
Specifically, Beckers (2020) estimates an augmented Taylor rule that includes a forecast for economic conditions and a number of indicators of financial conditions (e.g. bond spreads, option-implied volatility). The shocks are then constructed as the deviation of the actual policy rates from that implied by the rule. As such, this approach removes the anticipatory component of monetary policy by purging the changes in the policy rate of the central bank's systematic response to its own forecasts. We use the continuous measure that also orthogonalises the shock with respect to market expectations for the policy rate, though our results are near identical using the measure without this step.
We adopt this measure as our preferred shock measure because previous research found that it is able to overcome the so-called price puzzle in Australian data: that contractionary monetary policy is often estimated to raise prices. This is not the case for simpler Romer and Romer-style shocks (Bishop and Tulip 2017) or measures based on high-frequency changes in bond yields over a 90-minute window around announcements (Hambur and Haque 2023). We nonetheless consider these other measures for robustness in Tables B1–B4.
3.2.2 Estimation
We employ a local projection regression (Jordà 2005) to trace out the effect of a monetary policy shock at time t on our measures of innovative activity over a number of different time horizons h. This is a common approach in the literature (Ma 2023; Jordà et al 2020; Durante, Ferrando and Vermeulen 2022). Our regression takes the following form:
where h ≥ 0, denotes the time horizon, t denotes time and i indexes the firm (for firm-level regressions). We also estimate Equation (1) using aggregate variables which we can represent identically to the above, simply dropping the i subscripts. Inni,t+h is our measure of innovation. This is either at the aggregate level for (log) R&D, (log) patents or (log) trademarks, or at the firm level for our 0/1 indicator of whether a firm innovates. shockt is our continuous measure of monetary policy shocks described above. Typically, local projections control for macroeconomic variables to improve efficiency (Jordà 2005). We control for standard aggregate measures: gross domestic product (GDP), the consumer price index (CPI) and the trade-weighted index (TWI) exchange rate. For our firm-level regressions we also control for: (log) employment, turnover growth, (log) capital expenditure and age (a dummy if a firm is more than four years old). We include these with one lag.[6]
For firm-level regressions we cluster the standard error at the period level, reflecting the fact that the key variable of interest varies across time but not across firms. As a result, we have a small number of clusters, which can bias our standard errors downwards. To address this, we assess significance based on a T ‐distribution with t – n degrees of freedom, where t is the sample length and n is the number of coefficients on variables that do not vary across firms, as discussed in Cameron and Miller (2015). We do not allow for serial correlation as this is captured in the control variables.
4. Results
4.1 Aggregate innovation measures
In this section, we examine how monetary policy shocks affect patents, trademarks and R&D activity. In particular, we focus on the coefficients of the shock variable in Equation (1) over different time horizons and trace them out in Figure 1. For consistency, all the results of this paper are based on a 100 basis point contractionary shock.
Unlike the United States (Ma 2023), monetary policy shocks have no effect on the number of patents filed (Figure 1, top panel). This is consistent with Australia tending to be an importer of new technologies, rather than a producer (Majeed and Breunig 2023) and having fewer patents filed compared to the United States. However, when focusing on somewhat broader measures of innovation there is evidence that monetary policy can have a significant influence.
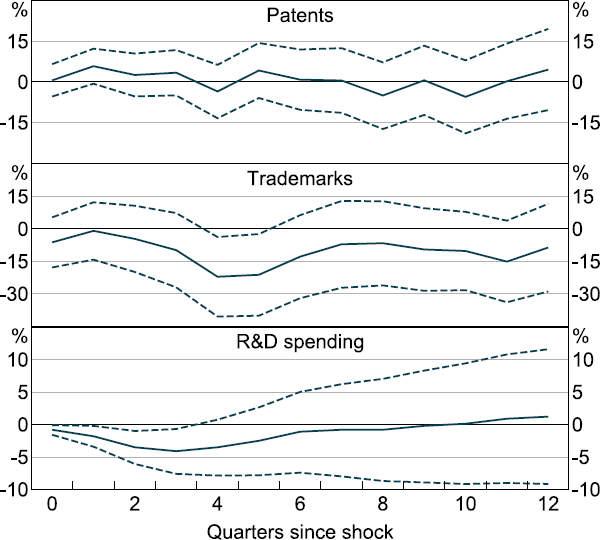
Notes: Results from local projection model of aggregate innovation metrics on monetary policy shock. Trademark sample excludes 1994–1995 due to break in time series. Patents and trademarks include only those with Australian filers. Models have eight lags of growth in GDP, TWI and CPI, and four lags of shocks and dependent variable. Results robust to other specifications. Dashed lines show 90 per cent confidence intervals, with Huber–White standard errors.
Sources: ABS; Authors' calculations; IP Australia.
In the year following a 100 basis point contractionary shock, R&D spending declines by around 5 per cent (bottom panel), while the number of trademarks falls by around 15 per cent one to two years after the shock (middle panel; marginally significant). The response of R&D spending is somewhat larger than documented in Moran and Queralto (2018), though it is also shorter lived – they document an 0.8 per cent decline, with the peak effect around four years after the shock. In part, this appears to reflect our use of a local projection model instead of a VAR. When we estimate a simple five-variable VAR where the shock is ordered first, as suggested by Plagborg-Møller and Wolf (2021), the peak effect is more sustained (see Figure B1). However, the peak effect is still larger and occurs earlier compared to Moran and Queralto (2018).[7] Our results are more in line with those from Ma and Zimmerman (2023), who also use local projections and find that aggregate investment in intellectual property falls by around 1 per cent one to two years after the shock, and that listed company R&D spending falls by around 3 per cent, with a peak effect two to three years after the shock. More generally, the 5 per cent response, while large, is not unreasonable in the context of this series – over the sample the year-ended growth rate of R&D spending has ranged between around –8 per cent and 22 per cent, with a standard deviation of around 7.5 per cent.[8]
4.2 Firm-level innovation and adoption measures
As discussed above, much of the literature to date has focused on relatively narrow measures of innovation that are unlikely to fully capture the broader effects of monetary policy on adoption of existing technologies by firms. This is even though technology adoption is a crucial mechanism in models such as that of Moran and Queralto (2018).
Focusing on our broader survey measure of innovation we find that a contractionary 100 basis point monetary policy shock has relatively little effect on the overall share of firms innovating (Table 1). But this average result hides very different outcomes for small and large firms. The share of SMEs (less than 200 employees) innovating falls by 6 percentage points the year after a 100 basis point contractionary monetary policy shock (Table 1). This equates to around 52,000 fewer firms innovating (based on the number of firms with 1–200 employees in 2019/20).
Year 0 | Year 1 | Year 2 | Year 3 | |
---|---|---|---|---|
All firms | ||||
Effect | −0.76 (1.65) |
−2.76* (1.47) |
1.29 (2.09) |
7.06** (2.67) |
R2 | 0.20 | 0.14 | 0.11 | 0.09 |
No of observations | 45,053 | 35,635 | 26,302 | 17,536 |
SMEs | ||||
Effect | −2.85 (1.61) |
−6.13*** (0.99) |
−2.45 (1.83) |
1.39 (1.54) |
R2 | 0.19 | 0.14 | 0.12 | 0.10 |
No of observations | 29,551 | 22,185 | 14,616 | 7,291 |
Large firms | ||||
Effect | 3.47 (2.64) |
2.70 (2.65) |
5.02* (2.35) |
9.80* (4.46) |
R2 | 0.19 | 0.11 | 0.07 | 0.06 |
No of observations | 15,502 | 13,450 | 11,686 | 10,245 |
Difference by size significant at: | 5 per cent level | 1 per cent level | 1 per cent level | 10 per cent level |
Notes: Significance assessed using T ‐ distribution with t – n degrees of freedom as suggested by Cameron and Miller (2015) to account for small number of clusters, where t is sample length and n is number of coefficients. All regressions include controls for industry, (lag) GDP growth, (lag) inflation, (lag) growth in the exchange rate, (lag) turnover growth and (lag) employment, and lag of the shock and dependent variable. ***, ** and * denote statistical significance at the 1, 5 and 10 per cent levels, respectively. Standard errors are shown in parentheses and are clustered at an annual level. |
In contrast, the share of larger firms (more than 200 employees) innovating increases by 5 percentage points two years after the shock, equating to around 200 firms (Table 1). This latter finding may seem surprising in the context of the existing literature. But it is consistent with some of the broader innovation literature that argues that innovation may be countercyclical, as firms may have the incentive to innovate when input costs are relatively cheap and when demand is weak (Aghion et al 2012). Larger firms may be better placed to take advantage of countercyclical conditions.
To put these numbers into context, from 2005/06 to 2019/20 the share of firms innovating rose consistently, increasing by around 7 percentage points by the end of the sample (DISER 2021). Given this, monetary policy appears to have meaningful effects on the share of firms innovating.[9] Moreover, the heterogeneity of results suggests that firms may be differentially exposed to different monetary policy channels. We explore this in Section 5.
4.3 Robustness
In this section, we test to see if our results differ substantially when we use other monetary policy shock measures. We consider four measures:
- The change in the policy rate (cash rate) itself.
- A version of the Beckers (2020) shock that does not purge market expectations, which we call ‘Beckers’.
- A Romer and Romer-style shock where the policy reaction function includes only economic variables and excludes the financial market variables used in Beckers (2020). This is taken from Bishop and Tulip (2017). We refer to this as ‘BT’.
- A measure based on high-frequency changes in bond yields based on a 90-minute window around announcements (Hambur and Haque 2023), which we call ‘levels shock’.
The results are provided in Tables B1 to B4. Using the Beckers variable gives qualitatively and quantitatively similar results to those we discuss above, consistent with the high correlation between the two shock measures. The results with the BT variable and the change in the policy rate are also qualitatively similar, with SMEs decreasing their innovation and large firms increasing, though the effects are not statistically significant. Interestingly, the evidence for the levels shock is qualitatively different, with some evidence of a decline in innovation for large firms. However, given the shortcomings with this measure discussed in Hambur and Haque (2023), the results from the Romer and Romer-style shocks remain our preferred estimates.
5. Through Which Channels Does Monetary Policy Affect Innovation?
As discussed in the literature review, there are several channels through which monetary policy and economic conditions could lower the amount of innovative activity in the economy. One is the demand channel: contractionary monetary policy may weaken aggregate demand and therefore lower the returns to innovation. Another is the credit constraint channel: monetary policy may lead to tighter credit conditions and make cash flow constraints more binding for some firms. In turn this may lessen their ability to fund and undertake innovative activity. To consider the importance of these channels we compare outcomes for firms that we would expect to be more exposed to the channels to outcomes for firms that we would expect to be less exposed.
5.1 Demand channel
Changing aggregate demand in the economy is an important channel for monetary policy. As a contractionary monetary policy shock lowers aggregate demand in the economy, it also lowers the potential future profits of a firm. As the likelihood of future profits is the key reason for firms to innovate (Aghion and Howitt 2008), the probability of firms innovating can decrease as aggregate demand falls. The same can be said for expansionary policy, with the directions flipped.
To consider the importance of the demand channel, we examine outcomes for exporting and non-exporting firms. Exporting firms are likely to be less exposed to domestic conditions, as their sales are not determined exclusively by the domestic economy. To do this we interact our shock variable with a dummy variable that takes the value of one if a firm ever exported while in the sample and zero otherwise. We use this to trace out the effect of a shock separately for exporters and non-exporters.
Consistent with the demand channel playing an important role, the negative effect of contractionary monetary policy on innovation is less evident for exporters (Table 2). These results are similar for SMEs and large firms (Table B5), though there is only a significant difference between exporters and non-exporters for the large firms. This suggests that the results do not simply reflect the fact that exporters tend to be larger, and that larger firms tend to increase innovation in response to a contractionary shock.
Year 0 | Year 1 | Year 2 | Year 3 | |
---|---|---|---|---|
Exporters | ||||
Effect | 0.24 (2.12) |
1.60 (1.96) |
4.29 (2.49) |
8.83* (4.01) |
R2 | 0.19 | 0.12 | 0.09 | 0.06 |
No of observations | 17,974 | 14,734 | 11,672 | 8,904 |
Non-exporters | ||||
Effect | −1.19 (1.78) |
−5.58*** (1.43) |
−1.14 (1.92) |
4.59** (1.93) |
R2 | 0.19 | 0.12 | 0.10 | 0.10 |
No of observations | 27,079 | 20,901 | 14,630 | 8,632 |
Difference by exporter status significant at: | na | 1 per cent level | 1 per cent level | na |
Notes: Significance assessed using T ‐ distribution with t – n degrees of freedom as suggested by Cameron and Miller (2015) to account for small number of clusters, where t is sample length and n is number of coefficients. All regressions include controls for industry, (lag) GDP growth, (lag) inflation, (lag) growth in the exchange rate, (lag) turnover growth and (lag) employment, and lag of the shock and dependent variable. ***, ** and * denote statistical significance at the 1, 5 and 10 per cent levels, respectively. Standard errors are shown in parentheses and are clustered at an annual level. |
It is important to note that there could be other differences between exporting and non-exporting firms that affect these results. For example, exporting firms tend to be more productive and may have better management. To try to isolate the demand effects, we next re-estimate the regression, but use a measure of US monetary policy shocks taken from Choi, Willems and Yoo (2023).[10] If the previous results reflected the demand channel, rather than other inherent differences between exporters and non-exporters, we might expect exporters to respond more strongly to overseas monetary policy shocks as they are more directly exposed to foreign demand than are non-exporting firms. This is the ‘trade channel’ of policy spillovers (Arbatli-Saxegaard et al 2022). This exercise is also valuable as it provides (to our knowledge) the first evidence on spillovers from US monetary policy onto innovation in another country.
The first thing to note is that contractionary US monetary policy shocks do appear to lower the share of firms innovating in Australia (Table 3). The effects appear larger, compared to the domestic shock. However, it is difficult to compare the magnitudes given the different implied persistence of the shocks.
Year 0 | Year 1 | Year 2 | Year 3 | |
---|---|---|---|---|
All firms | ||||
Effect | −5.73 (5.40) |
−11.02*** (3.20) |
−8.16 (5.17) |
−7.59 (8.88) |
R2 | 0.20 | 0.14 | 0.11 | 0.09 |
No of observations | 45,053 | 35,635 | 26,302 | 17,536 |
Exporters | ||||
Effect | −7.38 (6.97) |
−10.86* (5.37) |
−9.76 (6.29) |
−11.28 (10.17) |
R2 | 0.19 | 0.12 | 0.09 | 0.06 |
No of observations | 17,974 | 14,734 | 11,672 | 8,904 |
Non-exporters | ||||
Effect | −4.53 (5.04) |
−10.61** (3.79) |
−6.71 (4.95) |
4.59 (5.89) |
R2 | 0.19 | 0.12 | 0.10 | 0.09 |
No of observations | 27,079 | 20,901 | 14,630 | 8,632 |
Difference by exporter status significant at: | na | 1 per cent level | 1 per cent level | na |
Notes: Significance assessed using T ‐ distribution with t – n degrees of freedom as suggested by Cameron and Miller (2015) to account for small number of clusters, where t is sample length and n is number of coefficients. All regressions include controls for industry, (lag) GDP growth, (lag) inflation, (lag) growth in the exchange rate, (lag) turnover growth and (lag) employment, and lag of the shock and dependent variable. ***, ** and * denote statistical significance at the 1, 5 and 10 per cent levels, respectively. Standard errors are shown in parentheses and are clustered at an annual level. |
The second key finding is that the response for exporters appears if anything larger than for non-exporters, which is the opposite of the findings when we consider a shock to Australian monetary policy. This is observed for both SMEs and large firms (Table B6) and is consistent with other work focusing on the response of firm-level investment and sales in foreign countries following US policy shocks (Arbatli-Saxegaard et al 2022). While the differences are not statistically significant, the greater sensitivity of exporters to foreign shocks, and of non-exporters to domestic shocks, provides further evidence of the importance of the demand channel.
5.2 The credit constraint channel
Broadly defined, we can think of the credit constraint channel of monetary policy as capturing any way in which contractionary monetary policy (or weaker economic conditions) makes it harder for a firm to finance innovative activity. This can reflect: tighter aggregate credit supply; lower asset prices which reduce borrowers' collateral value and therefore borrowing capacity, the ‘financial accelerator channel’ (Bernanke, Gertler and Gilchrist 1999); or lower liquidity due to lower revenue or higher interest payments making existing financing constraints more binding (Jeenas 2023).
To examine the credit constraint channel directly, we explore an additional question in the BCS. This question asks whether a lack of access to additional funds is hampering the business's ability to innovate. We examine whether monetary policy shocks lead to a change in the share of firms reporting that lack of access to additional funds is limiting their innovative activity.[11]
Consistent with the credit constraint channel being important, contractionary monetary policy shocks lead to an increase in the likelihood that firms report that lack of funds is significantly hampering their ability to undertake innovation (Table 4). This is almost entirely driven by SMEs, which is consistent with the evidence that SMEs have a larger decline in innovation following a monetary policy shock. To put the results in context, over the sample around 17 per cent of SMEs note that a lack of funds is hampering their innovation (compared to 8 per cent for large firms). So a 100 basis point shock (which is very large historically) would cause the share of SMEs noting that a lack of funds are hampering innovation to increase by around 15 to 20 per cent. These findings are also consistent with the broader literature that finds that SMEs are more likely to be credit or cash constrained than larger firms – for example, Mancusi and Vezzulli (2010) and Bakhtiari et al (2020).
This provides fairly strong evidence that the credit constraint channel is important. As a further test, but one which relies less heavily on self-reported data, we examine whether the results differ for foreign- and domestically owned firms. Foreign-owned firms may be better able to access credit from overseas markets or from their overseas parent (Dahlquist and Robertsson 2001). Thus, they may be more sensitive to the global, not the domestic, cost of capital. As such, they may be less affected by domestic credit conditions.[12]
To look at whether foreign ownership mitigates the effect of monetary policy on innovation, we interact the monetary policy shock variable with a dummy variable for foreign ownership and then trace out the effects for domestically- and foreign-owned firms. If a firm indicates it has any level of foreign ownership (for the period that it is visible in our data), it is categorised as foreign owned. For this analysis we focus on large firms only, given the number of foreign-owned SMEs is small.
There is some evidence that contractionary monetary policy has less of a negative effect on foreign-owned firm innovation, compared to domestically owned firms (Table 5). While this evidence is less direct and could reflect factors other than the ability to access overseas financing, it is consistent with the credit supply channel playing an important role in the transmission of monetary policy to innovative activity and reinforces the above, more direct results.
Year 0 | Year 1 | Year 2 | Year 3 | |
---|---|---|---|---|
All firms | ||||
Effect | 1.40** (0.63) |
2.06*** (0.63) |
1.44 (0.99) |
2.37*** (0.54) |
R2 | 0.28 | 0.32 | 0.35 | 0.39 |
No of observations | 42,138 | 32,901 | 23,826 | 15,245 |
SMEs | ||||
Effect | 2.02* (1.00) |
2.92*** (0.70) |
2.45* (1.30) |
3.67*** (0.65) |
R2 | 0.28 | 0.32 | 0.34 | 0.37 |
No of observations | 28,216 | 20,966 | 13,518 | 6,630 |
Large firms | ||||
Effect | 0.16 (0.49) |
0.63 (1.41) |
0.25 (1.19) |
1.10 (0.83) |
R2 | 0.28 | 0.34 | 0.39 | 0.42 |
No of observations | 13,922 | 11,935 | 10,308 | 8,885 |
Difference by size significant at: | na | na | 10 per cent level | 10 per cent level |
Notes: Significance assessed using T ‐ distribution with t – n degrees of freedom as suggested by Cameron and Miller (2015) to account for small number of clusters, where t is sample length and n is number of coefficients. All regressions include controls for industry, (lag) GDP growth, (lag) inflation, (lag) growth in the exchange rate, (lag) turnover growth and (lag) employment, and lag of the shock and dependent variable. ***, ** and * denote statistical significance at the 1, 5 and 10 per cent levels, respectively. Standard errors are shown in parentheses and are clustered at an annual level. |
Year 0 | Year 1 | Year 2 | Year 3 | |
---|---|---|---|---|
Foreign owned | ||||
Effect | 6.78** (2.96) |
6.89** (3.09) |
7.58** (3.34) |
9.52* (4.80) |
R2 | 0.20 | 0.11 | 0.08 | 0.07 |
No of observations | 9,556 | 8,402 | 7,382 | 6,528 |
Domestically owned | ||||
Effect | −1.73 (2.22) |
−4.10 (3.18) |
0.81 (3.02) |
9.99 (5.79) |
R2 | 0.19 | 0.10 | 0.07 | 0.05 |
No of observations | 5,943 | 5,048 | 4,304 | 3,717 |
Difference by size significant at: | 1 per cent level | 10 per cent level | 1 per cent level | na |
Notes: Significance assessed using T ‐ distribution with t – n degrees of freedom as suggested by Cameron and Miller (2015) to account for small number of clusters, where t is sample length and n is number of coefficients. All regressions include controls for industry, (lag) GDP growth, (lag) inflation, (lag) growth in the exchange rate, (lag) turnover growth and (lag) employment, and lag of the shock and dependent variable. ***, ** and * denote statistical significance at the 1, 5 and 10 per cent levels, respectively. Standard errors are shown in parentheses and are clustered at an annual level. |
6. Macroeconomic Effects on Productivity
Thus far we have focused on measures of innovation rather than productivity. Moran and Queralto (2018) demonstrate in their model and empirically that shocks to R&D spending have an effect on productivity in the medium run. They show this focusing on the United States and using a cross-country panel (that includes Australia). However, given the vastly different structures of the Australian and US economies, it is worth examining whether the results for the United States hold when focusing only on Australia.
To examine if their results hold for Australia specifically, we reproduce the small VAR model used in Moran and Queralto (2018). We estimate a small, three variable VAR with annual data on GDP, TFP from Bergaud, Cette and Lecat (2016), and R&D spending from 1988 to 2019. As in Moran and Queralto (2018) we examine the effect of R&D shocks, which are identified by ordering R&D last in a Cholesky decomposition. This implies that R&D cannot affect TFP contemporaneously, consistent with the fact that it generally takes time for R&D expenditure to result in new technologies. While the assumption used to identify may still be strong, given we are using the same identification as Moran and Queralto (2018), it will give a direct Australian comparison to their US results.
Taking this approach, we find that a positive R&D shock leads to a persistent increase in TFP that peaks around five years after the shock (Figure 2). The magnitudes of the responses are somewhat larger than Moran and Queralto (2018) report, with an equivalent size increase in R&D (4 per cent) leading to a 1.6 per cent increase in TFP, compared to 0.4 per cent in Moran and Queralto. In the data, the volatility of the R&D shock is an order of magnitude smaller (around ½ per cent, compared to 4 per cent in Moran and Queralto (2018)), suggesting that such a large shock would be extremely unusual.[13] Again, our results are somewhat more in line with Ma and Zimmerman (2023). Drawing on other estimates, they suggest that a 100 basis point shock would lower TFP and output by 0.5 to 1 per cent, whereas our estimates put this around 1.6 per cent.
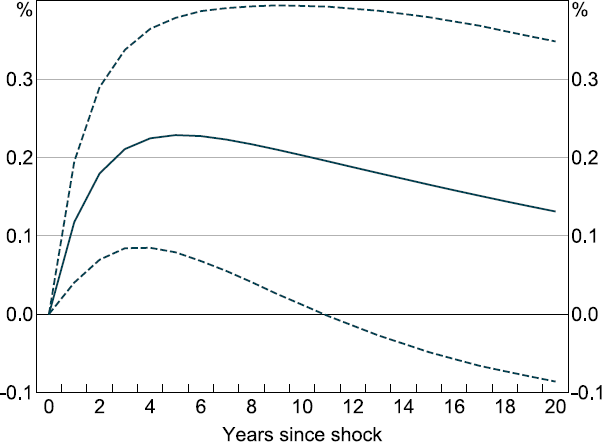
Notes: Response of TFP to an R&D spending shock from a VAR containing the log levels of real GDP, real R&D spending, and TFP. Based on a Cholesky decomposition with R&D spending ordered last. VAR(1) model. Dashed lines show 90 per cent confidence interval.
Sources: ABS; Authors' calculations; Bergeaud, Cette and Lecat (2016), ‘Long Term Productivity Database’ v2.6, available at <http://www.longtermproductivity.com/download.html>.
Interestingly, the response is also less long-lived compared to Moran and Queralto (2018): the peak occurs around five years after the shock for Australia, compared with around eight years in the United States. This could potentially reflect the fact that Australia imports innovation, and so while adoption of technologies declines the actual stock of global knowledge is unaffected, allowing firms to catch up more quickly (though still with a substantial lag).
Overall, while the exact magnitudes differ somewhat to Moran and Queralto (2018), these results suggest that changes in R&D and innovation can have long-run effects on productivity in Australia, as is evident in the United States.
7. Conclusion
There is a small but growing literature arguing that macroeconomic conditions and monetary policy shocks can have medium-run effects on productivity, and therefore living standards and economic activity. This has important implications for macroeconomic policy. While such effects are likely to cancel out over a business cycle, the potential for medium-run productivity scarring increases the importance of macro stabilisation policy. It also increases the costs associated with such policies being constrained, such as at the effective zero lower bound on interest rates. And it potentially alters the trade-offs a central bank faces when stabilising inflation and activity in the face of supply shocks, provided that inflation expectations can be kept anchored.
We provide the first evidence on the potential medium-run effects of monetary policy shocks and (indirectly) of economic conditions for a small open economy that imports innovation, rather than a large economy that creates it. Consistent with the overseas literature, we find evidence for Australia that contractionary (expansionary) monetary policy shocks are associated with a decline (increase) in R&D spending. We also find that declines (increases) in R&D spending are associated with lower (higher) levels of productivity in the medium term, though identifying the effects of R&D spending on productivity is more challenging.
When focusing on broader measures of innovation that may better capture adoption of technologies, a key driver of productivity growth, we find substantial heterogeneity in the response of firms. SMEs are less likely to innovate and adopt after a contractionary monetary policy shock, but large firms are more likely to do so. This appears to reflect the fact that SMEs and large firms have differing exposures to the various channels through which monetary policy can affect innovative activity. For example, exporting firms (which tend to be larger) are less likely to lower their innovative activity following a contractionary monetary policy shock, seemingly because they are less exposed to the ensuing softening in domestic demand. As such, monetary policy appears to affect innovation by affecting demand in the economy – so via the demand channel of monetary policy. Contractionary monetary policy shocks also lead to an increase in the share of firms (particularly SMEs) reporting that financial constraints are preventing them from innovating. As such, monetary policy also appears to affect innovation by influencing financial conditions and constraints – so via the financial channel of monetary policy.
A further novel aspect of our paper is considering the effects of US monetary policy shocks on the innovative activity of Australian firms. We find important spillovers from US monetary policy shocks onto the innovation activity of Australian firms. This effect is larger for Australian firms who export, again consistent with the demand channel of monetary policy.
Our results confirm that monetary policy, both domestic and foreign, and economic conditions can have medium-run effects on productivity and output by influencing the amount of innovative activity that occurs. However, they do not speak to the longer-run structural decline in productivity growth observed over the past two decades. Previous work has shown that this reflects structural declines in labour mobility, technology adoptions and competition which appear unrelated to the economic cycle (e.g. Andrews and Hansell 2021; Andrews et al 2022; Hambur 2023).
Appendix A: Data Description
Our survey measure of innovation is based on the definition of innovation:
An innovation is a new or improved product or process (or combination thereof) that differs significantly from the unit's previous products or processes and that has been made available to potential users (product) or brought into use by the unit (process). (OECD and Eurostat 2018, p 20)
This measure has been used in studies in Australia (DIIS 2016; Hendrickson et al 2018; Majeed and Breunig 2023) and overseas (OECD and Eurostat 2018), and has been shown to have a strong link with productivity.
Mean | Median | Share | |
---|---|---|---|
Employment (FTE) | 293 | 14 | |
Sales growth (%) | 6 | 3 | |
Age of firms (years) | 25 | 18 | |
Share of firms innovating (%) | 52 | ||
Share of firms > five years old (%) | 81 | ||
Share of firms where finance access hampers innovation (%) | 15 | ||
Share of SMEs (%) | 78 | ||
No of observations (ˋ000s) | 103 | ||
Sources: ABS; Authors' calculations. |
Mean | Median | Share | Mean | Median | Share | ||
---|---|---|---|---|---|---|---|
SMEs | Large firms | ||||||
Employment (FTE) | 22 | 8 | 1,246 | 569 | |||
Sales growth (%) | 6 | 3 | 8 | 4 | |||
Age of firms (years) | 21 | 15 | 44 | 31 | |||
Share of firms innovating (%) | 48 | 63 | |||||
Share of firms > five years old (%) | 78 | 90 | |||||
Share of firms where finance access hampers innovation (%) | 17 | 8 | |||||
No of observations (ˋ000s) | 80 | 20 | |||||
Exporters | Non-exporters | ||||||
Employment (FTE) | 624 | 79 | 143 | 9 | |||
Sales growth (%) | 5 | 3 | 7 | 3 | |||
Age of firms (years) | 34 | 24 | 22 | 15 | |||
Share of firms innovating (%) | 62 | 47 | |||||
Share of firms > five years old (%) | 89 | 79 | |||||
Share of firms where finance access hampers innovation (%) | 14 | 16 | |||||
No of observations (ˋ000s) | 32 | 70 | |||||
Foreign owned | Domestically owned | ||||||
Employment (FTE) | 906 | 355 | 136 | 10 | |||
Sales growth (%) | 6 | 4 | 6 | 3 | |||
Age of firms (years) | 35 | 23 | 23 | 16 | |||
Share of firms innovating (%) | 60 | 50 | |||||
Share of firms > five years old (%) | 85 | 81 | |||||
Share of firms where finance access hampers innovation (%) | 10 | 17 | |||||
No of observations (ˋ000s) | 21 | 81 | |||||
Sources: ABS; Authors' calculations. |
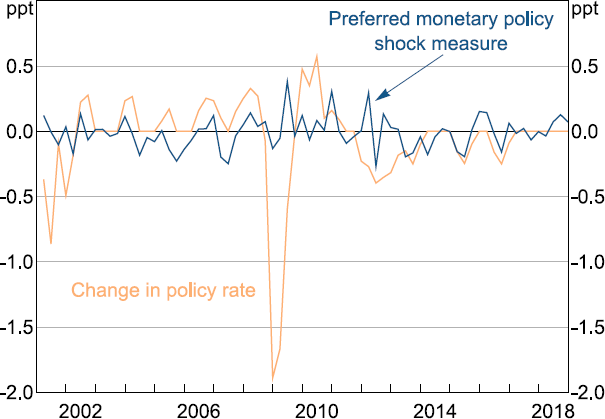
Sources: Authors' calculations; Beckers (2020); RBA.
Appendix B: Additional Regression Results
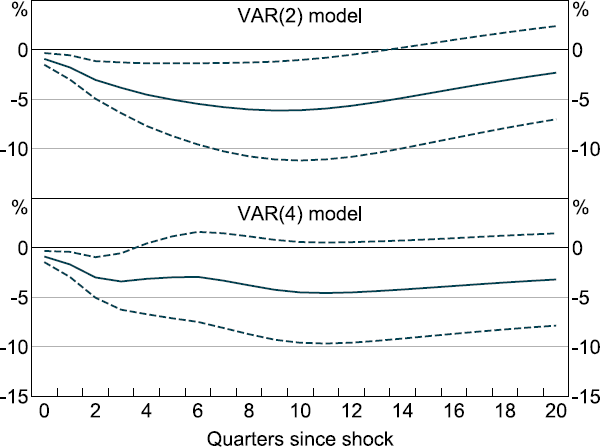
Notes: Response of R&D spending to monetary policy shock in a VAR containing the log levels of the CPI, real GDP, real R&D spending, the TWI, the level of the cash rate, and the Beckers (2020) shock. Based on a Cholesky decomposition with the shock measure ordered first. Dashed lines show 90 per cent confidence intervals.
Sources: ABS; Authors' calculations.
Year 0 | Year 1 | Year 2 | Year 3 | |
---|---|---|---|---|
All firms | ||||
Effect | −1.25* (0.65) |
0.03 (0.92) |
0.38 (0.64) |
1.25 (1.20) |
R2 | 0.20 | 0.14 | 0.11 | 0.09 |
No of observations | 45,053 | 35,635 | 26,302 | 17,536 |
SMEs | ||||
Effect | −1.28 (0.83) |
−0.29 (1.06) |
−0.25 (0.80) |
−0.64 (1.27) |
R2 | 0.19 | 0.13 | 0.12 | 0.10 |
No of observations | 29,551 | 22,185 | 14,616 | 7,291 |
Large firms | ||||
Effect | −1.03 (0.91) |
0.48 (1.19) |
1.05 (0.85) |
2.50 (1.73) |
R2 | 0.19 | 0.11 | 0.07 | 0.06 |
No of observations | 15,502 | 13,450 | 11,686 | 10,245 |
Notes: Significance assessed using T ‐ distribution with t – n degrees of freedom as suggested by Cameron and Miller (2015) to account for small number of clusters, where t is sample length and n is number of coefficients. All regressions include controls for industry, (lag) GDP growth, (lag) inflation, (lag) growth in the exchange rate, (lag) turnover growth and (lag) employment, and lag of the shock and dependent variable. ***, ** and * denote statistical significance at the 1, 5 and 10 per cent levels, respectively. Standard errors are shown in parentheses and are clustered at an annual level. |
Year 0 | Year 1 | Year 2 | Year 3 | |
---|---|---|---|---|
All firms | ||||
Effect | −4.38** (1.49) |
−3.52** (1.55) |
−0.03 (2.06) |
5.51** (2.26) |
R2 | 0.05 | 0.05 | 0.05 | 0.04 |
No of observations | 45,151 | 35,705 | 26,342 | 17,545 |
SMEs | ||||
Effect | −5.60*** (1.44) |
−6.44*** (1.13) |
−3.87* (1.84) |
−0.93 (1.67) |
R2 | 0.05 | 0.05 | 0.05 | 0.05 |
No of observations | 29,648 | 22,255 | 14,656 | 7,300 |
Large firms | ||||
Effect | −1.37 (3.01) |
1.01 (2.65) |
3.84 (2.64) |
10.48** (3.99) |
R2 | 0.03 | 0.02 | 0.03 | 0.03 |
No of observations | 15,503 | 13,450 | 11,686 | 10,245 |
Notes: Significance assessed using T ‐ distribution with t – n degrees of freedom as suggested by Cameron and Miller (2015) to account for small number of clusters, where t is sample length and n is number of coefficients. All regressions include controls for industry, (lag) GDP growth, (lag) inflation, (lag) growth in the exchange rate, (lag) turnover growth and (lag) employment, and lag of the shock and dependent variable. ***, ** and * denote statistical significance at the 1, 5 and 10 per cent levels, respectively. Standard errors are shown in parentheses and are clustered at an annual level. |
Year 0 | Year 1 | Year 2 | Year 3 | |
---|---|---|---|---|
All firms | ||||
Effect | −1.75 (1.09) |
−0.83 (1.40) |
0.19 (0.97) |
2.28 (1.69) |
R2 | 0.05 | 0.05 | 0.05 | 0.04 |
No of observations | 45,151 | 35,705 | 26,342 | 17,545 |
SMEs | ||||
Effect | −2.51 (1.50) |
−2.16 (1.45) |
−1.51 (1.22) |
−2.10 (1.43) |
R2 | 0.05 | 0.05 | 0.05 | 0.05 |
No of observations | 29,648 | 22,255 | 14,656 | 7,300 |
Large firms | ||||
Effect | −0.16 (1.18) |
0.47 (1.90) |
2.02* (1.09) |
4.94* (2.46) |
R2 | 0.03 | 0.02 | 0.03 | 0.03 |
No of observations | 15,503 | 13,450 | 11,686 | 10,245 |
Notes: Significance assessed using T ‐ distribution with t – n degrees of freedom as suggested by Cameron and Miller (2015) to account for small number of clusters, where t is sample length and n is number of coefficients. All regressions include controls for industry, (lag) GDP growth, (lag) inflation, (lag) growth in the exchange rate, (lag) turnover growth and (lag) employment, and lag of the shock and dependent variable. ***, ** and * denote statistical significance at the 1, 5 and 10 per cent levels, respectively. Standard errors are shown in parentheses and are clustered at an annual level. |
Year 0 | Year 1 | Year 2 | Year 3 | |
---|---|---|---|---|
All firms | ||||
Effect | 0.12 (0.26) |
−0.18 (0.42) |
−0.21 (0.25) |
−0.39 (0.44) |
R2 | 0.05 | 0.05 | 0.05 | 0.04 |
No of observations | 45,151 | 35,705 | 26,342 | 17,545 |
SMEs | ||||
Effect | 0.22 (0.38) |
0.05 (0.43) |
0.19 (0.35) |
0.81 (0.49) |
R2 | 0.05 | 0.05 | 0.05 | 0.05 |
No of observations | 29,648 | 22,255 | 14,656 | 7,300 |
Large firms | ||||
Effect | −0.11 (0.29) |
−0.37 (0.53) |
−0.59* (0.27) |
−1.02 (0.63) |
R2 | 0.03 | 0.02 | 0.03 | 0.03 |
No of observations | 15,503 | 13,450 | 11,686 | 10,245 |
Notes: Significance assessed using T ‐ distribution with t – n degrees of freedom as suggested by Cameron and Miller (2015) to account for small number of clusters, where t is sample length and n is number of coefficients. All regressions include controls for industry, (lag) GDP growth, (lag) inflation, (lag) growth in the exchange rate, (lag) turnover growth and (lag) employment, and lag of the shock and dependent variable. ***, ** and * denote statistical significance at the 1, 5 and 10 per cent levels, respectively. Standard errors are shown in parentheses and are clustered at an annual level. |
Year 0 | Year 1 | Year 2 | Year 3 | |
---|---|---|---|---|
SMEs | ||||
Exporters | ||||
Effect | −3.84 (1.98) |
−3.29 (1.94) |
−0.16 (3.92) |
4.07 (3.26) |
R2 | 0.19 | 0.14 | 0.13 | 0.10 |
No of observations | 7,733 | 5,788 | 3,847 | 2,009 |
Non-exporters | ||||
Effect | −2.24 (1.60) |
−6.90*** (1.21) |
−2.85 (1.88) |
0.99 (1.34) |
R2 | 0.19 | 0.13 | 0.10 | 0.09 |
No of observations | 21,818 | 16,397 | 10,769 | 5,282 |
Difference by exporter status significant at: | na | na | na | na |
Large firms | ||||
Exporters | ||||
Effect | 3.47 (2.76) |
4.76 (3.06) |
5.91* (2.62) |
9.73* (4.92) |
R2 | 0.20 | 0.11 | 0.08 | 0.06 |
No of observations | 10,241 | 8,946 | 7,825 | 6,895 |
Non-exporters | ||||
Effect | 3.65 (2.98) |
−1.49 (3.30) |
3.12 (2.63) |
9.79* (4.52) |
R2 | 0.18 | 0.09 | 0.07 | 0.06 |
No of observations | 5,261 | 4,504 | 3,861 | 3,350 |
Difference by exporter status significant at: | na | 10 per cent level | na | na |
Notes: Significance assessed using T ‐ distribution with t – n degrees of freedom as suggested by Cameron and Miller (2015) to account for small number of clusters, where t is sample length and n is number of coefficients. All regressions include controls for industry, (lag) GDP growth, (lag) inflation, (lag) growth in the exchange rate, (lag) turnover growth and (lag) employment, and lag of the shock and dependent variable. ***, ** and * denote statistical significance at the 1, 5 and 10 per cent levels, respectively. Standard errors are shown in parentheses and are clustered at an annual level. |
Year 0 | Year 1 | Year 2 | Year 3 | |
---|---|---|---|---|
SMEs | ||||
All firms | ||||
Effect | −7.00 (4.52) |
−10.33** (3.80) |
−9.85* (5.22) |
−21.19 (16.05) |
R2 | 0.19 | 0.14 | 0.12 | 0.10 |
No of observations | 29,551 | 22,185 | 14,616 | 7,291 |
Exporters | ||||
Effect | −11.44* (5.61) |
−11.76 (6.85) |
−18.81** (6.92) |
−16.62 (19.25) |
R2 | 0.19 | 0.14 | 0.13 | 0.10 |
No of observations | 7,733 | 5,788 | 3,847 | 2,009 |
Non-exporters | ||||
Effect | −5.48 (4.59) |
−9.59** (4.58) |
−6.93 (5.41) |
−25.36 (18.18) |
R2 | 0.19 | 0.12 | 0.10 | 0.09 |
No of observations | 21,818 | 16,397 | 10,769 | 5,282 |
Difference by size significant at: | na | na | na | na |
Large firms | ||||
All firms | ||||
Effect | −3.66 (9.19) |
−11.49* (6.05) |
−5.59 (5.71) |
−9.62 (8.97) |
R2 | 0.19 | 0.11 | 0.07 | 0.06 |
No of observations | 15,502 | 13,450 | 11,686 | 10,245 |
Exporters | ||||
Effect | −5.36 (9.22) |
−10.66 (6.44) |
−5.90 (7.54) |
−12.94 (10.87) |
R2 | 0.20 | 0.11 | 0.08 | 0.06 |
No of observations | 10,241 | 8,946 | 7,825 | 6,895 |
Non-exporters | ||||
Effect | −0.14 (10.20) |
−14.17 (7.93) |
−5.39 (4.51) |
−2.67 (7.54) |
R2 | 0.18 | 0.09 | 0.07 | 0.06 |
No of observations | 5,261 | 4,504 | 3,861 | 3,350 |
Difference by size significant at: | na | na | na | na |
Notes: Significance assessed using T ‐ distribution with t – n degrees of freedom as suggested by Cameron and Miller (2015) to account for small number of clusters, where t is sample length and n is number of coefficients. All regressions include controls for industry, (lag) GDP growth, (lag) inflation, (lag) growth in the exchange rate, (lag) turnover growth and (lag) employment, and lag of the shock and dependent variable. ***, ** and * denote statistical significance at the 1, 5 and 10 per cent levels, respectively. Standard errors are shown in parentheses and are clustered at an annual level. |
References
Aghion P, P Askenazy, N Bermna, G Cette and L Eymard (2012), ‘Credit Constraints and the Cyclicality of R&D Investment: Evidence from France’, Journal of the European Economic Association, 10(5), pp 1001–1024.
Aghion P and PW Howitt (2008), The Economics of Growth, The MIT Press, Cambridge.
Amador S (2022), ‘Hysteresis, Endogenous Growth, and Monetary Policy’, University of California, Davis, Department of Economics Working Paper No 348.
Andrews D, N Deutscher, J Hambur and D Hansell (2020), ‘The Career Effects of Labour Market Conditions at Entry’, Australian Treasury Working Paper No 2020-01.
Andrews D, J Hambur, D Hansell and A Wheeler (2022), ‘Reaching for the Stars: Australian Firms and the Global Productivity Frontier’, Australian Treasury Working Paper No 2022-01.
Andrews D and D Hansell (2021), ‘Productivity-enhancing Labour Reallocation in Australia’, Economic Record, 97(317), pp 157–169.
Anzoategui D, D Comin, M Gertler and J Martinez (2019), ‘Endogenous Technology Adoption and R&D as Sources of Business Cycle Persistence’, American Economic Journal: Macroeconomics, 11(3), pp 67–110.
Arbatli-Saxegaard E, M Firat, D Furceri and J Verrier (2022), ‘U.S. Monetary Policy Shock Spillovers: Evidence from Firm-level Data’, IMF Working Paper No WP/22/191.
Argente D, S Baslandze, D Hanley and S Moreira (2020), ‘Patents to Products: Product Innovation and Firm Dynamics’, Federal Reserve Bank of Atlanta Working Paper 2020-4.
Atun RA, I Harvey and J Wild (2007), ‘Innovation, Patents and Economic Growth’, International Journal of Innovation Management, 11(2), pp 279–297.
Bakhtiari S, R Breunig, L Magnani and J Zhang (2020), ‘Financial Constraints and Small and Medium Enterprises: A Review’, Economic Record, 96(315), pp 506–523.
Ball LM (2009), ‘Hysteresis in Unemployment: Old and New Evidence’, NBER Working Paper No 14818.
Baqaee DR, E Farhi and K Sangani (forthcoming), ‘The Supply-side Effects of Monetary Policy’, Journal of Political Economy.
Barlevy G (2004), ‘The Cost of Business Cycles under Endogenous Growth’, The American Economic Review, 94(4), pp 964–990.
Barlevy G (2007), ‘On the Cyclicality of Research and Development’, The American Economic Review, 97(4), pp 1131–1164.
Beckers B (2020), ‘Credit Spreads, Monetary Policy and the Price Puzzle’, RBA Research Discussion Paper No 2020-01.
Benigno G and L Fornaro (2018), ‘Stagnation Traps.’, The Review of Economic Studies, 85(3), pp 1425–1470.
Bergeaud A, G Cette and R Lecat (2016), ‘Productivity Trends in Advanced Countries between 1890 and 2012’, The Review of Income and Wealth, 62(3), pp 420–444.
Bernanke BS (2022), 21st Century Monetary Policy: The Federal Reserve from the Great Inflation to COVID-19, W.W. Norton & Company, New York.
Bernanke BS, M Gertler and S Gilchrist (1999), ‘The Financial Accelerator in a Quantitative Business Cycle Framework’, in JB Taylor and M Woodford (eds), Handbook of Macroeconomics: Volume 1C, Handbooks in Economics 15, Elsevier, Amsterdam, pp 1341–1393.
Bianchi F, H Kung and G Morales (2019), ‘Growth, Slowdowns, and Recoveries’, Journal of Monetary Economics, 101, pp 47–63.
Bishop J and P Tulip (2017), ‘Anticipatory Monetary Policy and the “Price Puzzle”’, RBA Research Discussion Paper No 2017-02.
Blanchard OJ and LH Summers (1986), ‘Hysteresis and the European Unemployment Problem’, in S Fischer (ed), NBER Macroeconomics Annual 1986, 1, The MIT Press, Cambridge, pp 15–78.
Brown JR, SM Fazzari and BC Petersen (2009), ‘Financing Innovation and Growth: Cash Flow, External Equity, and the 1990s R&D Boom’, The Journal of Finance, 64(1), pp 151–185.
Cameron AC and DL Miller (2015), ‘A Practitioner's Guide to Cluster-Robust Inference’, The Journal of Human Resources, 50(2), pp 317–372.
Choi S, T Willems and SY Yoo (2023), ‘Revisiting the Monetary Transmission Mechanism through an Industry-level Differential Approach’, Bank of England Staff Working Paper No 1,024, rev December 2023.
Comin D and M Gertler (2006), ‘Medium-term Business Cycles’, The American Economic Review, 96(3), pp 523–551.
Dahlquist M and G Robertsson (2001), ‘Direct Foreign Ownership, Institutional Investors, and Firm Characteristics’, Journal of Financial Economics, 59(3), pp 413–440.
Davis SJ and J Haltiwanger (forthcoming), ‘Dynamism Diminished: The Role of Housing Markets and Credit Conditions’, American Economic Journal: Macroeconomics.
D'Este P, N Amara and J Olmos-Peñuela (2016), ‘Fostering Novelty while Reducing Failure: Balancing the Twin Challenges of Product Innovation’, Technological Forecasting and Social Change, 113(Part B), pp 280–292.
DIIS (Department of Industry, Innovation and Science) (2016), Australian Innovation System Report 2016, Office of the Chief Economist, Department of Industry, Innovation and Science, Canberra.
DISER (Department of Industry, Science, Energy and Resources) (2021), ‘Australian Innovation System Monitor’, October.
Durante E, A Ferrando and P Vermeulen (2022), ‘Monetary Policy, Investment and Firm Heterogeneity’, European Economic Review, 148, Article 104251.
Fornaro L and M Wolf (2023), ‘The Scars of Supply Shocks: Implications for Monetary Policy’, Journal of Monetary Economics, 140 (Supplement), pp S18–S36.
Garga V and SR Singh (2021), ‘Output Hysteresis and Optimal Monetary Policy’, Journal of Monetary Economics, 117, pp 871–886.
Georgiadis G (2016), ‘Determinants of Global Spillovers from US Monetary Policy’, Journal of International Money and Finance, 67, pp 41–61.
Hambur J (2023), ‘Did Labour Market Concentration Lower Wages Growth Pre-COVID?’, RBA Research Discussion Paper No 2023-02.
Hambur J and Q Haque (2023), ‘Can We Use High-frequency Yield Data to Better Understand the Effects of Monetary Policy and Its Communication? Yes and No!’, RBA Research Discussion Paper No 2023-04.
Hendrickson L, D Taylor, L Ang, K Cao, T Nguyen and F Soriano (2018), ‘The Impact of Persistent Innovation on Business Growth’, Office of the Chief Economist, Department of Industry, Innovation and Science Research Paper 2/2018.
Herbst EP and BK Johannsen (2020), ‘Bias in Local Projections’, Board of Governors of the Federal Reserve System Finance and Economics Discussion Series No 2010-010, rev January 2021.
Huber K (2018), ‘Disentangling the Effects of a Banking Crisis: Evidence from German Firm and Counties’, The American Economic Review, 108(3), pp 868–898.
Ikeda D and T Kurozumi (2019), ‘Slow Post-financial Crisis Recovery and Monetary Policy’, American Economic Journal: Macroeconomics, 11(4), pp 82–112.
Jeenas P (2023), ‘Firm Balance Sheet Liquidity, Monetary Policy Shocks, and Investment Dynamics’, Barcelona School of Economics, BSE Working Paper 1409.
Jensen PH and E Webster (2011), ‘Macroeconomic Conditions and Determinants of Commercialisation’, Cambridge Journal of Economics, 35(1), pp 125–143.
Jordà Ò (2005), ‘Estimation and Inference of Impulse Responses by Local Projections’, The American Economic Review, 95(1), pp 161–182.
Jordà Ò, SR Singh and AM Taylor (2020), ‘The Long-run Effects of Monetary Policy’, Federal Reserve Bank of San Francisco Working Paper 2020-01, rev May 2023.
Kearns J, A Schrimpf and FD Xia (2023), ‘Explaining Monetary Spillovers: The Matrix Reloaded’, Journal of Money, Credit and Banking, 55(6), pp 1535–1568.
Ma C (2023), ‘Firm Liquidity and the Innovation Channel of Monetary Policy’, Job Market Paper, University of Texas, 8 November.
Ma Y and K Zimmermann (2023), ‘Monetary Policy and Innovation’, NBER Working Paper No 31698.
Majeed O, A Balaguer, D Hansell, L Hendrickson, A Latcham and T Satherley (2021), ‘What Drives High Growth? Characteristics of Australian Firms’, Economic Record, 97(318), pp 350-364.
Majeed O and R Breunig (2023), ‘Determinants of Innovation Novelty: Evidence from Australian Administrative Data’, Economics of Innovation and New Technology, 32(8), pp 1249-1273.
Malmberg C (2005), ‘Trademark Statistics as Innovation Indicators? – A Micro Study’, Lund University, CIRCLE Electronic Working Paper No 2005/17.
Mancusi ML and A Vezzulli (2010), ‘R&D, Innovation and Liquidity Constraints’, Knowledge, Internationalization and Technology Studies, Università Bocconi, KITeS Working Paper No 30/2010.
Mendonça S, TS Pereira and MM Godinho (2004), ‘Trademarks as an Indicator of Innovation and Industrial Change’, Research Policy, 33(9), pp 1385-1404.
Moran P and A Queralto (2018), ‘Innovation, Productivity, and Monetary Policy’, Journal of Monetary Economics, 93, pp 24-41.
OECD (Organisation for Economic Co-operation and Development) (2015), The Future of Productivity, OECD Publishing, Paris.
OECD and Eurostat (2018), Oslo Manual 2018: Guidelines for Collecting, Reporting and Using Data on Innovation, 4th edn, The Measurement of Scientific, Technological and Innovation Activities, OECD Publishing, Paris.
Ouyang M (2009), ‘The Scarring Effect of Recessions’, Journal of Monetary Economics, 56(2), pp 184-199.
Ouyang M (2011), ‘On the Cyclicality of R&D’, The Review of Economics and Statistics, 93(2), pp 542-553.
Plagborg-Møller M and CK Wolf (2021), ‘Local Projections and VARs Estimate the Same Impulse Responses’, Econometrica, 89(2), pp 955-980.
Queralto A (2020), ‘A Model of Slow Recoveries from Financial Crises’, Journal of Monetary Economics, 114, pp 1-25.
Queralto A (2022), ‘Monetary Policy in a Model of Growth’, Board of Governors of the Federal Reserve System International Finance Discussion Papers No 1340.
Ramey VA (2016), ‘Macroeconomic Shocks and Their Propagation’, in JB Taylor and H Uhlig (eds), Handbook of Macroeconomics: Volume 2A, Handbooks in Economics, Elsevier, Amsterdam, pp 71-162.
Romer CD and DH Romer (2004), ‘A New Measure of Monetary Shocks: Derivation and Implications’, The American Economic Review, 94(4), pp 1055-1084.
Sedláček P and M Ignaszak (2021), ‘Productivity, Profitability and Growth’, Centre for Economic Policy Research Discussion Paper DP16205.
Sedláček P and V Sterk (2017), ‘The Growth Potential of Startups over the Business Cycle’, The American Economic Review, 107(10), pp 3182-3210.
Stadler GW (1990), ‘Business Cycle Models with Endogenous Technology’, The American Economic Review, 80(4), pp 763–778.
Copyright and Disclaimer Notice
BLADE Disclaimer
The following Disclaimer Notice refers to data and graphs sourced from the Australian Bureau of Statistics' BLADE (Business Longitudinal Analysis Data Environment) database.
The results of these studies are based, in part, on data supplied to the ABS under the Taxation Administration Act 1953, A New Tax System (Australian Business Number) Act 1999, Australian Border Force Act 2015, Social Security (Administration) Act 1999, A New Tax System (Family Assistance) (Administration) Act 1999, Paid Parental Leave Act 2010 and/or the Student Assistance Act 1973. Such data may only be used for the purpose of administering the Census and Statistics Act 1905 or performance of functions of the ABS as set out in section 6 of the Australian Bureau of Statistics Act 1975. No individual information collected under the Census and Statistics Act 1905 is provided back to custodians for administrative or regulatory purposes. Any discussion of data limitations or weaknesses is in the context of using the data for statistical purposes and is not related to the ability of the data to support the Australian Taxation Office, Australian Business Register, Department of Social Services and/or Department of Home Affairs' core operational requirements.
Legislative requirements to ensure privacy and secrecy of these data have been followed. For access to MADIP and/or BLADE data under Section 16A of the ABS Act 1975 or enabled by section 15 of the Census and Statistics (Information Release and Access) Determination 2018, source data are de-identified and so data about specific individuals has not been viewed in conducting this analysis. In accordance with the Census and Statistics Act 1905, results have been treated where necessary to ensure that they are not likely to enable identification of a particular person or organisation.
Acknowledgements
We are grateful to Matt Read, Beth Webster, Petr Sedláček, John Simon and Tim Taylor, and seminar participants at the Tax and Transfer Policy Institute (ANU) and RBA for their comments on the paper. The views expressed in this paper are those of the authors and do not necessarily reflect the views of the Reserve Bank of Australia. The authors are responsible for any errors.
Footnotes
Economic Research Department, Reserve Bank of Australia [*]
Crawford School of Public Policy, Australian National University [**]
Baqaee, Farhi and Sangani (forthcoming) propose a different mechanism whereby contractionary shocks lead to a reallocation of resources towards lower productivity firms. [1]
In a related literature, Sedláček and Ignaszak (2021) consider the role that firm-level demand can play in incentivising innovation, and in turn aggregate growth. [2]
See <https://data.gov.au/dataset/ds-dga-d61374dd-1a62-4132-913d-35d90cfdac81/details>. [3]
As a robustness test, we also estimate the models including firms in the regression for horizons 0, 1 and 2 only if we could observe their innovation at horizon 3. This ensures that the share of large and small firms remains balanced. The results by firm size are similar to our baseline, though there is slightly more evidence of an immediate effect. [4]
For a chart of this shock measure see Figure A1. [5]
The results are robust to including more or fewer lags of the RHS variables, as well as to including the contemporaneous controls, which imposes the implicit assumption that monetary policy cannot affect current conditions (Ramey 2016). Exclusion of the control for age does not affect the results. [6]
We experimented with a VAR identified using timing restrictions as in Moran and Queralto (2018). This provided no evidence of a significant effect on R&D spending, likely reflecting the strong (and likely inaccurate) identification assumption. [7]
Note that the unconditional standard deviation is much larger than the standard deviation of the structural R&D shock from the small VAR discussed later, suggesting much of the volatility reflects factors such as aggregate demand. [8]
The response may seem large relative to how much the share of innovating firms in the economy varies. But it is important to keep in mind that a 100 basis point monetary policy shock is larger than any shock which has occurred in the sample. [9]
This measure is based on high-frequency changes in bond yields. We use this measure as it is available for a wide range of countries, which could facilitate future analysis. [10]
The survey question asks firms about what factors are hampering their innovative activity. As well as a lack of access to additional funds, firms can note: lack of skilled workers; cost of development; regulations and compliance; or uncertain demand for new goods/services. Firms can select more than one factor. [11]
That said, the global cost of capital may affect domestic costs. [12]
We experimented with looking directly at the effects of monetary policy shocks on productivity. We were unable to identify any effects. However, this likely reflects the lack of quarterly data on TFP, resulting in our estimates being based on a small number of observations with annually aggregated shocks. [13]